基于改进激活函数的单层神经网络
【摘要】 作为20世纪80年代以后人工智能领域研究的热点,人工神经网络的研究已经取得了很大的进展,相关的学习理论和方法已经发展成为一门界于物理学、数学、计算机科学、和神经生物学之间的交叉学科。它在模式识别,图像处理,智能控制,组合优化,金融预测与管理,通信,机器人以及专家系统领域得到广泛的应用,解决了许多现代计算机至今完全无法解决的实际问题,在许多领域中成为了一个不可或缺的部分。但是在上述这些应用中也会发现人工神经网络的一些不足,比如学习速度慢、泛化能力弱、稳定性差的等不足。
本文提出了一个新的激活函数bump形函数,改进的激活函数的支撑集在[a,b]上,通过调节a,b两个参数。因此神经网络不需要阈值,而且引入一个参数,使得网络具有更好的适应性,新的激活函数就是建立在这种bump形函数上。然后改进激活函数的BP算法,绘制出迭代次数—误差曲线来比较配备新的激活函数的神经网络和经典神经网络的收敛性。通过比较分析,实验证明构造的新的激活函数,改进的激活函数的单层神经网络比一般的单层神经网络具有更高的学习效率。
【关键词】 神经网络,激活函数,bump形函数,BP算法
Single layer neural network based on improved activation function
【Abstract】 As a hot spot in the field of artificial intelligence after 1980s, the research of artificial neural network has made a great progress, Relevant learning theories and methods have been developed as a cross discipline in physics, mathematics, computer science, and neuroscience. It is widely applied in the field of pattern recognition, image processing, intelligent control, combinatorial optimization, financial prediction and management, communication, robotics and expert system, neural network has solved many practical problems which the modern computer is still can not solve now, neural network has become an indispensable part in many domains. But in these applications we also find some deficiencies in the artificial neural network, such as slow learning speed, weak generalization ability, and poor stability.
This paper constructs a new activation function of bump shape function, improve the activation function of the support set in [a, b], by controlling a, b of the two parameters. Therefore the neural network no need threshold, and introduce a parameter, the network has better adaptability and the new activation function is built on the bump shape function. Then we improve the activation function of BP algorithm, and draw the iterations-error curves to compare the convergence of neural network owning new activation function with classical neural network. Through the comparative analysis, the experimental results show that the new activation function of the structure, the improved activation function of the single layer neural network has a higher learning efficiency than the ordinary one.
【Key Words】 neural network,activation function,bump shape function,BP algorithm
目 录
1 绪 论
1.1 研究背景及研究意义
1.2国内外研究综述
1.2.1国外研究综述
1.2.2 国内研究综述
1.3研究思路及方法
1.4论文的组织结构
2 激活函数
2.1 人工神经网络概述
2.1.1 人工神经网络的基本特征
2.1.2 人工神经网络模型
2.2 常见激活函数及其作用
2.3 新激活函数
3 改进激活函数的算法
3.1 误差函数
3.2 BP算法
4 验证结果
4.1 实验对象
4.2 实验结果
结 论
参考文献
附 录
致 谢
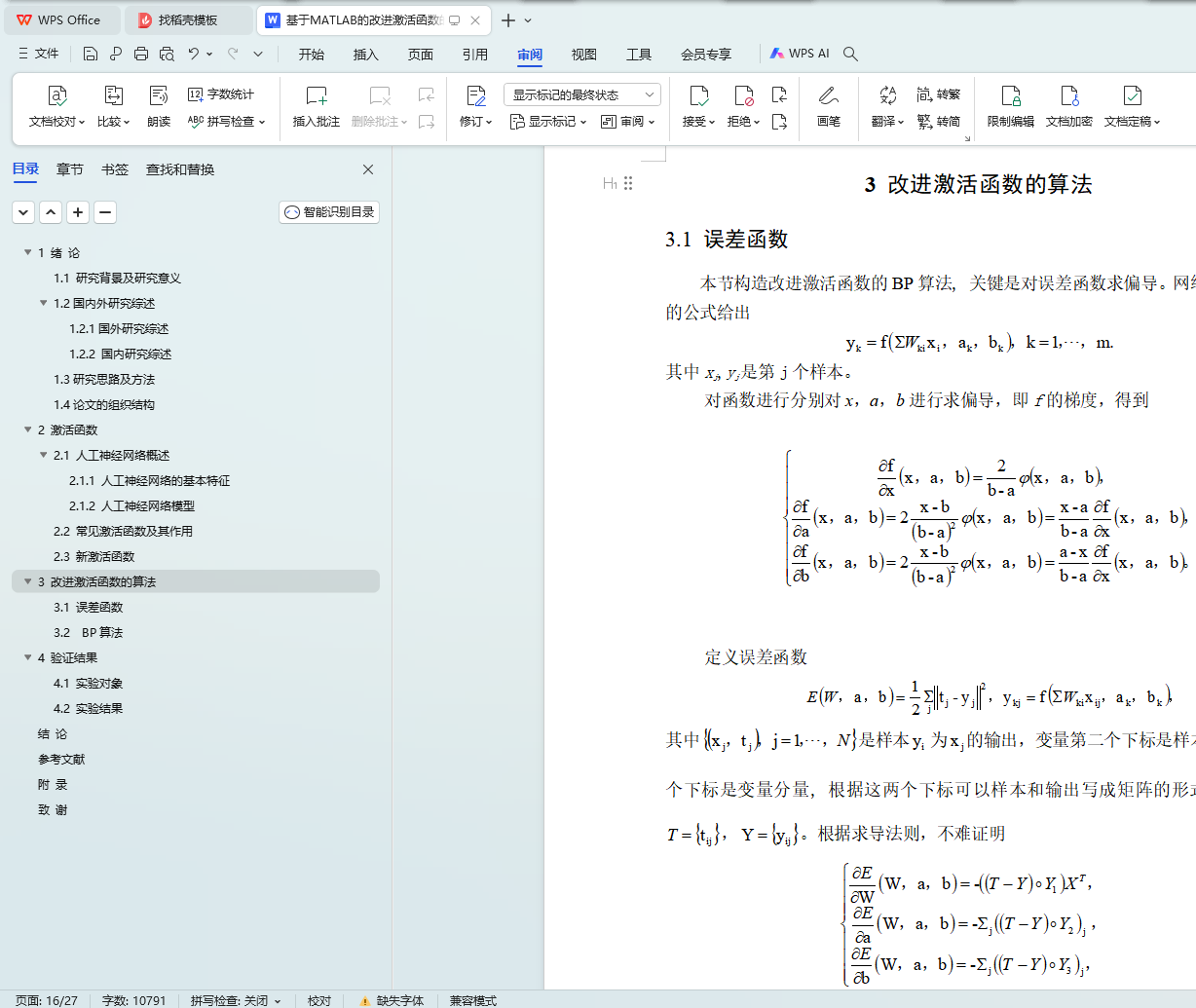
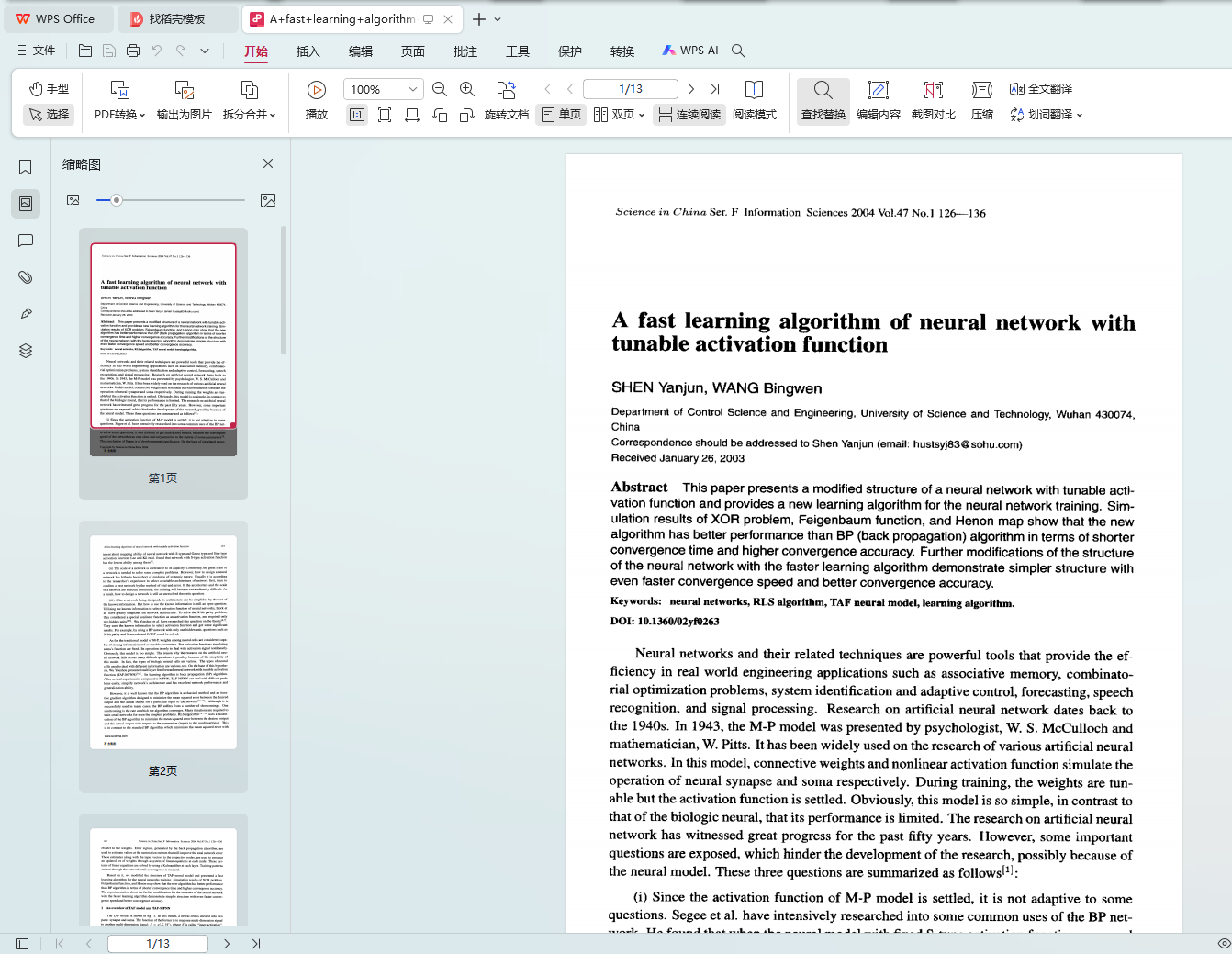
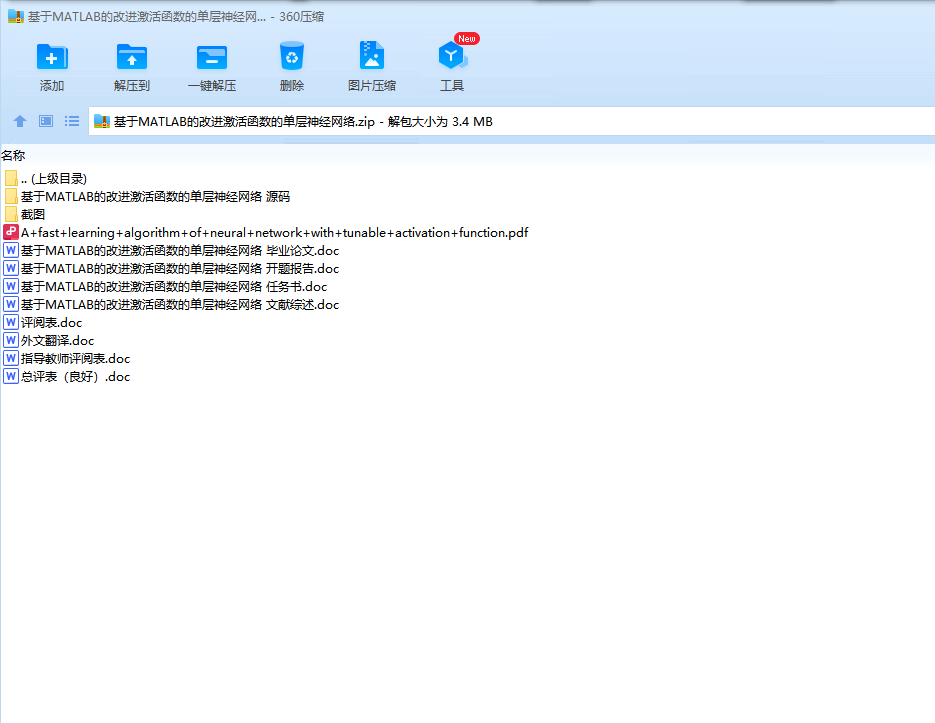
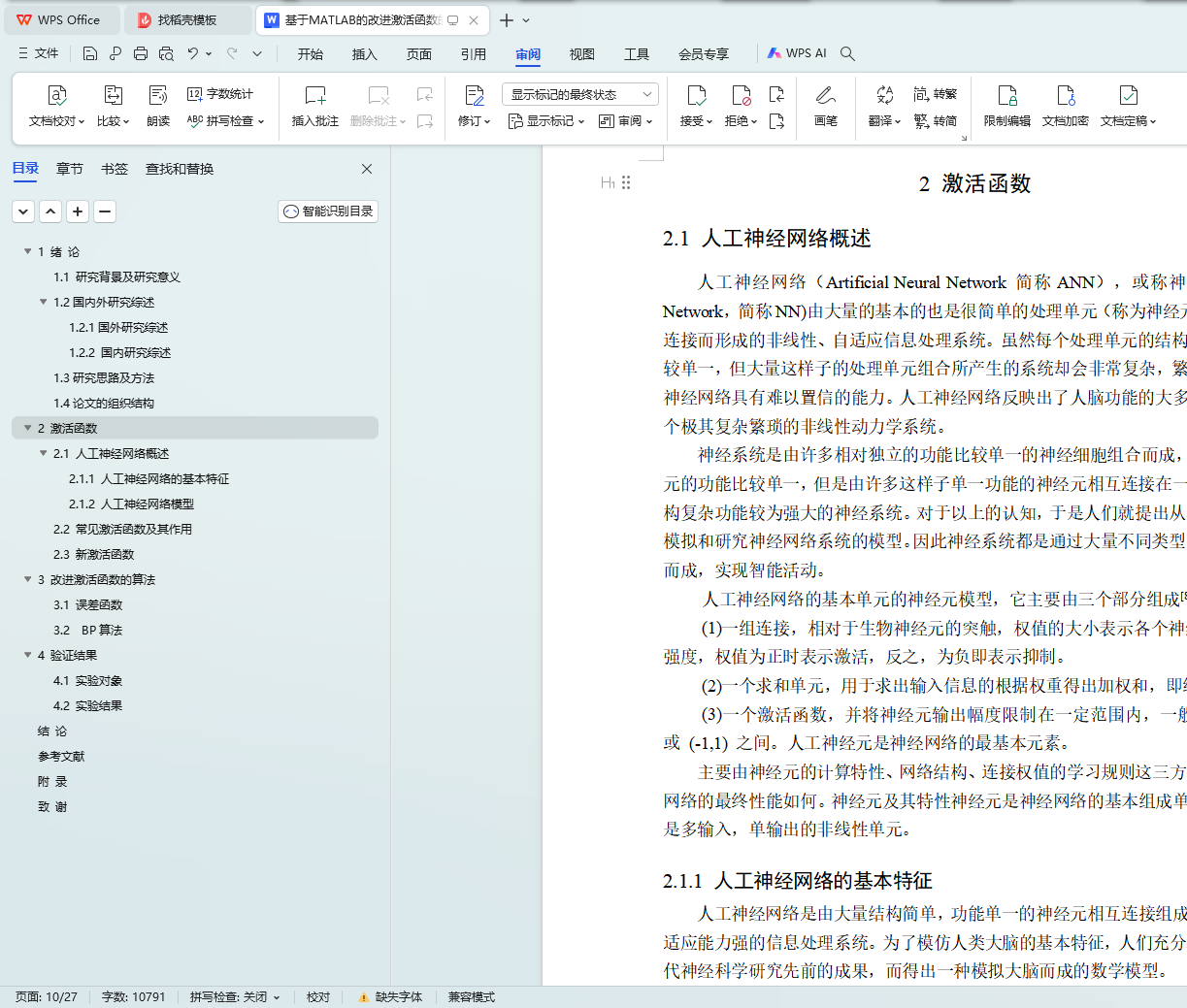
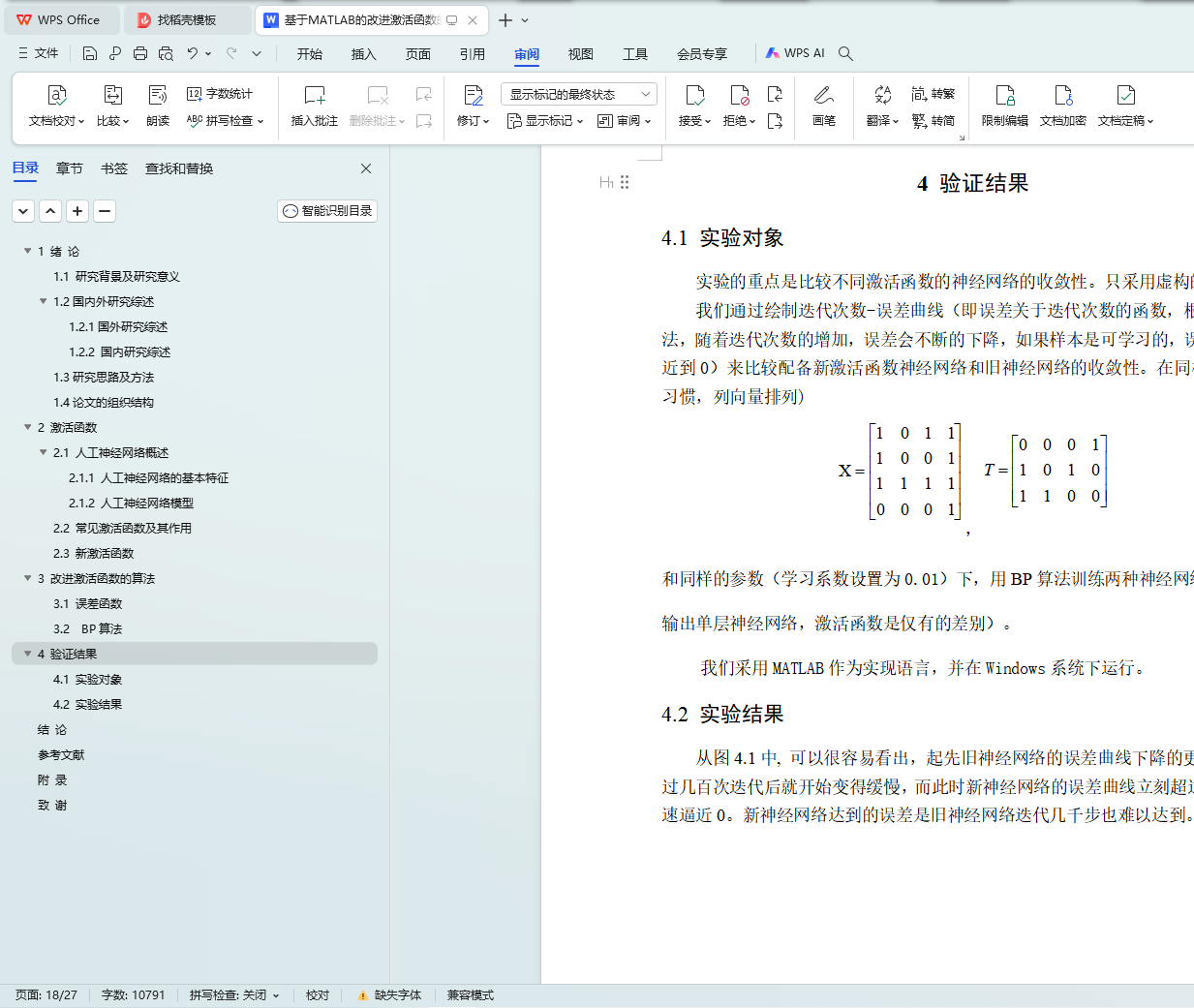
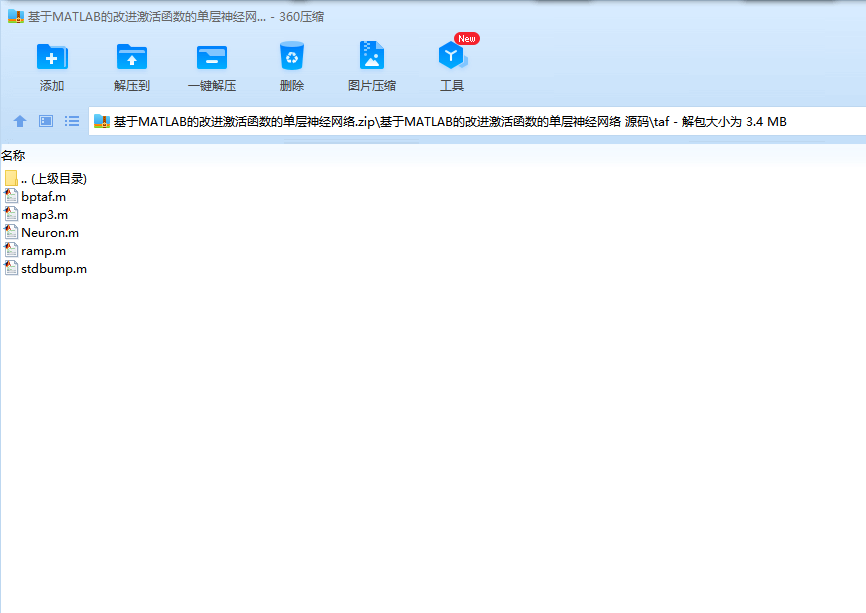
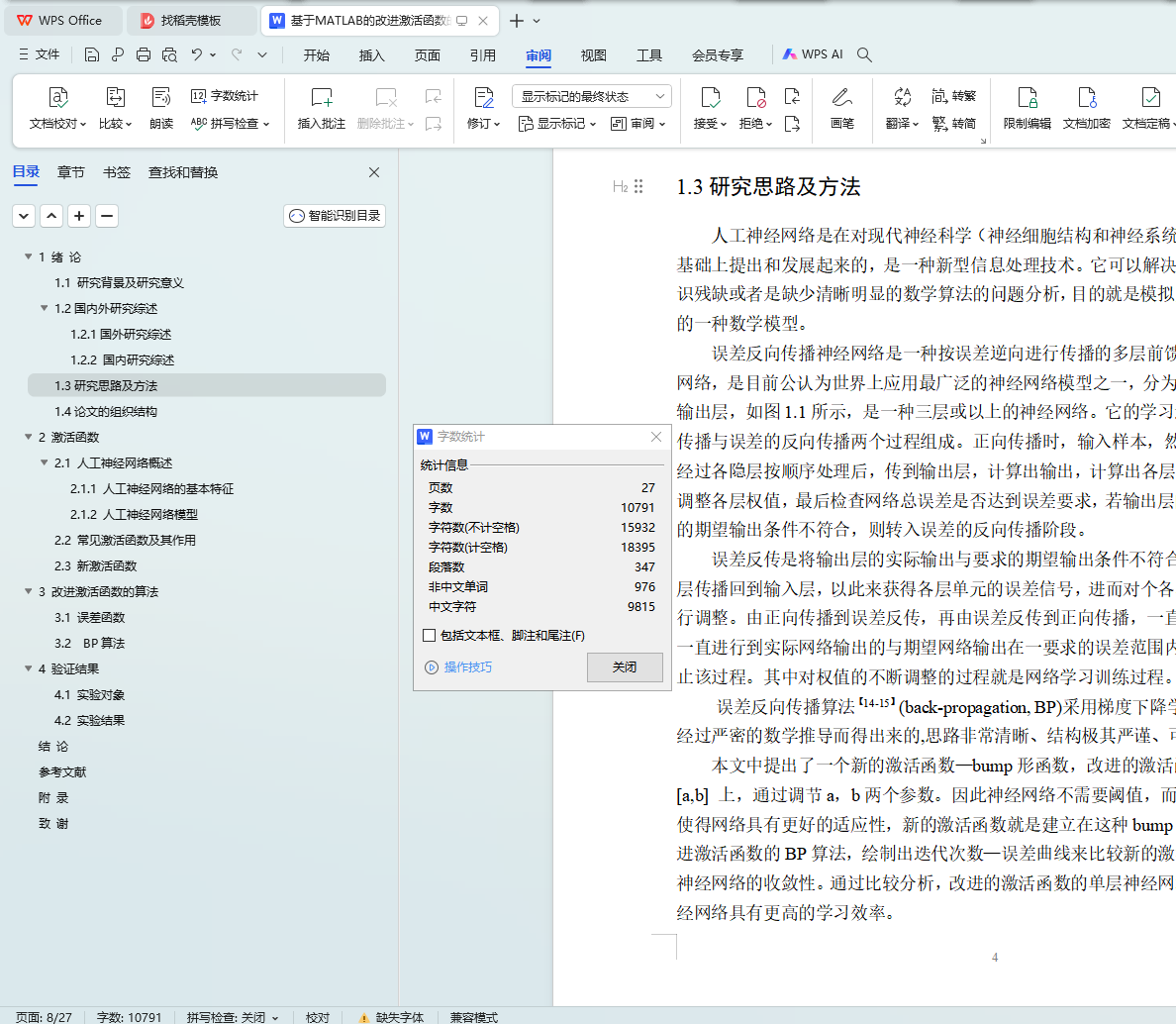
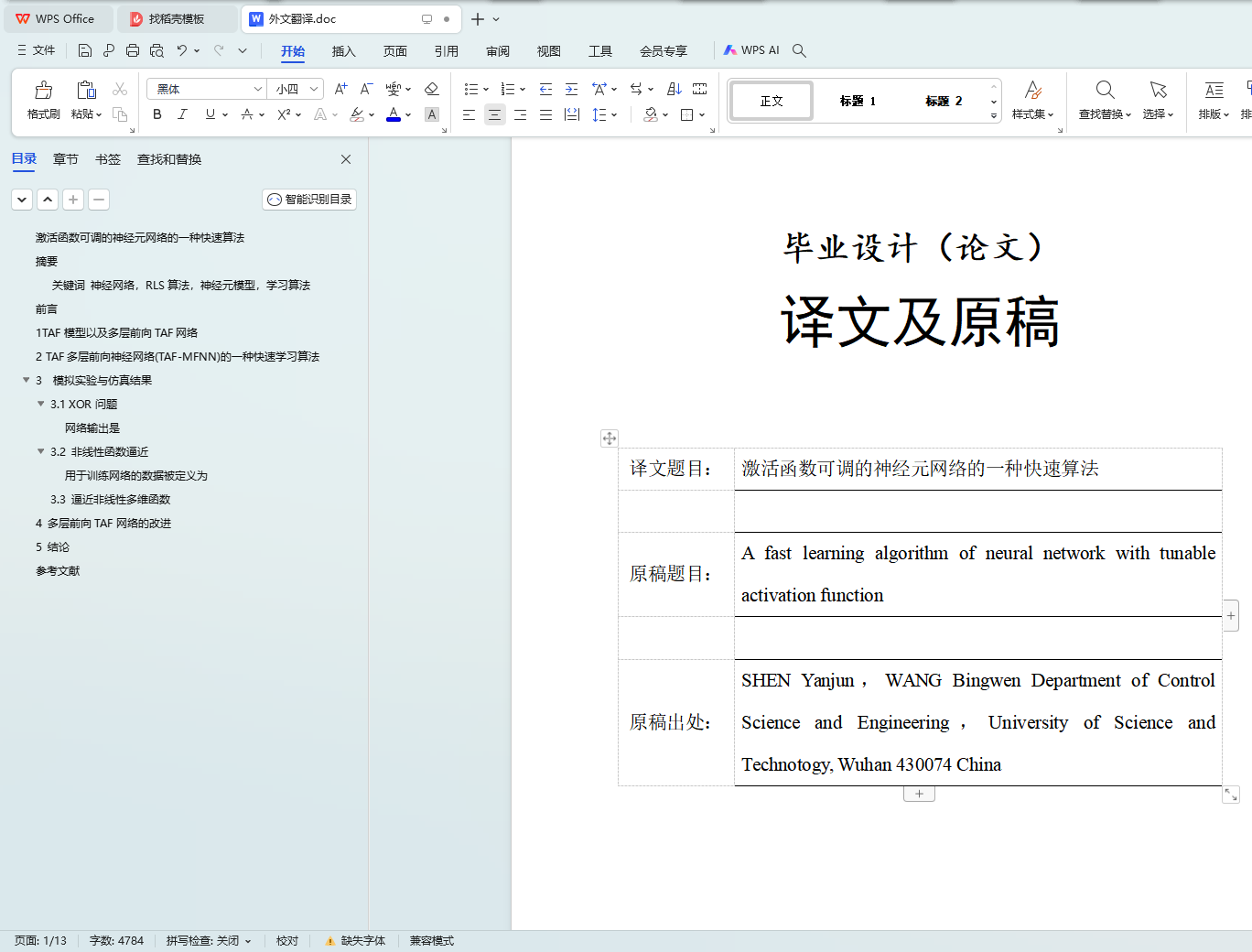
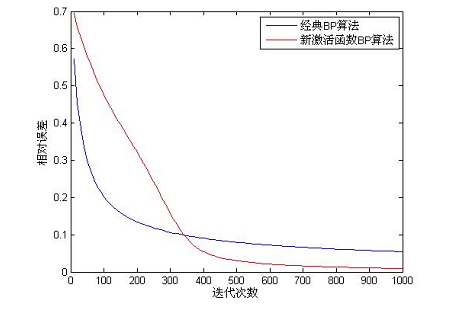
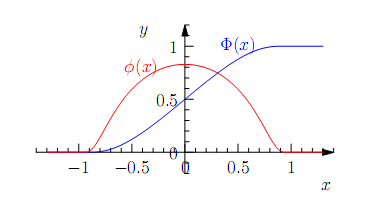
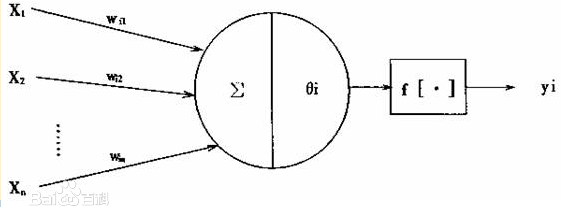
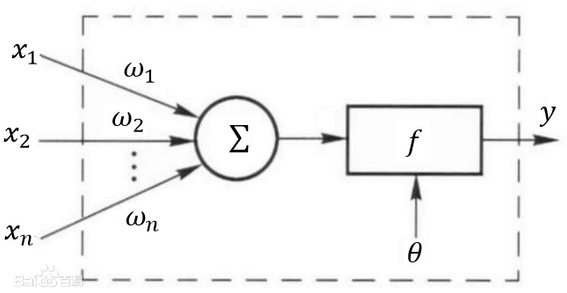
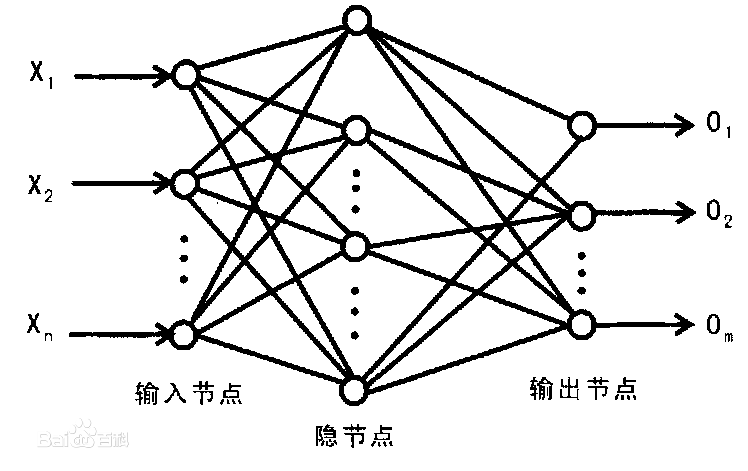