摘 要
共轭梯度法由于内存需求量小,迭代形式简单,收敛速度快等优点,受到广泛的研究和关注,并被广泛地应用于实际问题的求解。基于已有研究成果,本文提出分别求解无约束优化问题和非线性单调方程组的两种共轭梯度算法,建立算法的全局收敛性,并用数值试验验证算法的有效性。对于非线性方程组问题,本文修正一个新的搜索方向。
在众多的共轭梯度法中,满足充分下降条件的充分下降共轭梯度法往往更为有效。本文重点研究这类方法,并用它们求解一般的非线性无约束优化问题和非线性方程组。从求解一般可微的无约束优化问题出发,讨论了一类充分下降共轭梯度法的统一框架,然后,将该框架下的共轭梯度法加以推广,用于求解带有凸约束的非线性方程组和不可微的无约束凸优化问题。
在适当的假设条件下证明其充分下降性和全局收敛性。通过数值实验中,证明本文所设计的算法NN的数值结果优于算法PRP。对新算法进行数值测试,以验证方法的有效性。通过数值实验,并在与原算法的比较中,证明数值结果更好。
关键词:共轭梯度法,非线性,方程组,最优化,收敛性
Abstract
Conjugate gradient method has been widely studied and paid attention to because of its small memory demand, simple iterative form and fast convergence speed, and has been widely used in solving practical problems. Based on the existing research results, two conjugate gradient algorithms are proposed to solve unconstrained optimization problems and nonlinear monotone equations respectively. The global convergence of the algorithm is established and the effectiveness of the algorithm is verified by numerical experiments. For nonlinear equations, a new search direction is modified.
In many conjugate gradient methods, the sufficient descending conjugate gradient method satisfying the sufficient descent condition is often more effective. This paper focuses on these methods and uses them to solve general nonlinear unconstrained optimization problems and nonlinear equations. from solving the general differentiable unconstrained optimization problem, we discuss a unified framework for a fully descending conjugate gradient method. then, the conjugate gradient method under this framework is extended to solve nonlinear equations with convex constraints and non-differentiable unconstrained convex optimization problems.
Prove its full degradability and global convergence under appropriate assumptions. Through numerical experiments, it is proved that the numerical results NN the proposed algorithm are better than PRP. algorithm The new algorithm is numerically tested to verify the effectiveness of the method. Through numerical experiments, and compared with the original algorithm, it is proved that the numerical results are better.
Keywords: conjugate gradient method, nonlinearity, equations, optimization, convergence
目 录
摘 要
Abstract
1绪论
1.1选题背景及意义
1.2国内外相关研究情况
1.3研究主要内容
2 全局收敛性及条件
2.1 FR方法
2.2 PRP方法和HS方法
2.3 CD方法
2.4 DY方法
3 共轭梯度法求解非线性改进简介
3.1 杂交共轭梯度法
3.2 共轭梯度法簇
3.3 最短余量法
3.4 Beale-Powell重新开始方法
4 Hager-Zhang共轭梯度算法改进
4.1 引言
4.2 共轭梯度算法
4.3 下降条件
4.4 全局收敛性
5 总结与展望
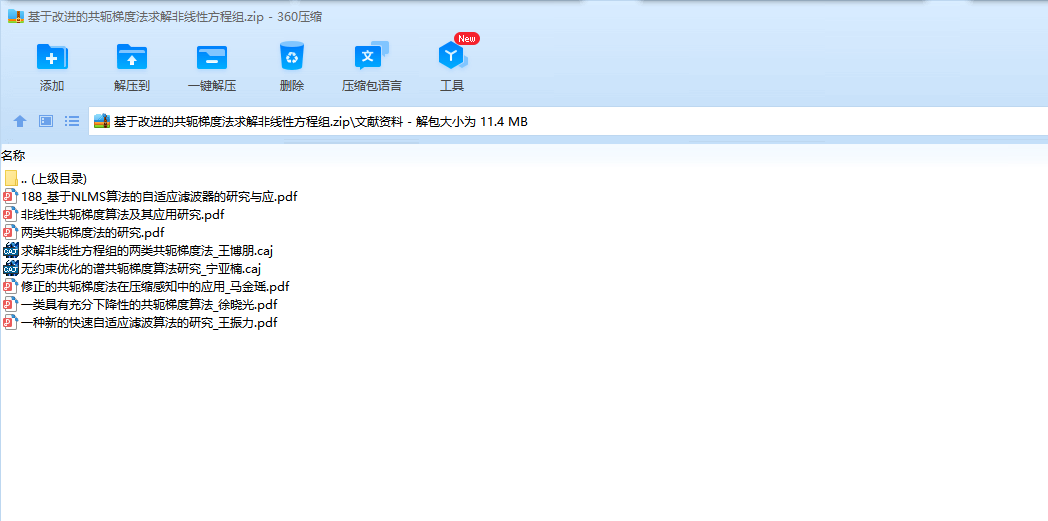
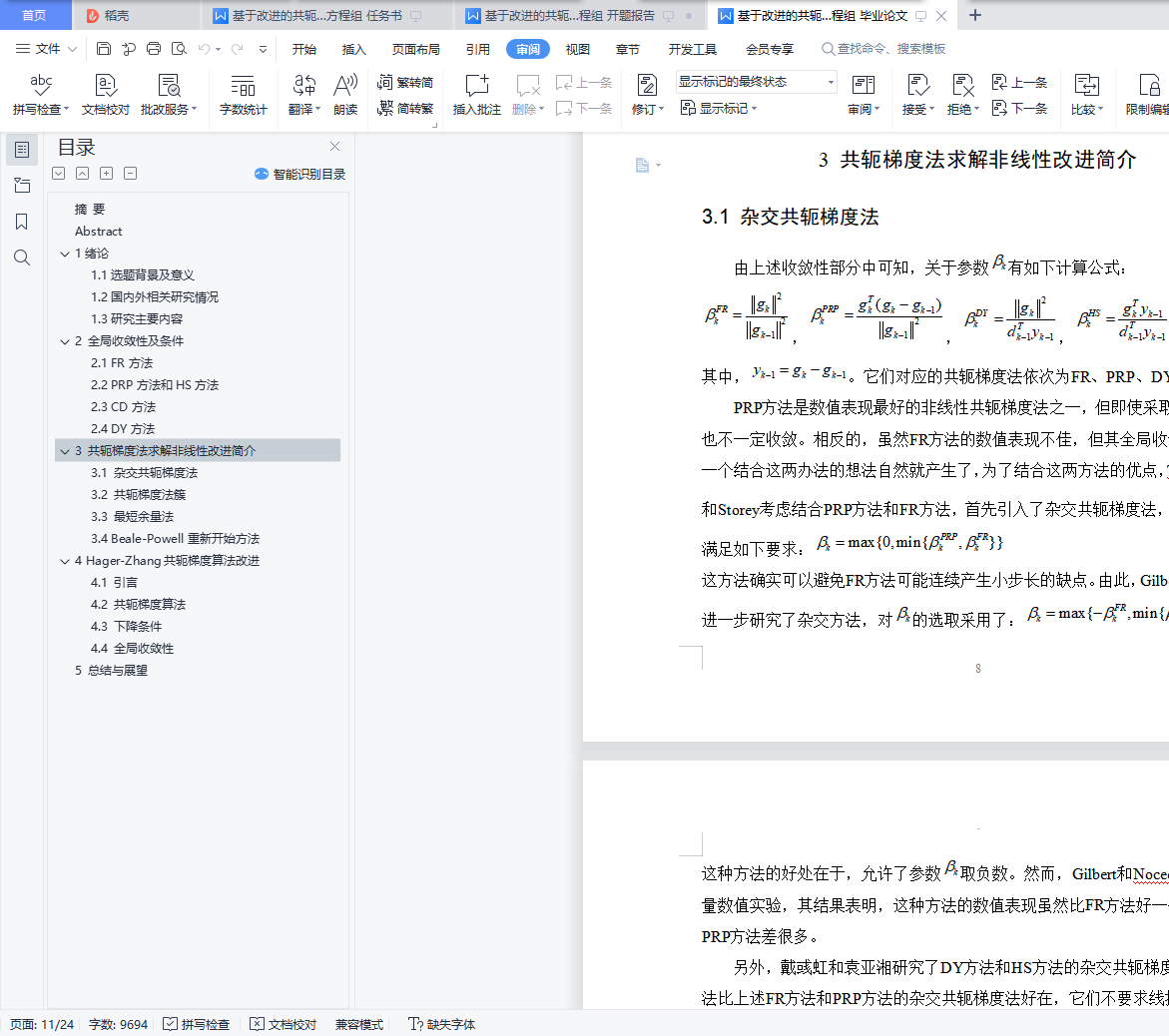
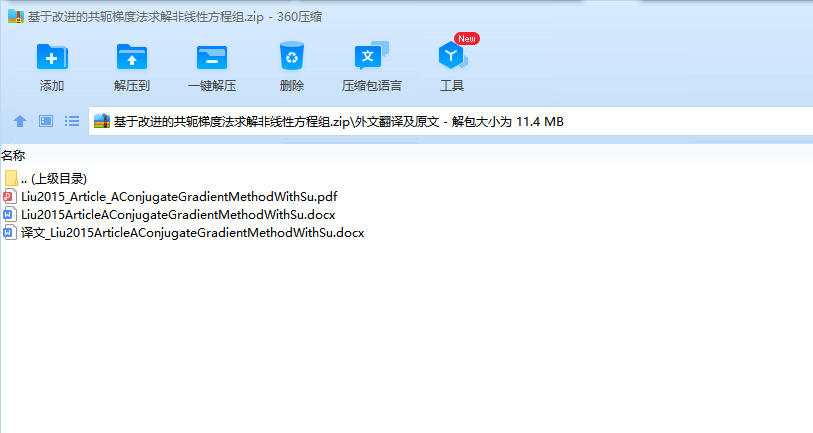
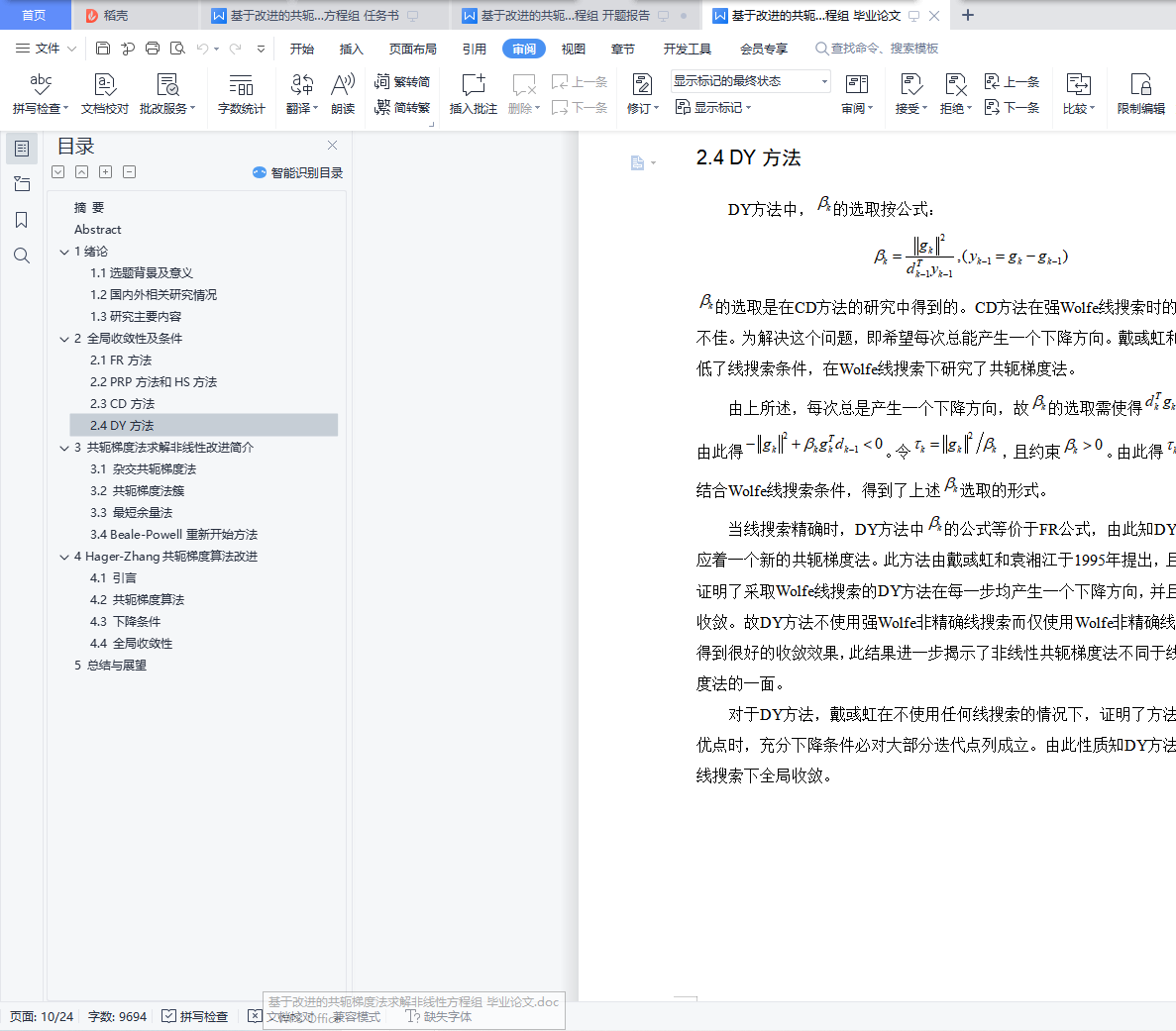
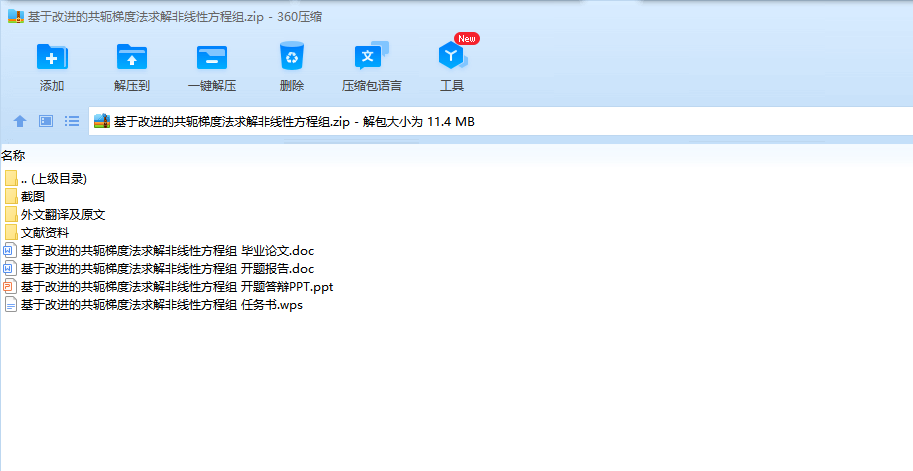
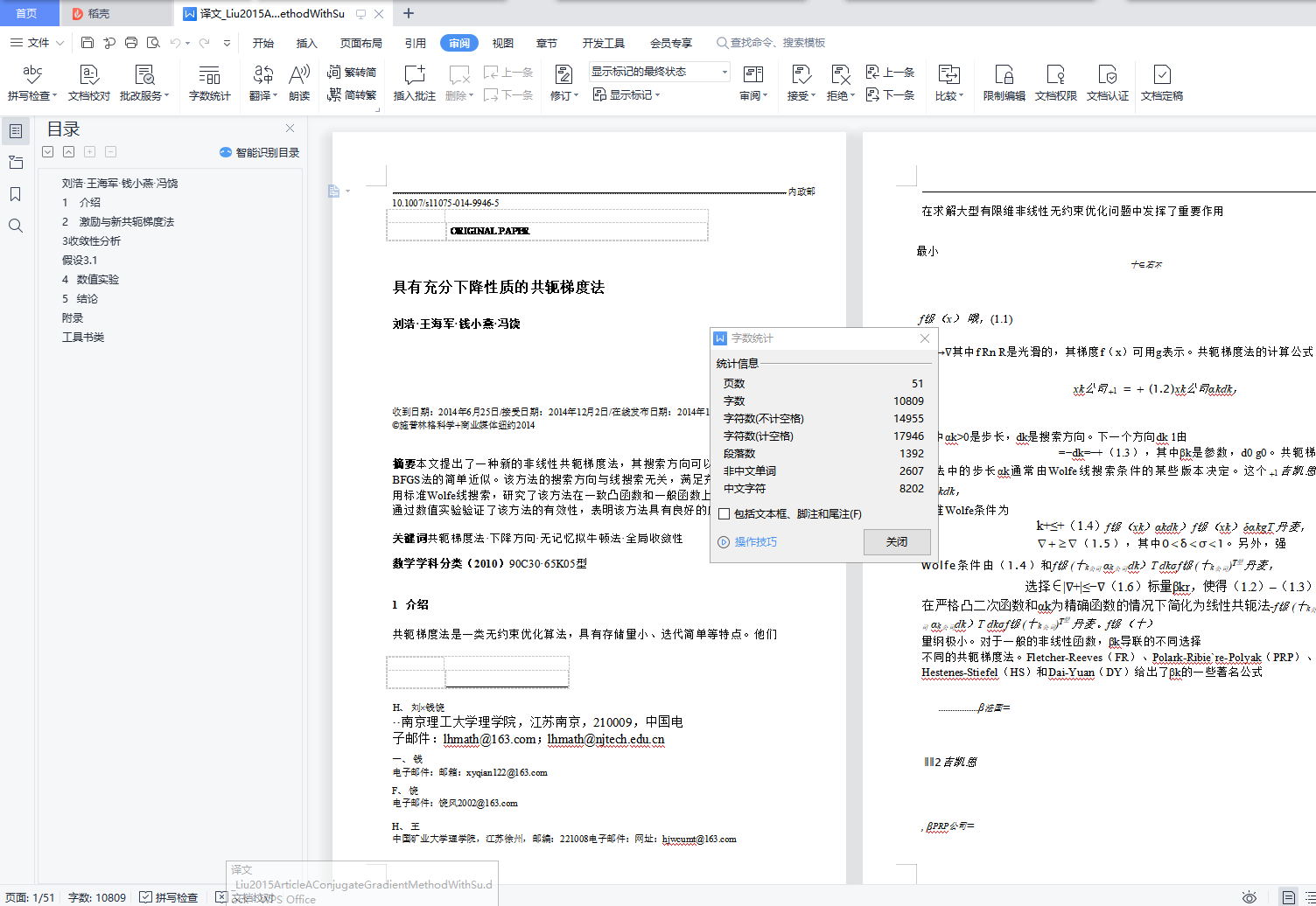
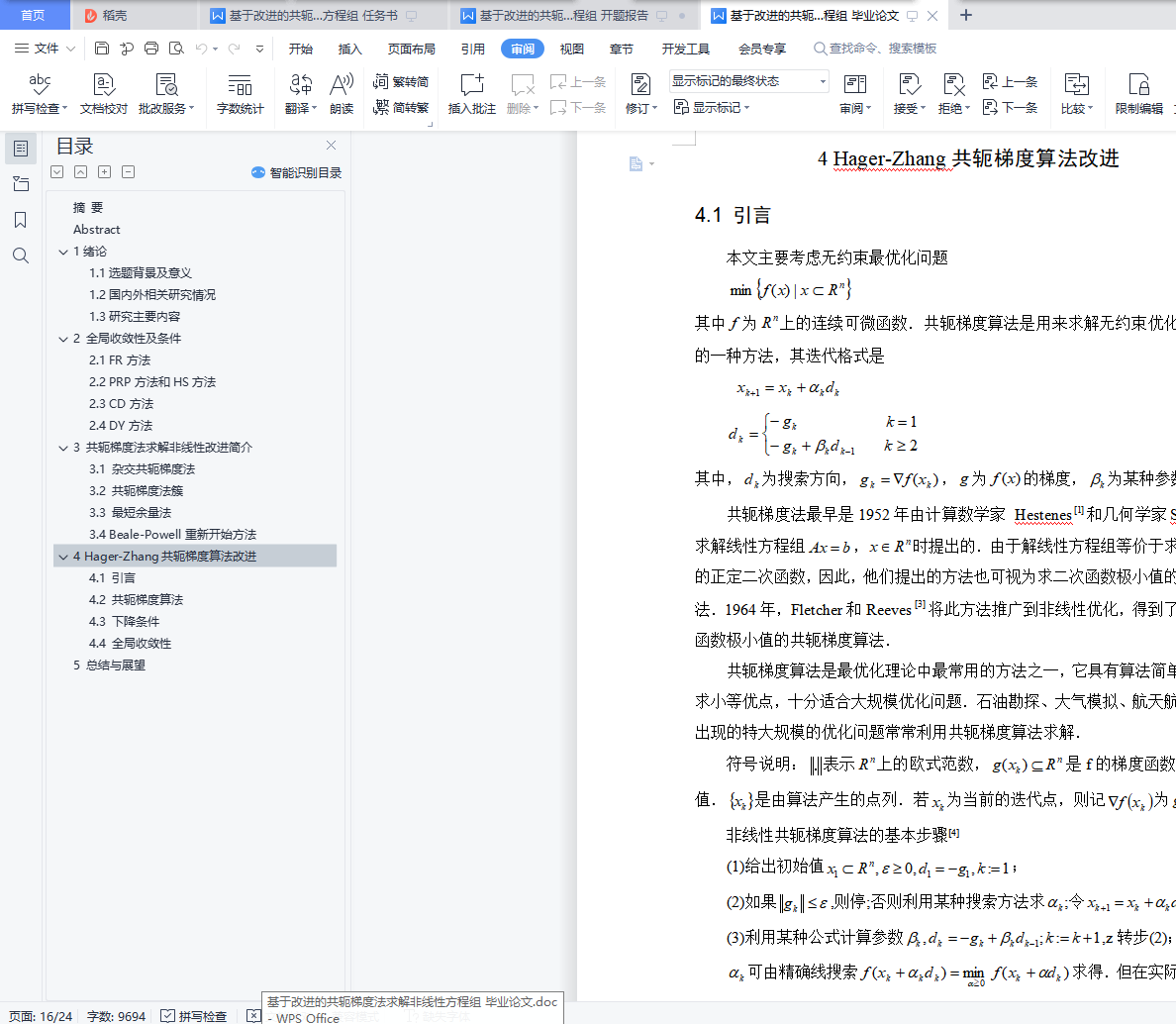
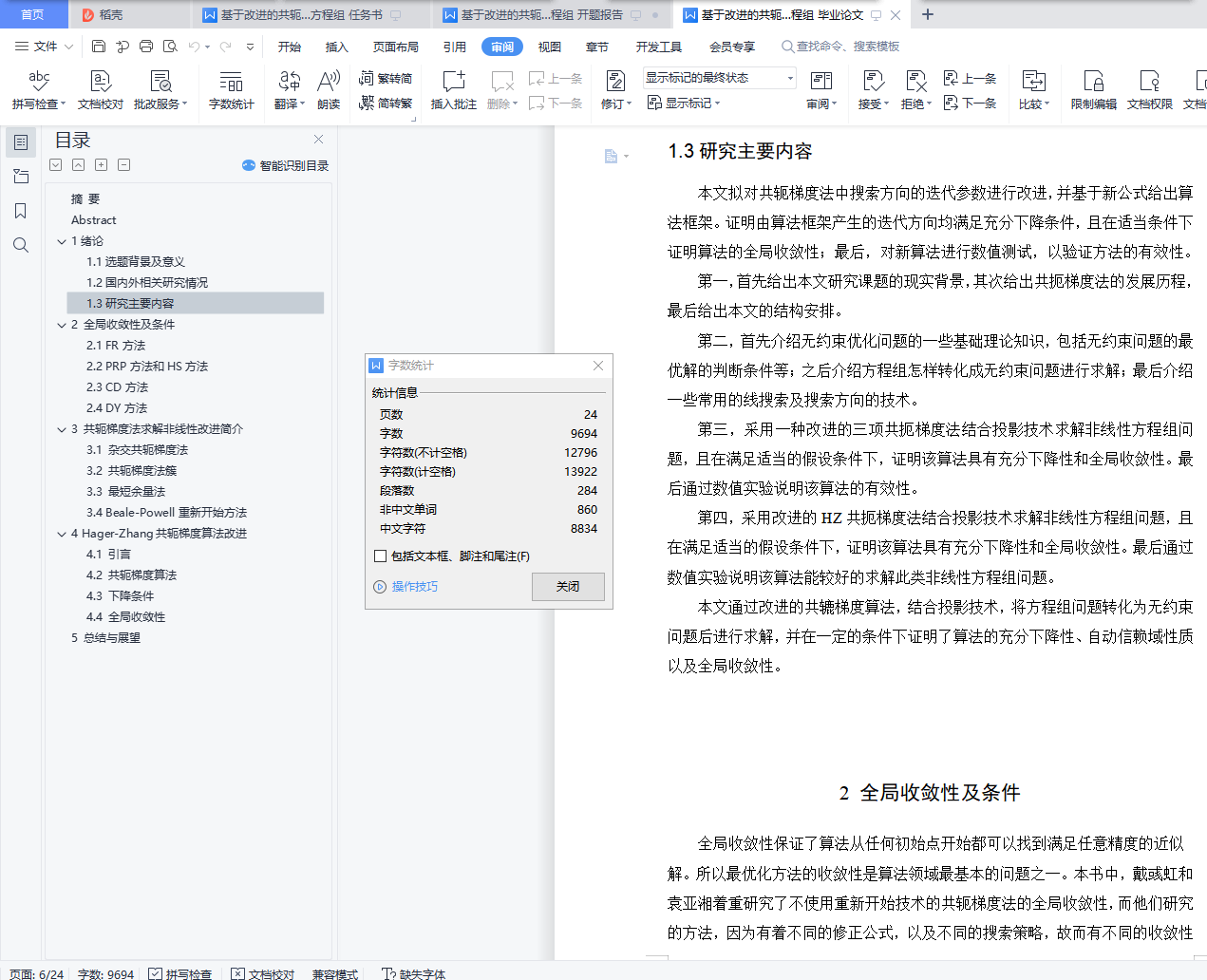
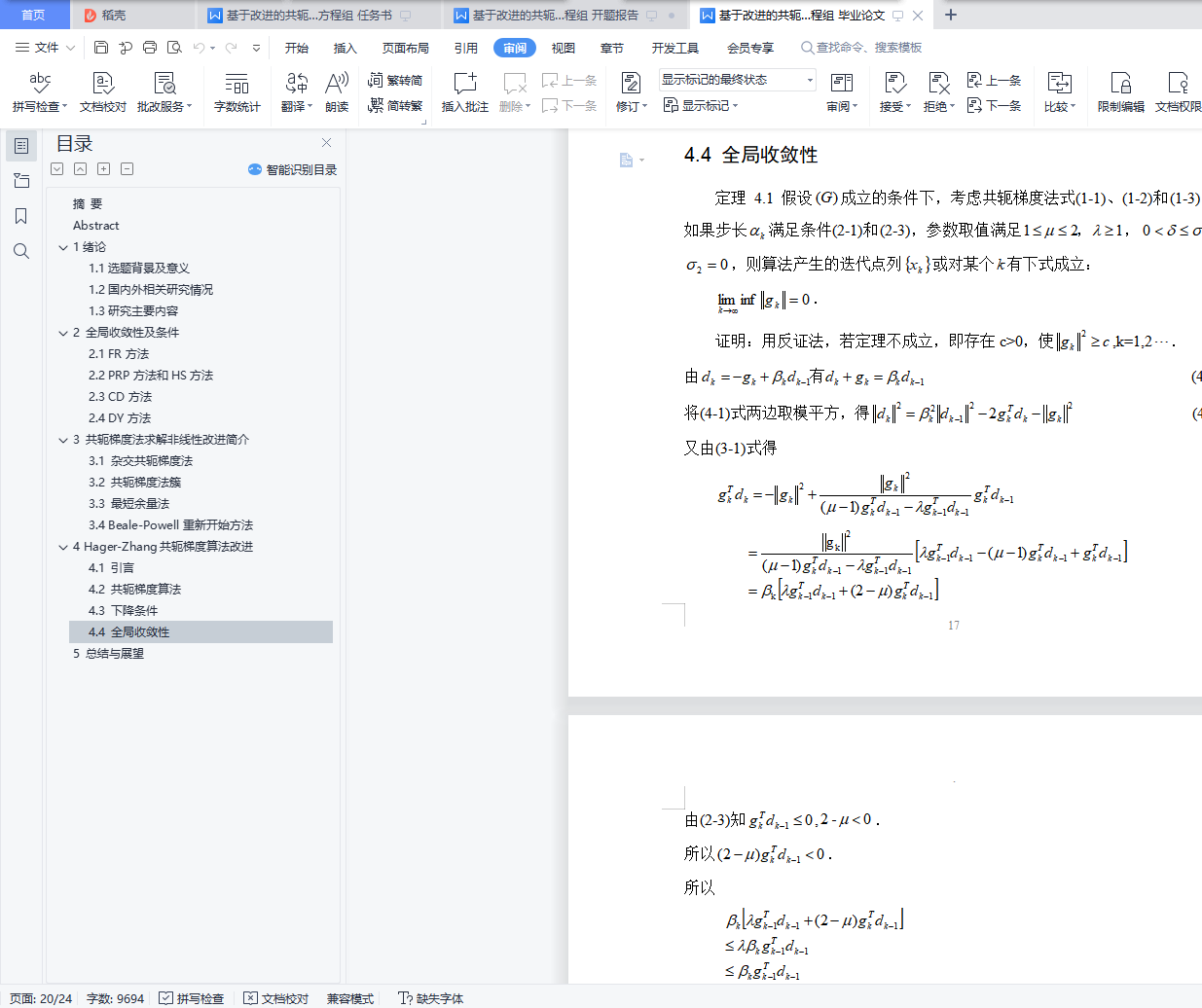
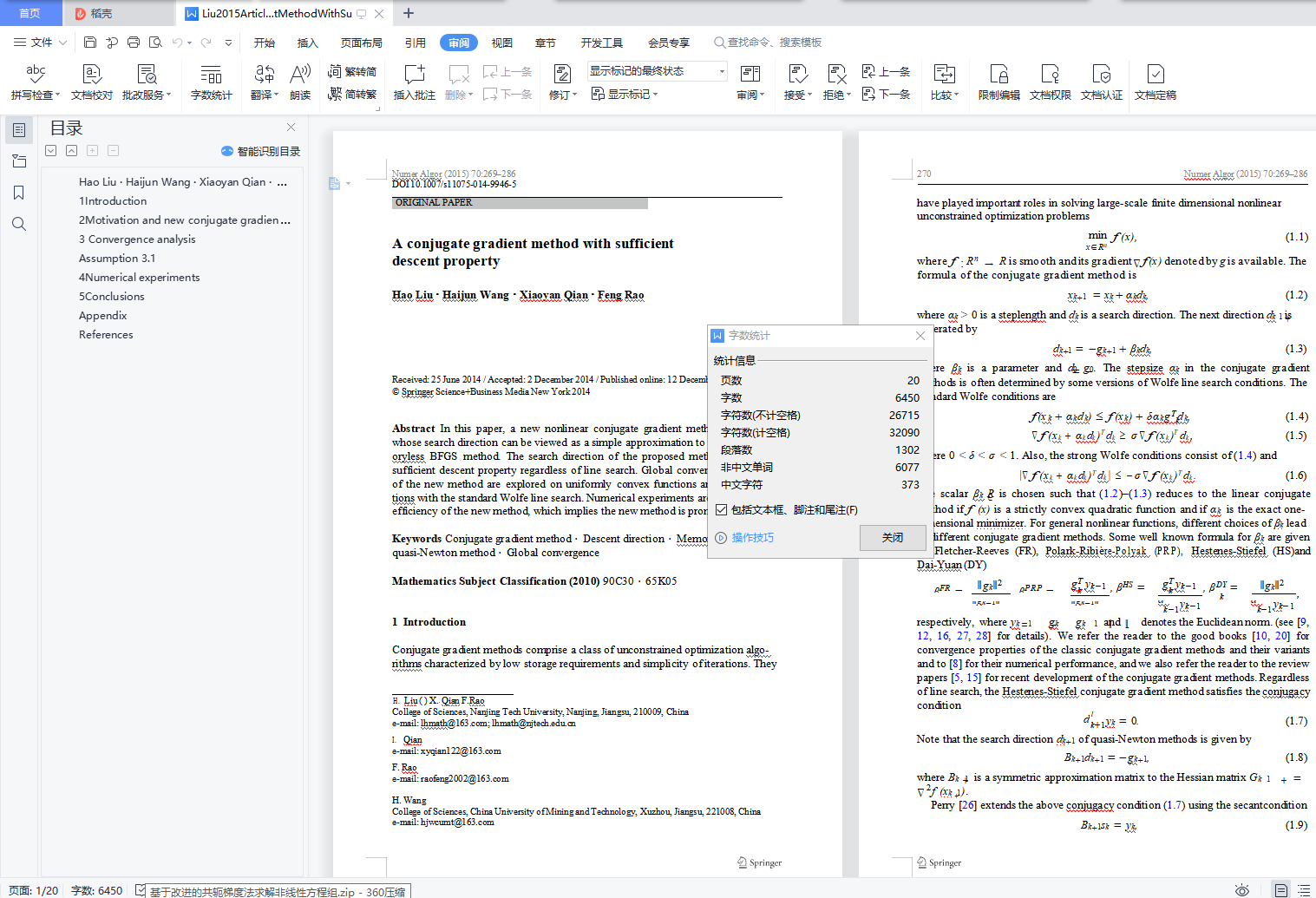
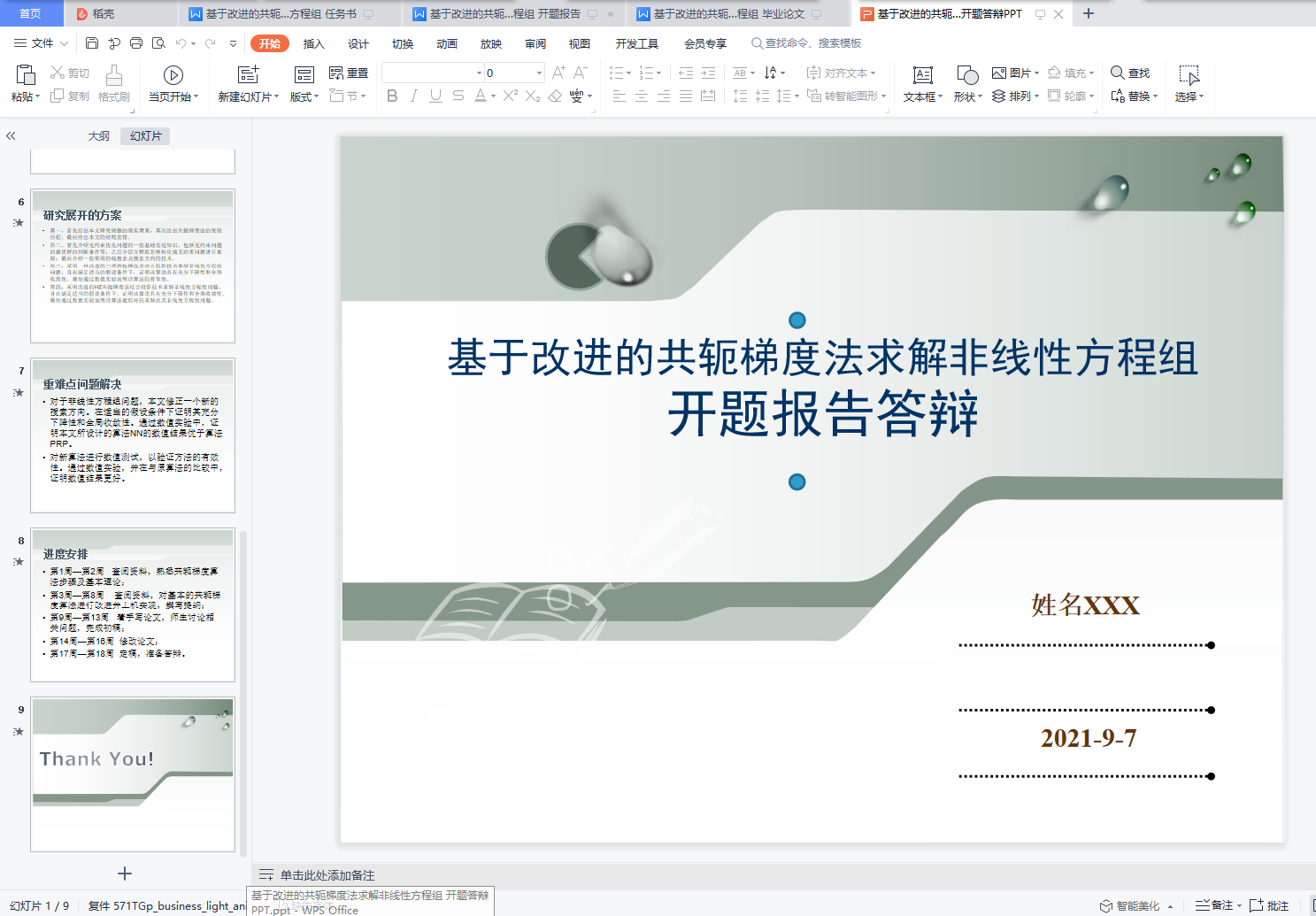