结合PCA与LDA算法的人脸识别研究
【摘要】 人脸识别技术的研究有很重要的现实意义。在一个国家的军事上,治安等领域都有应用,居民用户门锁、公共视频的监控、边检查证、汽车通行驾照等方面都有很广泛的实际用途。在民众生活和商业贸易领域,各种各样的银行卡和各类保险公司的人脸确认和辨别都有很大的实用意义。人脸的识别也作用在其他很多贴近生活的领域,如家庭娱乐,如主人的智能玩具,家庭机器人和实际的面部虚拟播放器。
通过对比单一的PCA方法进行特征提取后的人脸识别与结合PCA+LDA算法后的人脸识别率,达到了人脸识别算法的实验性验证和结果分析。用提取出特征脸的方法进行识别,这也是主成分分析法在实际应用中的一部分。该方法中一种比较通用且基础的就是K-L变换,经过多年来的验证改变换已经在模式识别领域内取得了较好的结果。在进行人脸识别之前将测试集里的图片在子空间上进行投影并得出一组系数,通过训练集与测试集系数的两相对比就能够得出那一张训练图像与测试图像更接近,从而得出识别的匹配结果。
【关键词】 主分量分析法,线性判别分析法,人脸识别,特征脸
Face Recognition Based on PCA and LDA Algorithms
【Abstract】 The research of face recognition technology has very important practical significance. In a country's military, public security and other fields have applications, residential user door locks, public video monitoring, border inspection and verification, automobile driving license and other aspects have a wide range of practical USES. In the field of people's life and commercial trade, the face recognition and discrimination of various bank CARDS and various insurance companies are of great practical significance. Face recognition is also used in many other areas close to life, such as home entertainment, such as owners' smart toys, home robots and real face virtual players.
The experimental verification and result analysis of the face recognition algorithm were achieved by comparing the face recognition after feature extraction with the face recognition rate after combining PCA+LDA algorithm with a single PCA method. It is also a part of the practical application of principal component analysis (PCA) to extract the characteristic face for recognition. One of the general and basic methods is k-l transformation, which has achieved good results in the field of pattern recognition after years of verification. Before face recognition, the images in the test set are projected on the subspace and a set of coefficients are obtained. By comparing the coefficients of the training set and the test set, the training image is more similar to the test image, and the matching result of recognition can be obtained.
【Key Words】 PCA,LDA,Face recognition,Eigenface
目 录
1 绪 论
1.1 研究背景与意义
1.2 国内外研究现状以及存在的问题
1.2.1 国内外研究现状
1.2.2 可以提取的人脸图像特征
1.2.3 特征提取的研究方法
1.2.4 特征提取存在的问题
2 人脸识别技术概括
2.1 人脸识别技术的基本框架
2.2 研究的重难点
2.3 本文方法概述
3 人脸特征提取方法
3.1 基于PCA算法的特征提取
3.1.1 PCA算法的基本原理
3.1.2 PCA算法进行人脸识别的过程概括
3.1.3 人脸空间的建立
3.1.4 生成特征脸
3.1.5 人脸图像重建
3.1.6 PCA的特点以及存在的问题
3.2 基于LDA算法的线性判别分类
3.2.1 Fisher判别准则
3.2.2 LDA线性判别分类方法
3.2.3 LDA算法的缺点
3.3 基于PCA+LDA算法的特征提取步骤
4 本文方法的实现以及结果
4.1 人脸库的选取
4.2 算法的有效性分析
4.3 影响识别率的因素分析
4.3.1 PCA特征子空间维数Z与识别率的关系
4.3.2 LDA投影矩阵系数K与识别率的关系
4.3.3 类别的增加对识别率的影响
4.4 实验结果总结
结 论
参考文献
附 录
致 谢
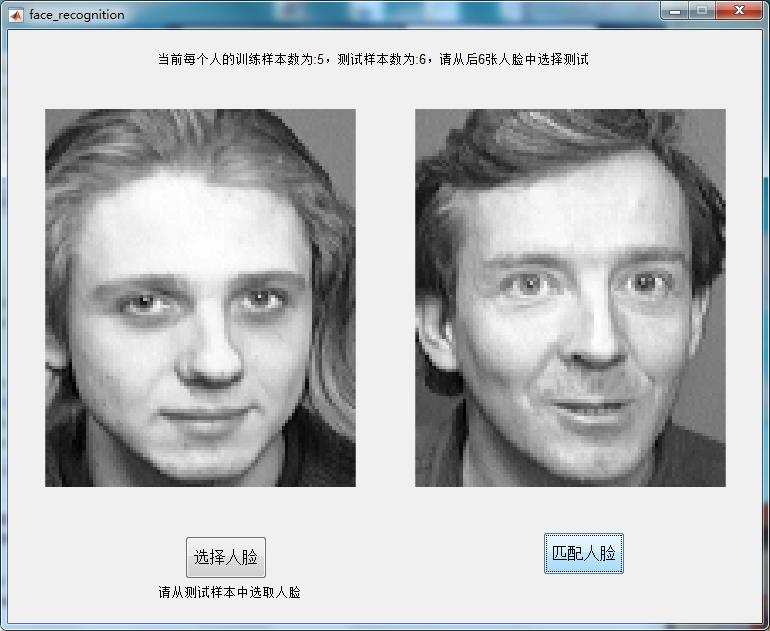
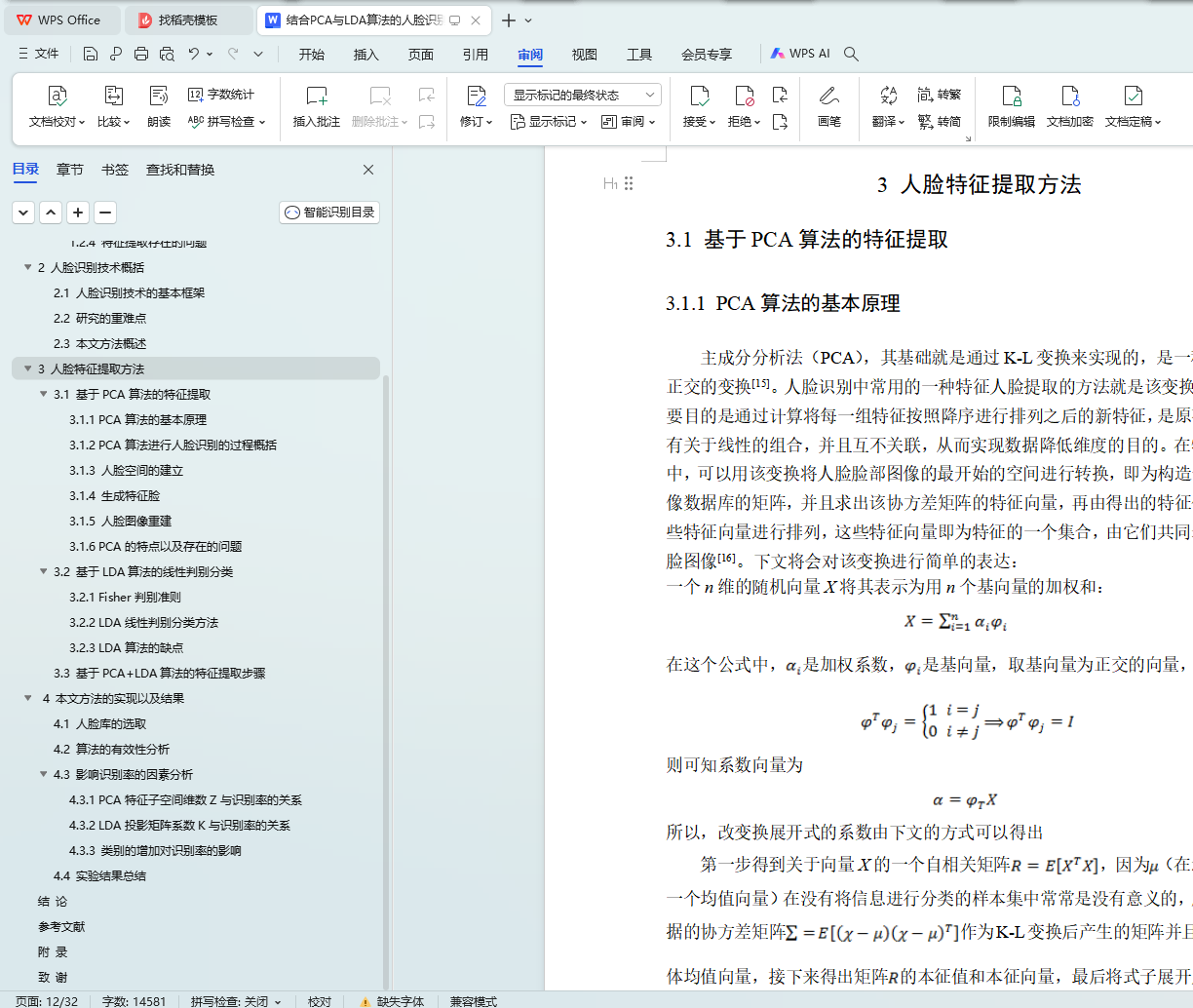
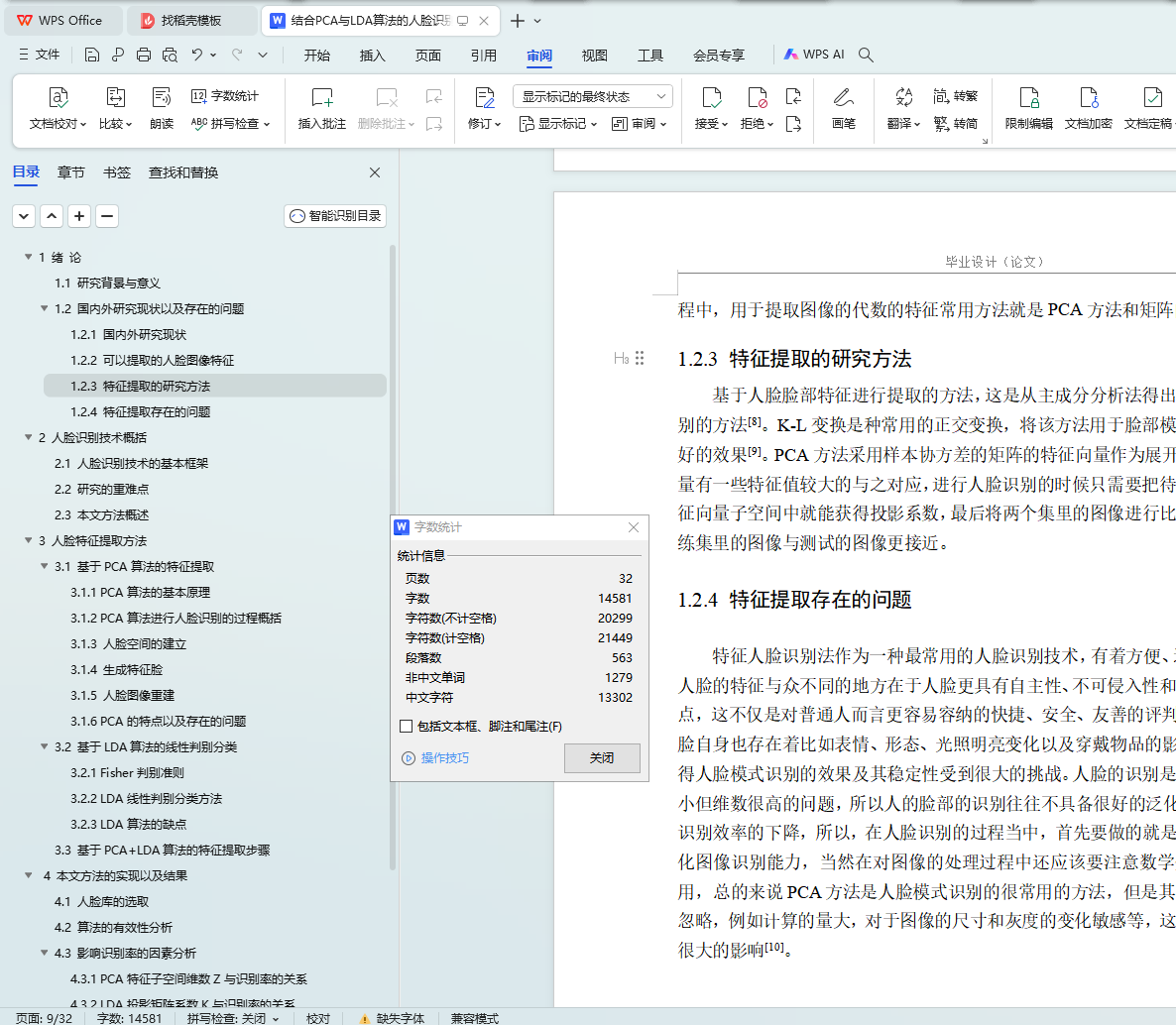
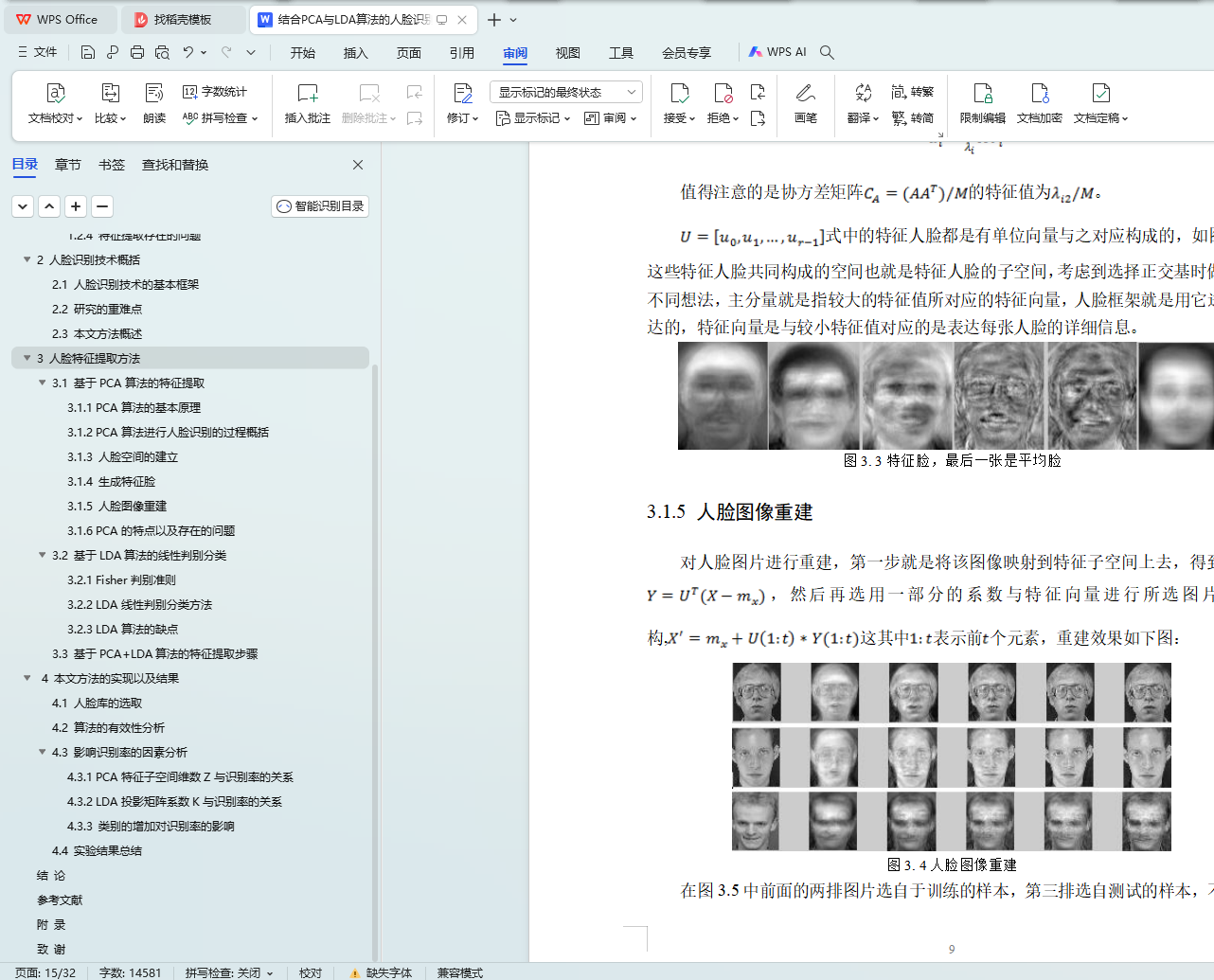
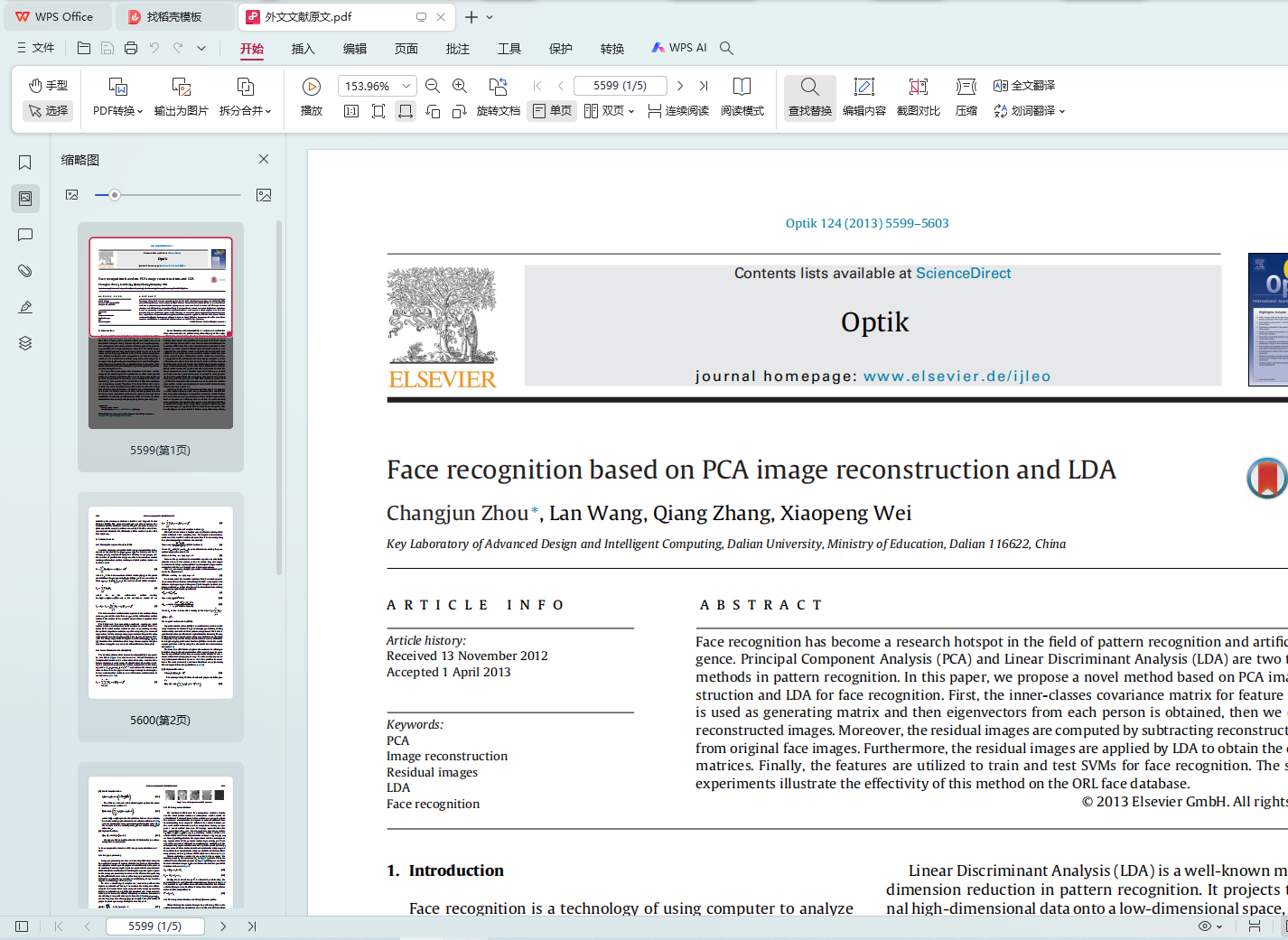
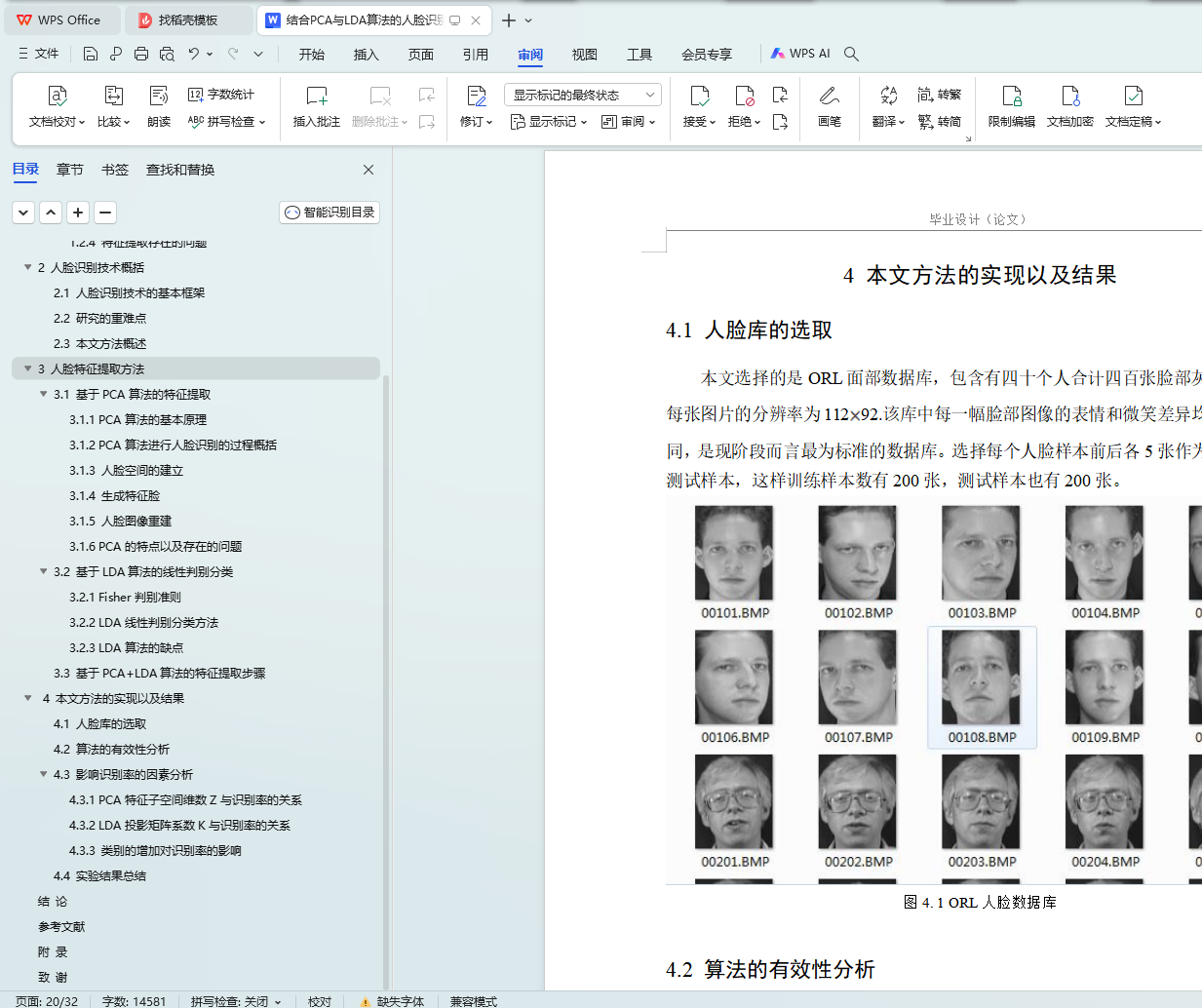
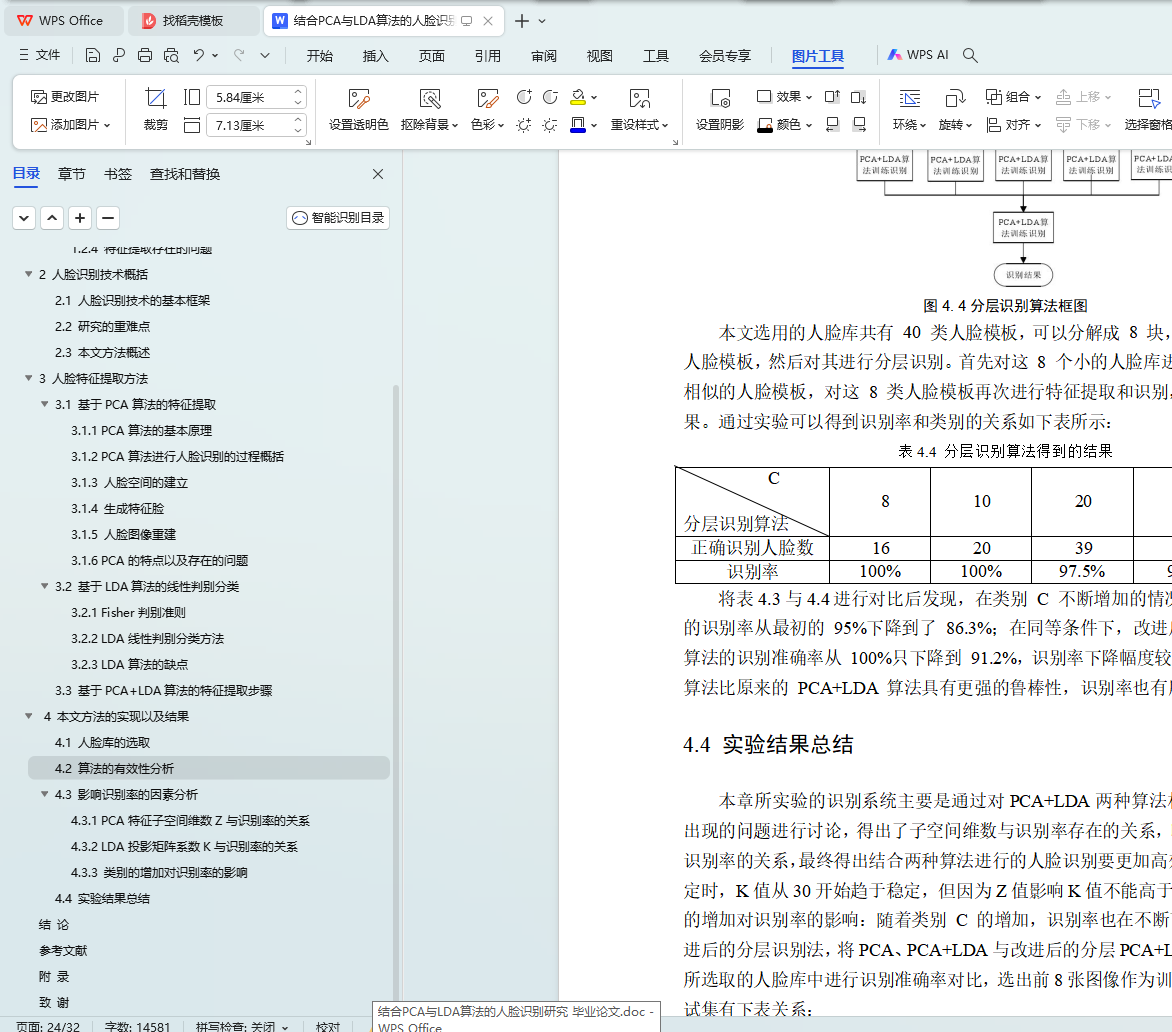
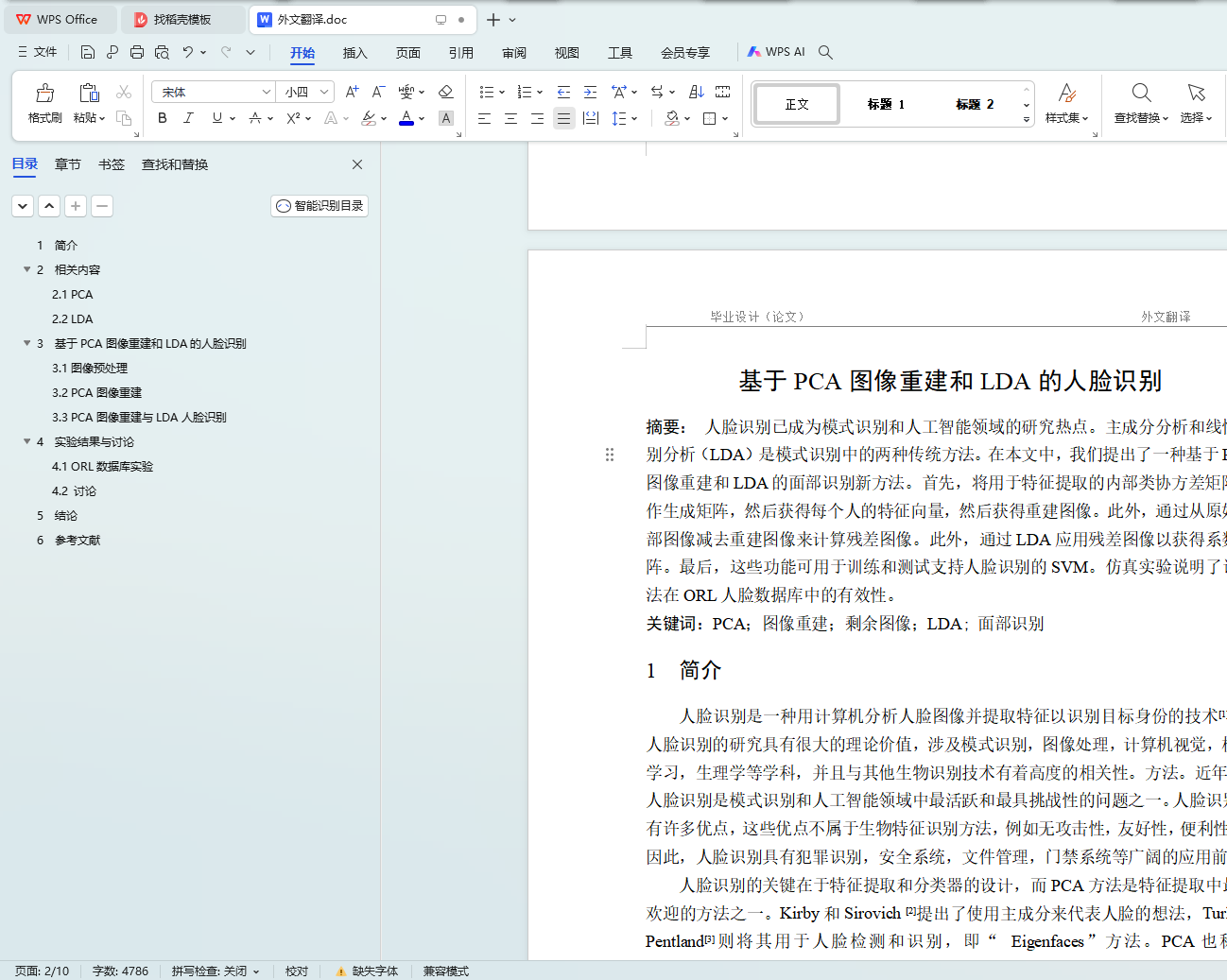
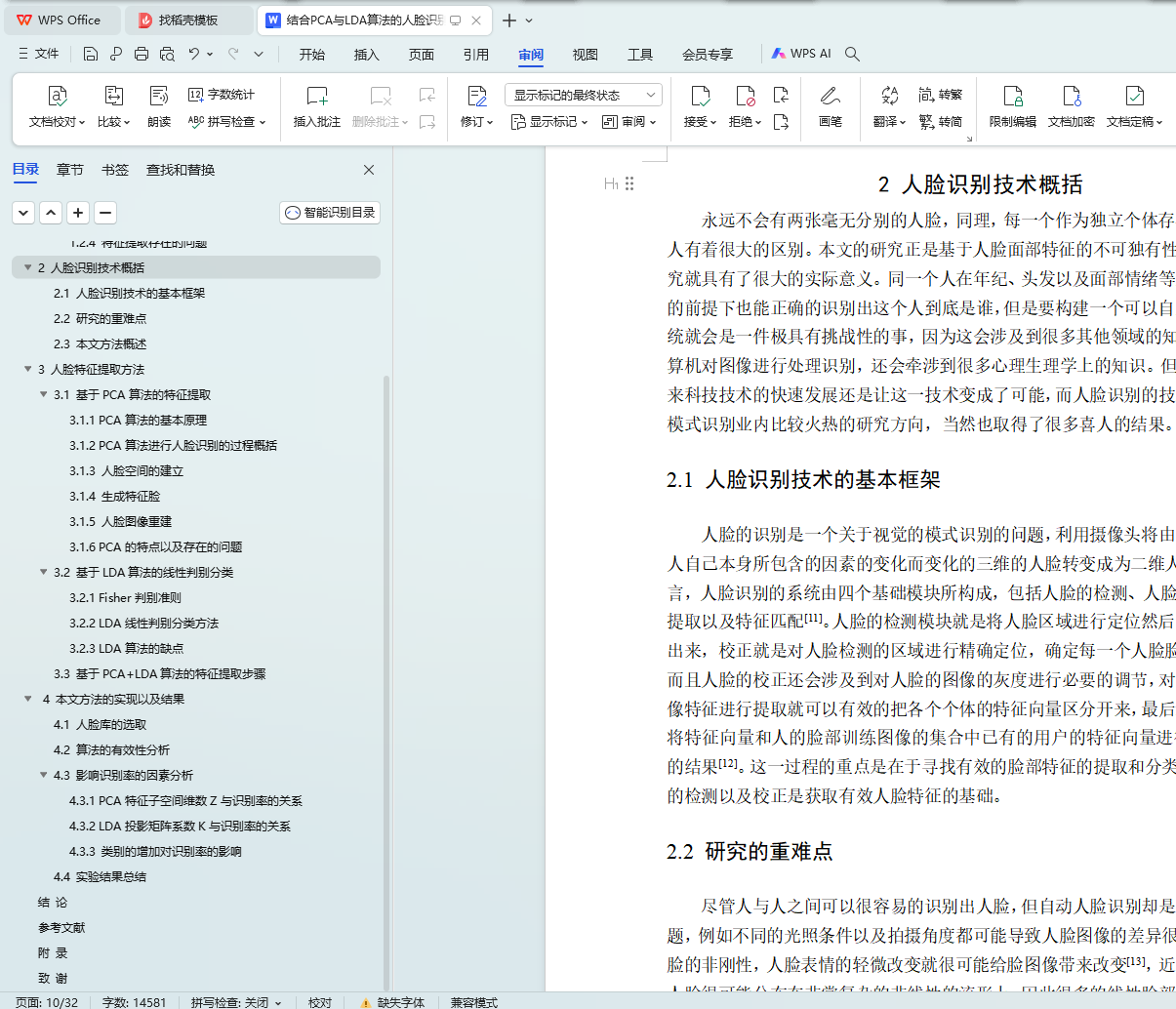
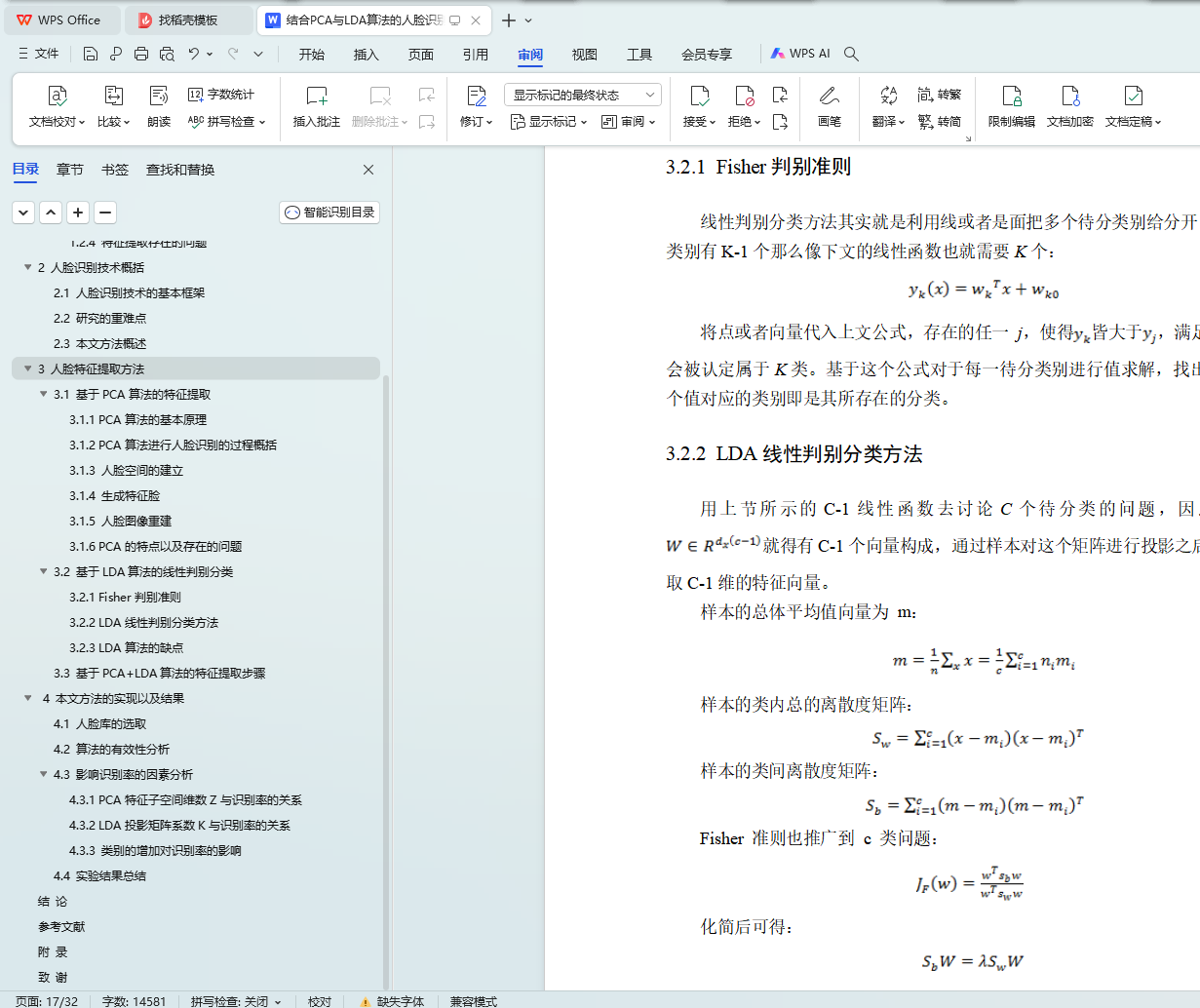
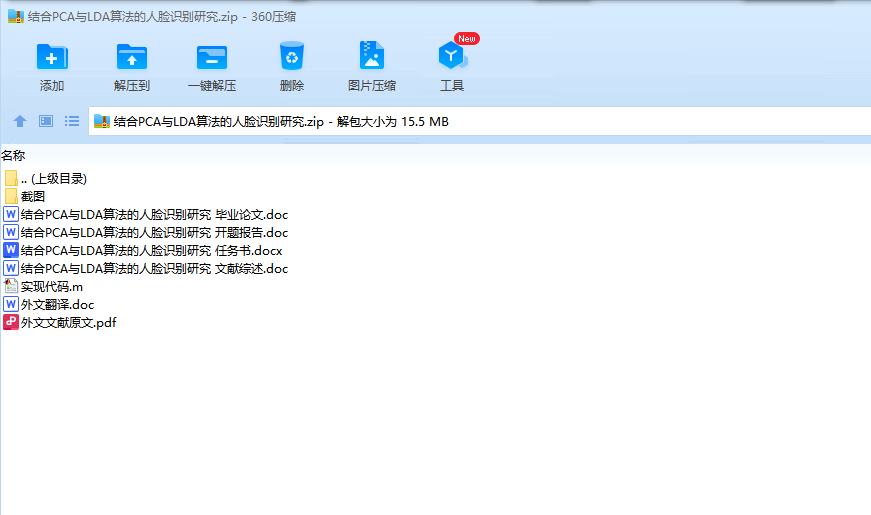
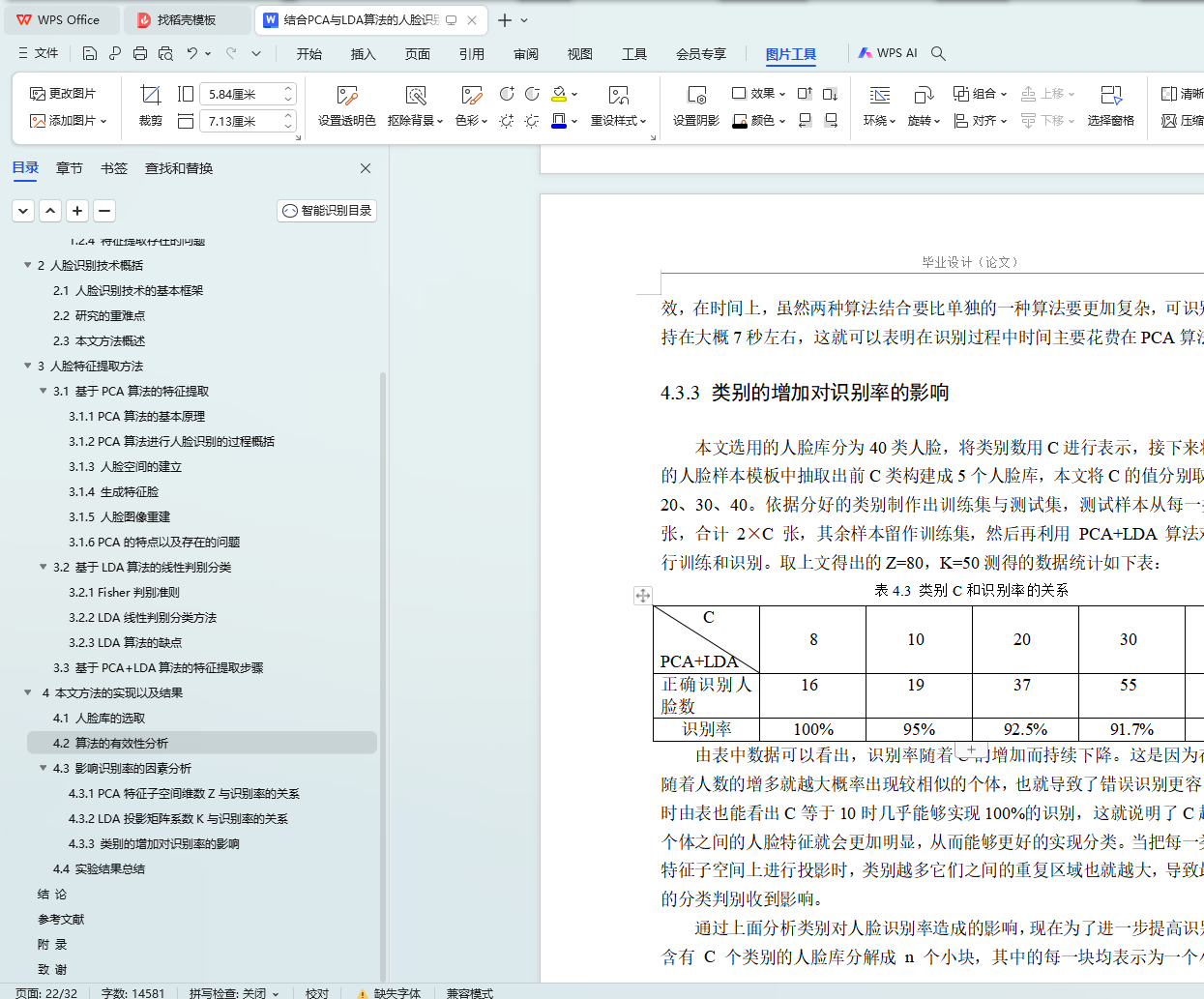
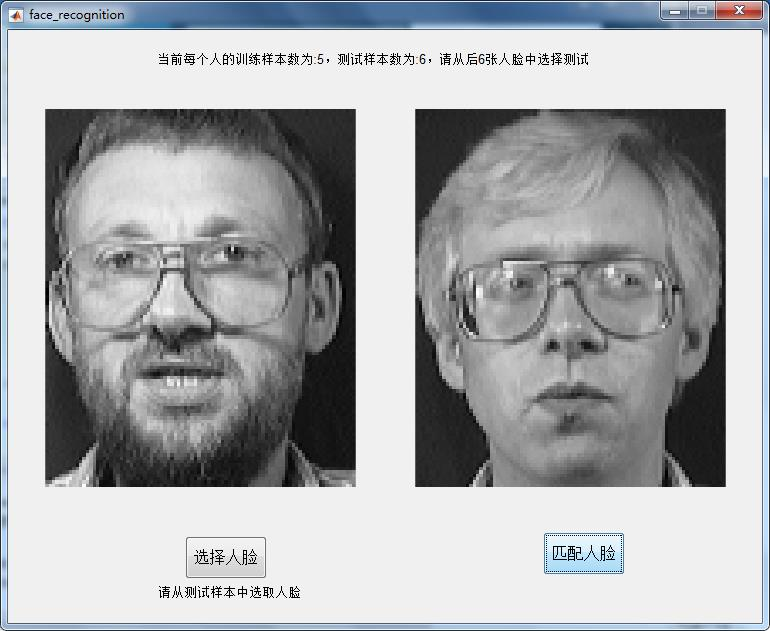
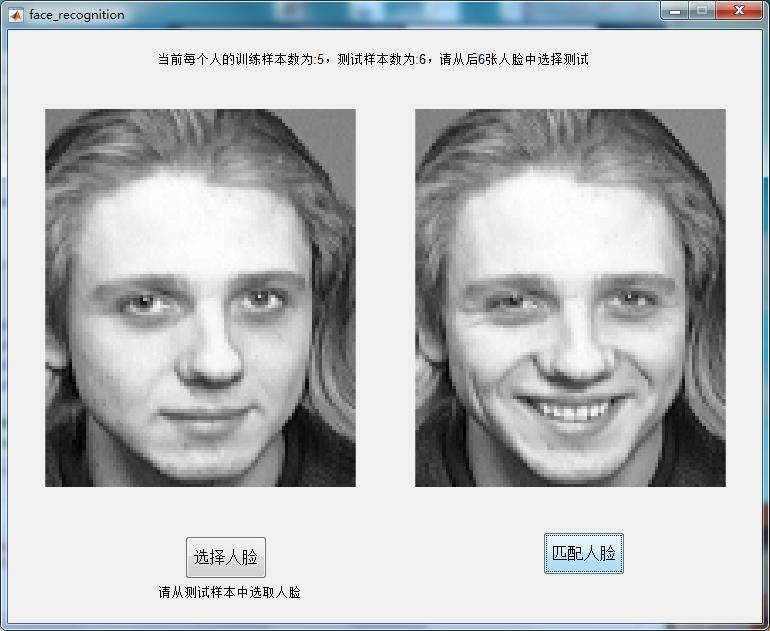
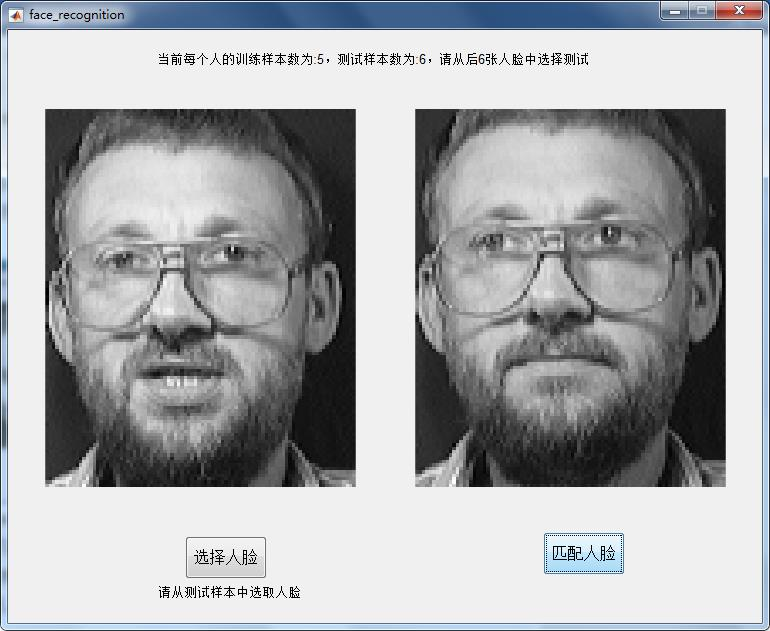