包含词性的朴素Bayes分类在文本分类中的应用
【摘要】 由于国内网络时代的迅速发展,我们可以在网络上获得大量的数据。为了更好的对数据进行处理,我们可以依据词性对文本进行分类处理。本篇论文的研究重点是分析了朴素贝叶斯算法对基于词性的文本分类的应用,然后对使用了朴素贝叶斯的文本分类模型进行了论述,在此基础上通过搭建给予python的文本分类平台,进行了实验准备和数据分类,经过实验数据表明本论文提出朴素Bayes分类算法具有较好的分类效率和性能表现。
【关键词】 朴素贝叶斯算法,文本分类,中文分词,算法设计
——
【Abstract】 With the rapid development of the Internet age, people can get a lot of data through the Internet. In order to improve this situation, text can be classified. This paper studies the application of naive Bayes algorithm in text classification, gives the basic principle of text classification technology and naive Bayes algorithm, and discusses the text classification model of naive Bayes. Based on this, a text classification platform for python was built, and experimental preparation and data classification were carried out. The experimental data showed that the naive Bayes classification algorithm proposed in this paper has good classification efficiency and performance.
【Key Words】 Naive Bayes algorithm, text classification, Chinese word segmentation, algorithm design
目 录
1 绪 论
1.1 研究背景及意义
1.1.1 研究背景
1.1.2 研究意义
1.2 研究现状
1.3 研究内容与结构
2文本分类技术与朴素贝叶斯原理
2.1 数据预处理
2.1.1 中文分词
2.1.2 去除停用词
2.1.3 特征选择
2.2 文本分类的种类及算法
2.2.1 朴素贝叶斯算法
2.2.2 Logistic回归分析
2.2.2 KNN算法
2.3 朴素贝叶斯分类器
2.3.1 朴素贝叶斯的原理推导
2.3.2 朴素贝叶斯算法
3 基于朴素贝叶斯的文本分类
3.1 朴素贝叶斯分类流程
3.1.1 TFIDF特征选择
3.1.2 朴素贝叶斯模型计算流程
3.2基于朴素贝叶斯的文本分类模型
4 实验结果与分析
4.1实验环境
4.2实验数据及结果评价指标
4.2.1 数据来源
4.2.2 评价指标
4.3语料库预处理
4.3.1 非中文字符过滤
4.3.2 去停用词表
4.3.3 文本分词
4.4 实验步骤
4.4.1 数据准备
4.4.2 特征向量化
4.4.3 分类模型训练
4.4.4 实验结果分析
结 论
参考文献
附录
致 谢
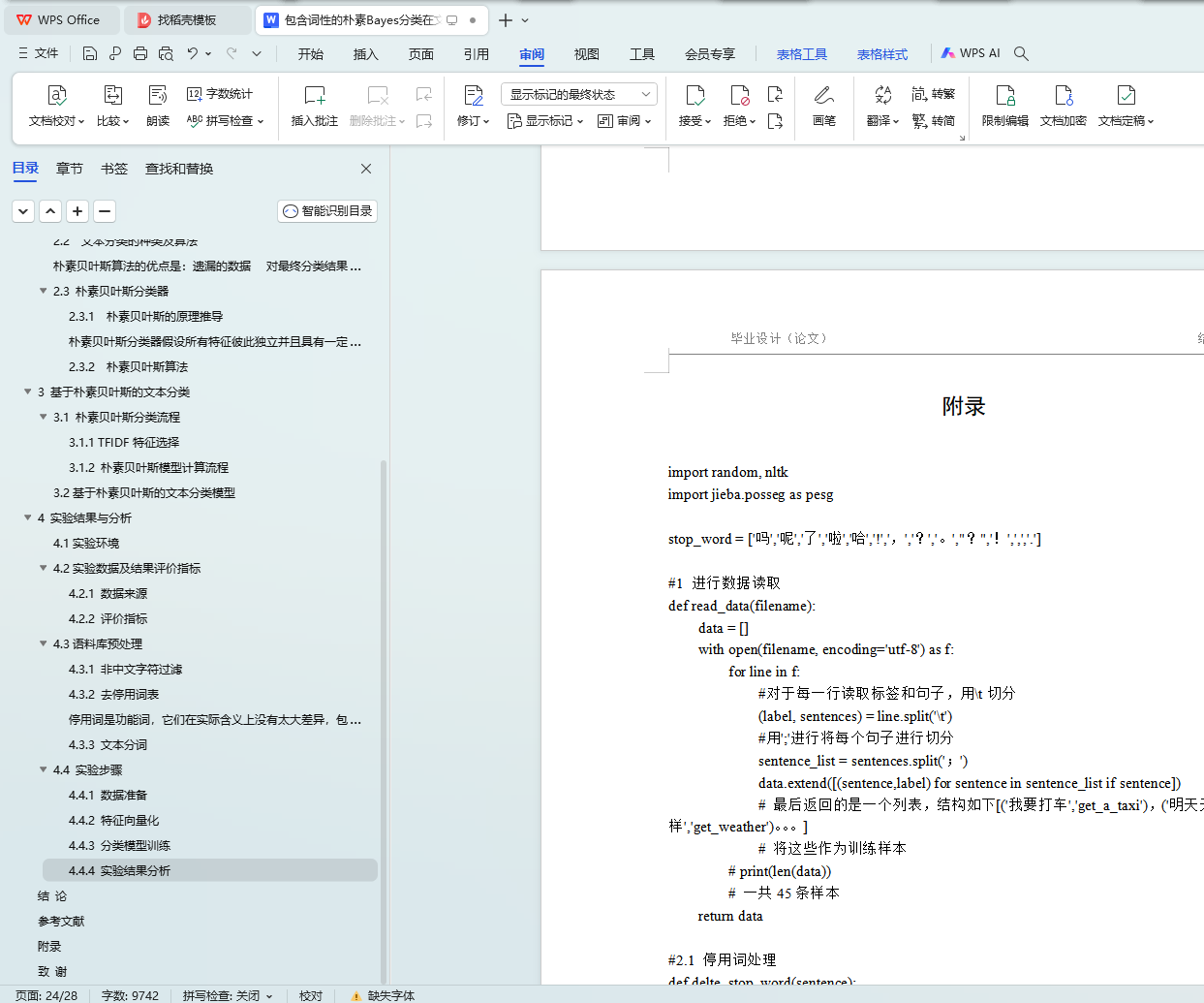
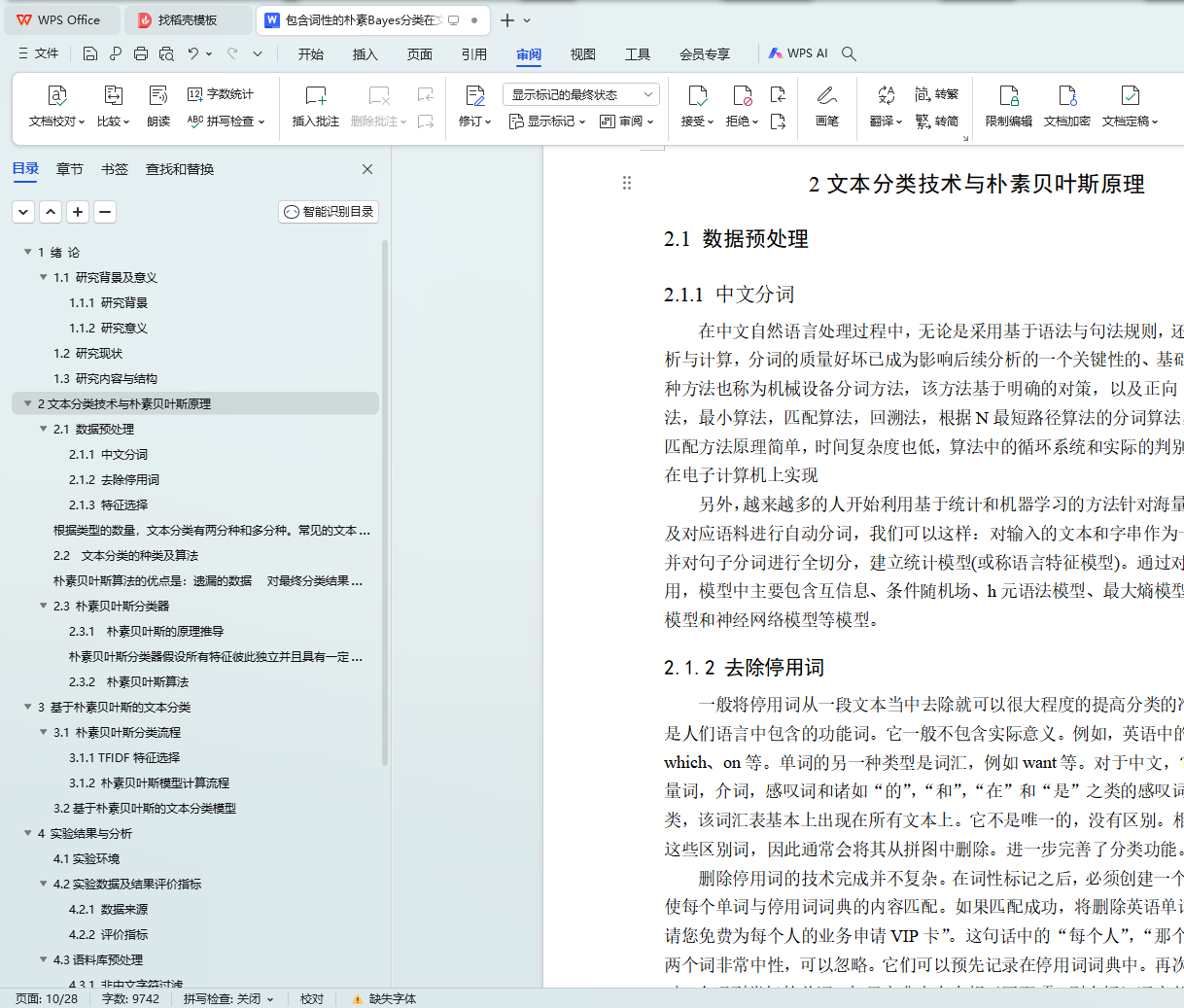
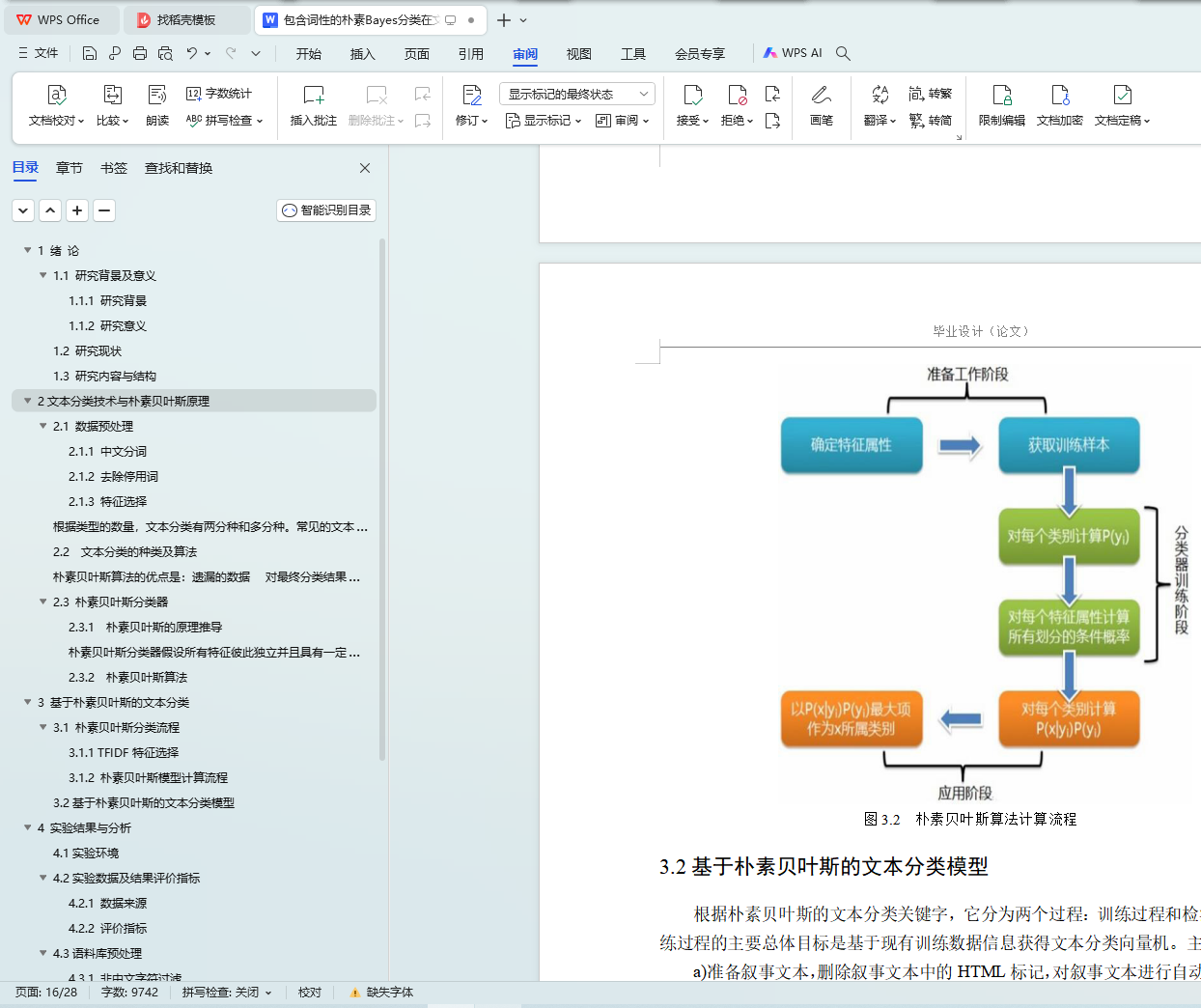
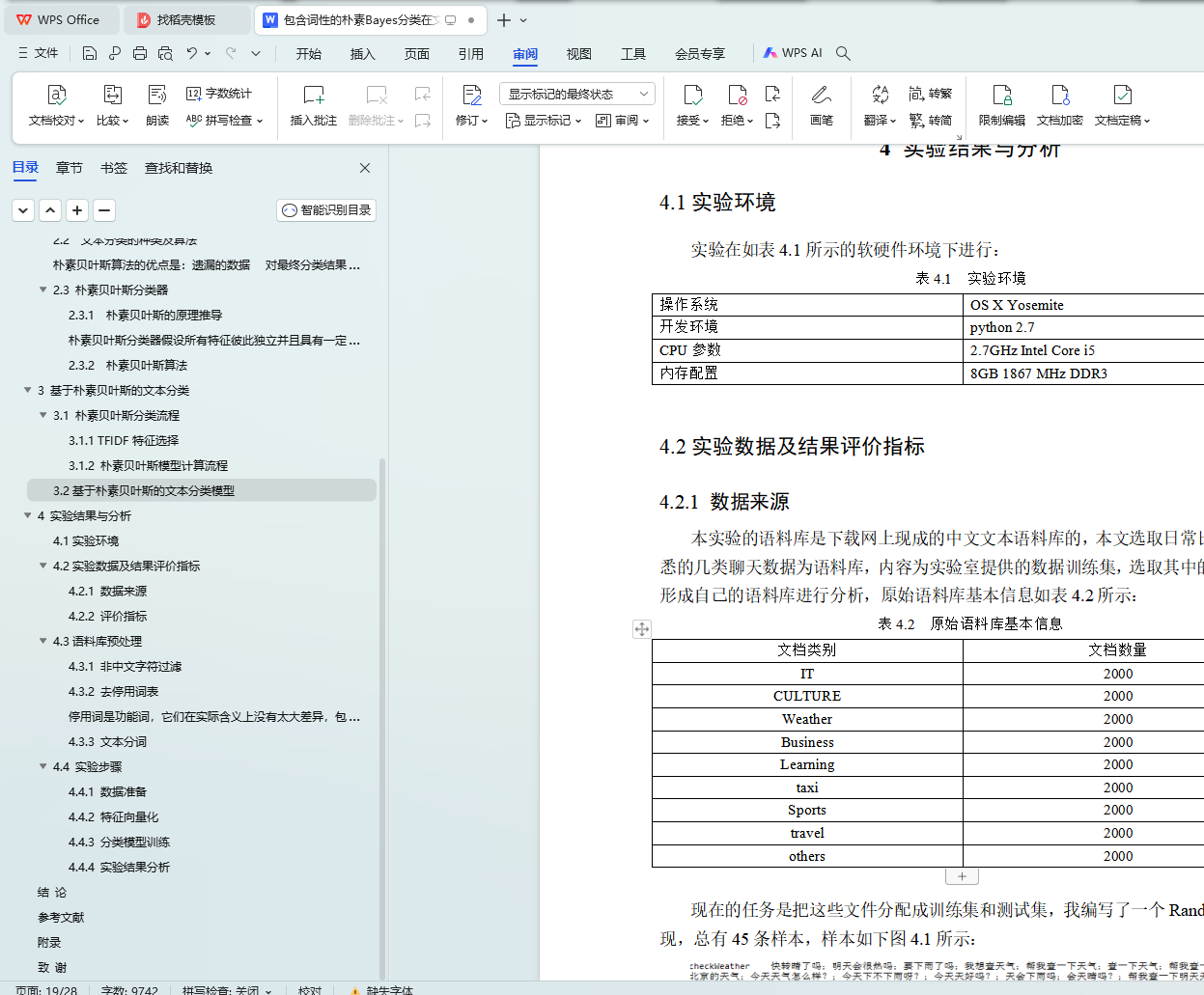
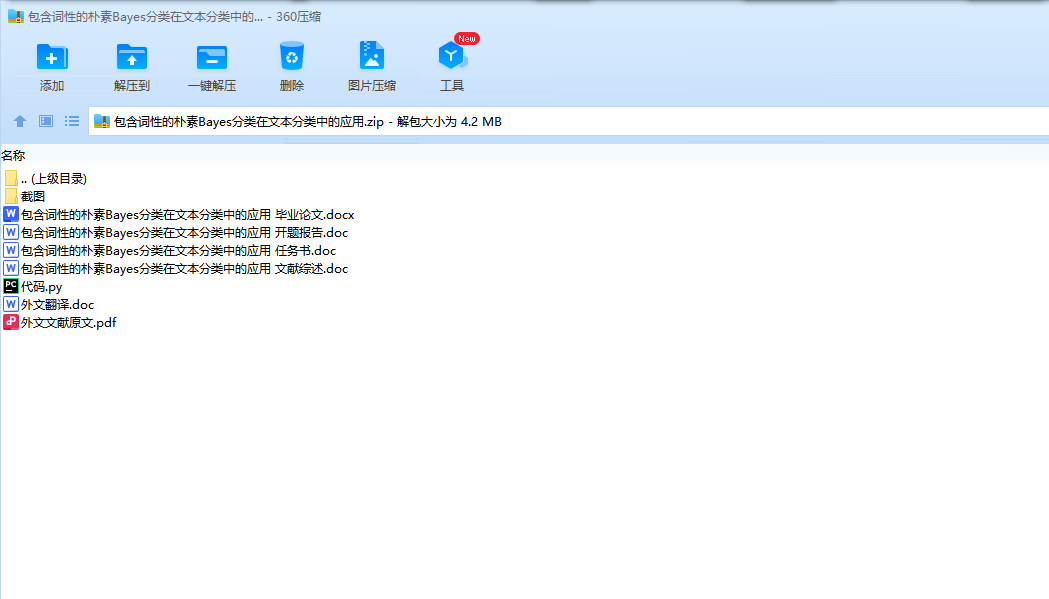
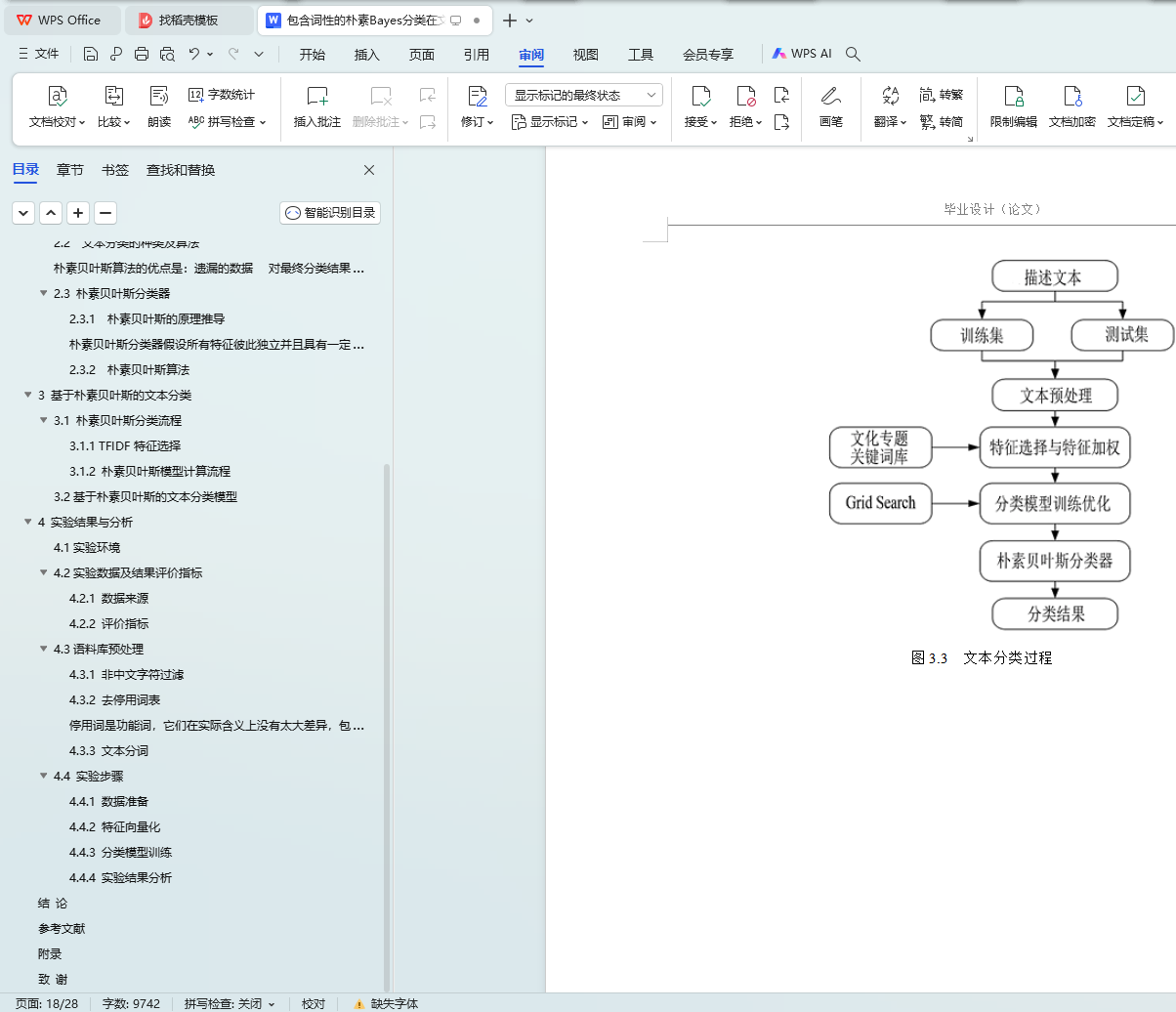
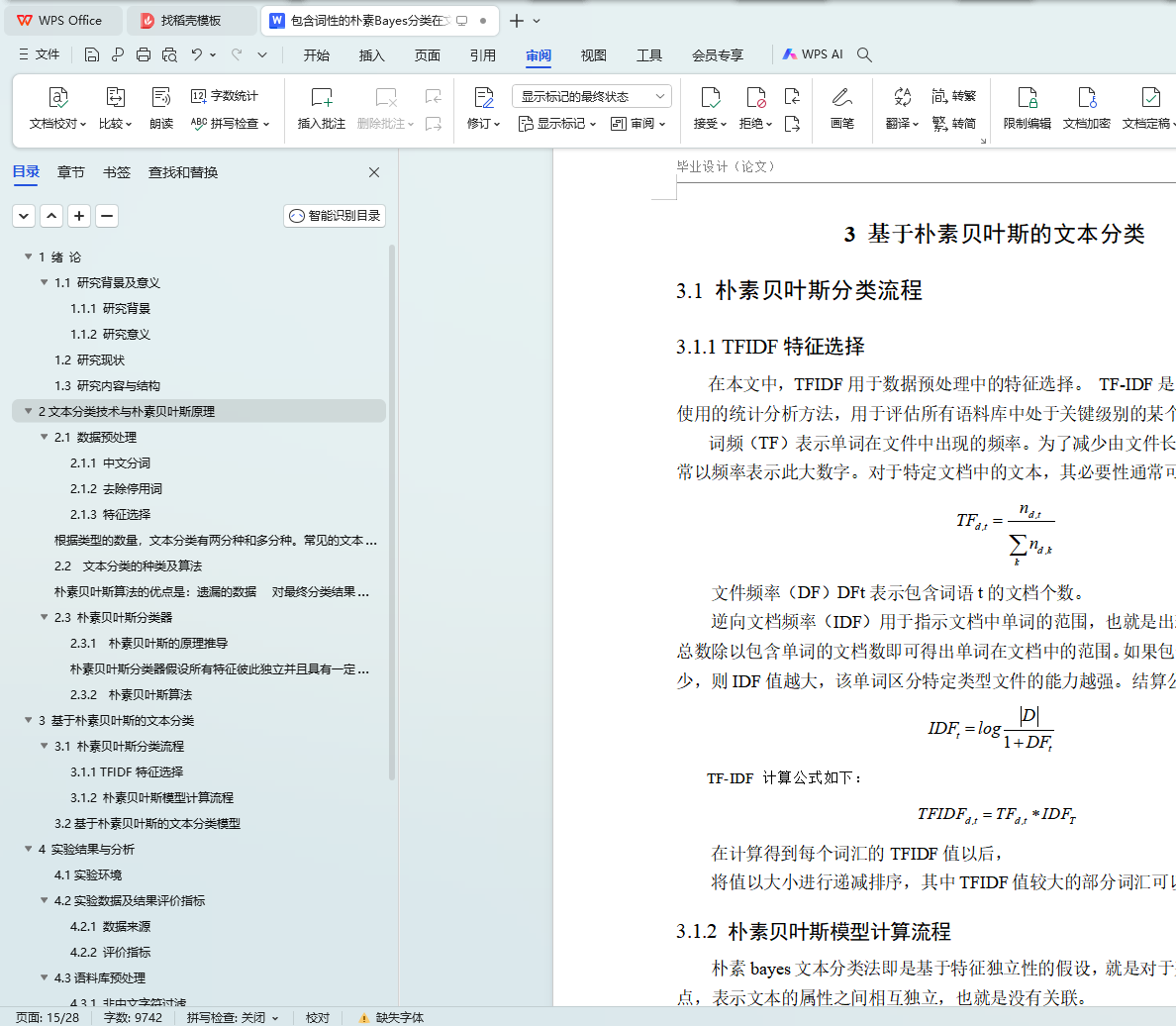
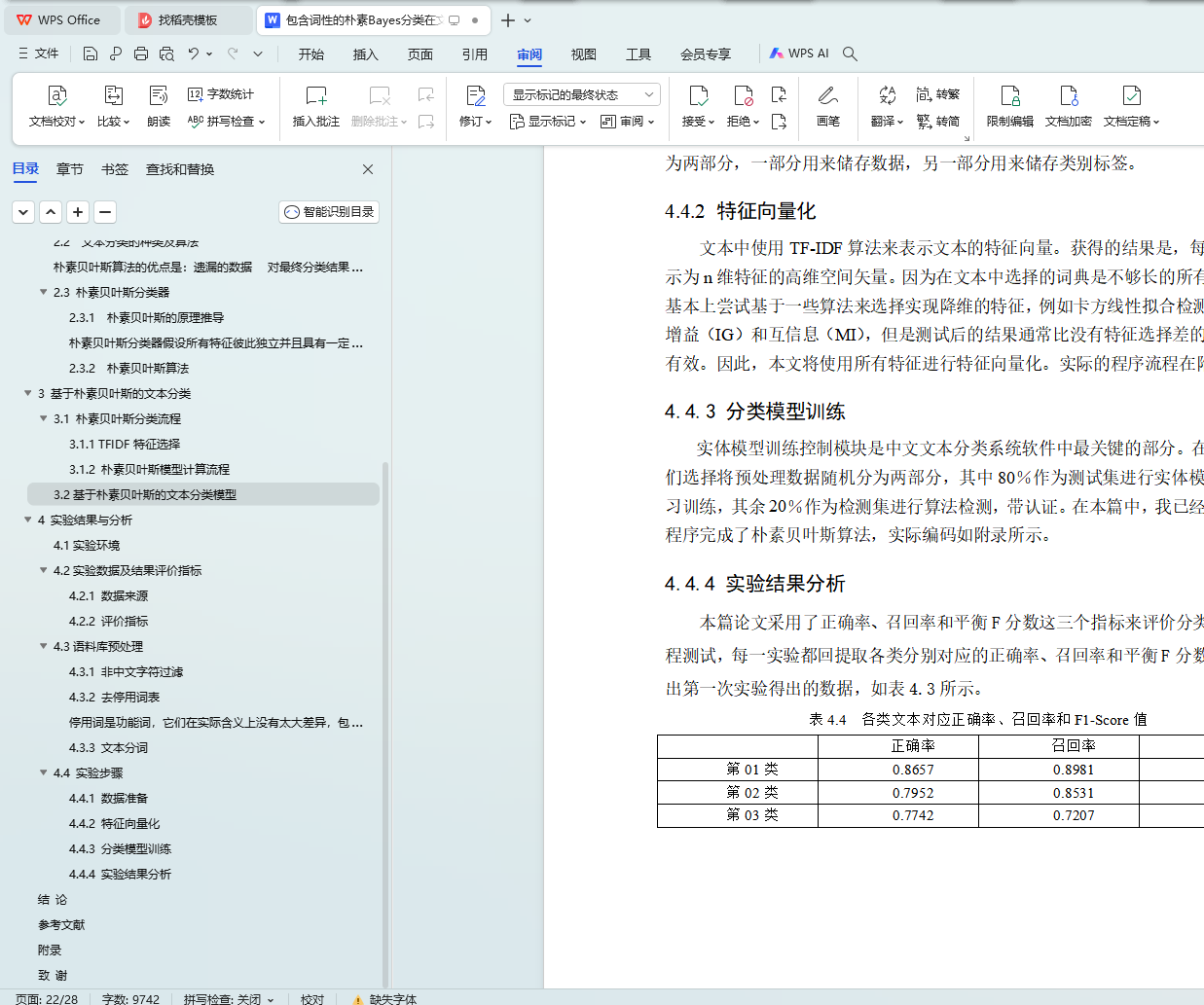
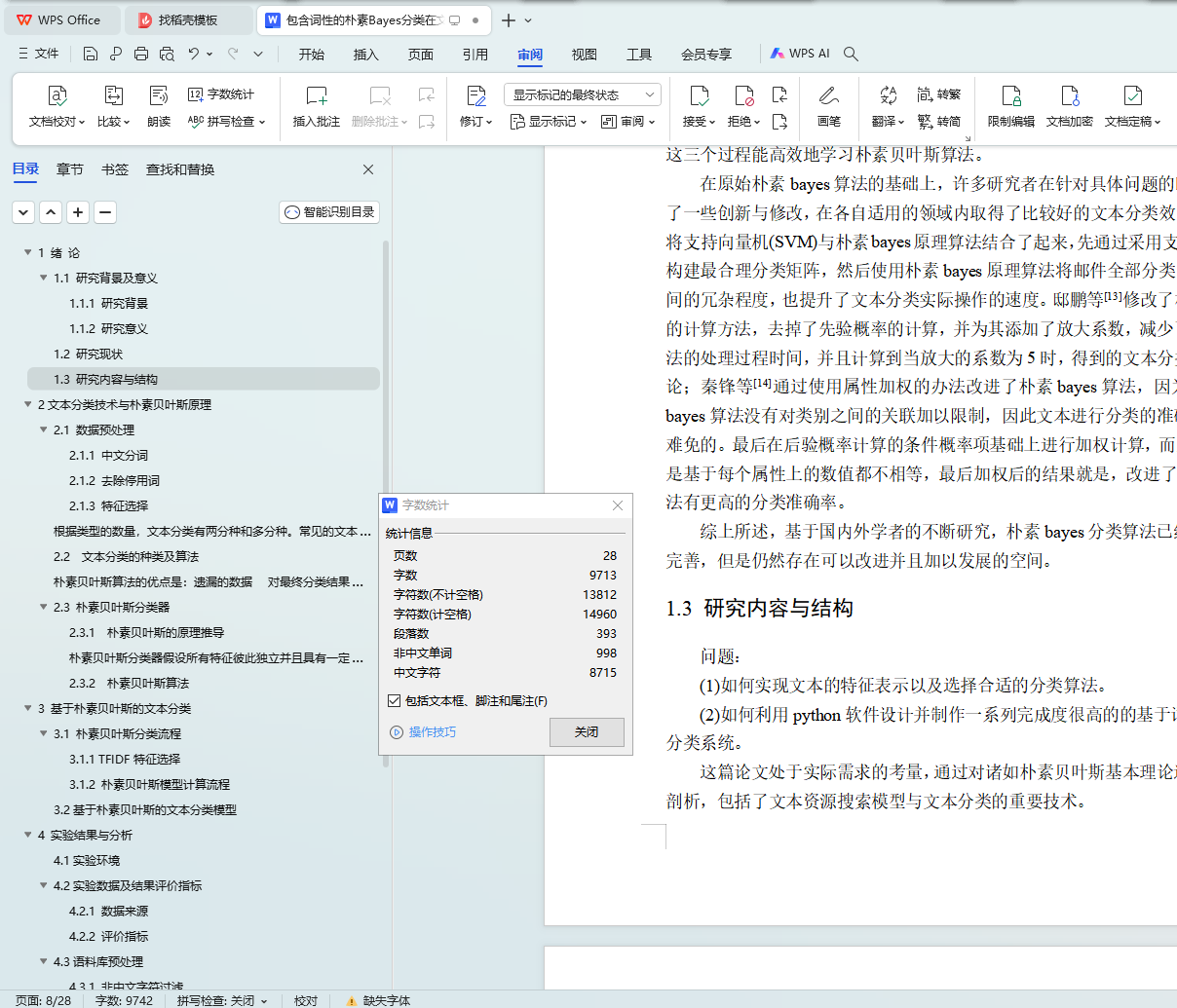
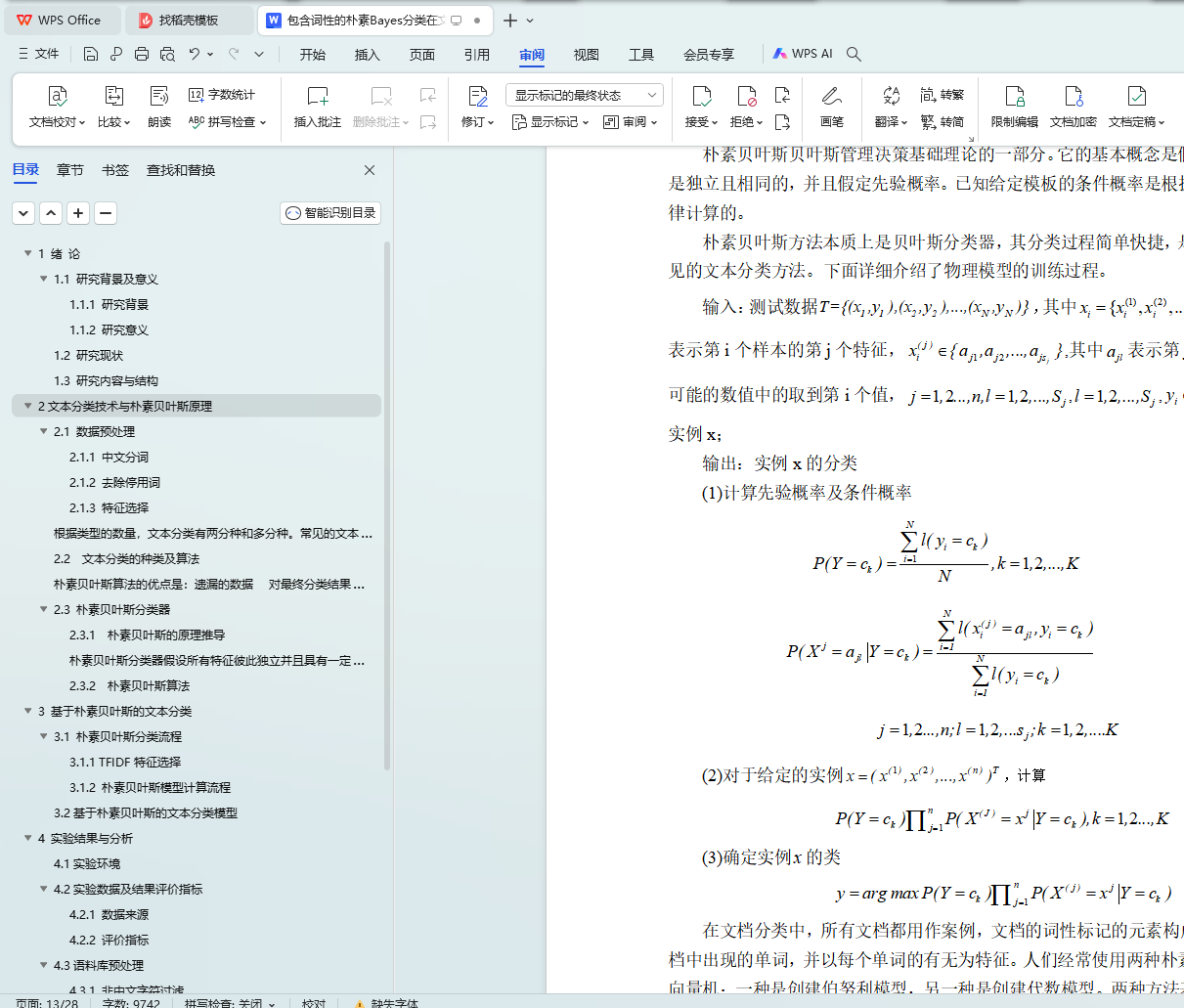
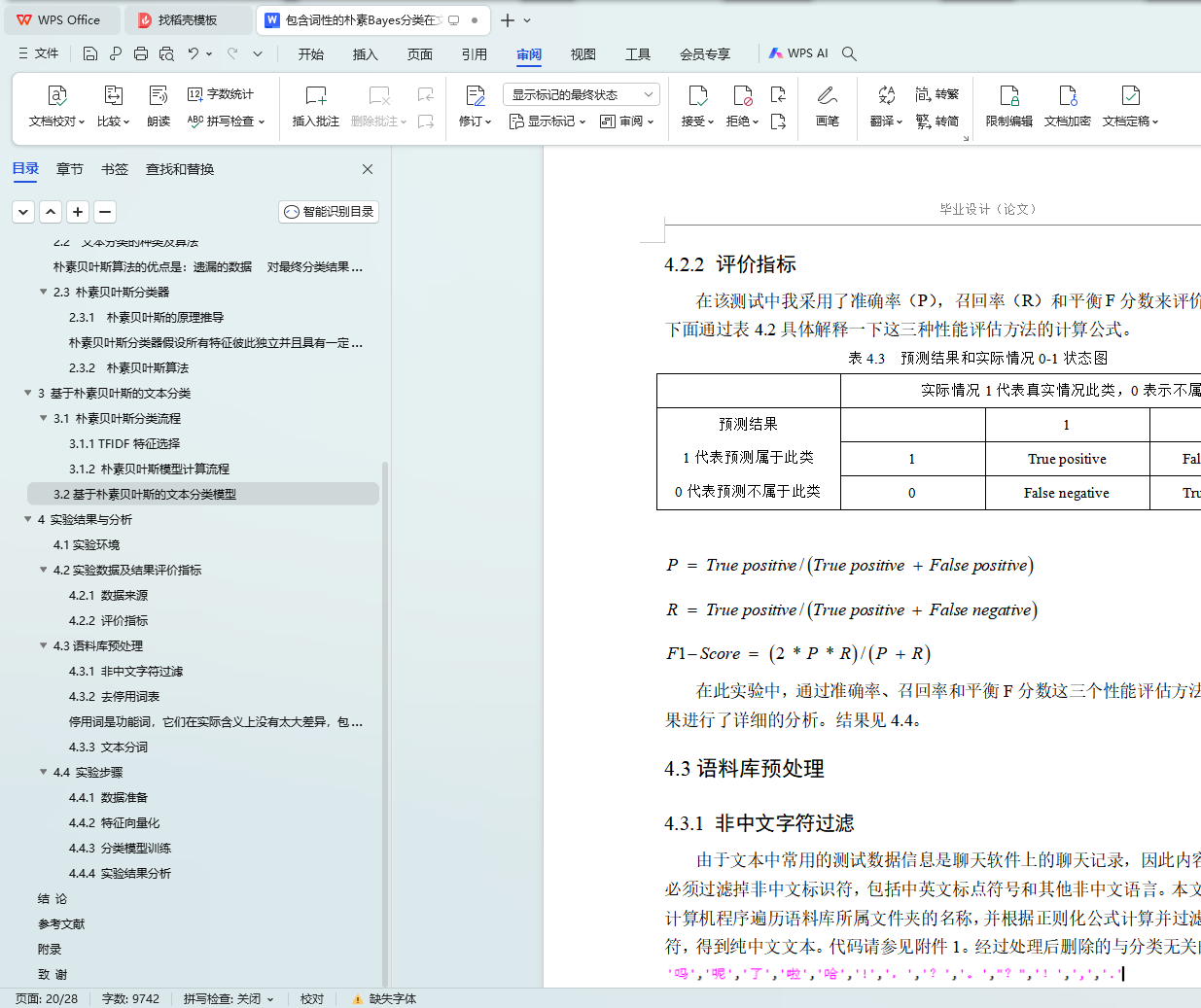
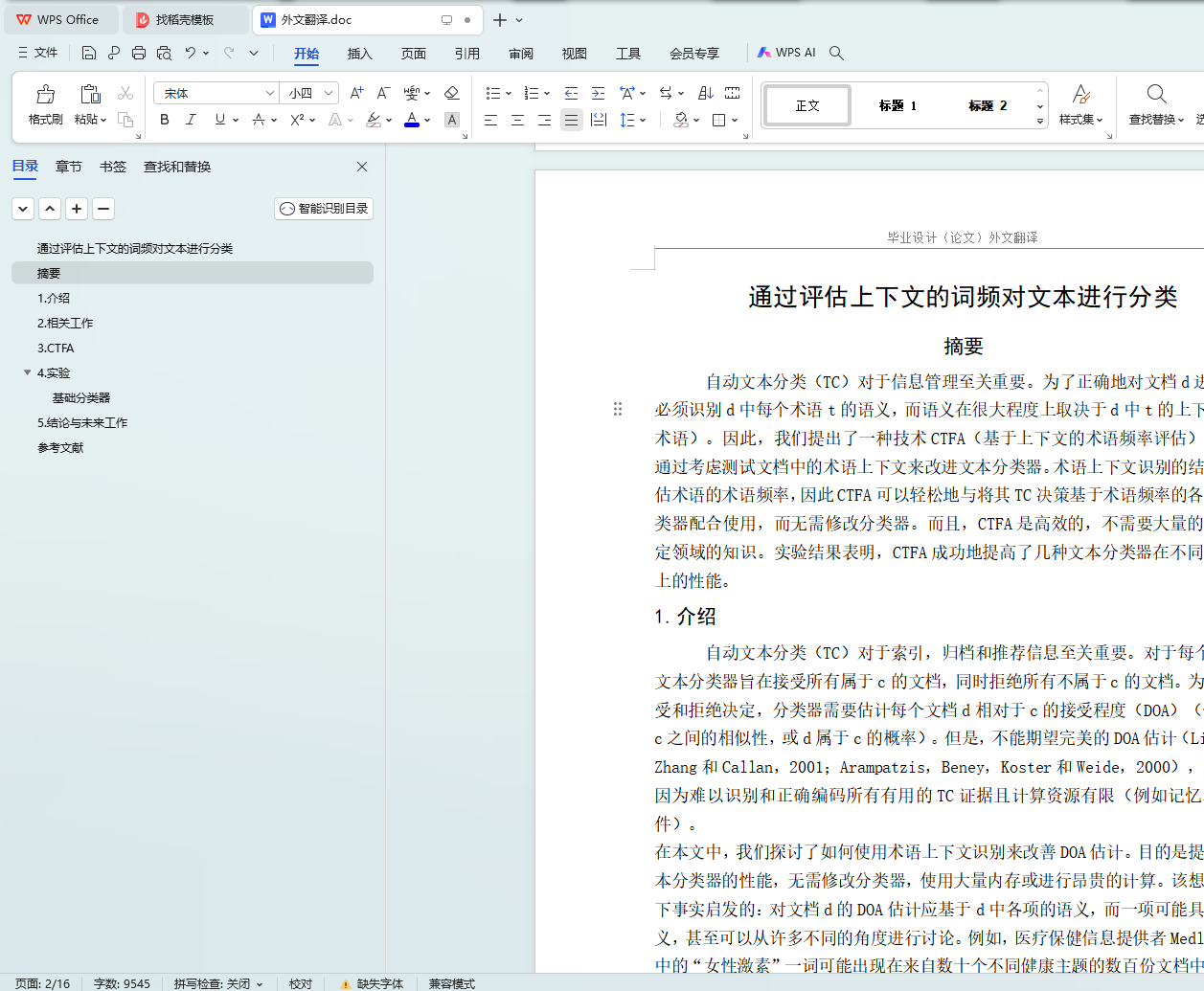
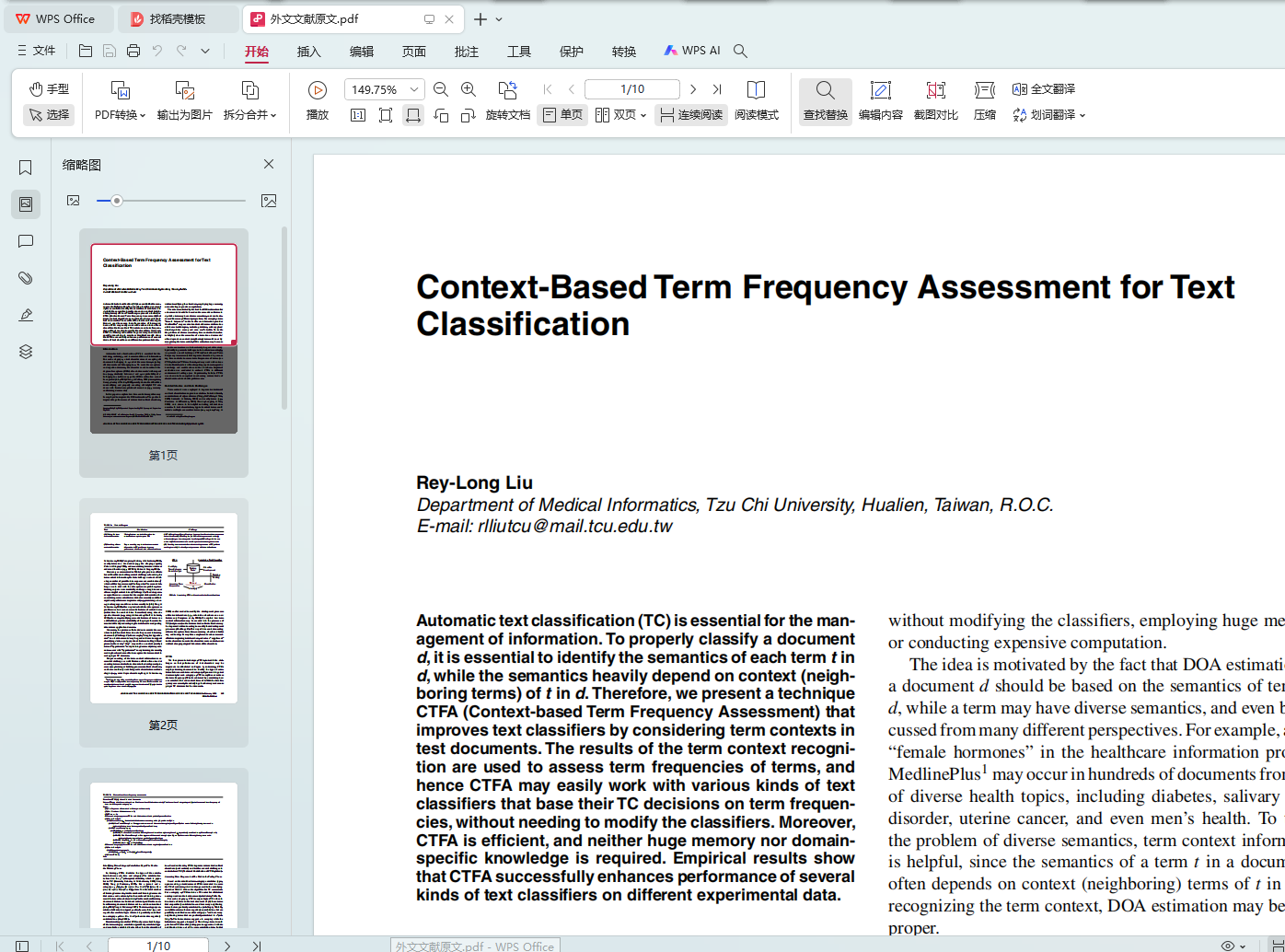
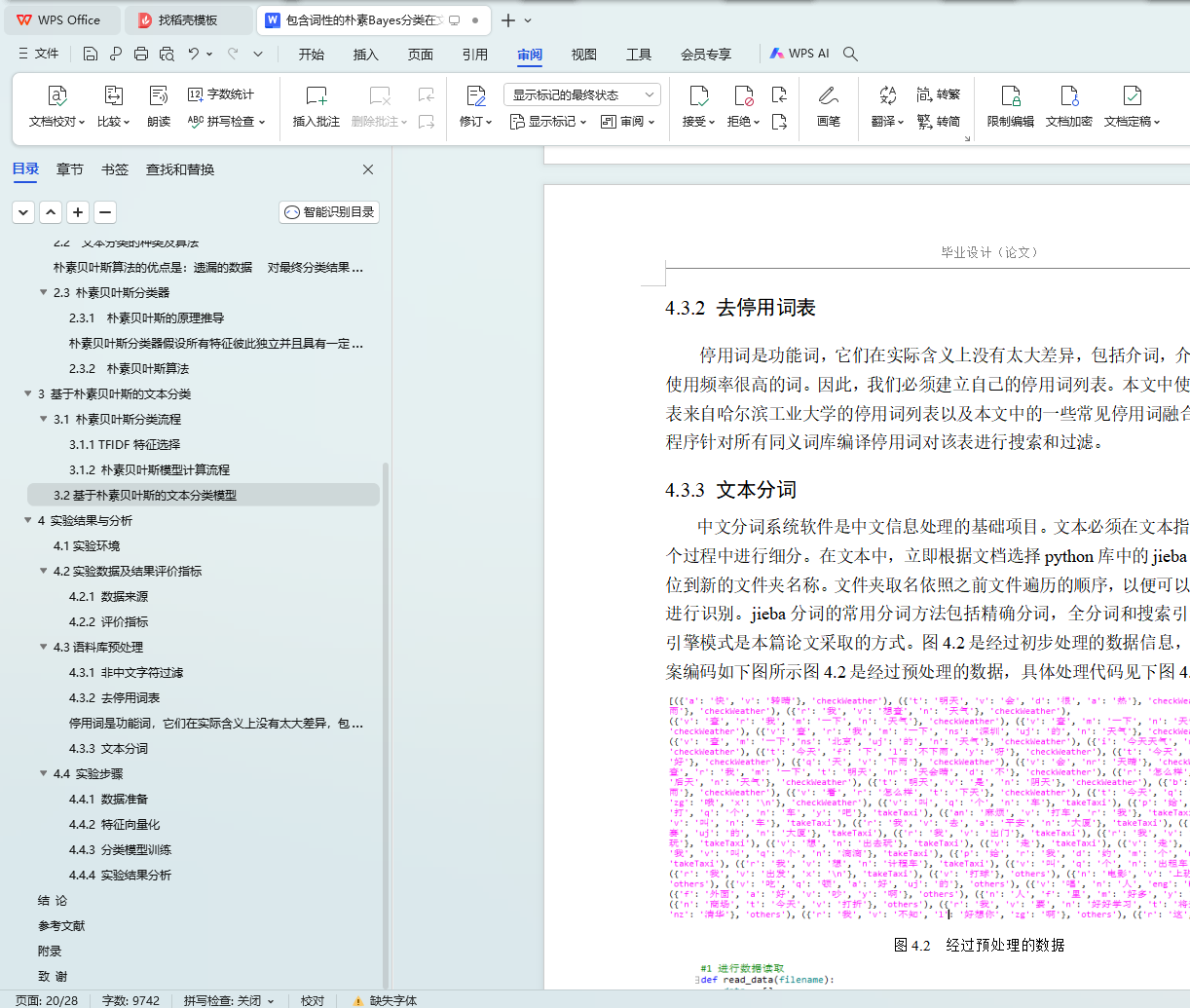