基于贝叶斯理论的分类问题
【摘要】 随着科技的发展现在世界上已经有用着许多种类的分类器,然而所有的分类器都逃不过准确度这一个关键点,准确度越高的分类器能提供越精准的分类成果,而贝叶斯分类器恰巧是现有的所有的分类器准确度较高的能极大程度避免误差的一个分类器;也可以说是在有已知的条件的状况下进行分类后会能够极大程度的减少产生平均风险的一个分类器。
在这个信息时代,每天都有着大量的信息产生与传递,人们对信息的筛选和分类的问题更加精确,所以在本次实验中将使用朴素贝叶斯分类器进行实验。所以本次实验将通过给予大量的数据并对此使用监督性学习的方法进行分类与学习,并且本次实验将选取多组不同的数据进行分类,并且通过对比后的出结论;同时将使用不同的分类器模型与之对比,最后通过分别得出他们的分类精度并进行对比,通过所得的结果进行分析得到朴素贝叶斯分类器的优势。
【关键词】 朴素贝叶斯,SVM,监督性学习,分类问题
E-Commerce Product Review Sentiment Classification Based on a Naive Bayes Continuous Learning Framework
【Abstract】 Discriminative classifiers tend to have lower asymptotic classification errors, while generative classifiers can be more accurate when the training set size is small. In this paper, we examine the construction of hybrid models from categorical data, where we use logistic regression (LR) as a discriminative component, and naïve Bayes (NB) as a generative component. We adopt a bias-variance tradeoff based strategy, with the objective of minimizing the sum of these two errors. Specifically, the proposed heuristic consists of functions of training sample size and conditional dependence among features. These functions serve as proxies for model variance and model bias. We implement our method on 25 different classification datasets, and find that the hybrid model does better than pure LR and pure NB. Our proposed method is competitive with random forest. Although the hybrid model fails to beat LASSO in predictive performance, as suggested by the experimental results, the difference appears to be insignificant when the number of features is small.
【Key Words】 Naïve Bayes,Logistic regression,Hybrid discriminative-generative model,Bias-variance strategy,Model construction heuristic
目 录
1 绪 论
1.1 选题背景及研究意义
1.2 国内外研究现状
1.3 论文研究思路及方法
1.2 论文结构安排
2分类与分类器
2.1 分类及分类器概念
2.1.1 分类
2.1.2 分类器
2.2 性能评价指标
2.3 准确度评价方法
2.4 小结
3贝叶斯理论与分类器
3.1 条件概率和乘法定理
3.2 全概率公式与贝叶斯公式
3.3 极大似然值估计及后验假设
3.3.1 极大后验假设
3.3.2 极大似然估计
3.4 朴素贝叶斯分类模型
3.5 SVM模型
3.6朴素贝叶斯分类模型优缺点
3.7 小结
4 分类实验
4.1分类模型
4.2 Monks3分类
4.2.1 朴素贝叶斯分类
4.2.2 SVM分类
4.3 House分类
4.3.1 朴素贝叶斯分类
4.3.2 SVM分类
4.4 Bupa分类
4.4.1 朴素贝叶斯分类
4.3.2 SVM分类
4.5 Wine分类
4.5.1 朴素贝叶斯分类
4.5.1 SVM分类
4.5 小结
4 分析与展望
4.1 分析
4.2 展望
4.2.1 应用
4.2.2 不足与发展
结 论
参考文献
附 录
致 谢
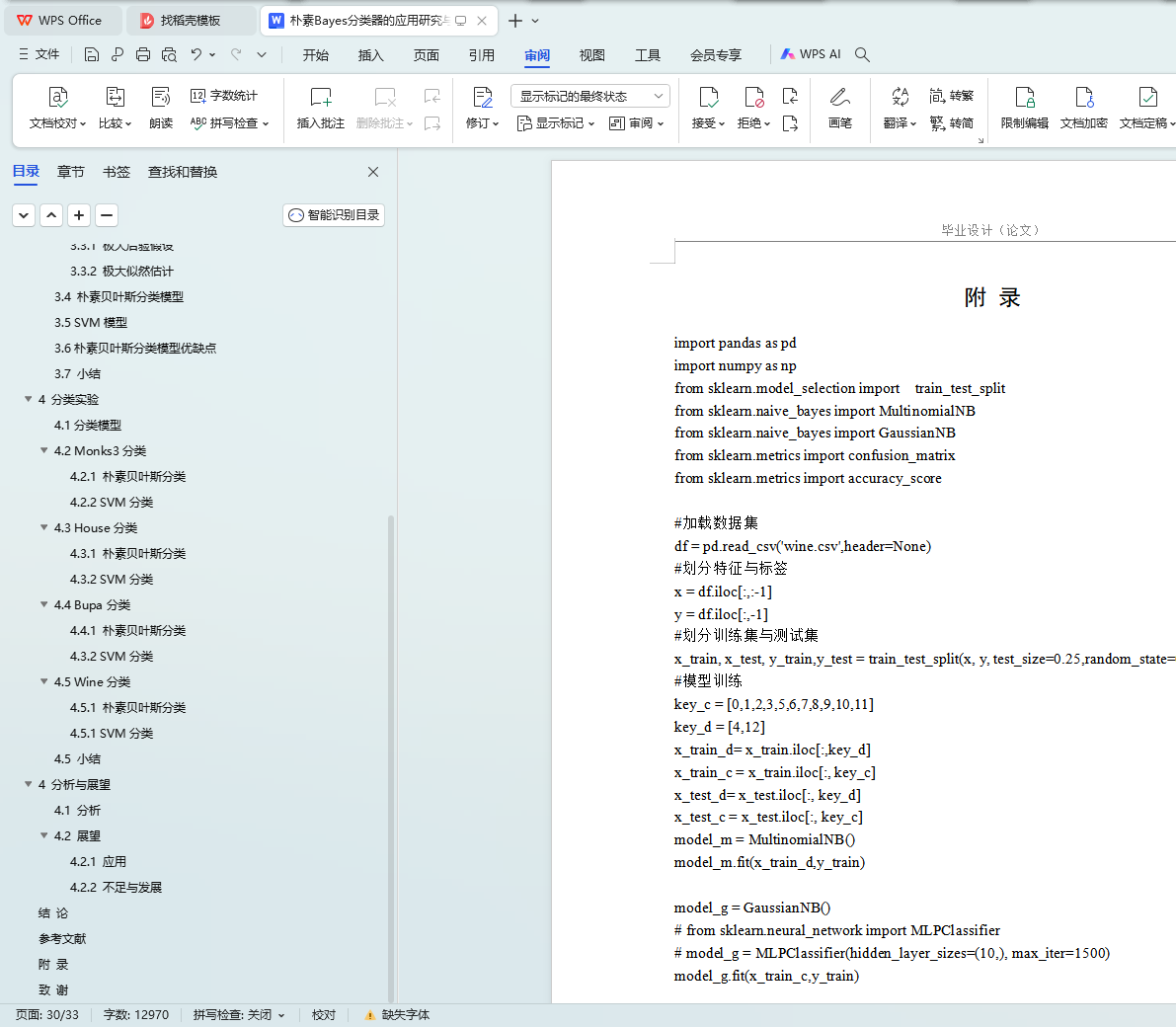
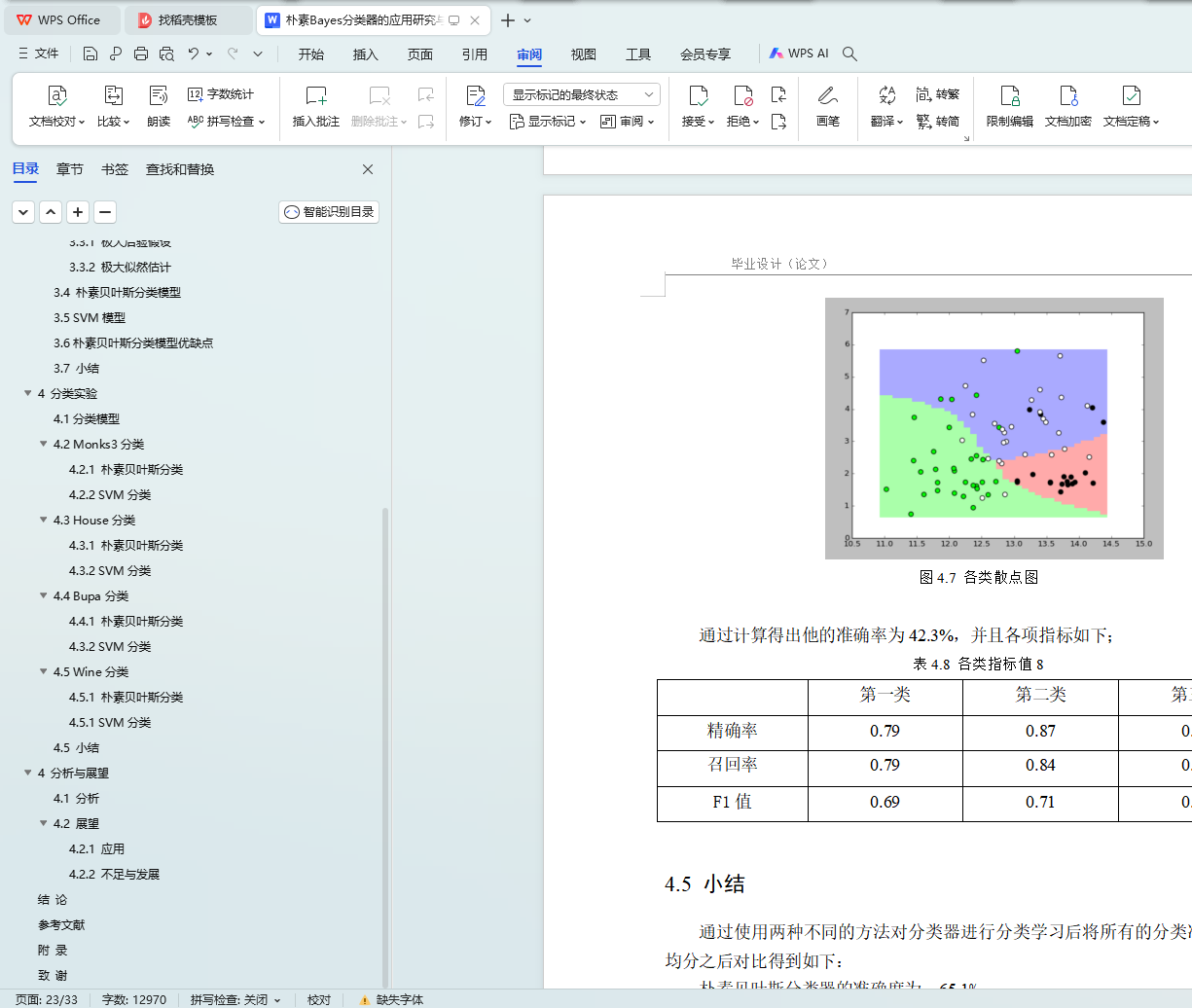
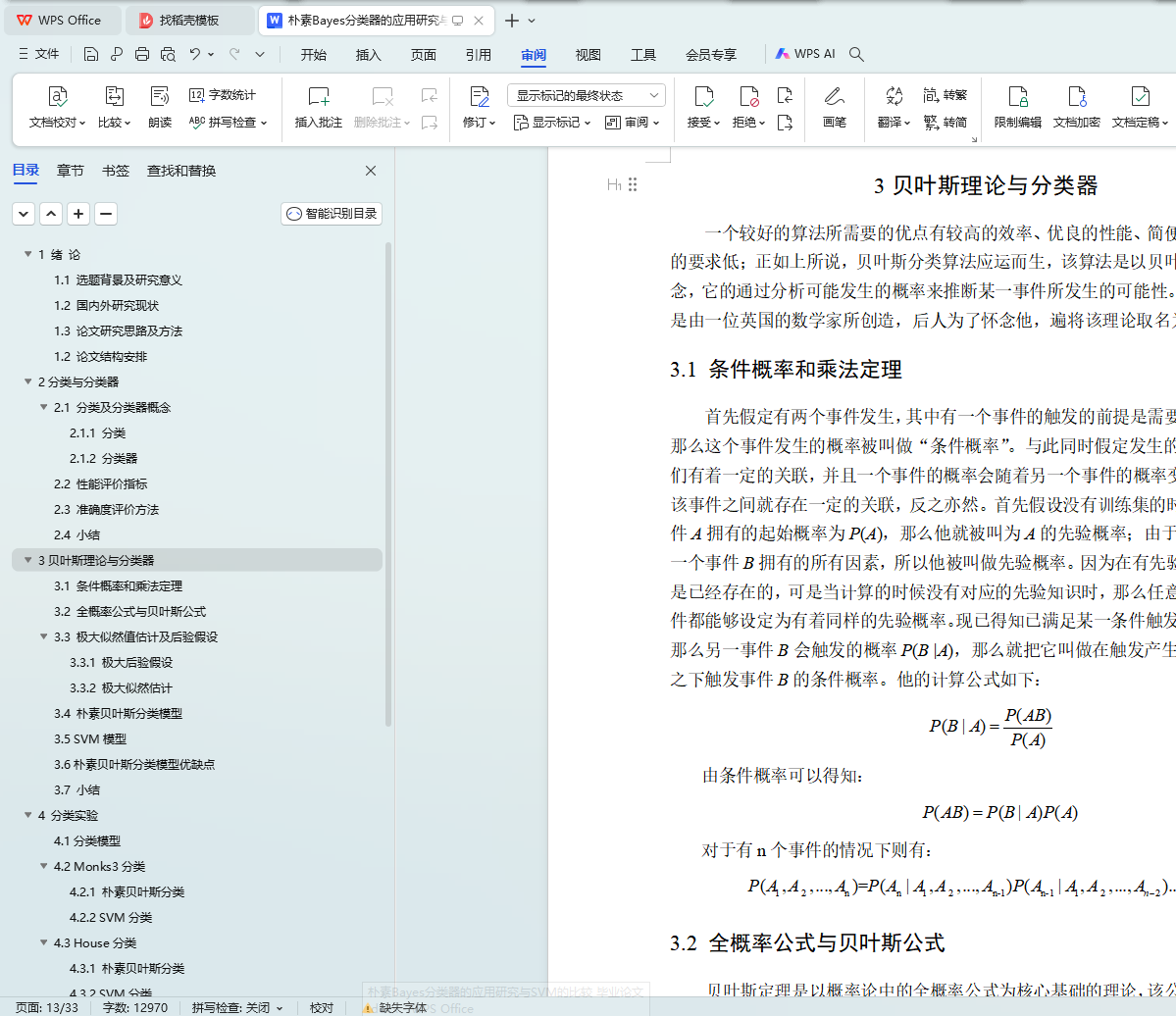
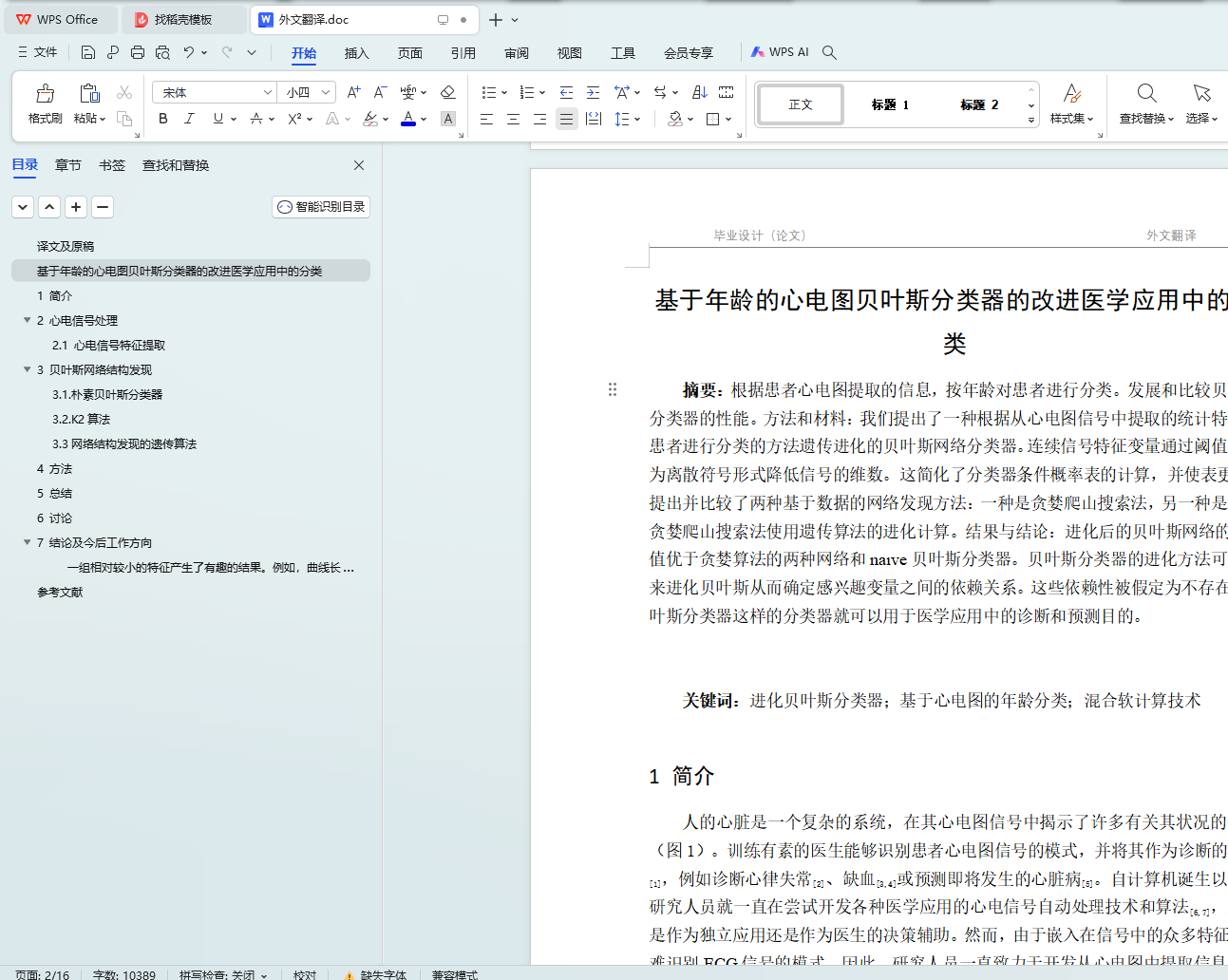
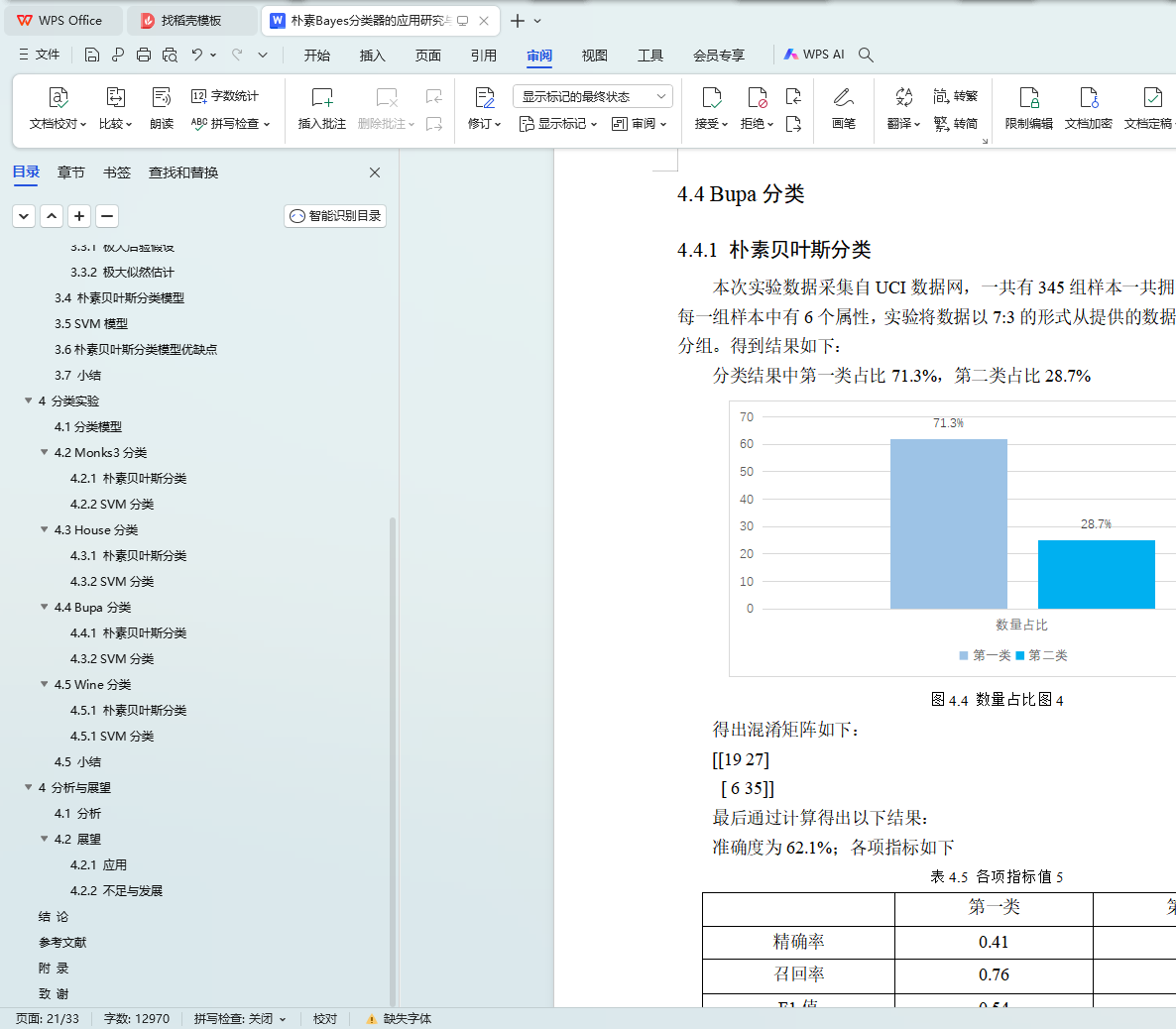
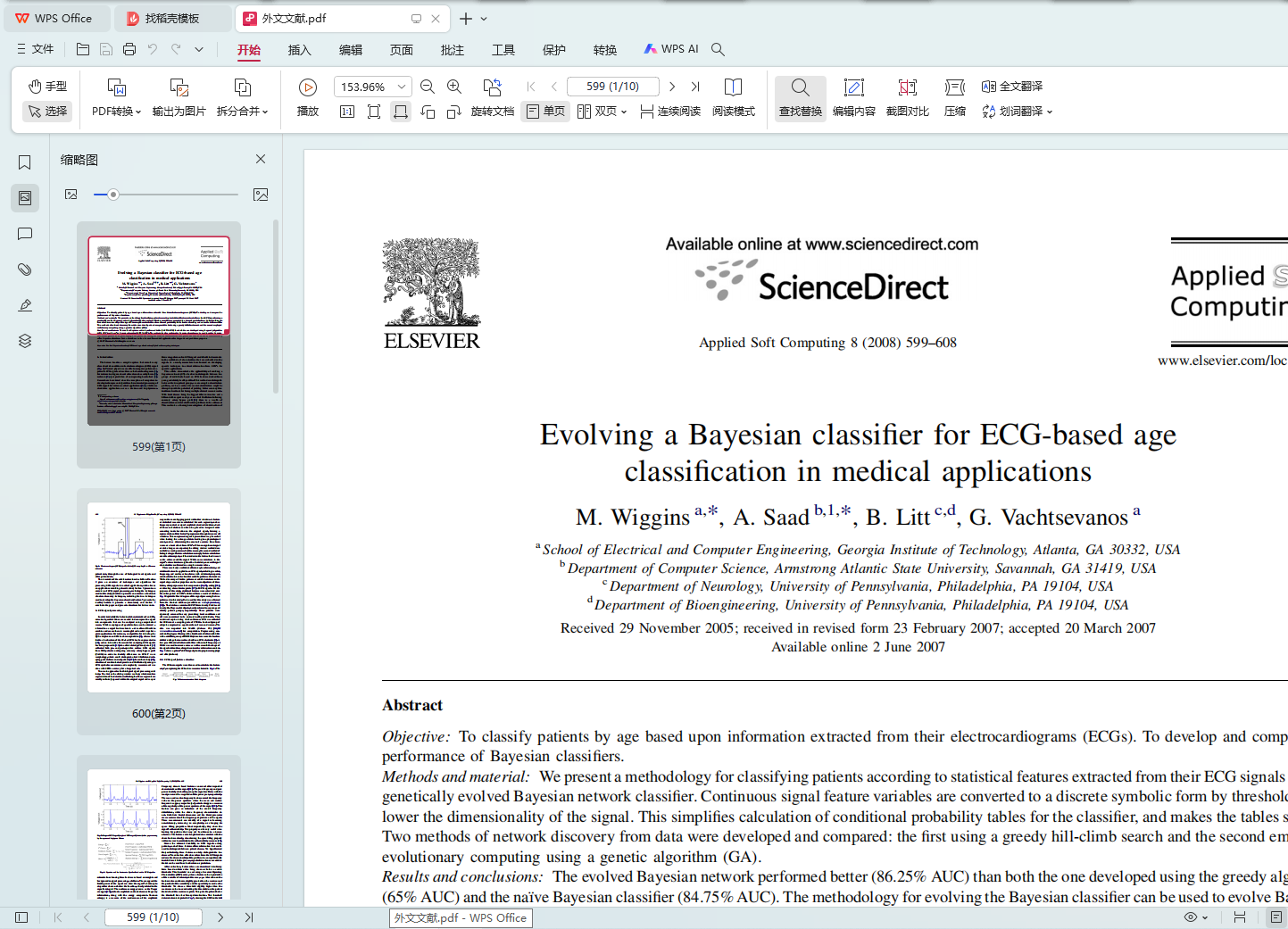
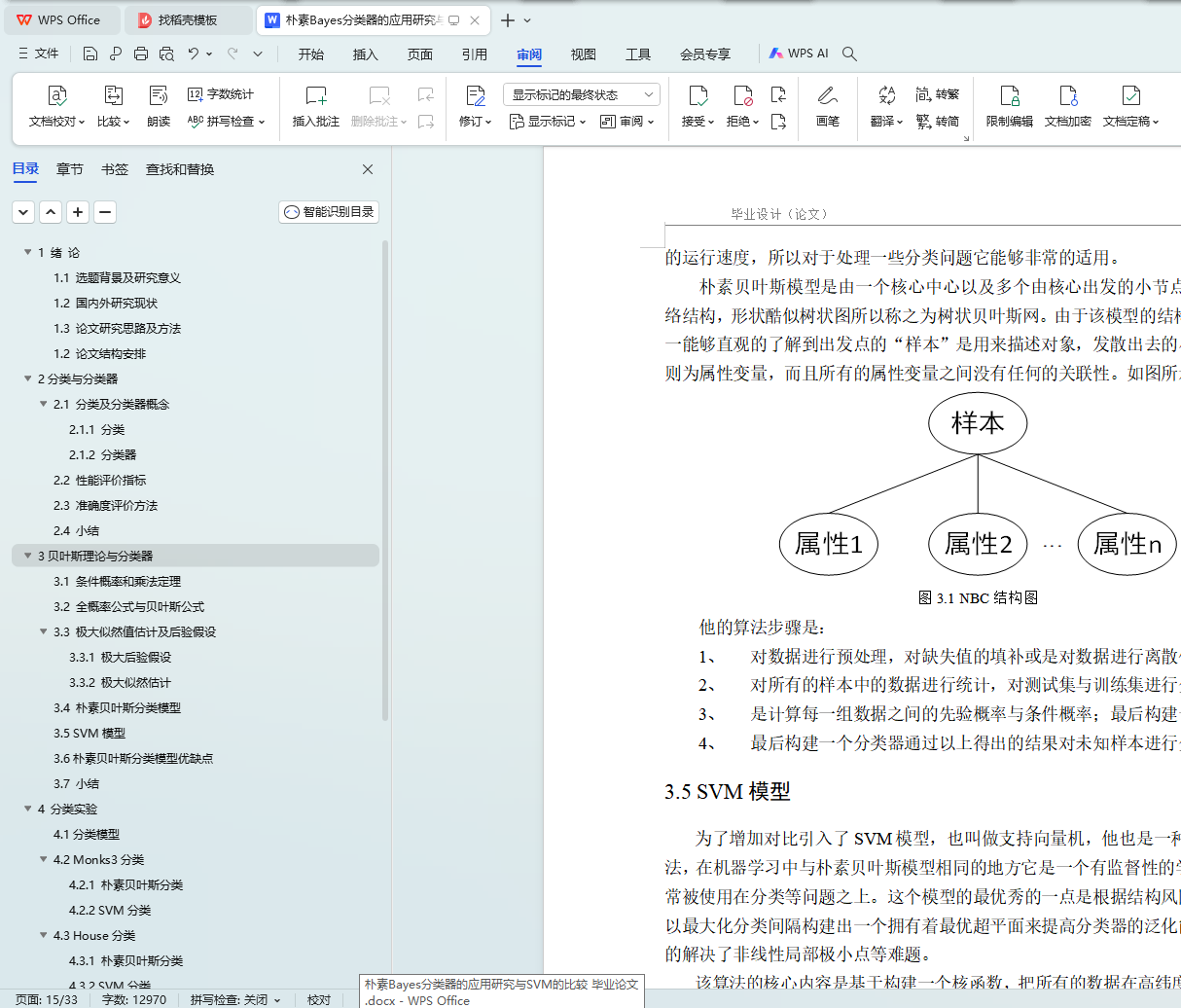
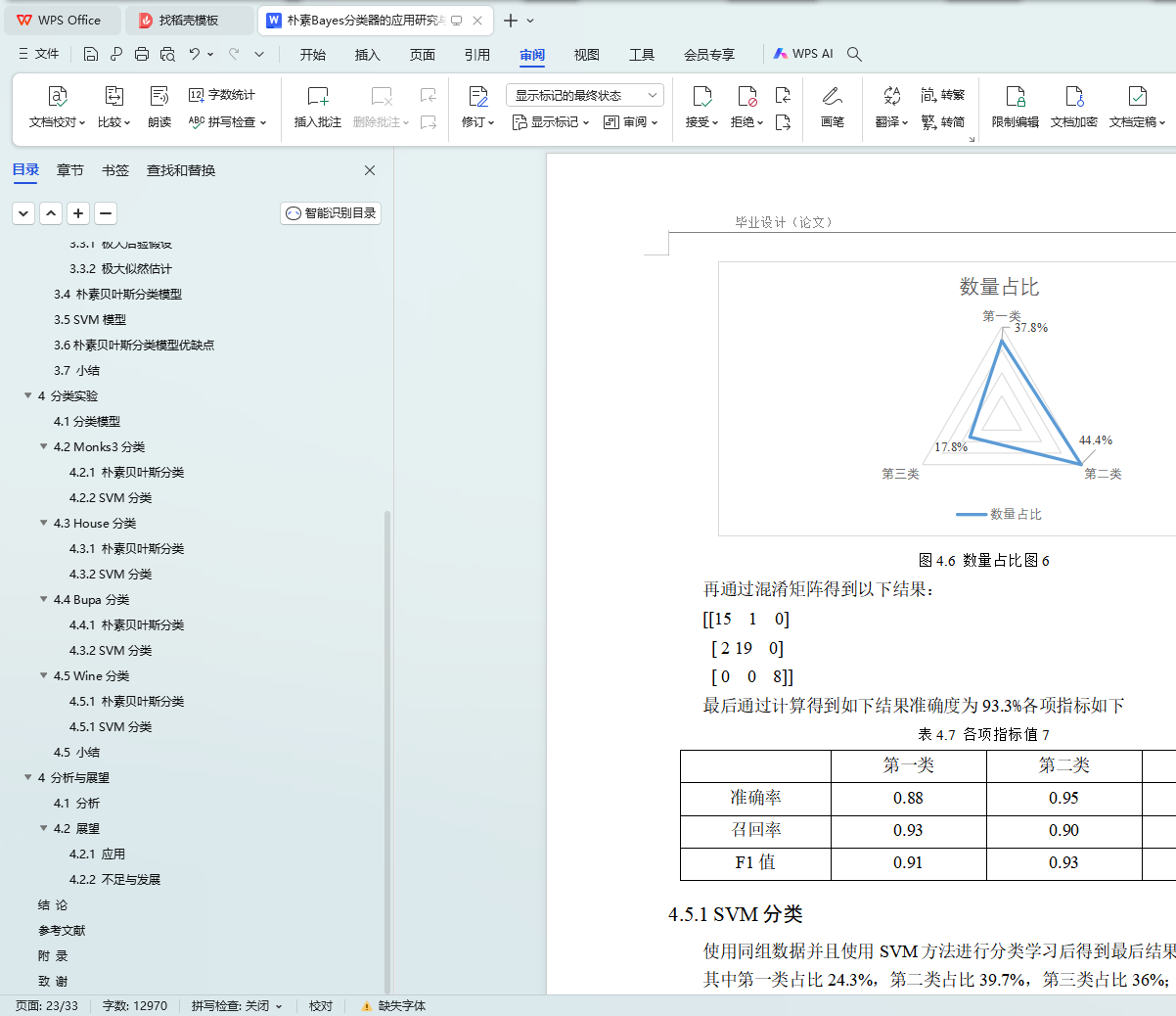
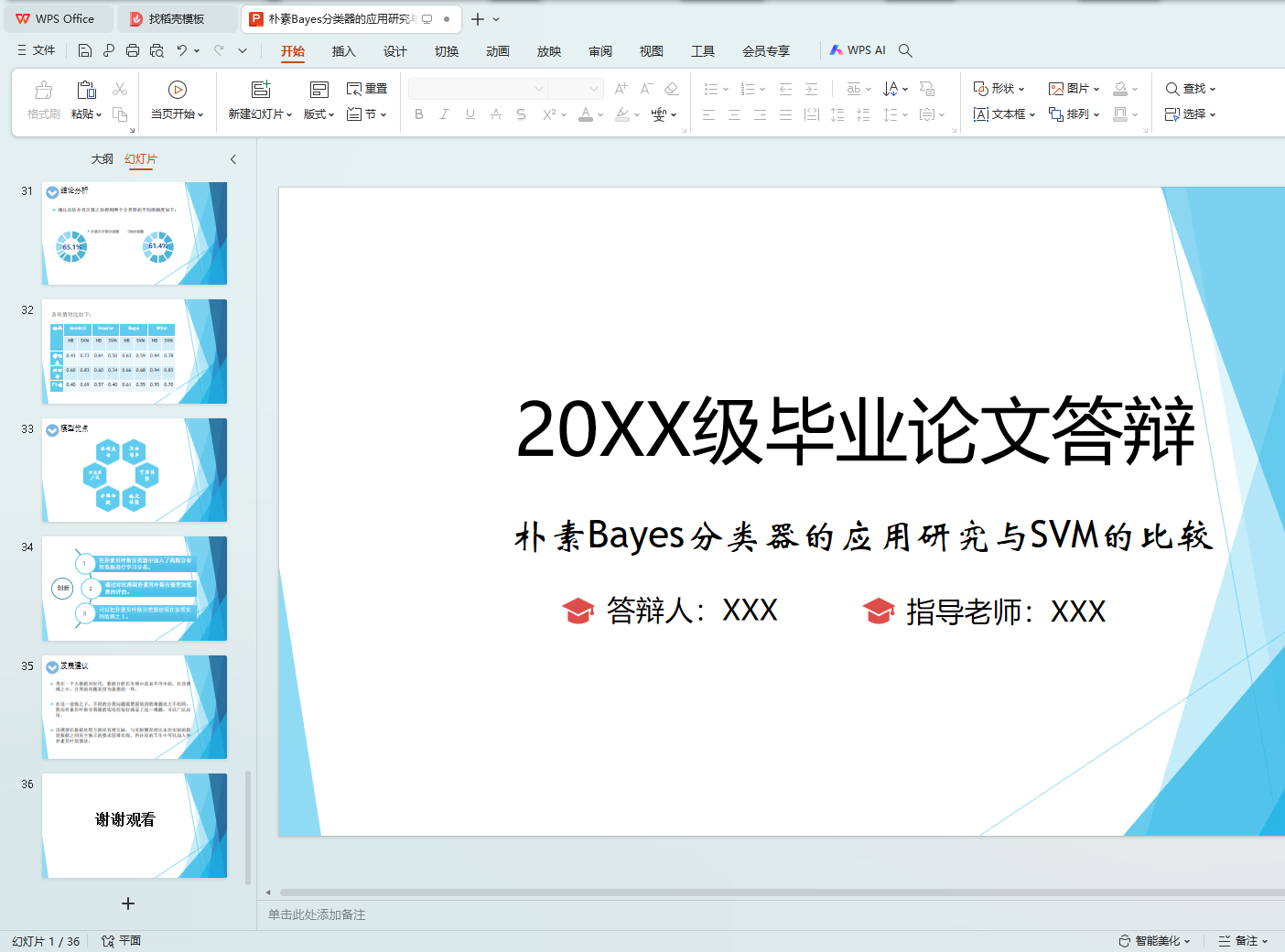
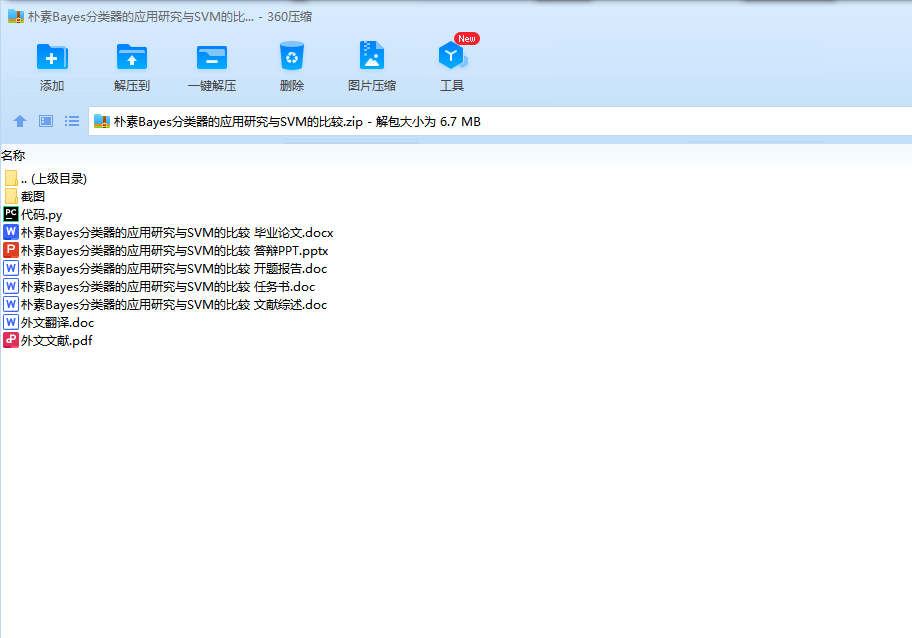
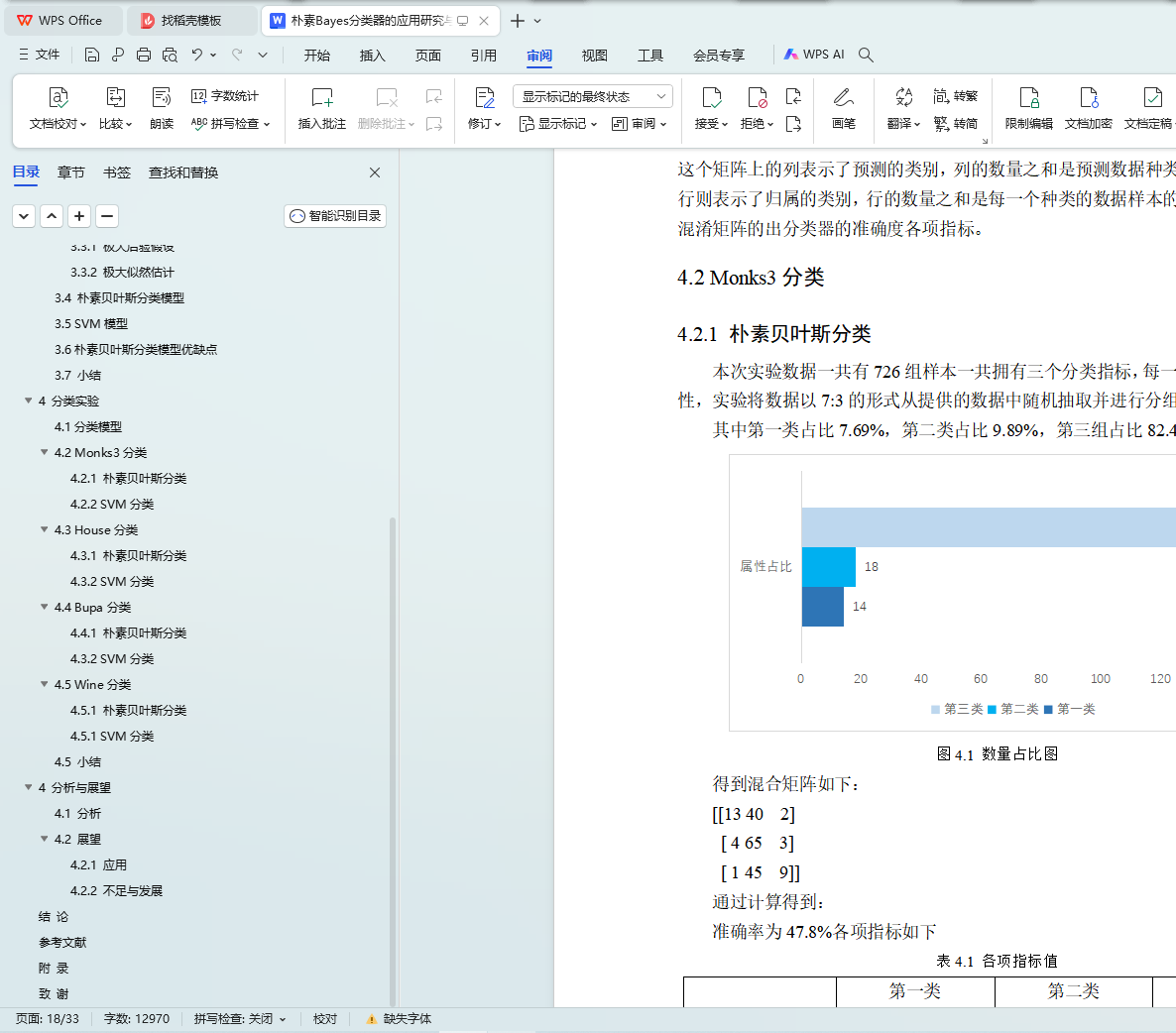
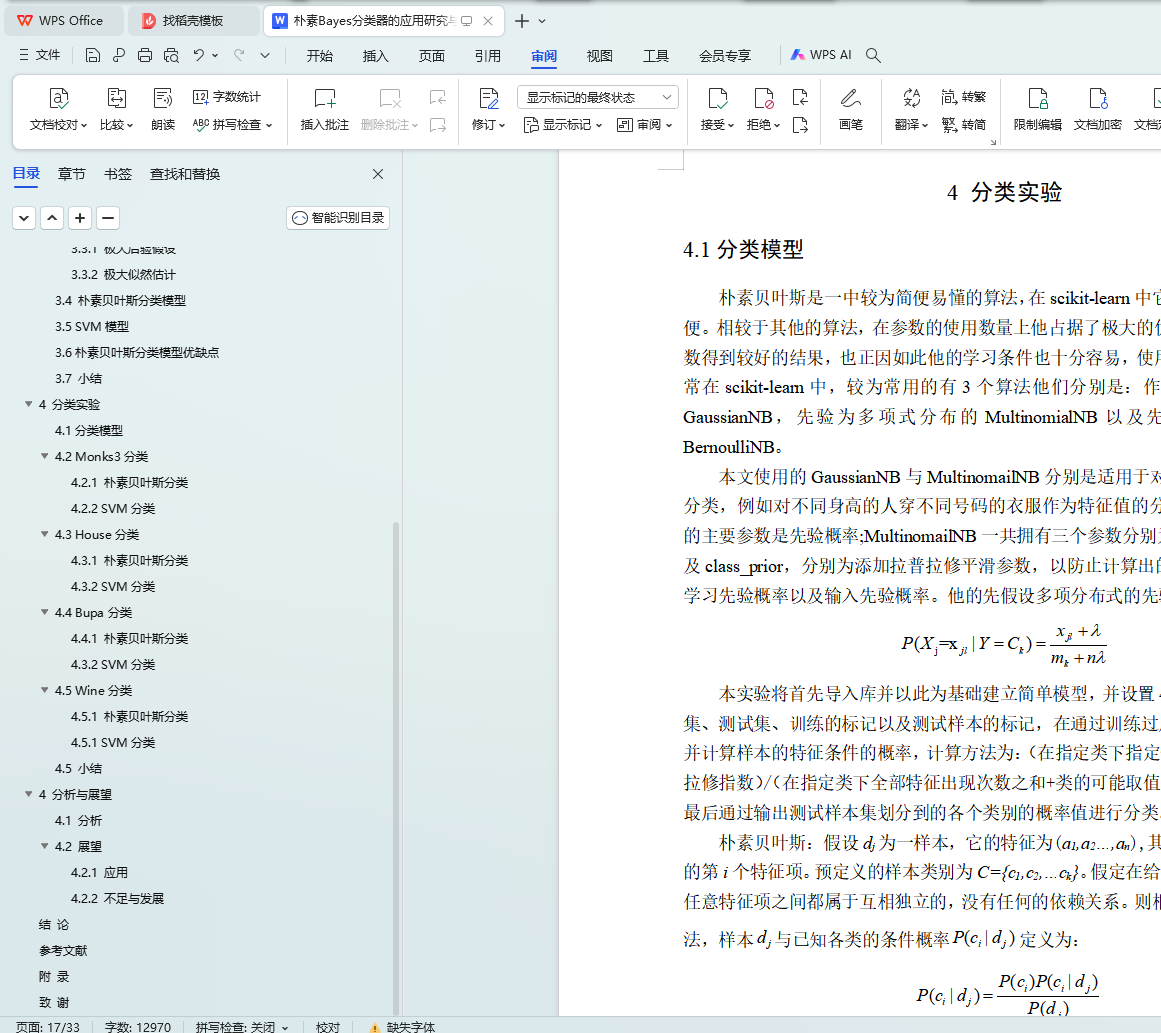
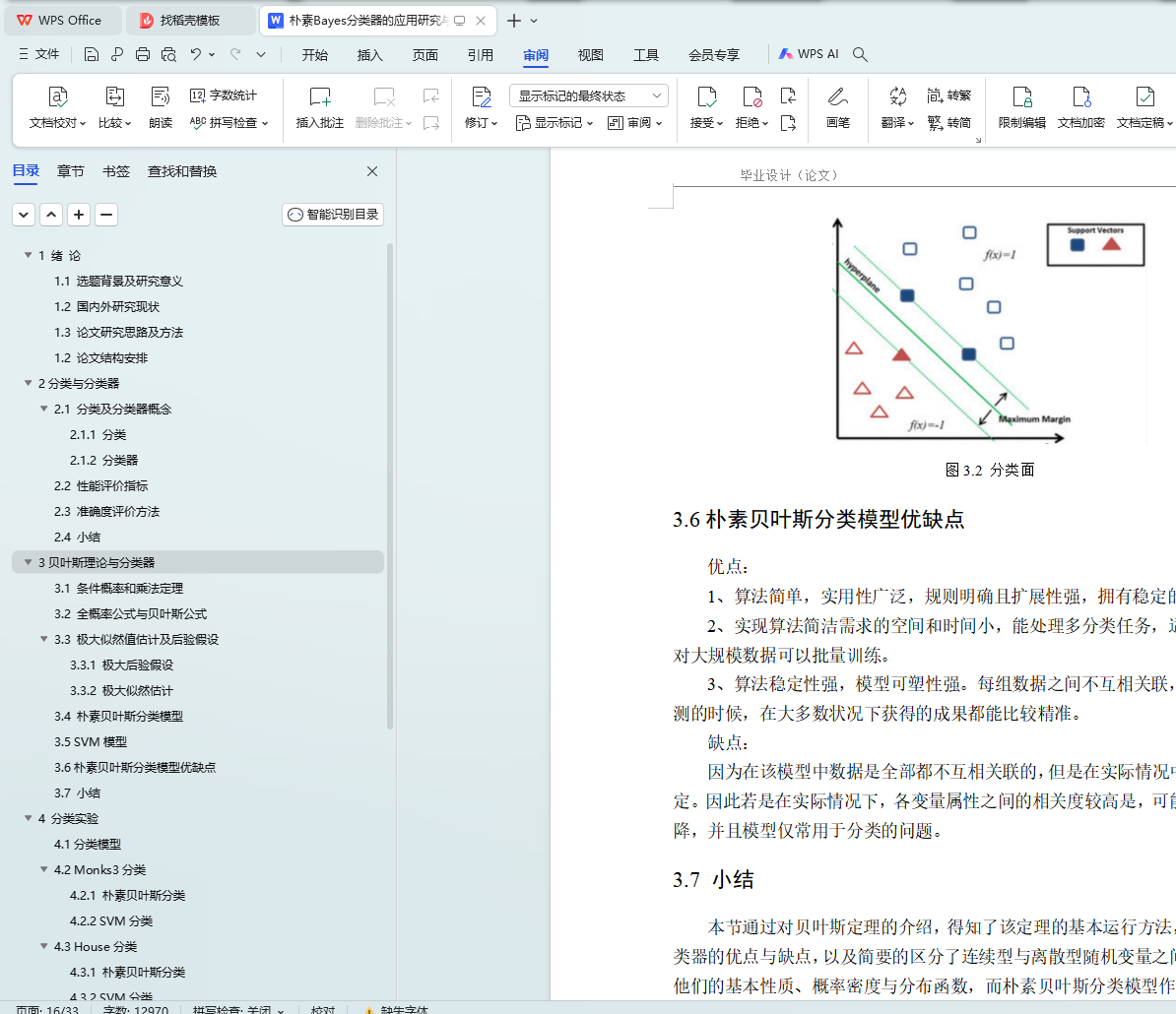
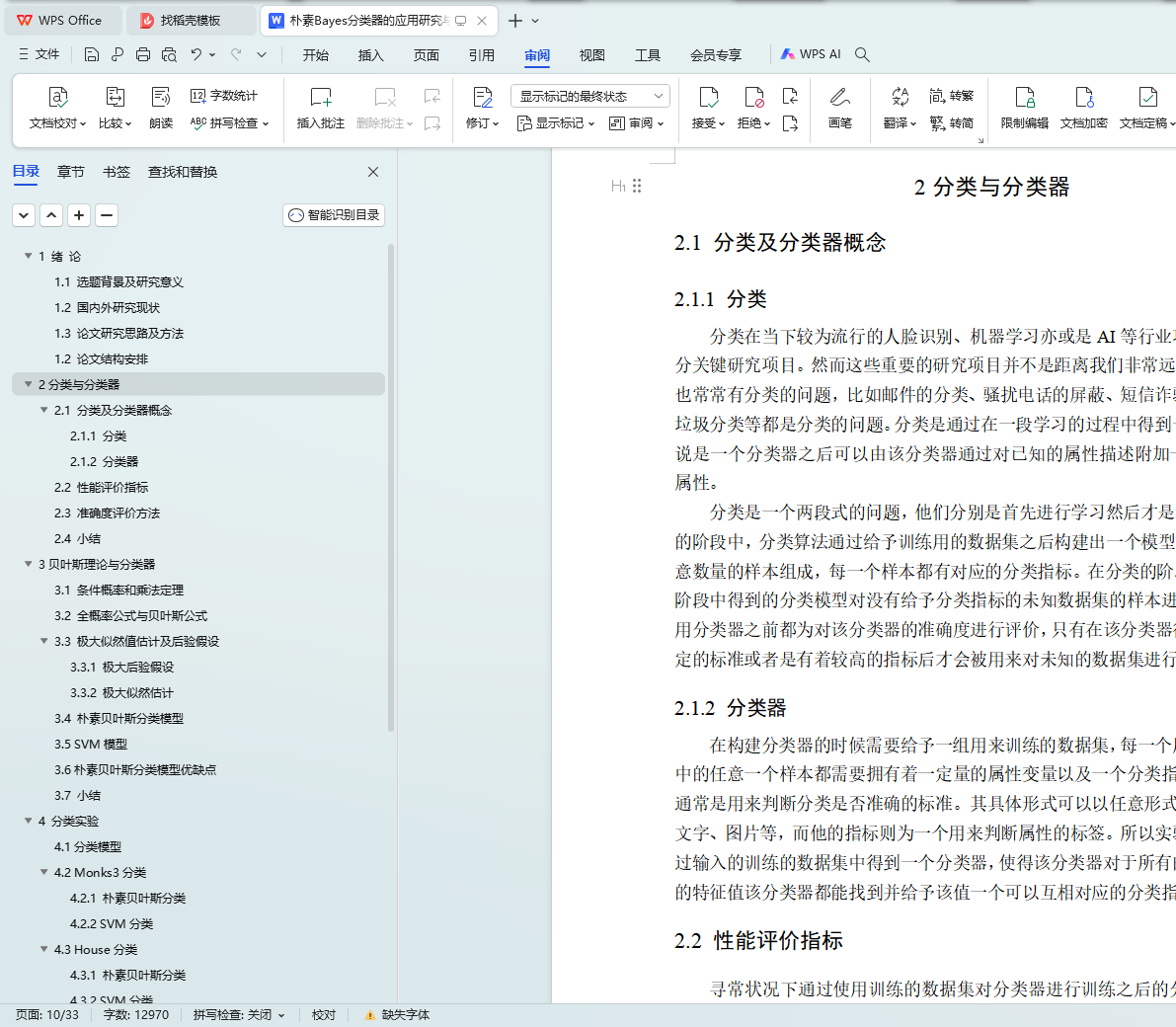
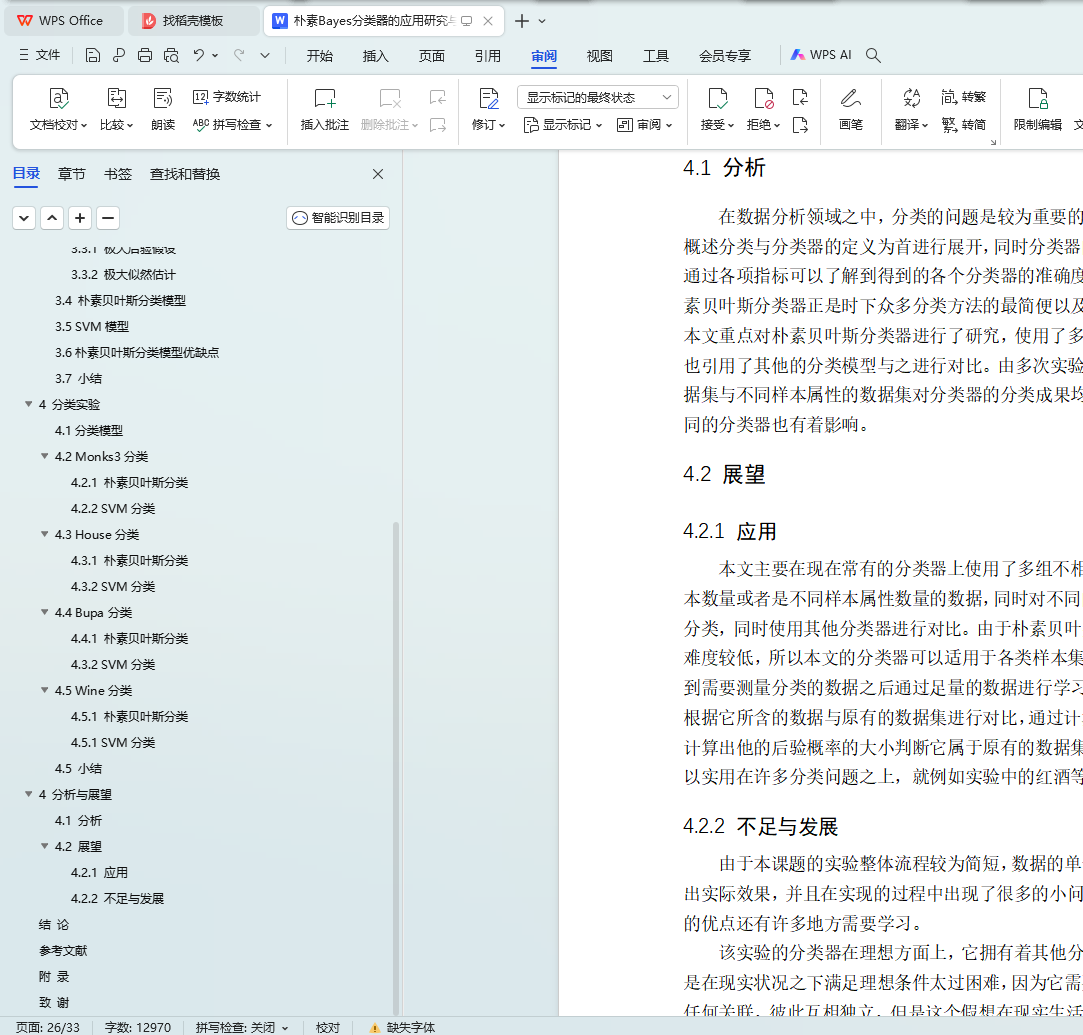
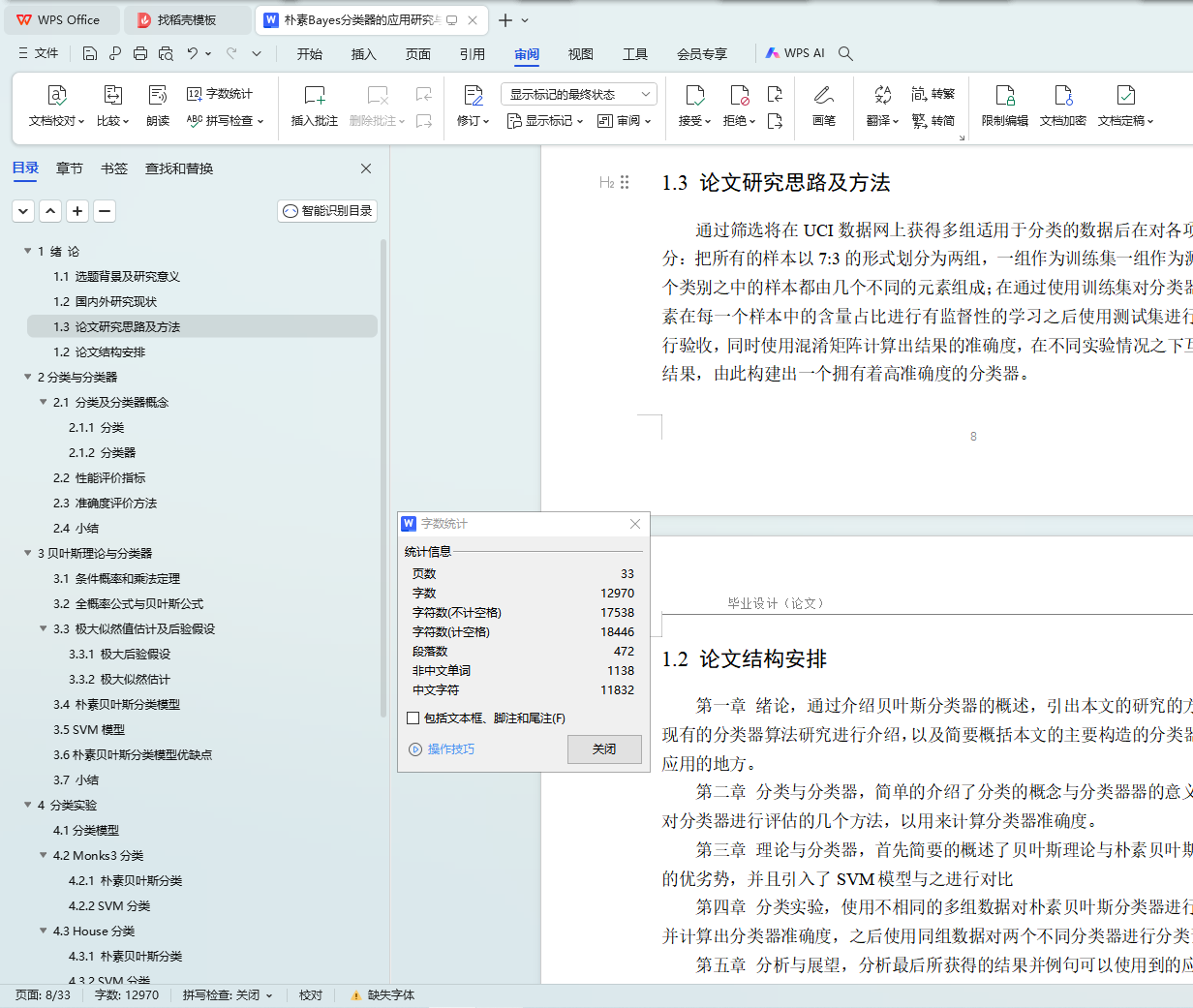
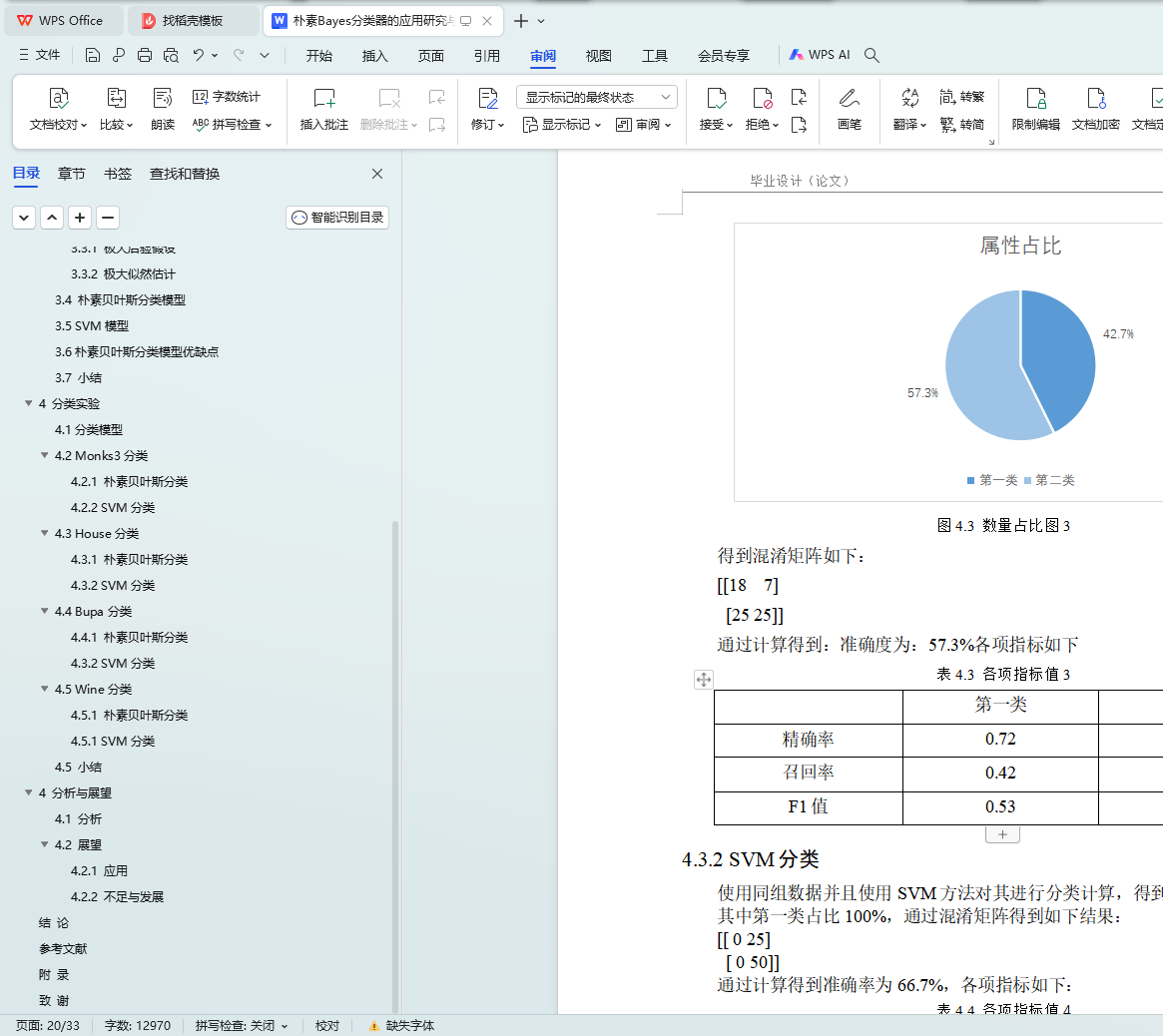