摘 要
在互联网时代下,信息量呈指数级增长。海量数据以0、1 方式编码存放在磁盘等设备中非常方便,但是对于人类来说,我们的脑容量有限,在海量数据中挑选出适合自己的信息变得非常困难。简直如大海捞针,即使使用了分类方法和搜索引擎,我们还是要花大量时间去挑选适合自己的信息,并且经常选取了错误的信息。在这样的情况下, 个性化推荐系统应运而生。从起初的基于内容的推荐,到现在最常用的协同过滤推荐等推荐算法,我们从繁琐庞大的信息中解脱出来,可以接受大量系统推荐给我们的信息。
在大多数电子商务网站上,都运行着个性化推荐系统,不仅提高了用户购买率,还提高了用户体验。其系统内部实现由于基础数据不同也各有不同,而协同过滤算法被广泛应用到这些系统中,发挥着巨大的作用。
本文首先详细介绍了协同过滤算法与推荐系统概要,分析了各种协同过滤算法的优缺点。然后设计了一个简易的电影资源推荐系统,并使用 python、微信公众号等技术实现了这个推荐系统,最后对这个推荐系统做了简单的测试和结果分析。
关键词:个性化推荐系统;协同过滤;机器学习;数据挖掘;数据分析;python
Abstract
In the Internet age, the amount of information is exponential. Huge amounts of data to 0, 1, encoded in devices such as disk is very convenient, but for humans, our brain capacity is limited, selected for their own information in the huge amounts of data becomes very difficult.It's like looking for a needle in a haystack, and even using a taxonomy and search engine, we still have to spend a lot of time sorting out what's right for us and often picking the wrong information. In this case, the personalized recommendation system comes into being.Based on the recommendations from the content, from the beginning to now, the most commonly used collaborative filtering recommendation recommendation algorithm, such as free from complex huge information, we can accept a large number of system is recommended for our information.
On most e-commerce sites, the personalized recommendation system is running, which not only improves the user purchase rate, but also improves the user experience.The internal implementation of the system is different due to the different basic data, and the collaborative filtering algorithm is widely applied to these systems and plays a huge role.
In this paper, we first introduce the collaborative filtering algorithm and recommendation system profile, and analyze the advantages and disadvantages of various collaborative filtering algorithms.Then design a simple movie recommendation system resources, and using the python, micro letter of public technology implements this recommendation system, finally, the recommendation system has made the simple test and the result analysis.
Key words:Personalized recommendation system;Collaborative filtering;Machine learning;Data mining;Data analysis;Python
目 录
摘 要 I
Abstract II
第一章 绪论 1
1.1 课题背景及意义 1
1.2 国内外研究现状 2
1.3 论文内容结构 2
第二章 协同过滤与相关技术 3
2.1 推荐算法介绍 3
2.1.1 基于内容的推荐算法 3
2.1.2 协同过滤算法 4
2.1.3 协同过滤算法分类 4
2.1.4 协同过滤优化 5
2.1.5 协同过滤推荐的优缺点 5
2.1.6 应用场景 7
2.2 相关技术介绍 7
第三章 系统分析与设计 9
3.1 需求分析 9
3.2 系统设计 10
3.2.1 微信公众号 11
3.2.2 接口定义 12
3.2.3 前后端交互接口 13
3.2.4 文本请求 14
3.2.5 事件处理 14
3.2.6 web服务器 15
3.3 数据库设计 17
3.3.1 电影信息表 17
3.3.2 其他表结构 17
3.4 推荐算法设计 18
第四章 系统实现 20
4.1 数据采集 20
4.1.1 爬虫概要 20
4.1.2 数据库结构 22
4.1.3 爬虫主要逻辑 23
4.1.4 页面分析 24
4.1.5 数据处理 25
4.2 基础功能实现 26
4.2.1 后端主要逻辑 26
4.2.1 搜索功能 30
4.2.2 评价功能 32
4.3 协同过滤推荐实现 35
4.3.1 读取用户曲线 35
4.3.2 用户相似程度 36
4.3.3 获得最终结果 37
4.4 解决冷启动问题 38
4.4.1 解决方案 38
4.4.2 代码实现 39
4.4.3 其他完善方案 39
第五章 实验结果分析 40
5.1 推荐功能测试 40
5.1.1 协同过滤推荐 40
5.1.2 基于内容推荐 41
5.2 推荐功能优化 43
5.2.1 重复推荐 43
5.2.1 响应时间 43
5.2.2 避免冷启动 43
5.3 评价功能优化 43
5.3.1 影片名纠纷 44
5.3.2 鼓励评分 44
5.4 其他辅助功能 44
5.4.1 功能说明书 44
5.4.2 收藏夹 45
总 结 46
参考文献 47
致 谢 48
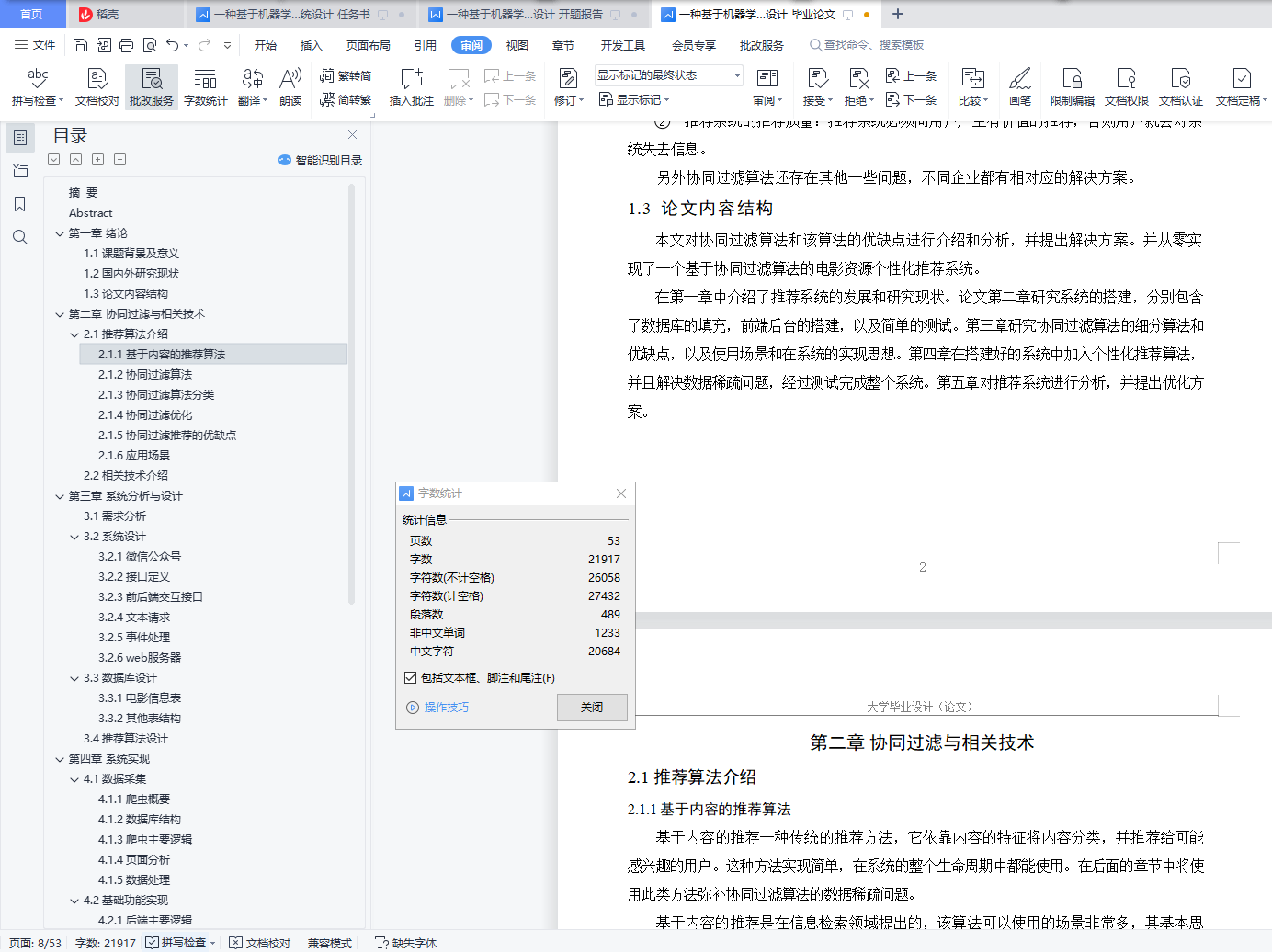
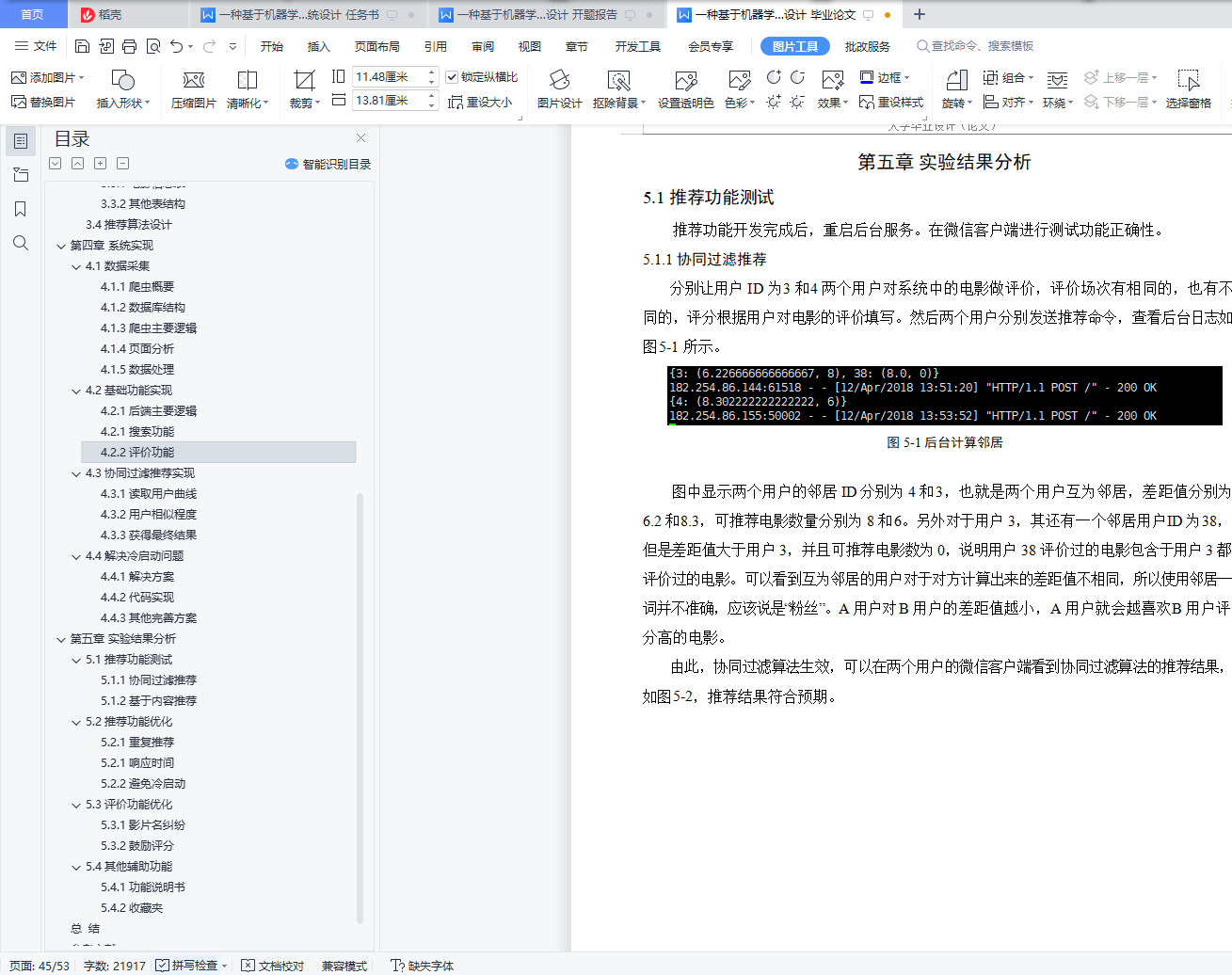
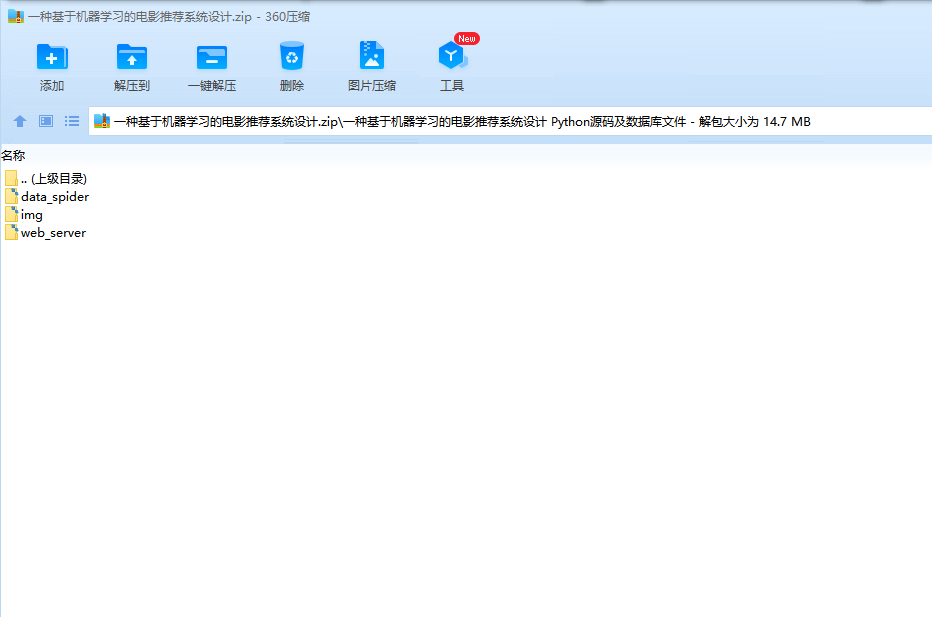
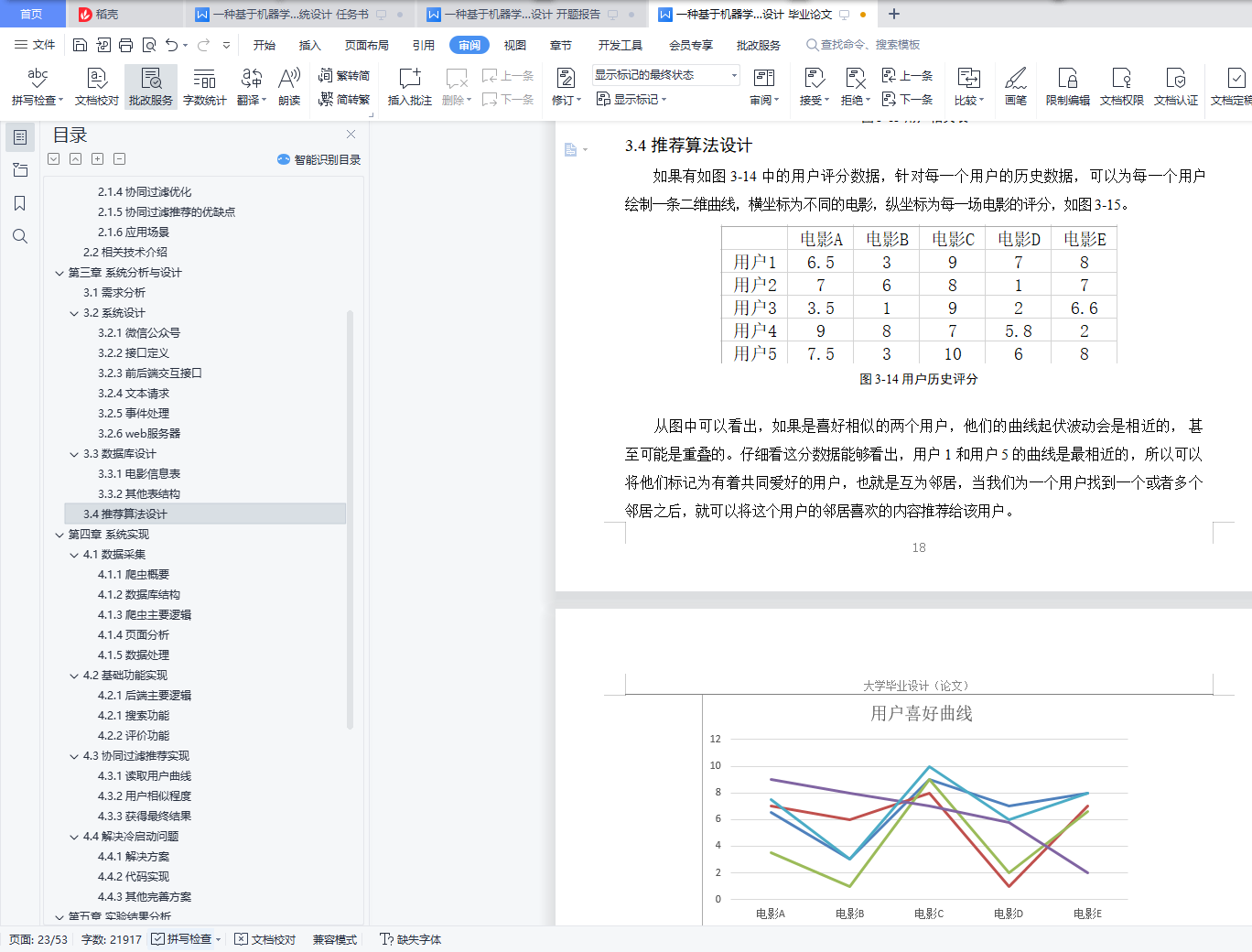
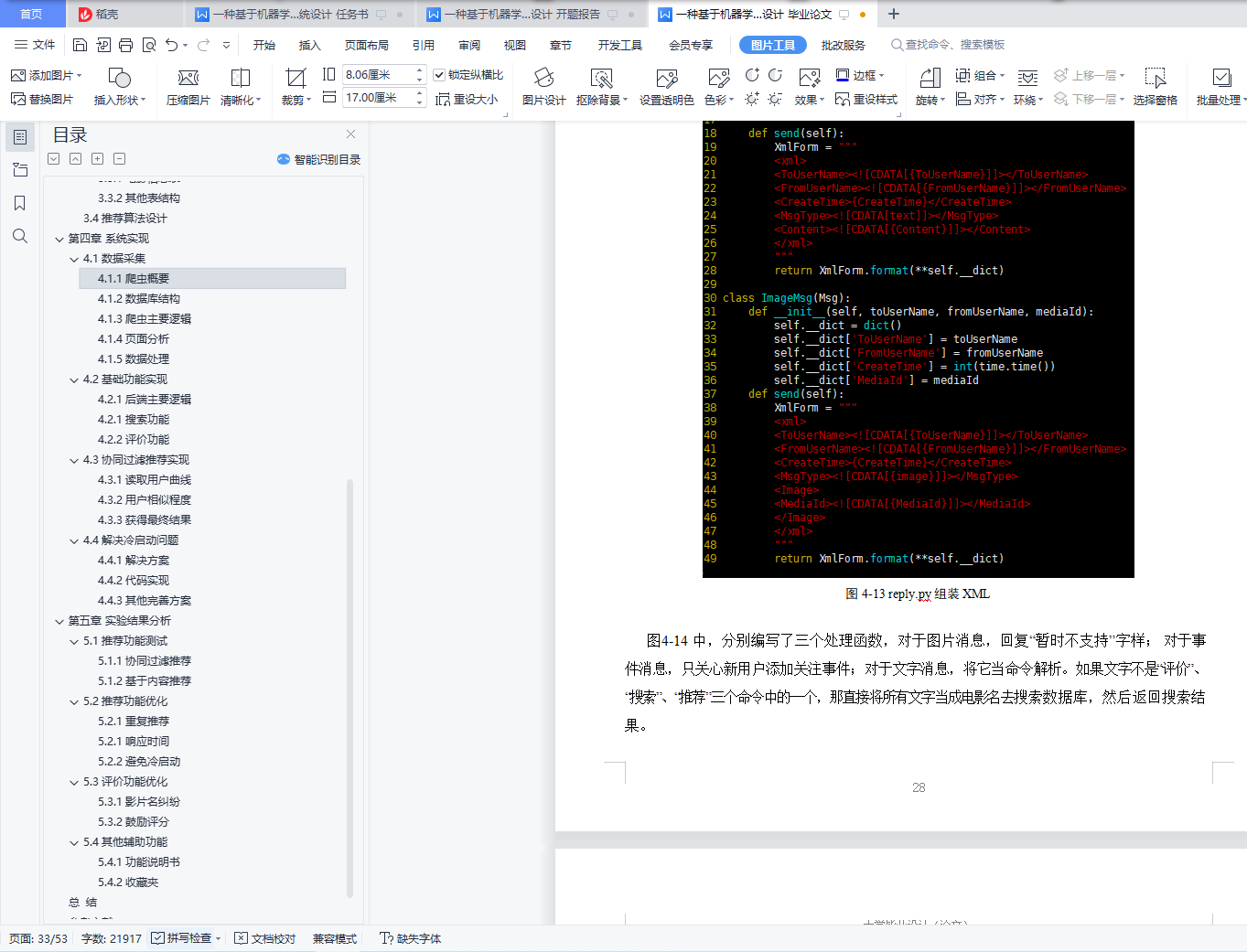
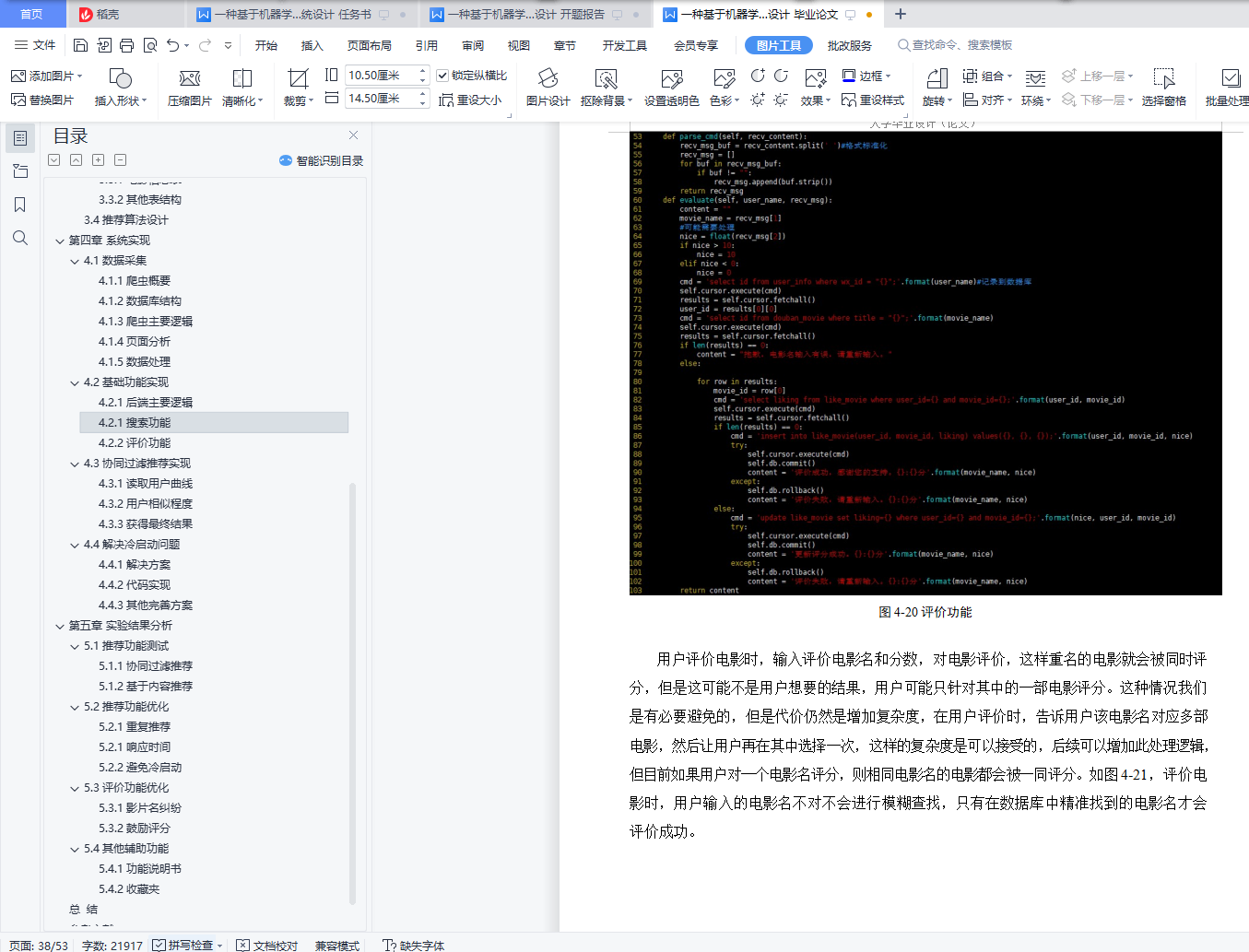
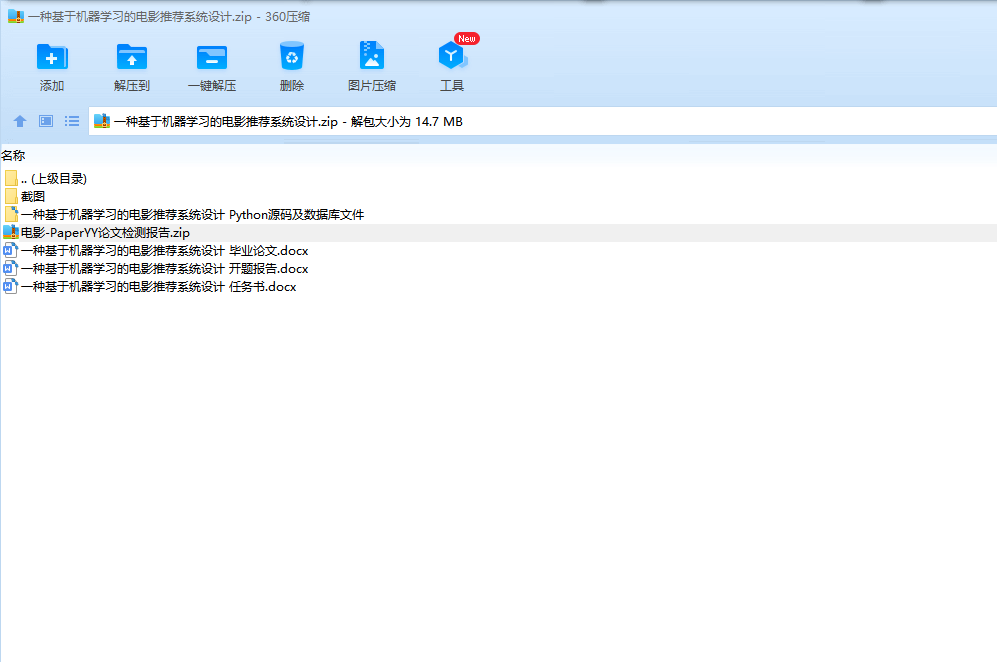
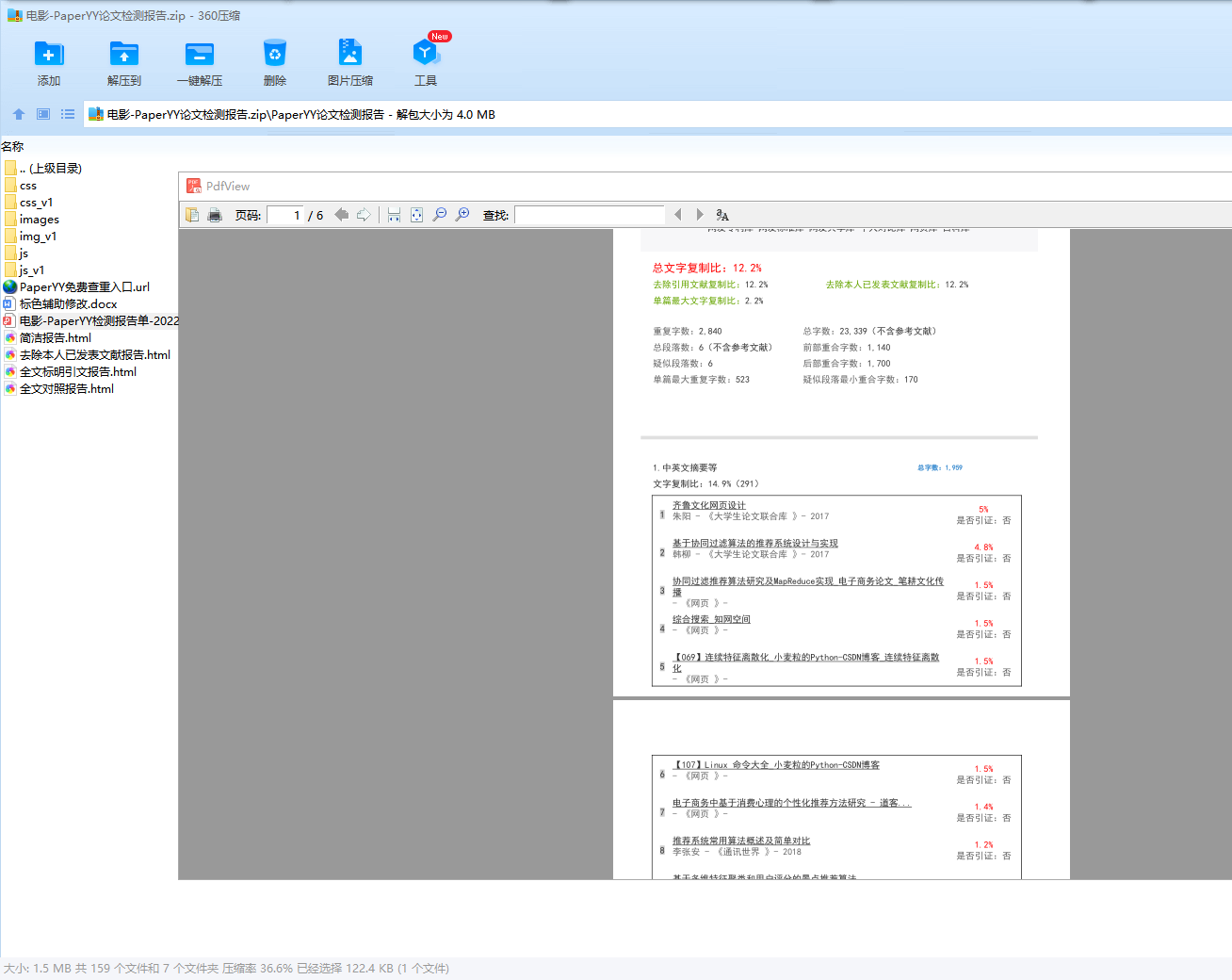
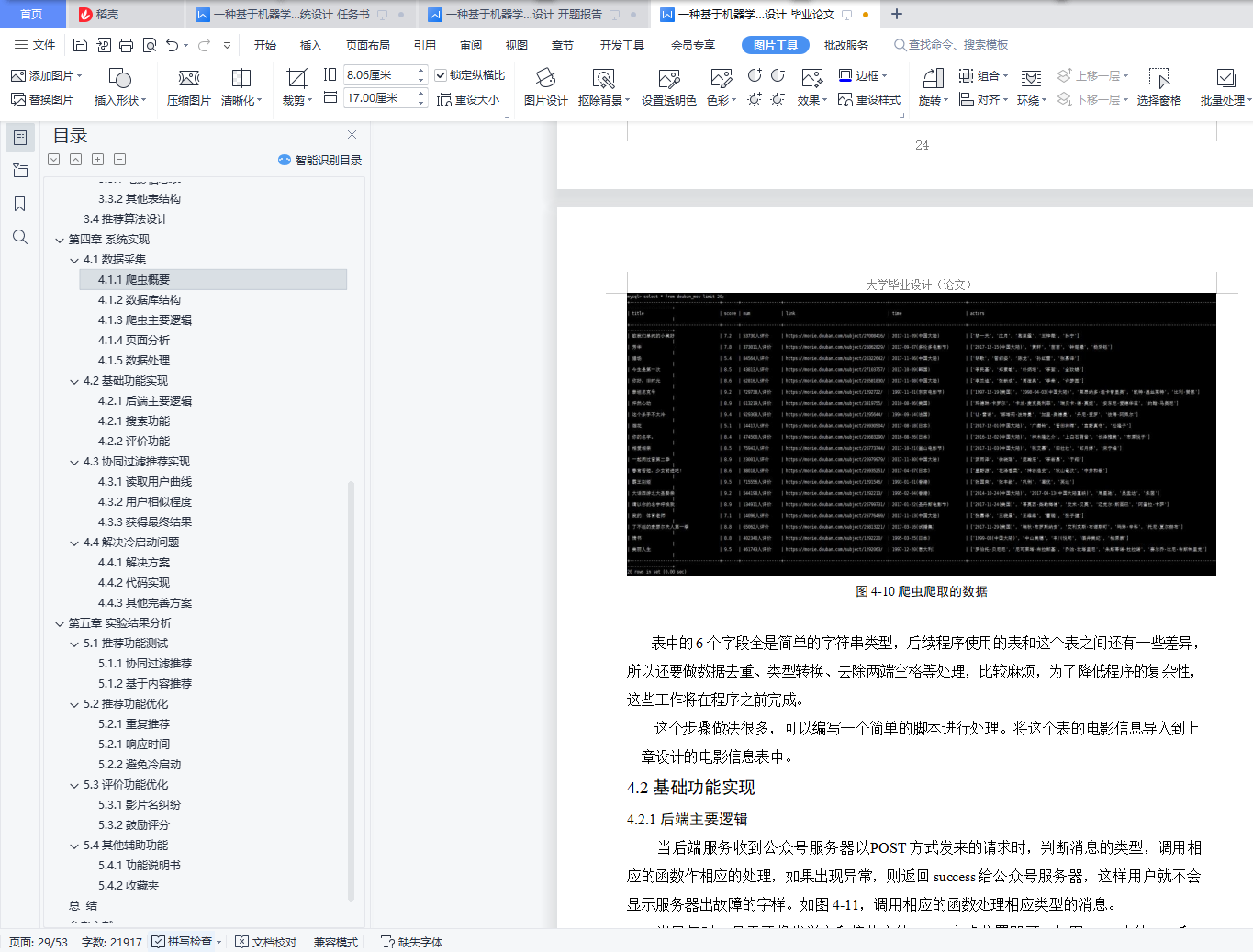
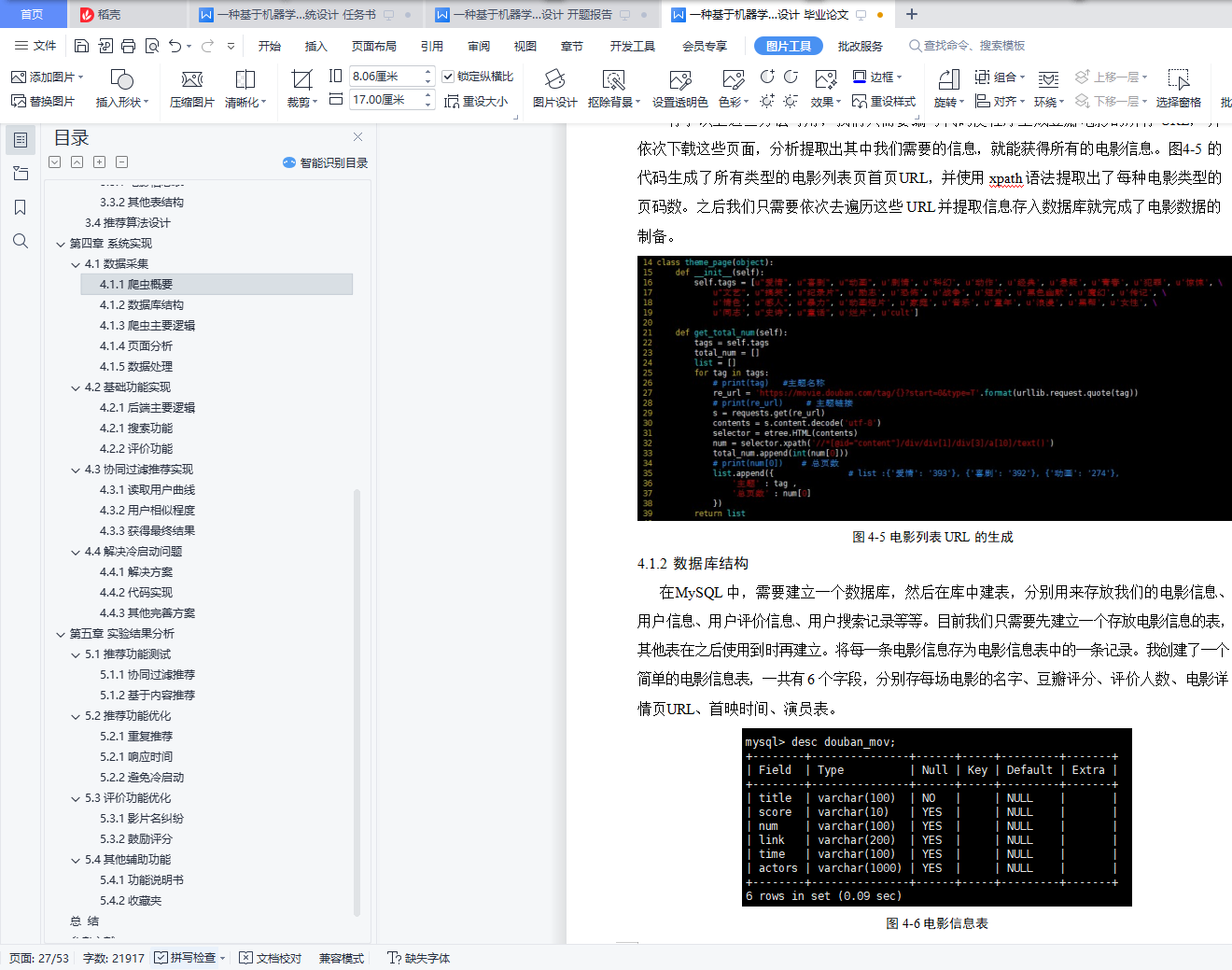
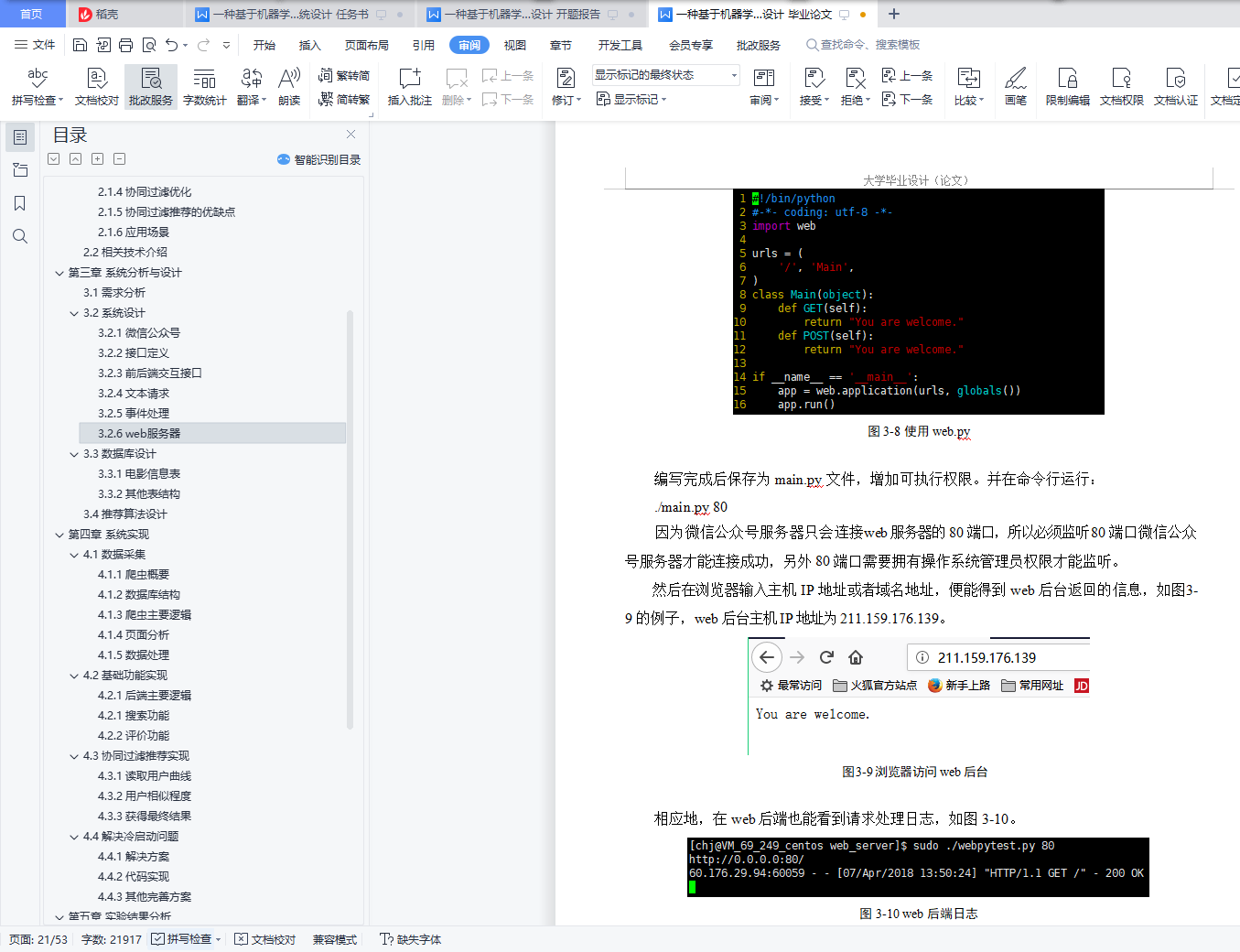
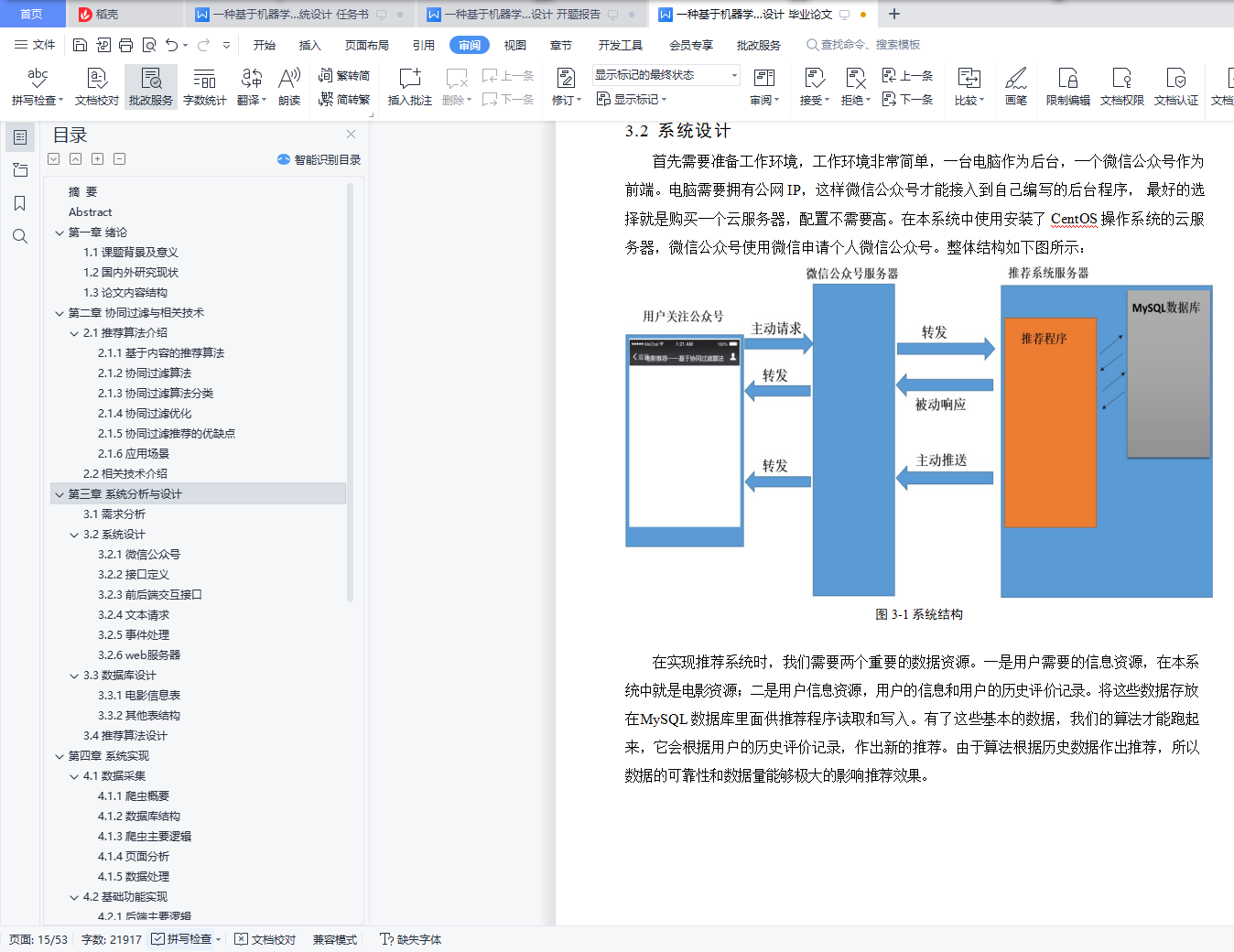
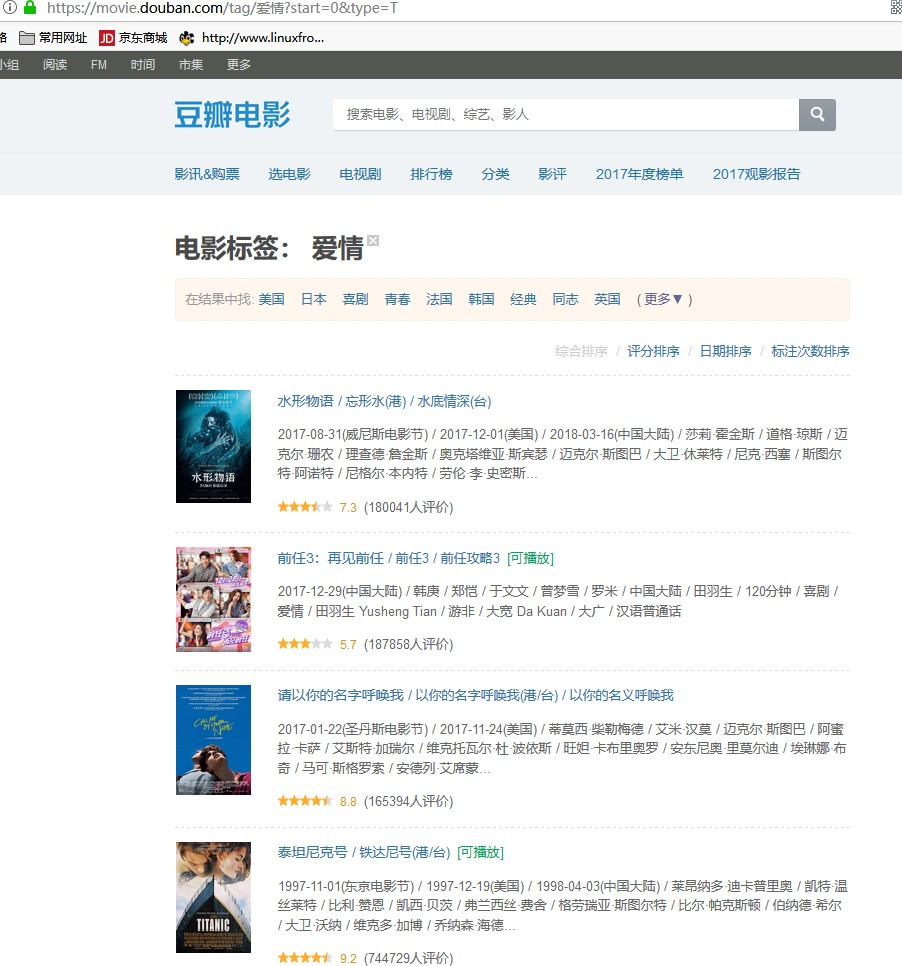
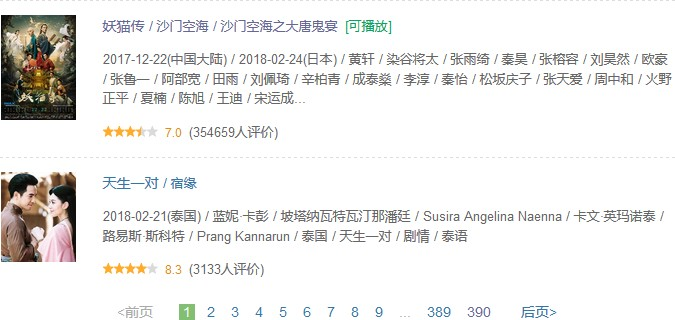
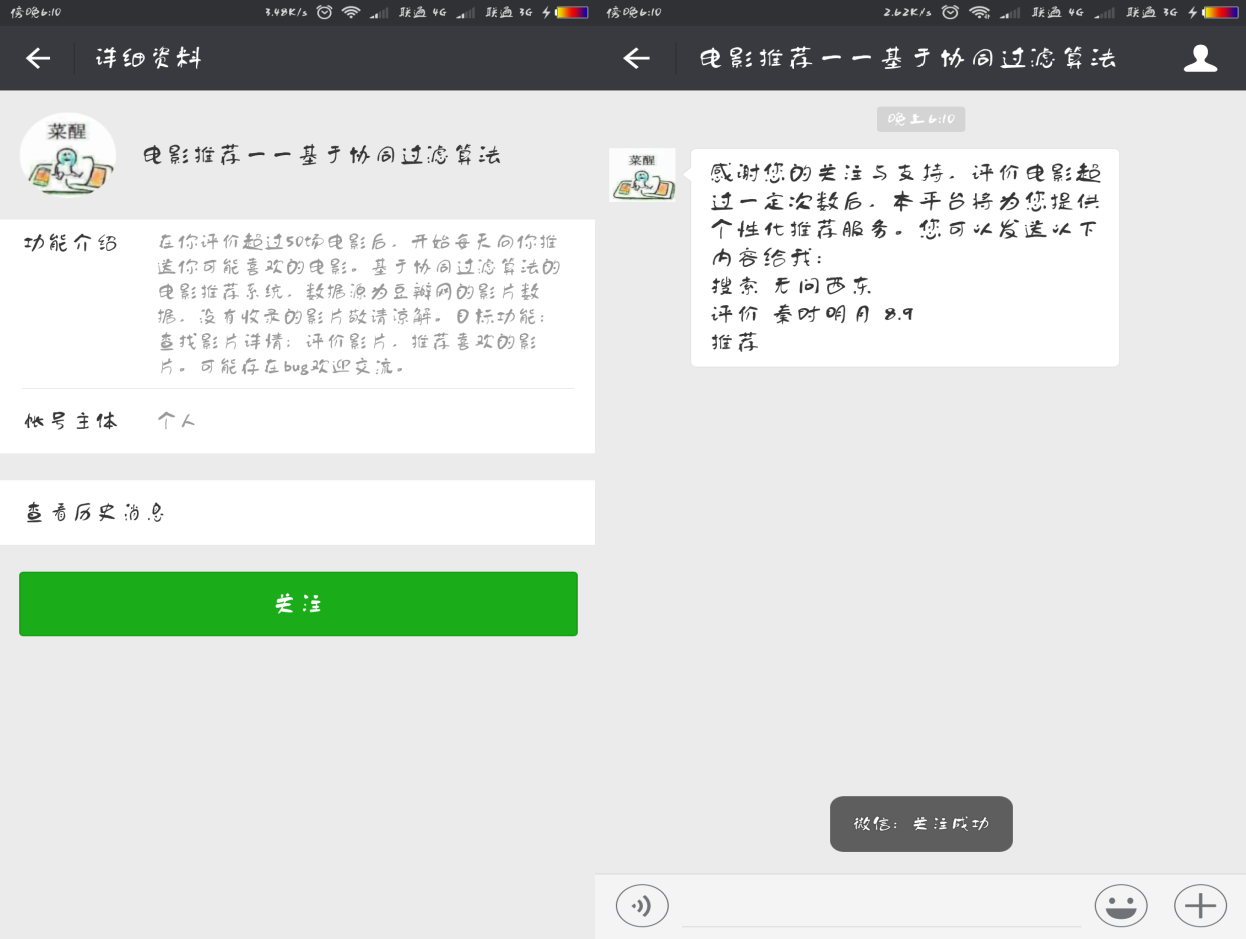
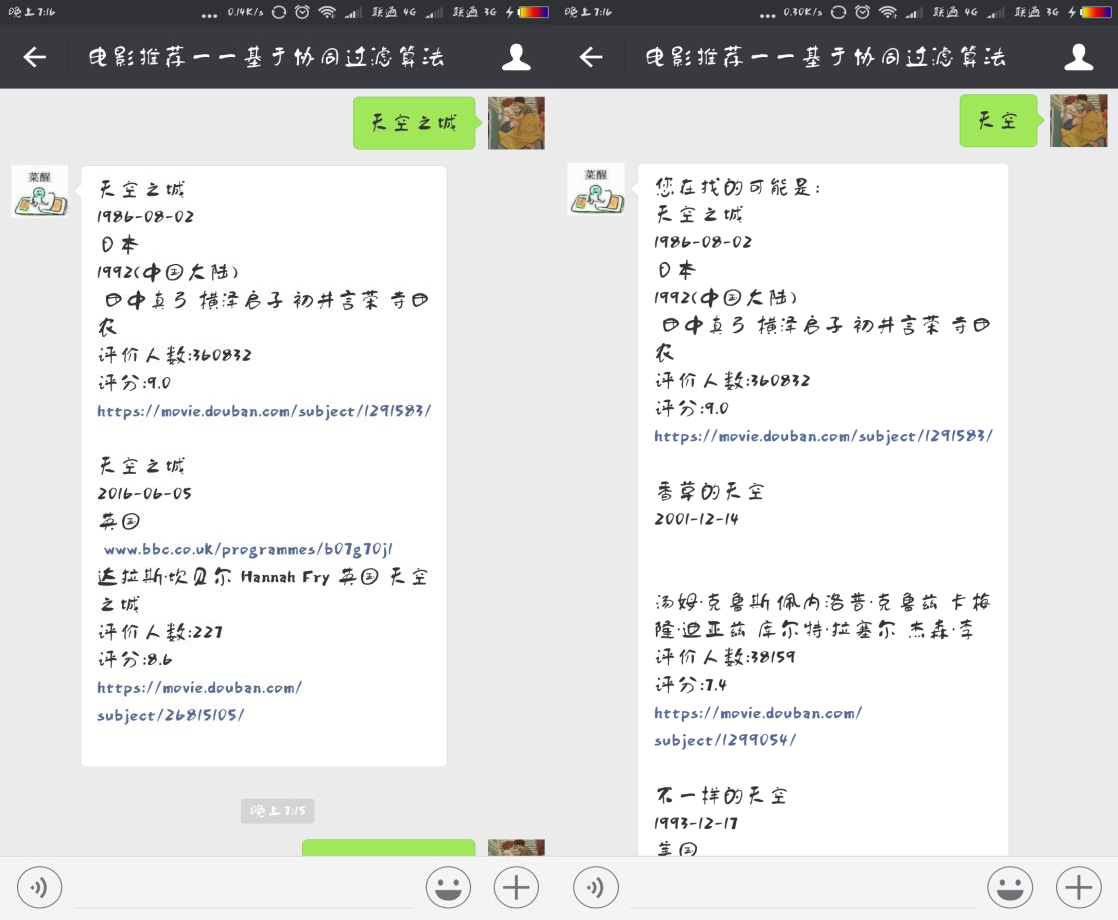
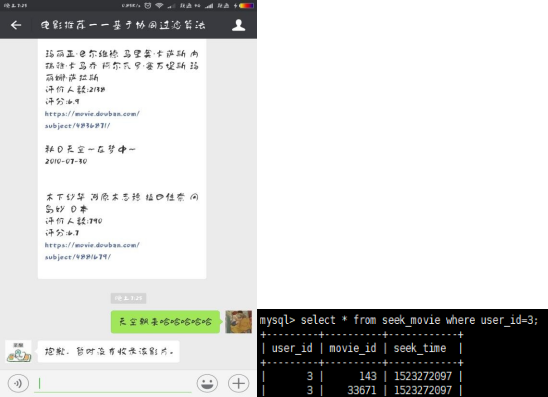

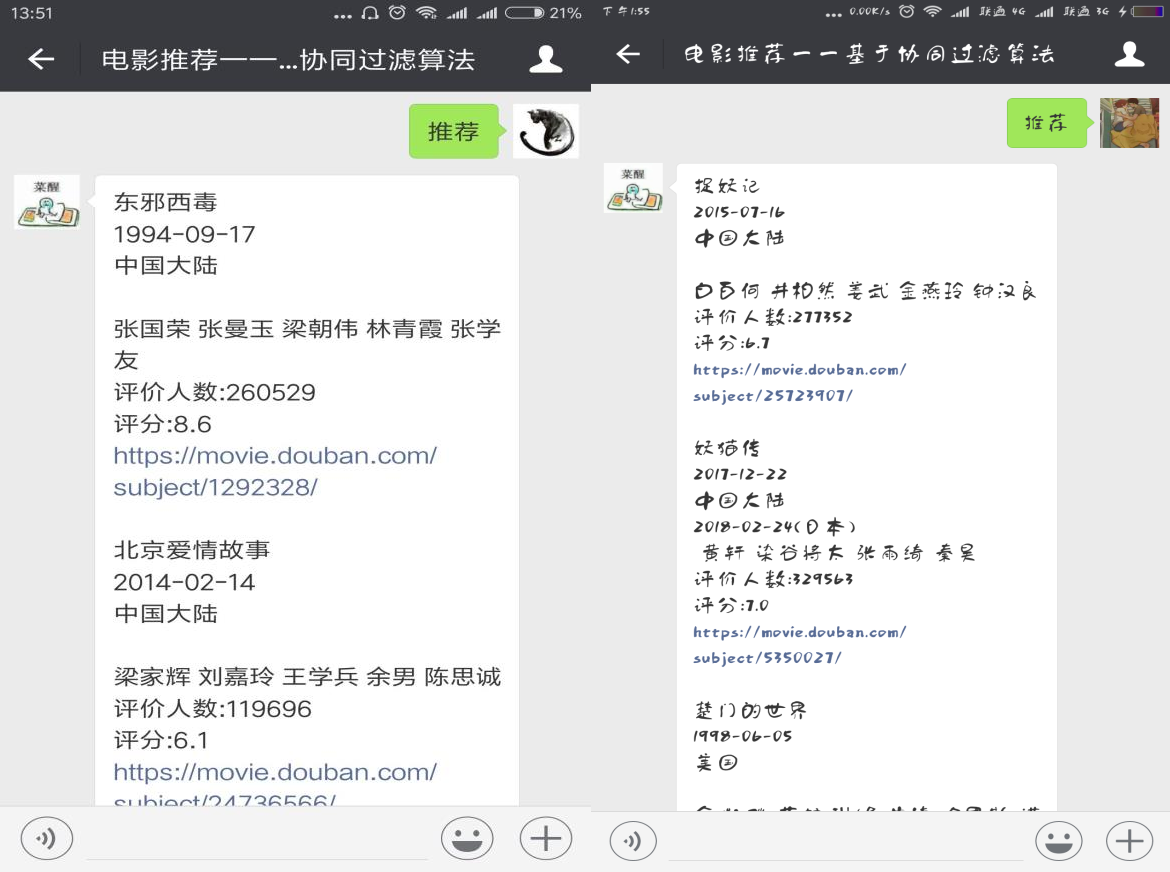
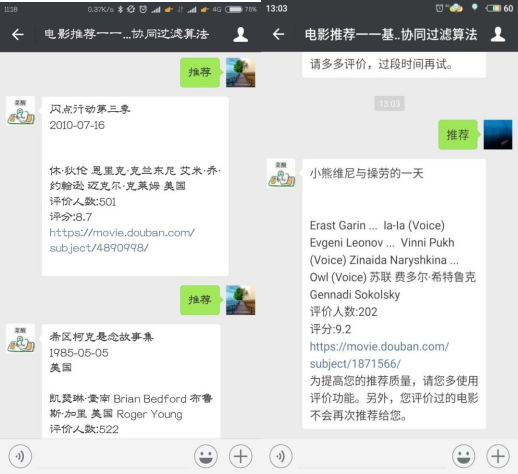