摘要
随着淘宝,京东等电子商务网站的蓬勃发展,消费者越来越多的选择网购.消费者可以在这些电子商务网站上可以获得自己心仪的商品的各种信息.目前淘宝网在电子商务中占有绝对的地位,目前拥有近5亿的注册用户,每天通过电子商务网站上进行购物的消费者有6000万,平均每分钟有4.8万件商品被销售.淘宝是致力于提供基础服务的平台,由其他商家进入进行销售活动.但是对于商家的资格认证没有太多的要求,因此在淘宝里面既有着著名品牌旗舰店,也有普通的商家,从而在淘宝网中如何选择心仪的商品成为了一个非常困难的问题.在消费者的网购调查中可以发现消费者在购物时最注重的是商品的用户评价,因为商品的用户评价可以反映出不同消费者对于商品的真实感观,从而确定商品的综合质量.如何高效的识别出消费者对于所购买的商品的评论情感倾向,对于消费者购买商品有很重要的意义. 本课题主要是通过对商品的评价信息的研究和分析,提出一种新的商品推荐系统,让消费者能够快速选择心仪的商品.本课题主要从以下几个方面进行了分析研究: 针对目前消费者在淘宝网购物的需求和其商品推荐排序不一致的问题进行分析:首先,通过分析消费者的购物心理活动和消费习惯,然后通过淘宝API获取到的商品评价数据进行预处理,计算用户评价信息的情感值;其次,在多属性排序算法的基础上改进,依据各个特征的不同权重比例来进行加权,形成新的商品综合排序算法;最后,构建平台界面展示商品,推荐出消费者心仪的商品. 本课题的研究摒弃了目前淘宝现存在的基于人气,销量,信用,价格等特征的单个因素排序方式和基于这些特征的综合排序方法,避免了由于某些商家通过竞价排名,投机等不良行为获得的排名靠前.同时综合考虑商品的用户评价情感值,销量,商家的信誉等因素,深入分析了各因素之间的关系,创造性地提出了一种基于商品各属性关系的综合商品排序算法.理论分析和实验表明,本课题的研究节省了消费者搜索商品的时间,推荐的商品也更加符合消费者的需求,同时抑制了假冒伪劣产品的销售,在实际应用方面具有优越性.
关键词:淘宝开放平台;用户评价;情感分类;多属性排序
summary
With the booming development of e-commerce websites such as Taobao and Jingdong, more and more consumers choose online shopping.Consumers can access various kinds of items on these e-commerce sites.At present, Taobao occupies an absolute position in e-commerce. At present, it has nearly 500 million registered users. There are 60 million consumers who shop through e-commerce websites every day, and an average of 48,000 goods are sold per minute.Taobao is a platform dedicated to providing basic services, with other businesses enter sales activities.However, there are no many requirements for the qualification certification of merchants. Therefore, there are both famous brand flagship stores and ordinary merchants in Taobao, so how to choose the favorite goods in Taobao has become a very difficult problem.In the online shopping survey of consumers, it can be found that consumers pay the most attention to the user evaluation of goods when shopping, because the user evaluation of goods can reflect the true perception of different consumers on the goods, so as to determine the comprehensive quality of goods.How to effectively identify the emotional tendency of consumers to comment on the goods purchased has a very important significance for consumers to buy goods.This project is mainly to put forward a new commodity recommendation system, through the research and analysis of the commodity evaluation information, so that consumers can quickly choose their favorite products.This topic mainly from the following aspects: to analyze the current consumer shopping demand on Taobao and their product recommendation ranking inconsistent problems: first, by analyzing the consumer shopping psychological activities and consumption habits, then through Taobao API of commodity evaluation data, calculate the user evaluation information sentiment, second, based on the multi-attribute ranking algorithm, weighted according to the different weight ratio of each feature to form a new comprehensive commodity sorting algorithm; finally, build the platform interface to display goods and recommend consumers.The research of this topic abandons the current Taobao ranking method of individual factors based on popularity, sales volume, credit, price and other characteristics and the comprehensive ranking method based on these characteristics, and avoids the top ranking of some merchants through bidding ranking, speculation and other bad behaviors.At the same time, comprehensively considering the commodity user evaluation emotional value, sales volume, merchant reputation and other factors, the relationship between various factors is deeply analyzed, and creatively put forward a comprehensive commodity sorting algorithm based on the relationship of various commodity attributes.Theoretical analysis and experiments show that the research of this topic saves consumers the time to search for goods, and the recommended goods are more in line with the needs of consumers, and suppresses the sales of fake and shoddy products, which is superior in practical application.
Key words: Taobao open platform; user evaluation; emotional classification; multi-attribute sorting
目录
一、什么是推荐系统
4.1 项目目录结构
4.6 二值化数据库处理
五、测试结果
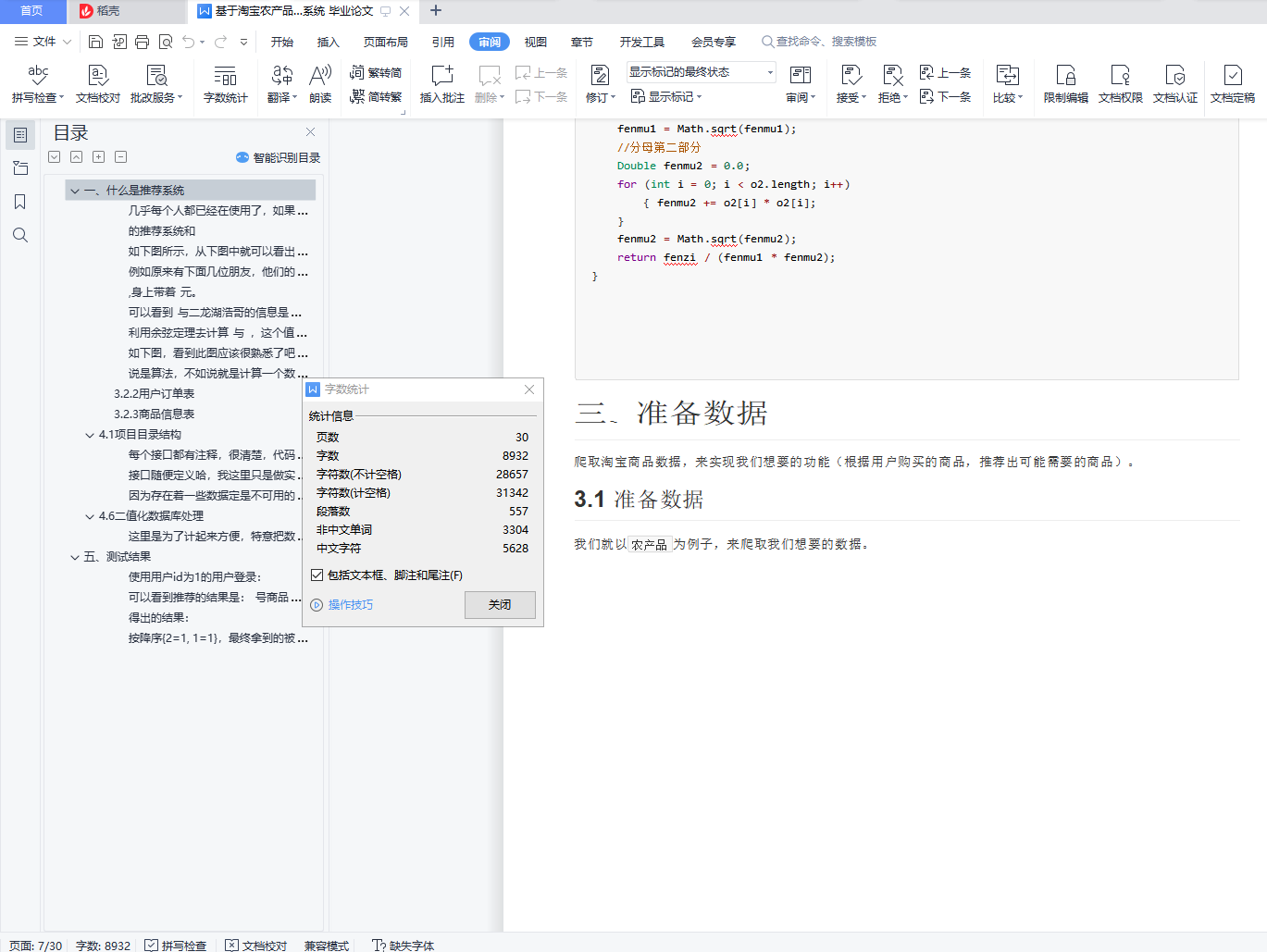
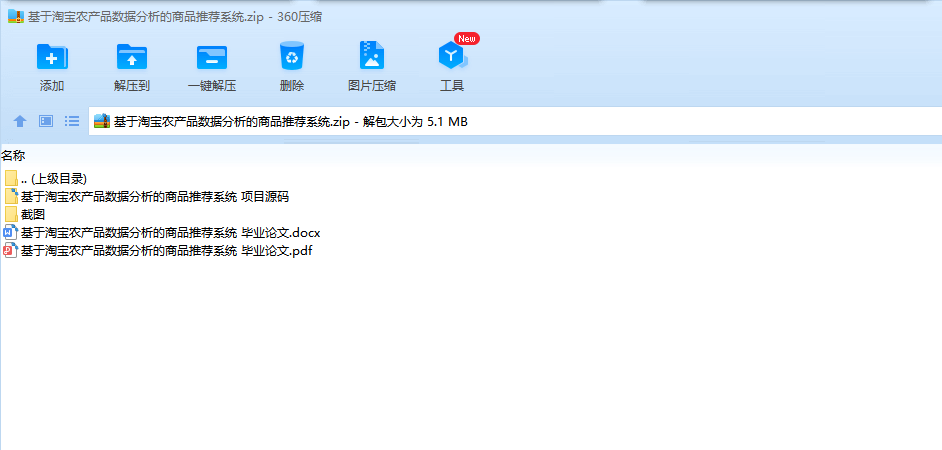

UI7ZAK0{G3(ADU]XNQ{D.png)
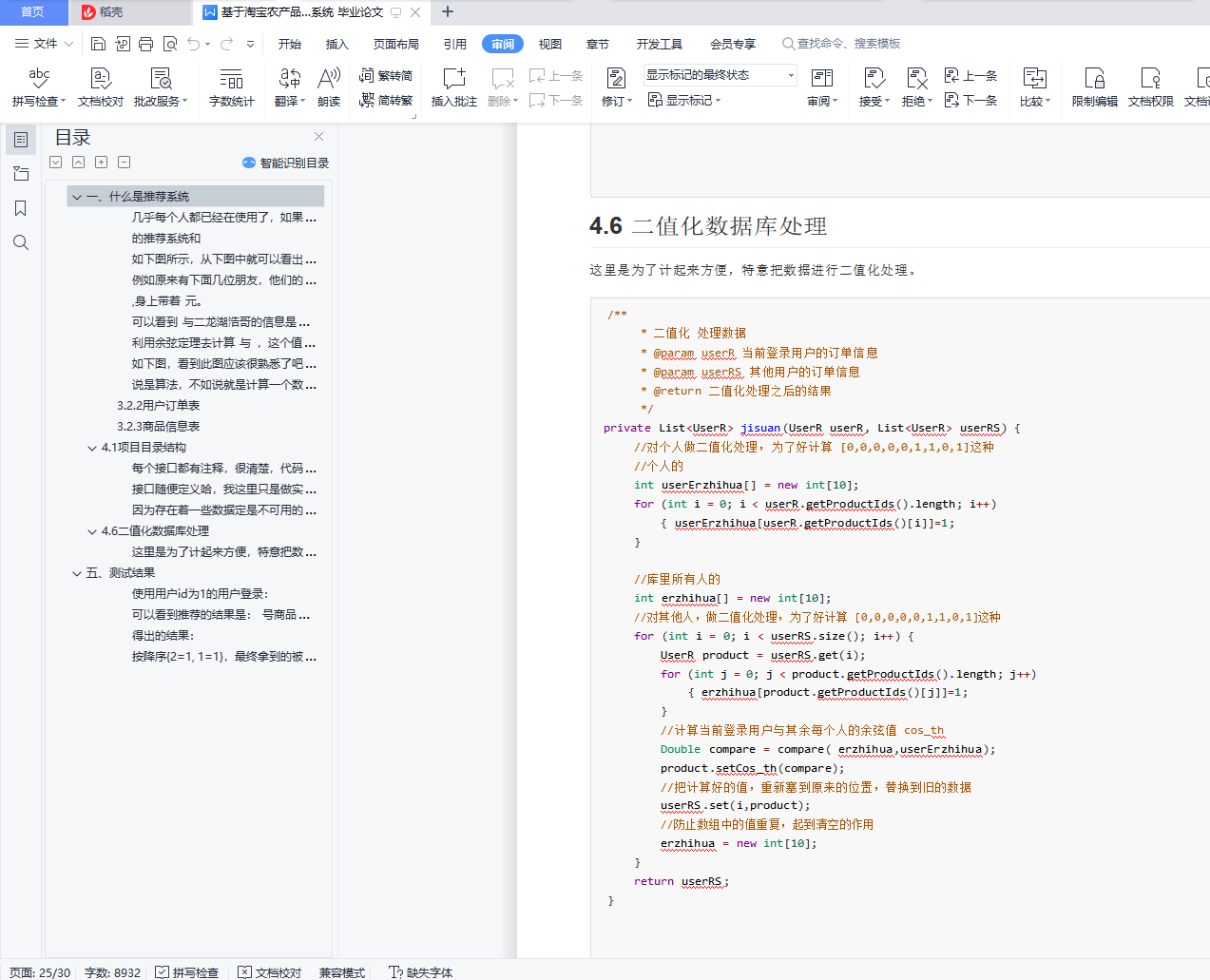
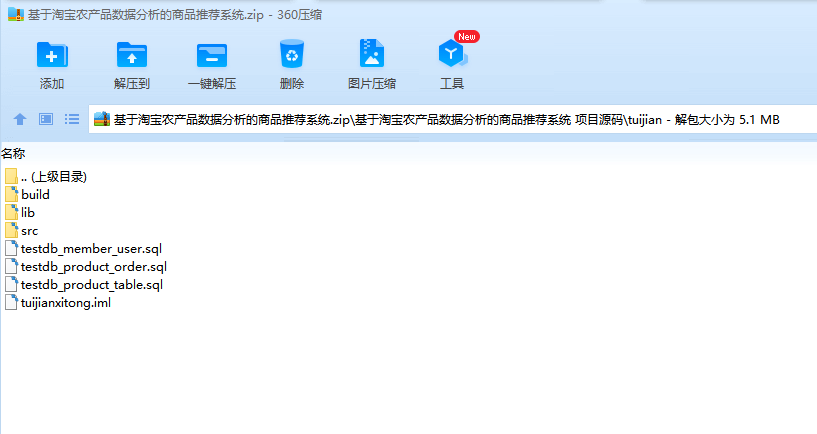
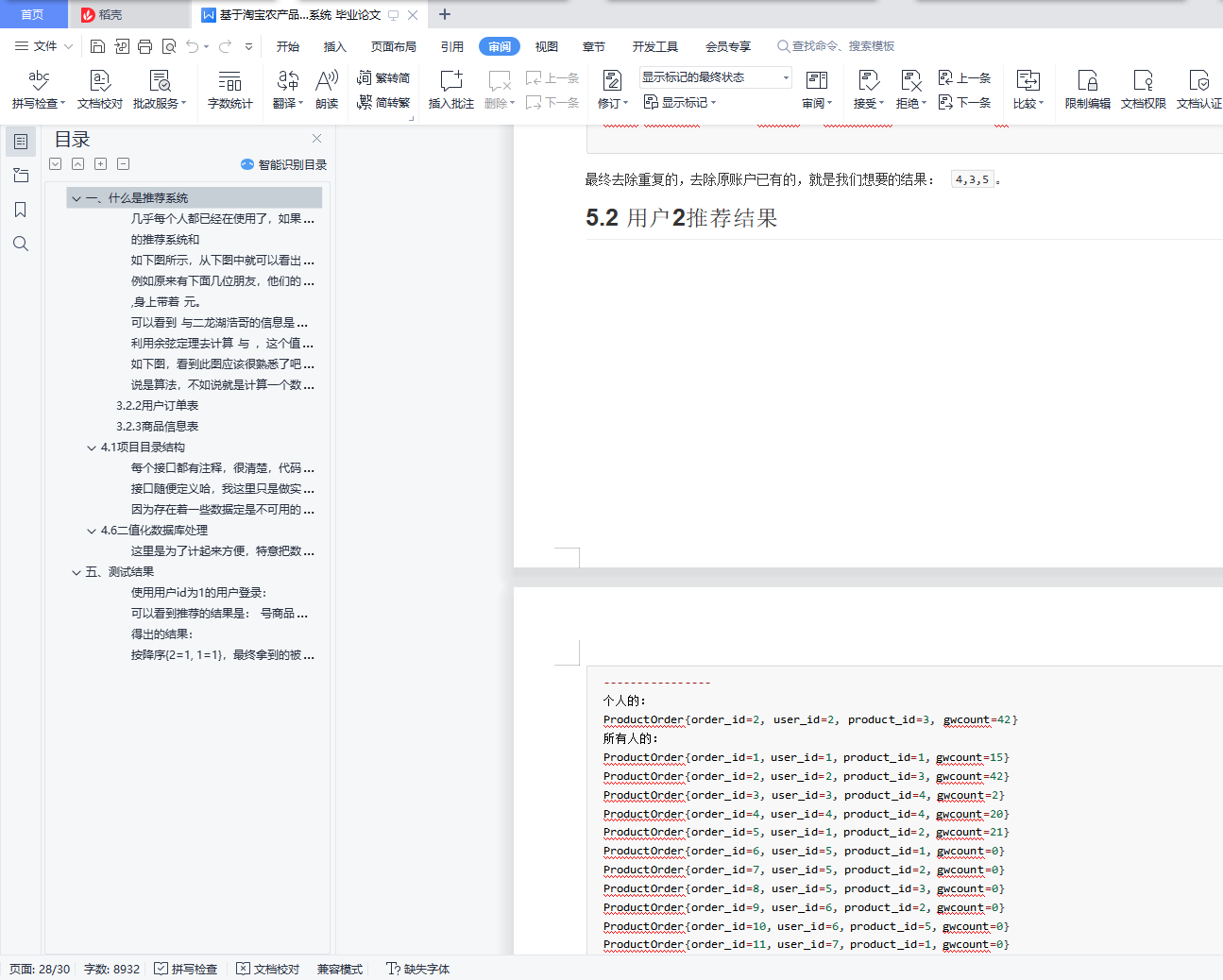



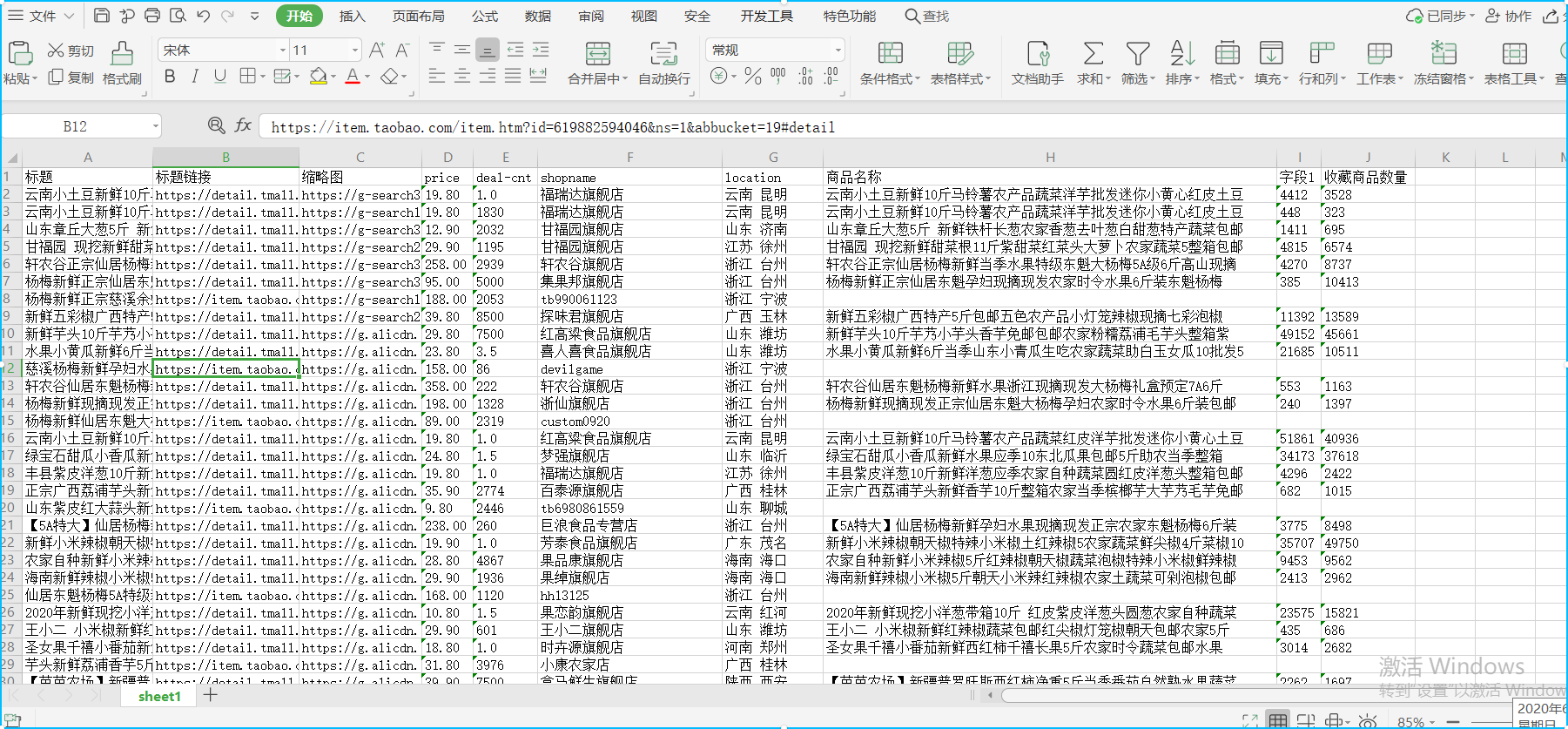
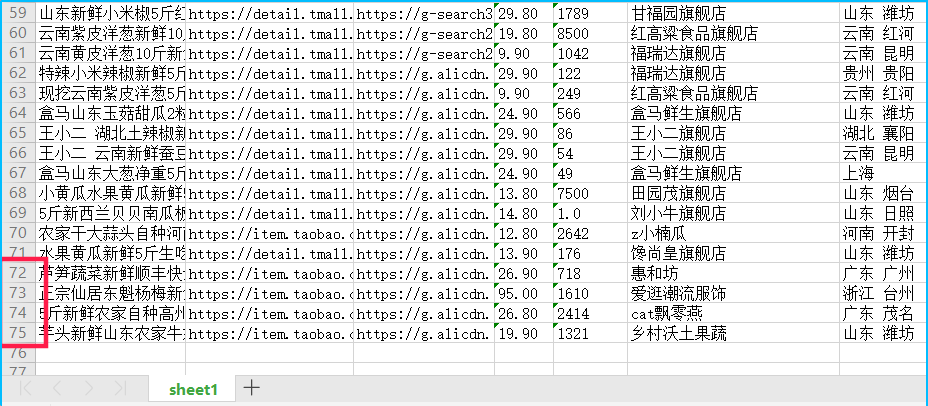
