为短文本推荐合适的emoji — 基于上下词及语境
的CBOW多层神经网络分类模型研究
Abstract Aiming at the feature sparsity and context dependence of short texts, this paper proposes a short text classification method based on CBOW multilayer neural network. Using the sentiment tendency of short text itself, this paper adopts TF-IDF-CF characterization method, on the other hand, associates the short text context with the CBOW model to optimize the emoji of the chat data. Experiments show that this hybrid model is higher in classification performance better than the traditional Bayesian or SVM classification model. In the Kaggle competition private leaderboard it achieved a higher accuracy of 0.17554.
Key words Short text classification CBOW model Improved weight TF-IDF-CF method Multi-layer neural network classification Data Mining
摘要 针对短文本的特征稀疏性和上下文依赖性等特点, 本文提出一种基于CBOW多层神经网络的短文本分类方法. 利用短文本本身的情感倾向, 一方面采用改进权值的TF-IDF-CF特征化方法, 另一方面关联短文本上下文语境运用CBOW模型对聊天数据进行最优emoji推荐. 实验表明这种混合模型在分类性能上比传统的贝叶斯或支持向量机分类模型高出很多, 在Kaggle竞赛private榜上取得0.17554的较高准确率.
关键词 短文本分类 CBOW模型 改进权值TF-IDF-CF方法 多层神经网络分类 数据挖掘
目录
1引言 2
2具体方法 2
2.1预处理 2
2.2特征选择方法 TF-IDF 3
2.3朴素贝叶斯分类器 3
2.4词向量 4
2.5CBOW模型 5
2.5.1CBOW模型实现细节 6
3难点与方法选择动机 7
3.1难点①: 短文本特征化 7
3.1.1改进权值的TF-IDF方法选择动机 9
3.2难点②: 发现与创造优秀的模型 10
3.2.1CBOW模型选择动机 10
3.3难点③: 构建神经网络训练模型 11
4 总结 12
5 说明点及复现代码说明 13
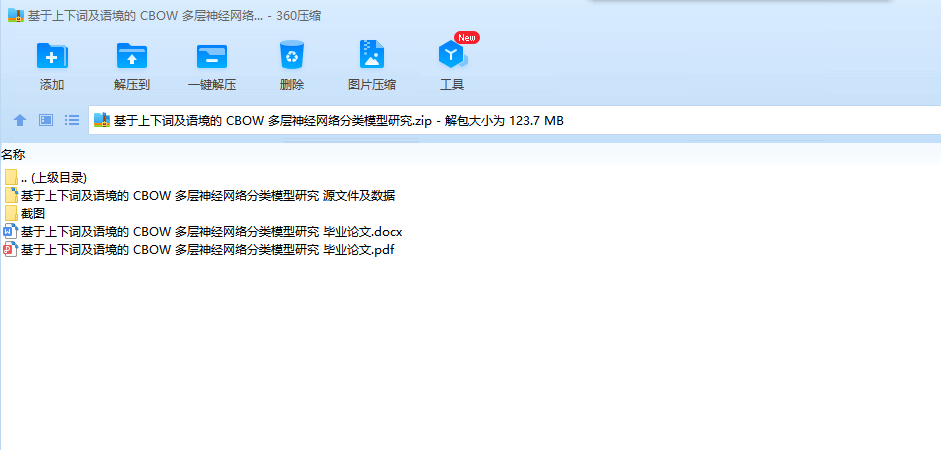
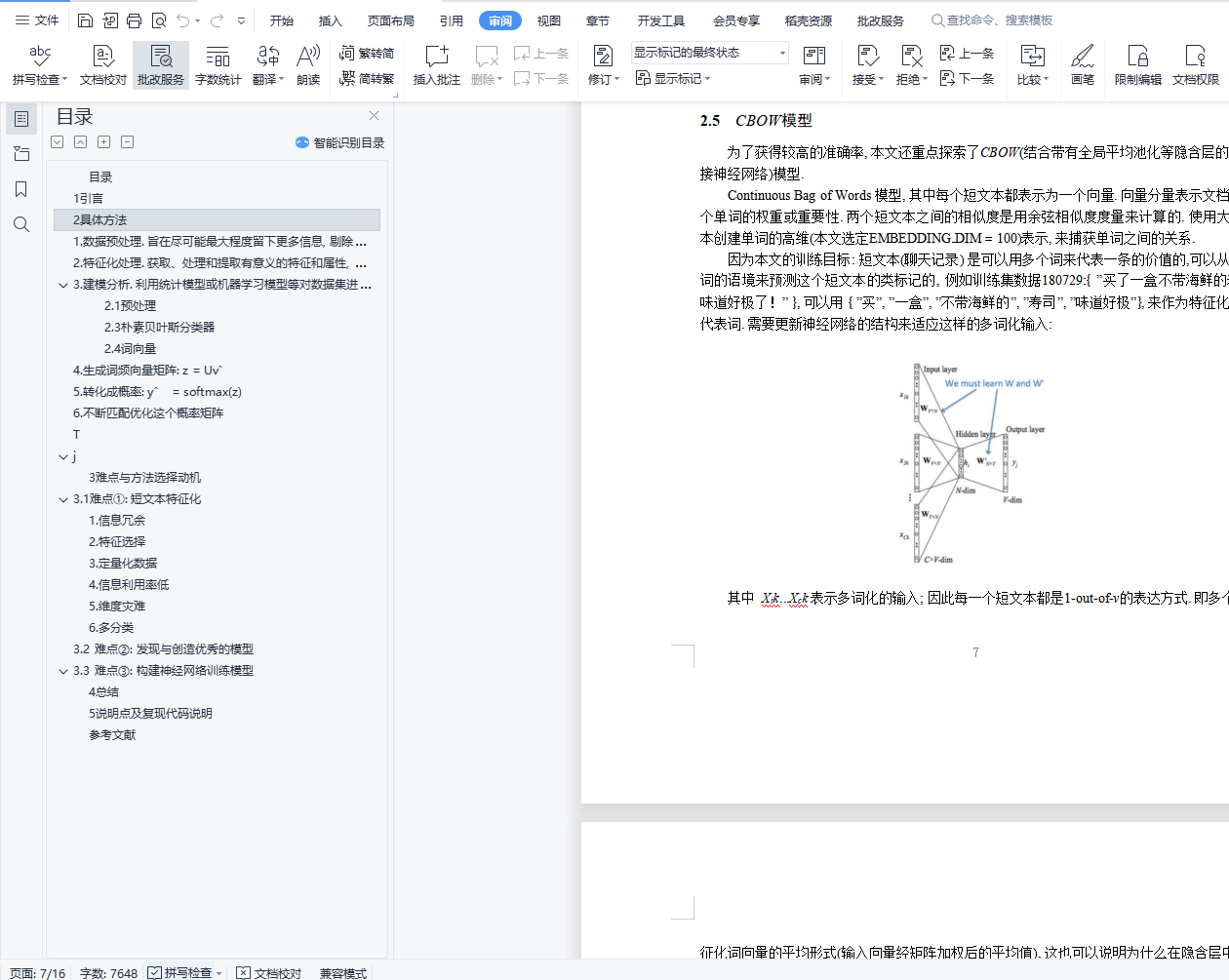
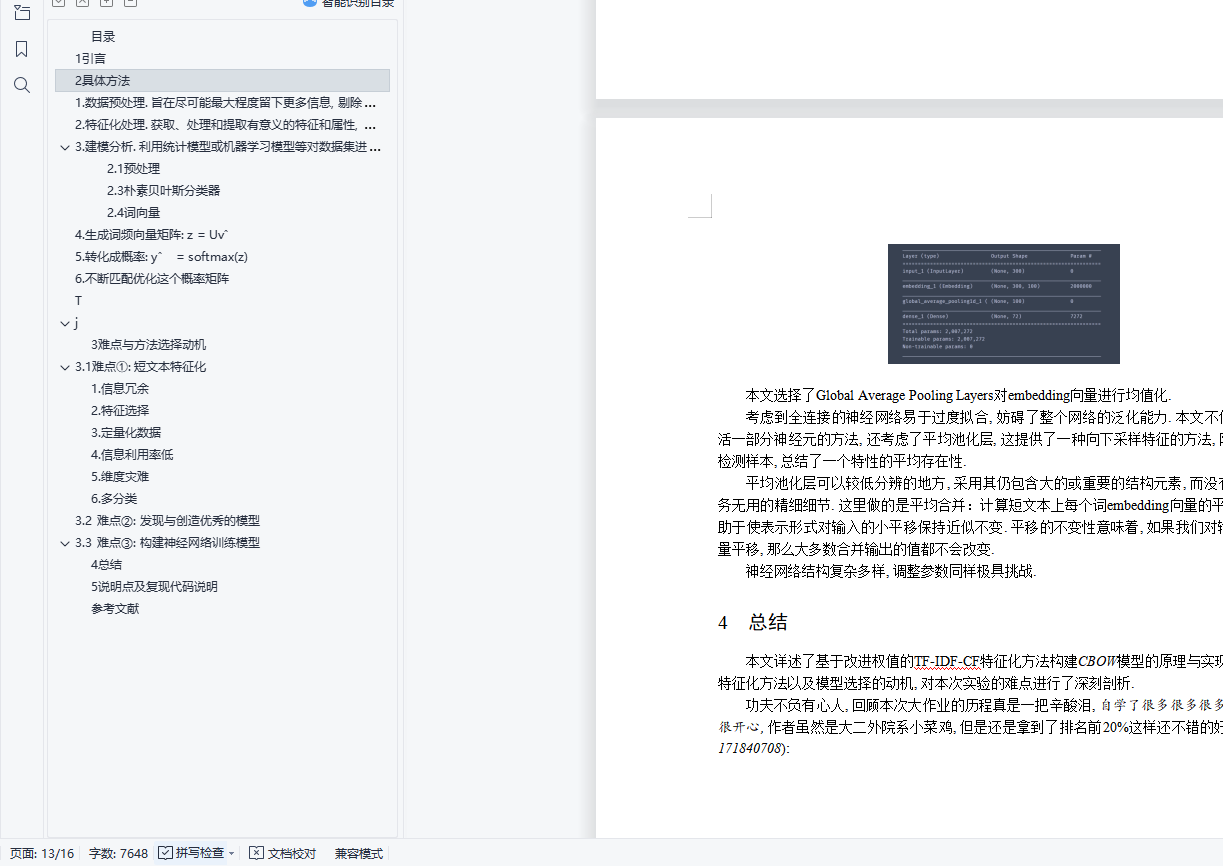
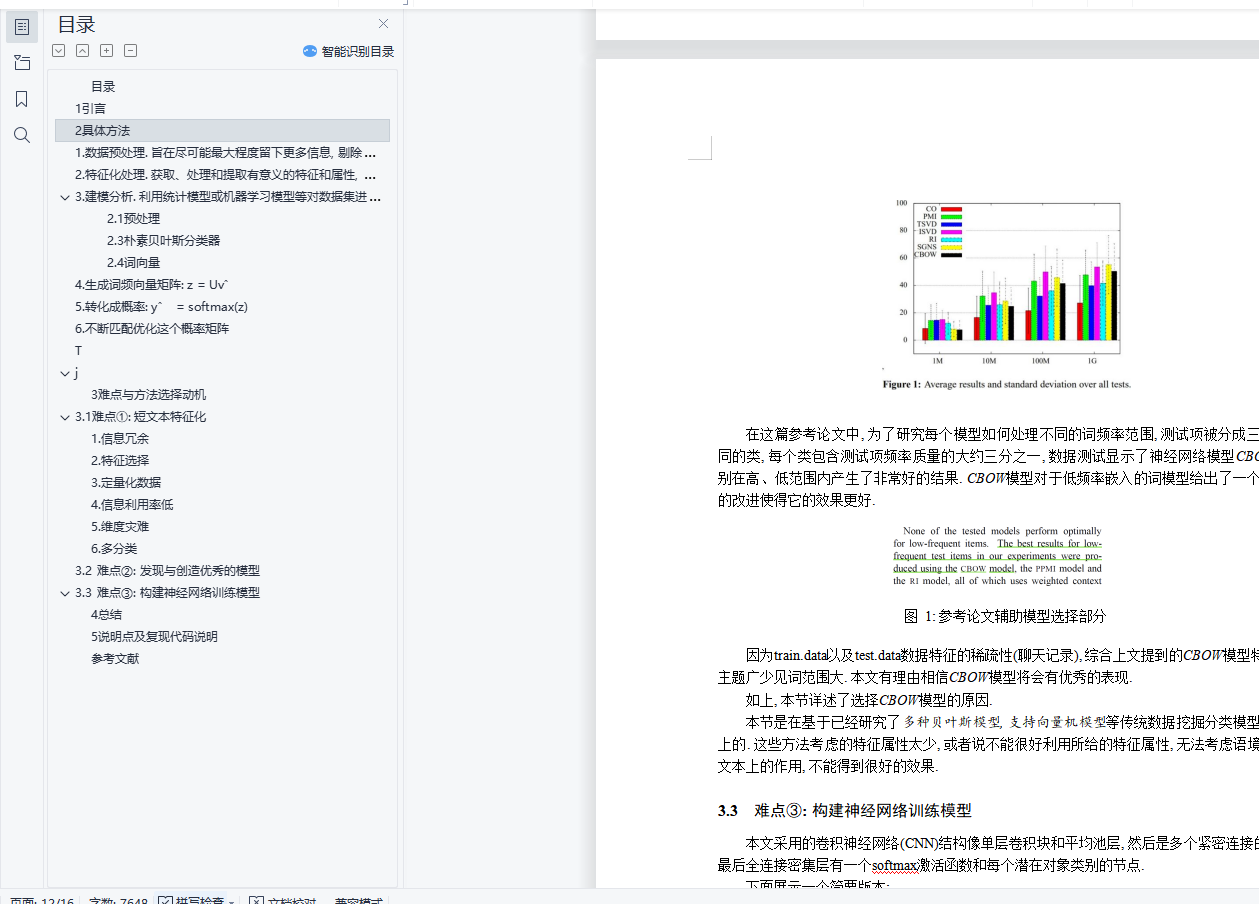
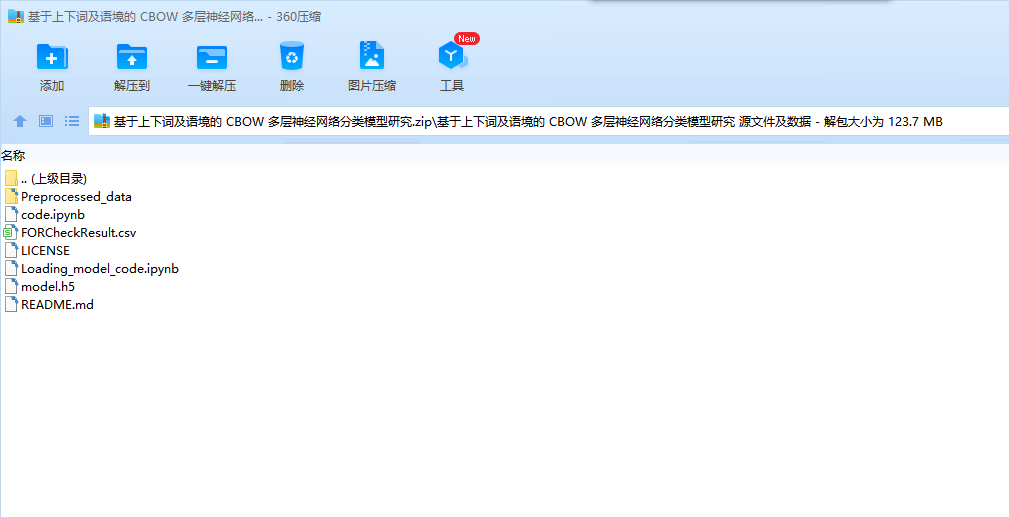
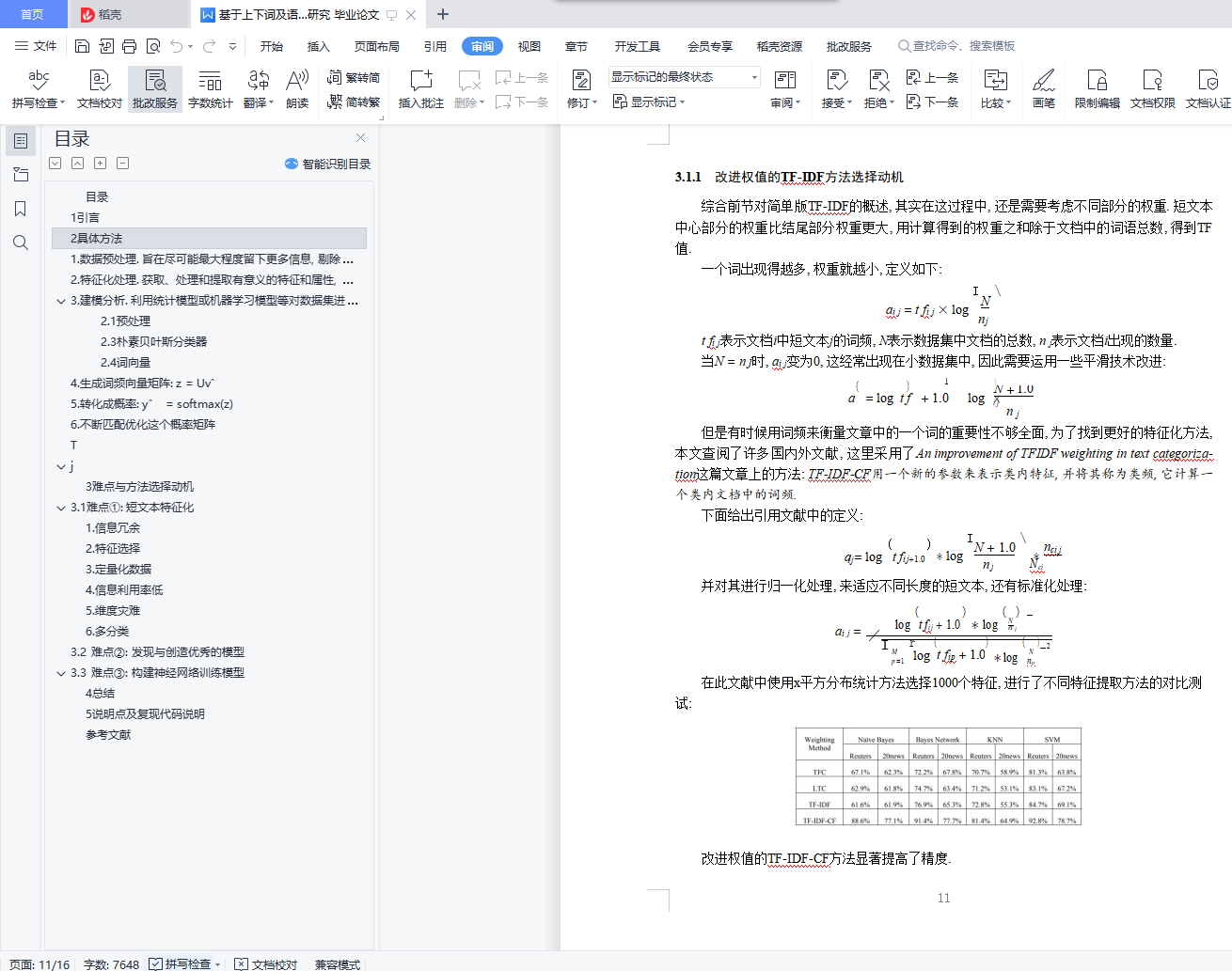
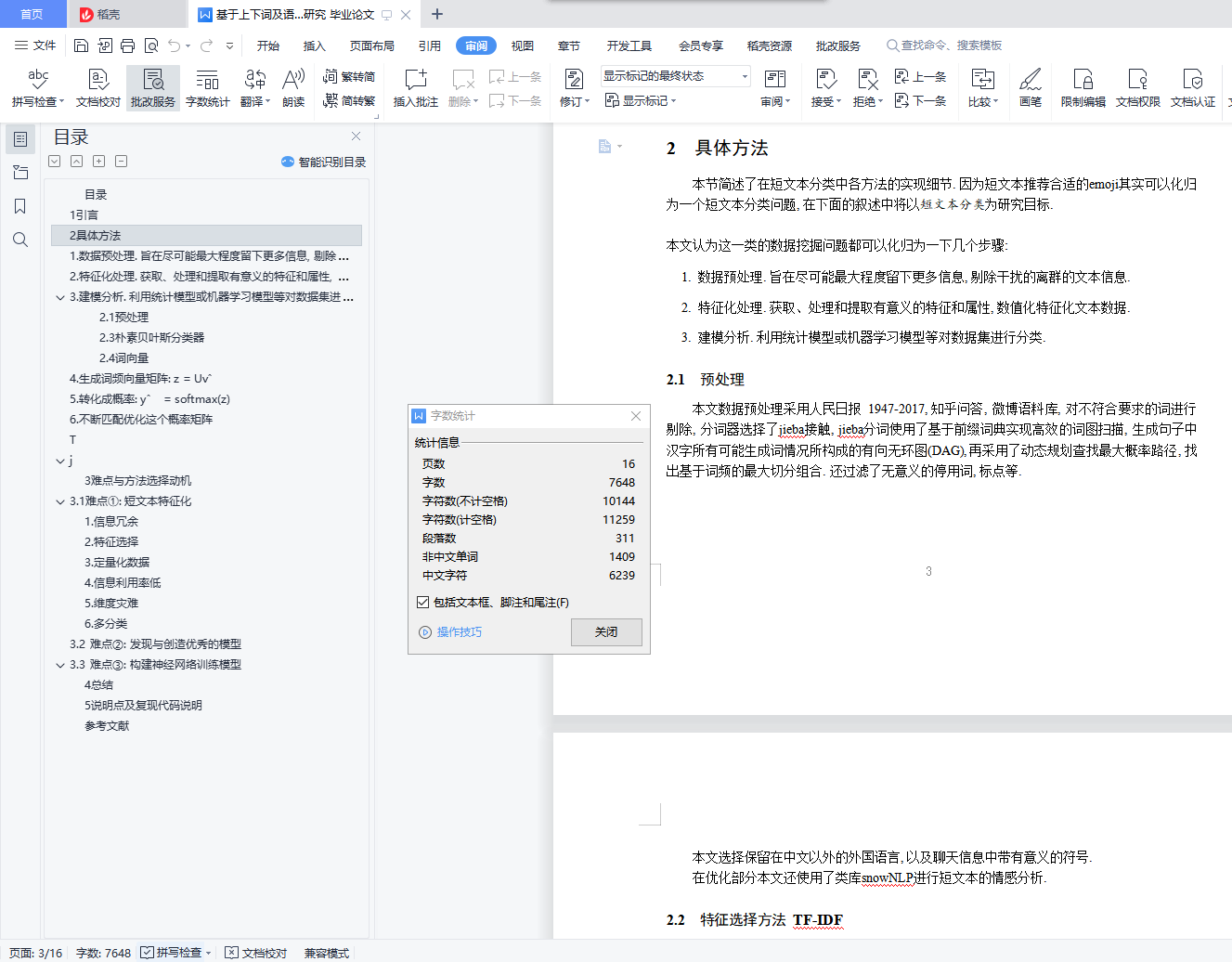
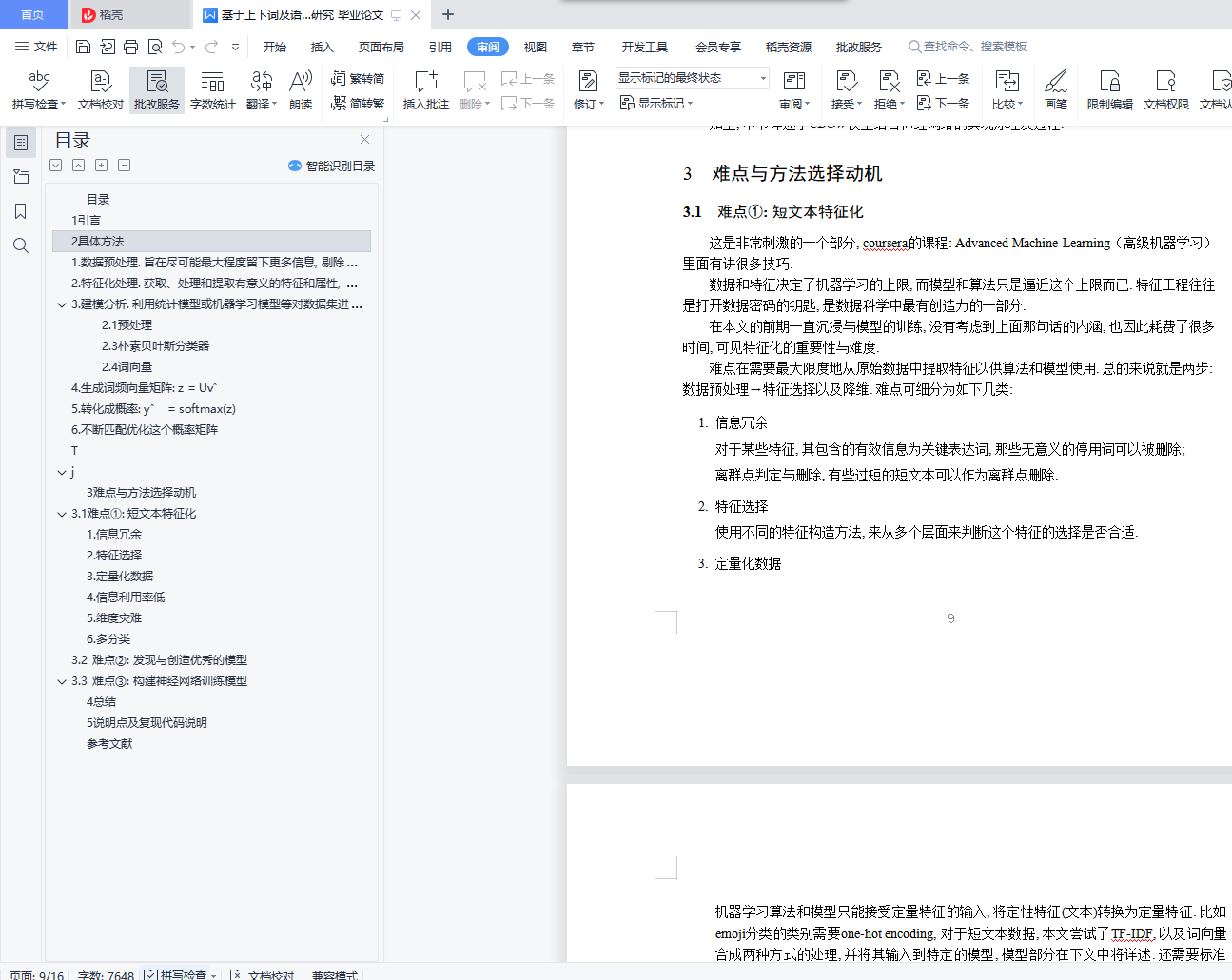