摘 要
目前大规模图计算已经成为大数据处理的核心技术之一,在诸多领域得到了广泛的应用。GPU具有强大的并行计算能力,可以满足图计算存在的高性能及实时性等需求,因此利用GPU来加速图计算的研究得到越来越多的关注。经调研发现,目前基于GPU的图计算系统可以处理的图数据规模有限;系统所采用的整体同步并行计算模型(Bulk Synchronous Parallel Computing Model, BSP)要求顺序执行计算和通信任务,使得系统在计算完成之后需要进行大量线程的同步,而且在执行通信任务时,GPU设备和主机设备都处于空闲,造成计算资源的浪费;GPU设备和主机设备之间都是通过PCIe总线连接,因此PCIe带宽也制约着系统性能。
基于多GPU实现的图计算系统Purin,解决了当前基于GPU的图计算系统存在可以处理数据规模有限、GPU利用率不足和通信开销大等问题。首先,Purin系统设计实现了适合多种编程方式的数据存储和表示方式,并采用基于流式或随机的方式进行数据划分,将划分后的数据块分别传输到不同的GPU设备中。其次,根据划分后顶点的计算特征,将计算任务分成两类,并对其设置不同的优先级。然后,利用异步流技术,设计高效的任务管理机制,对GPU上不同计算任务、GPU-CPU通信任务和CPU上消息聚合任务的执行顺序进行管理。其中,针对GPU上计算任务的执行,提供了两种编程方法以及相应的优化策略。最后,系统收集和整理所有GPU设备的最新的信息,从而得到最终结果。
系统为用户提供了用于实现两种编程方式的编程接口。同时,实验结果表明,Purin图计算系统在单个GPU环境下执行BFS算法时,比Cusha系统有至少3.0倍的性能提升。在多个GPU环境下, 针对大多数测试所用的数据集的处理, Purin系统与当前领先的系统Gunrock相比有明显的性能提升。同时,系统本身表现出良好的可扩展性。
关键词:图计算,GPU,通信开销
Abstract
Large-scale graph processing has been one of the core technologies of big data processing, and has been applied in various fields. GPU has the characteristics of strong parallel capability and high bandwidth, and graph processing technology needs to meet high-performance and real-time requirements. So, many researchers pay more attention on large-scale graph processing on GPU in recent years. However, the problem size which traditional graph processing systems can handle is limited by GPU memory capacity. Also the traditional graph processing systems are developed based on BSP (Bulk Synchronous Parallel Computing Model), which requires the sequential execution of the computer and communication tasks. It results in heavy thread synchronization overhead and wasting the computing resources. What’s more, communication overhead between GPU and CPU restricts the performance of large-scale graph processing on multiple GPUs.
Graph processing system called Purin ,which is based on the multi-GPU, aims to solve the problem that current systems has limited data size, heavy communication overhead and utilization of GPU resources. Firstly, Purin uses simple data representation, and provides random and stream based graph partitioning methods. Then Purin transfers the partitioned data to the GPU devices. Secondly, system assigns the computation task to data graph in each GPU device according to the characteristic of partitioned data, and sets different priorities for different computer tasks. Thirdly, system manages the execution order of different tasks by using the asynchronous streams provided by CUDA. Also system provides two different programming model and some optimization strategies to implement the graph algorithm. Finally, Purin terminates the execution on GPUs and collects the results from all devices.
Purin provides effective APIs to the programmer for easy programming, which hides the details of programming on GPU. Our experiment shows that Purin outperforms other state-of-the-art approach Cusha by over 3.0x when running BFS algorithm on one GPU, and shows significant performance improvement over multi-GPUs based system Gunrock when processing datasets most datasets on multi-GPUs. Also, Purin exhibits good scalability.
Key words: Graph Processing, GPU, Communication Overhead
目 录
摘 要 I
Abstract II
1 绪论
1.1 研究背景 (1)
1.2 国内外研究现状 (2)
1.3 研究内容 (5)
1.4 论文框架结构 (6)
2 系统架构与设计
2.1 GPU概述 (7)
2.2 问题分析 (8)
2.3 系统架构与功能模块 (10)
2.4 系统关键问题与难点分析 (12)
2.5 小结 (14)
3 系统实现及关键技术
3.1 数据存储和数据划分 (15)
3.2 任务划分和任务管理 (18)
3.3 编程模型和编程接口 (24)
3.4 小结 (29)
4 系统测试和性能分析
4.1 实验测试环境、数据和算法 (30)
4.2 系统性能测试与分析 (32)
4.3 小结 (38)
5 总结与展望 (39)
致 谢 (42)
参考文献 (44)
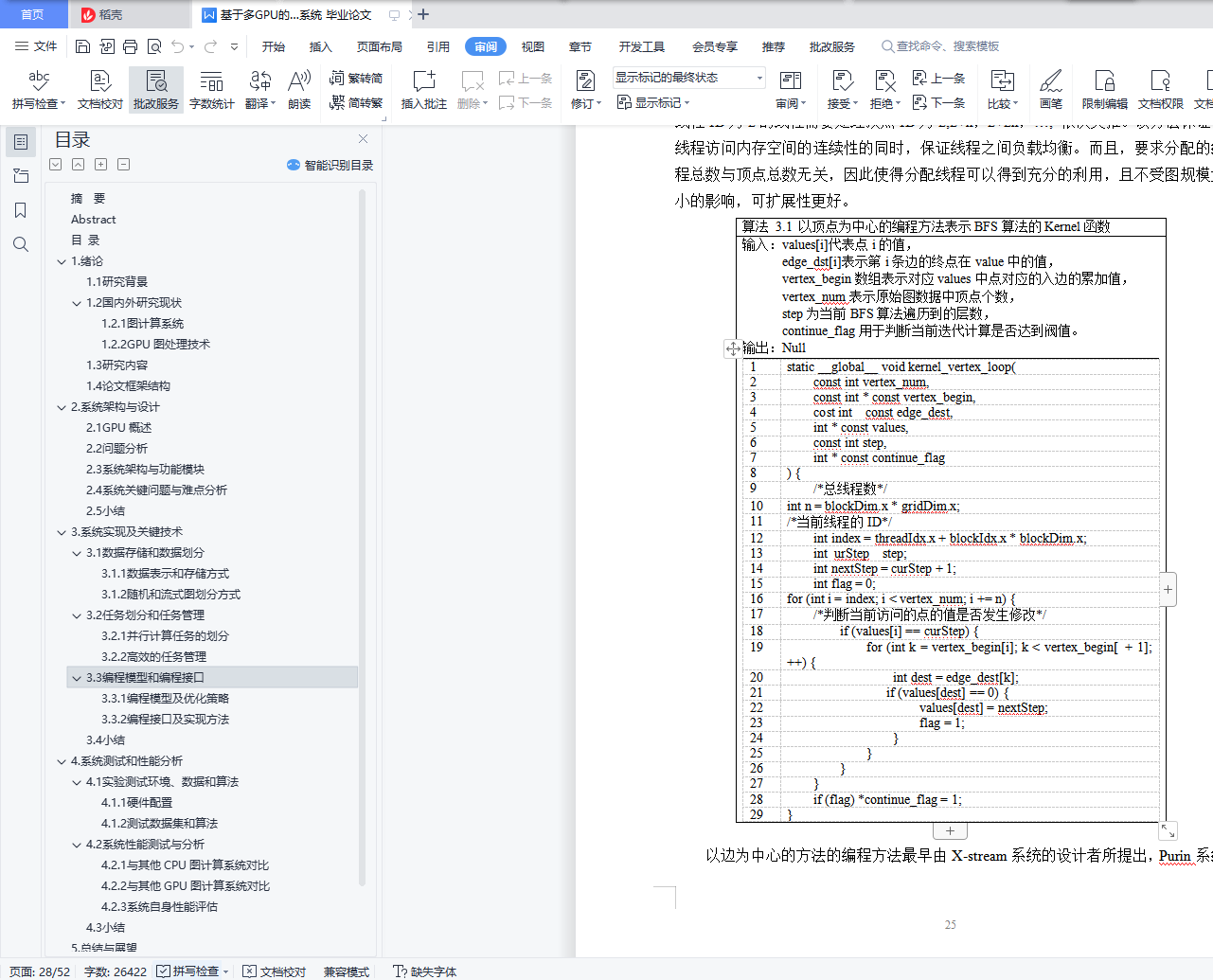
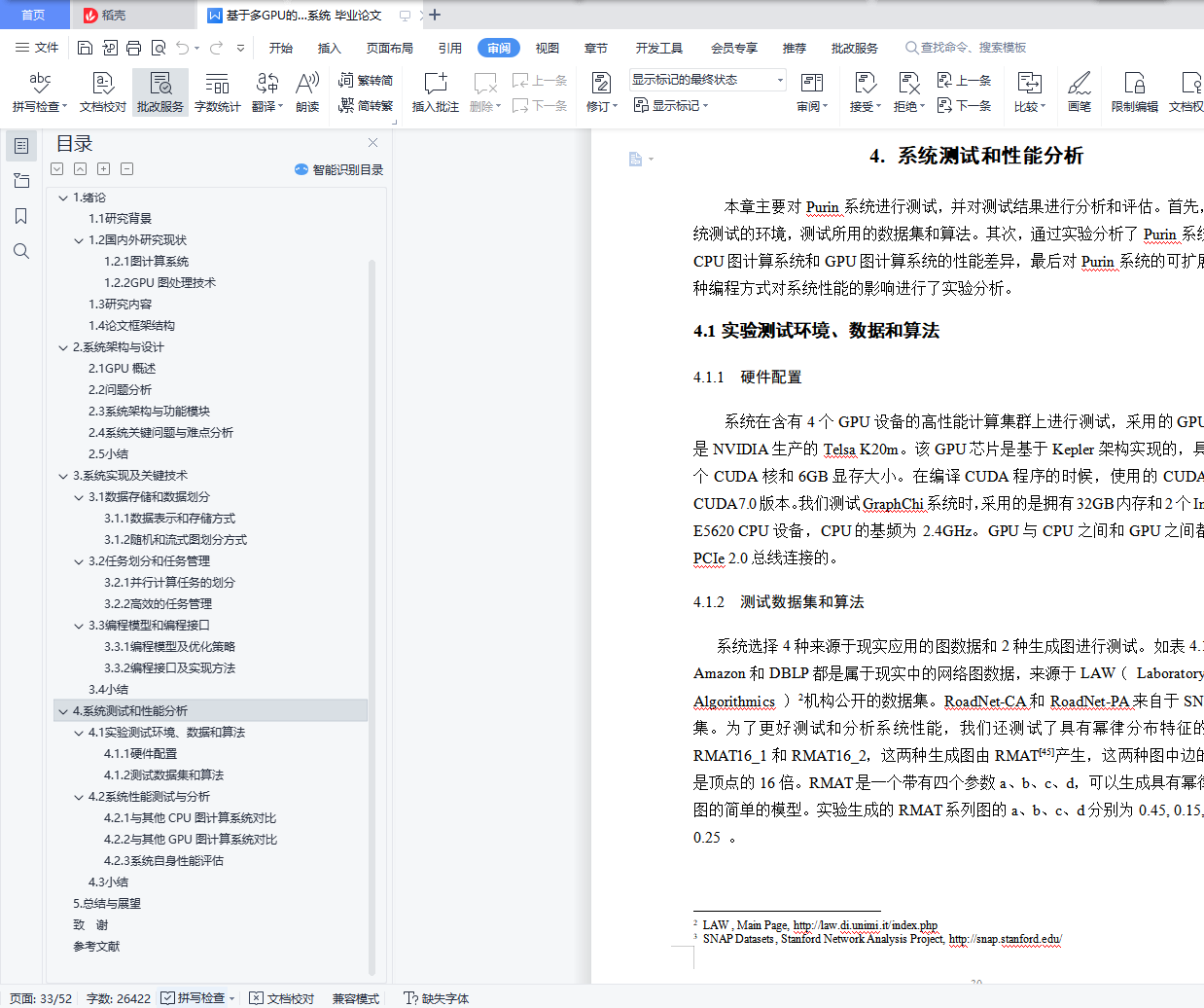
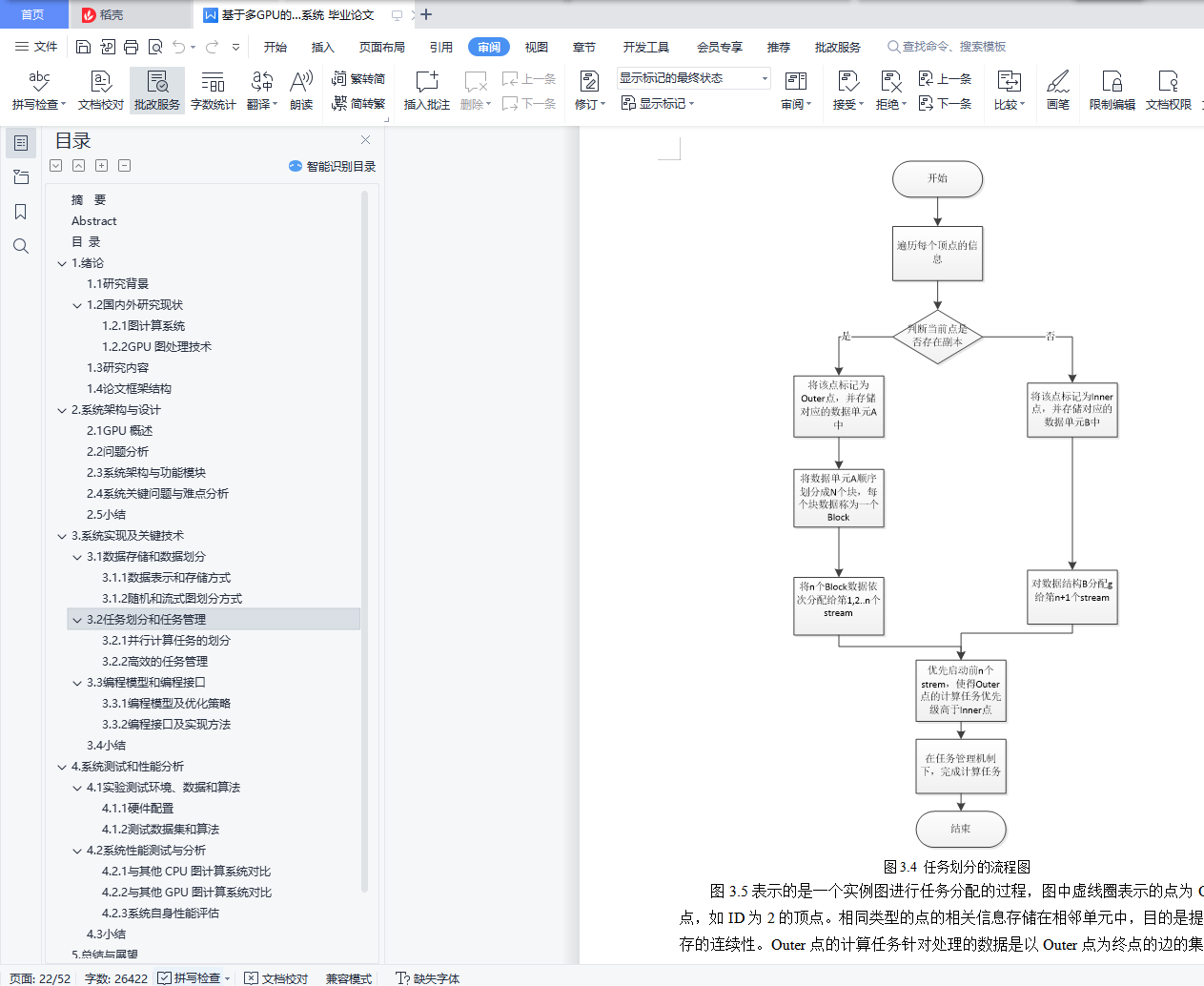
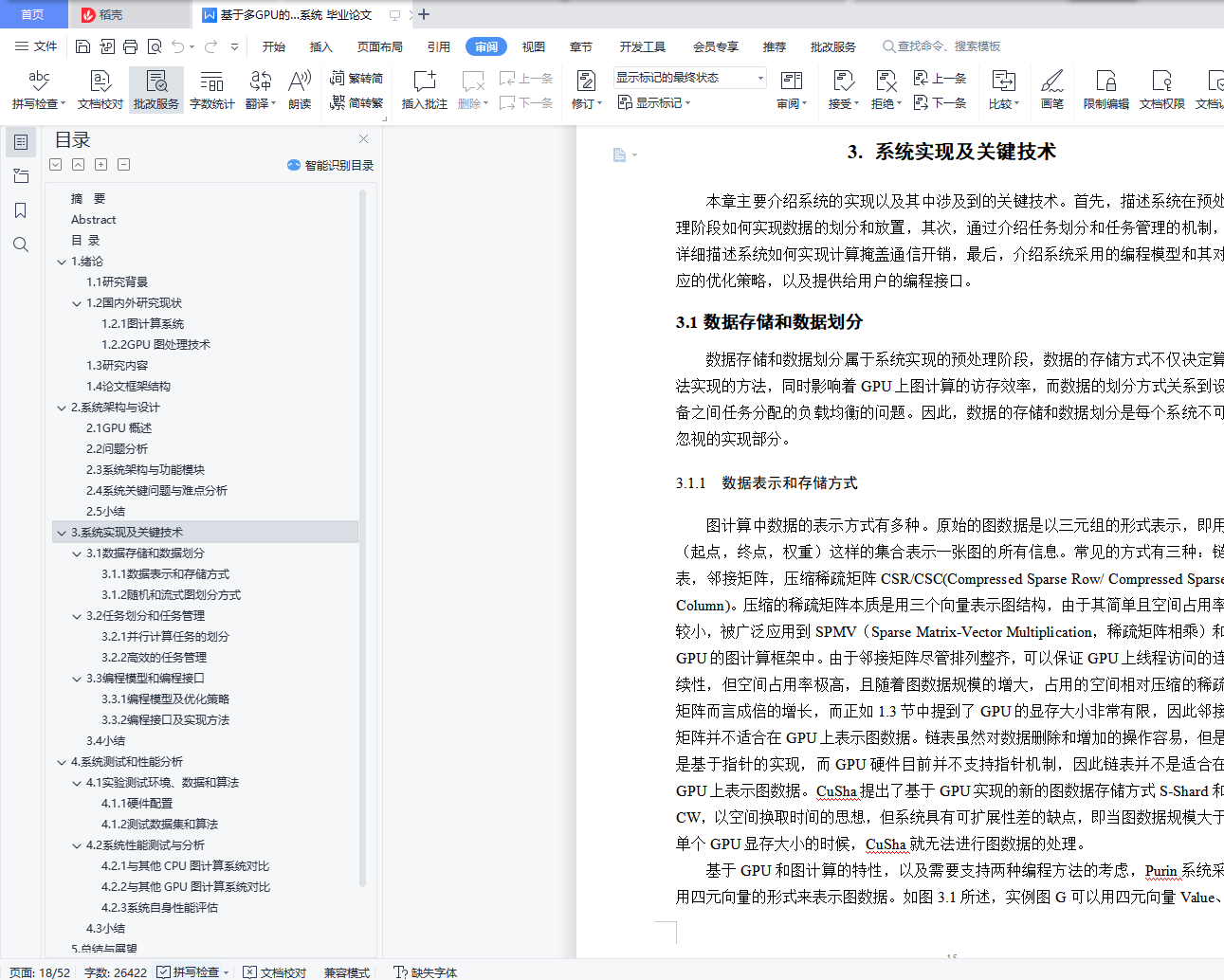
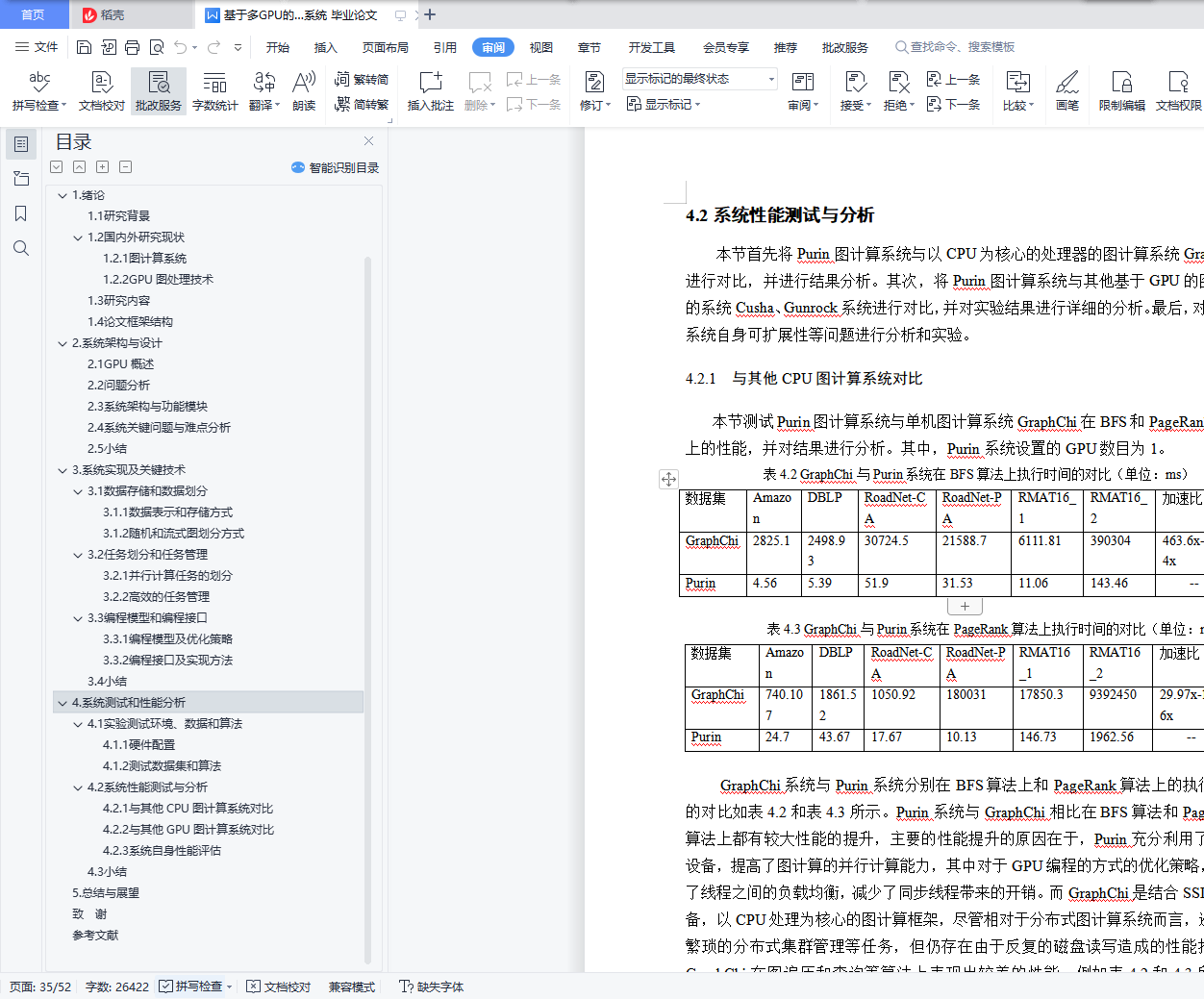
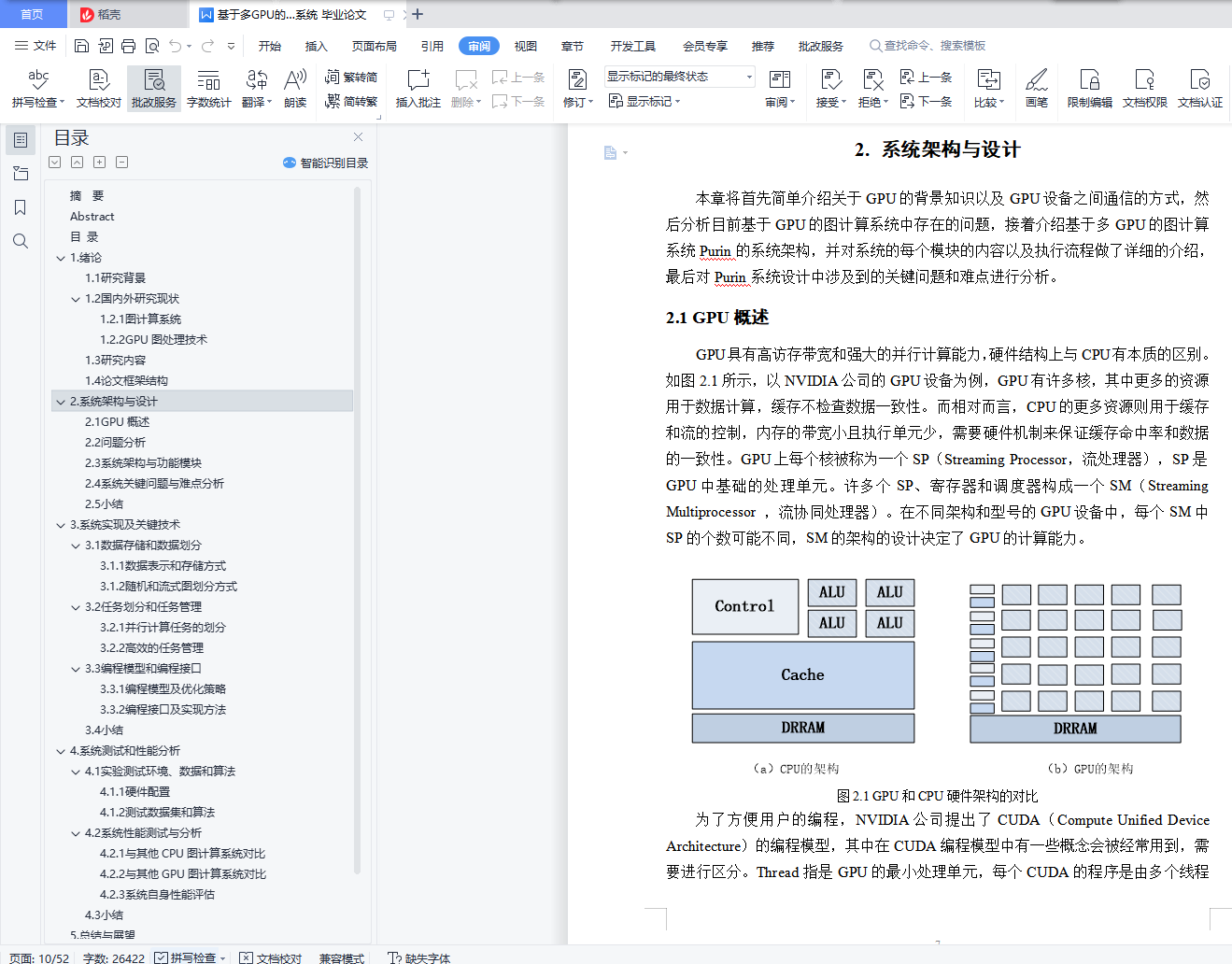
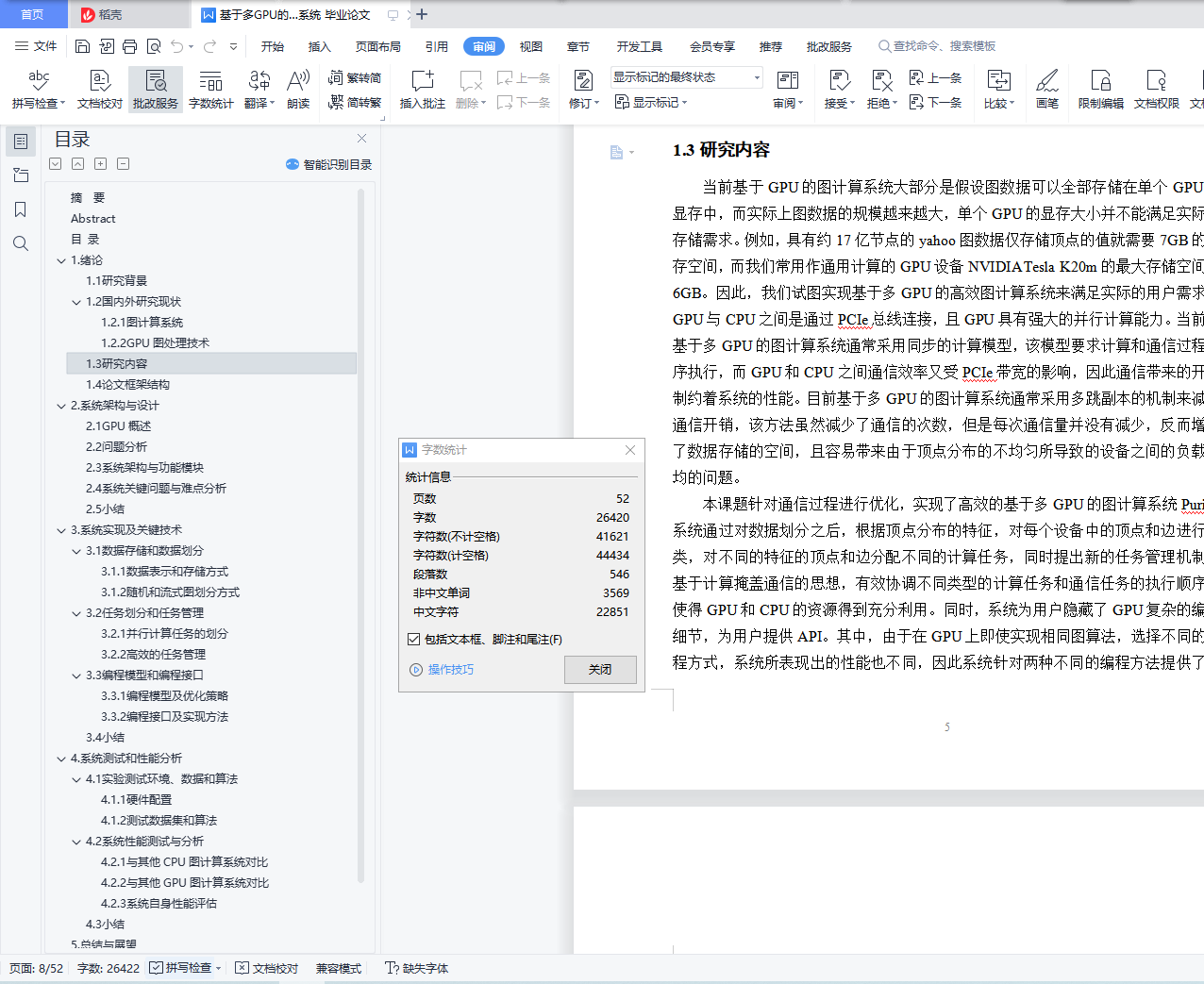
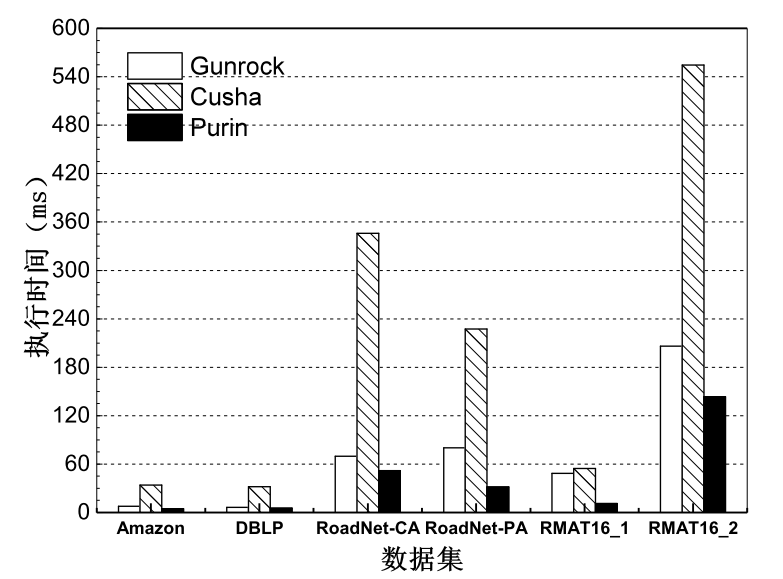
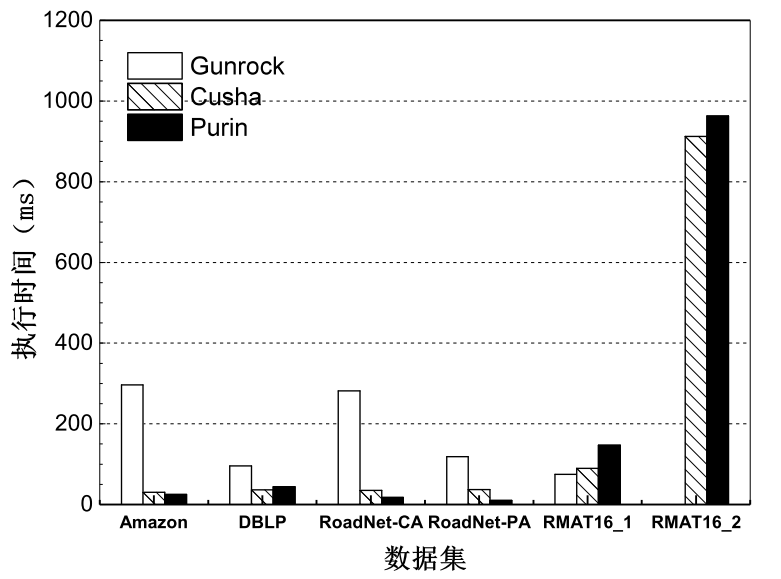
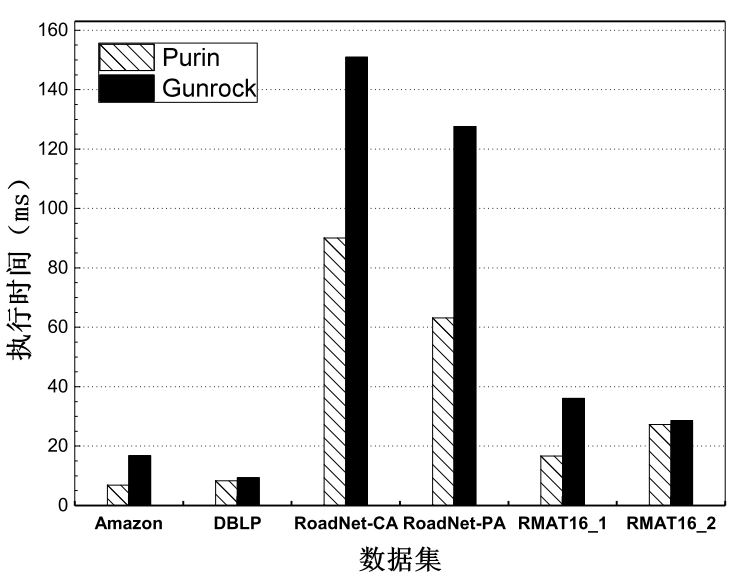
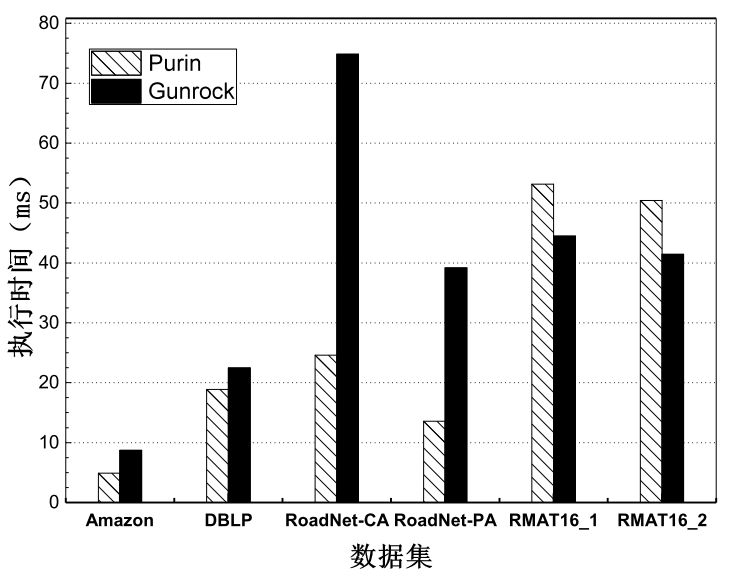
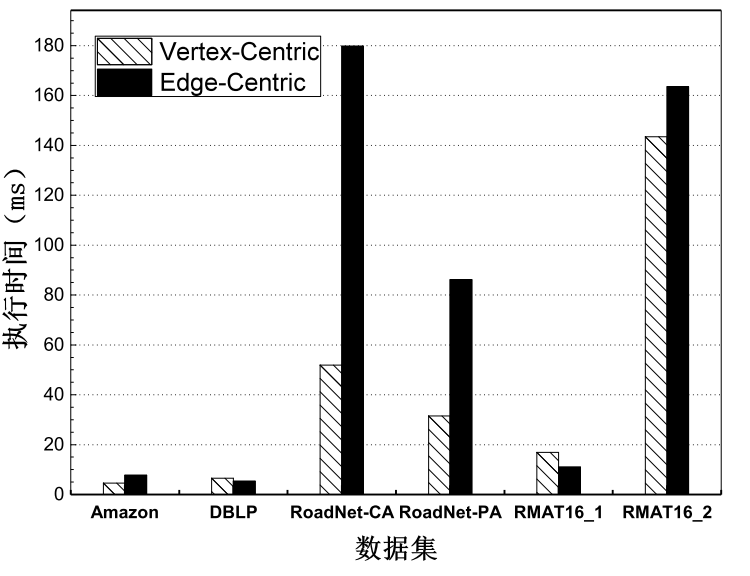
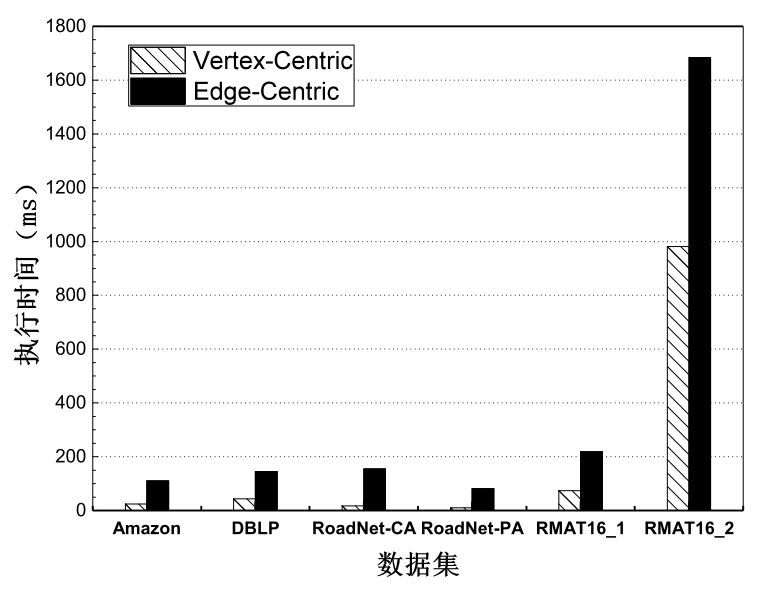
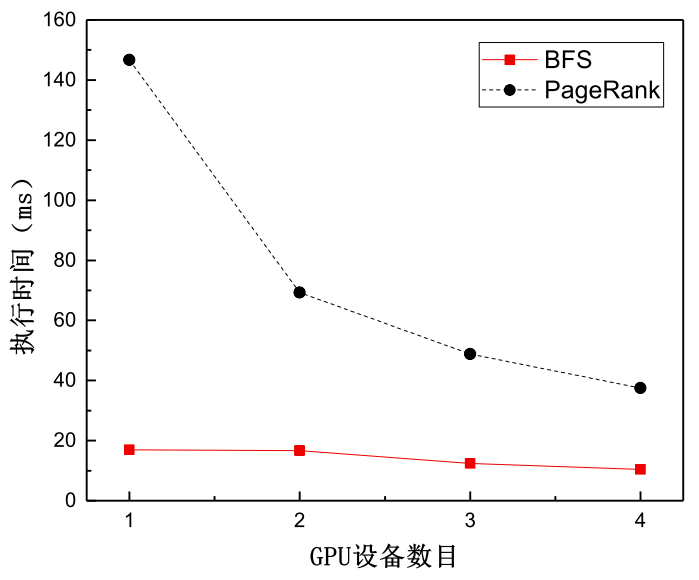