Abstract
With the development of medical and health services, as well as the advance of treatment technology, there are more health demands in our society. The rapid growth of the absolute value of medicare fund expenditures has put lots of pressures on countries’ national health care system. However, the medical insurance fund is the direct key to the health care system. The increases of fund expenditures may cause a relatively shortage of fund and put pressures on the sustainable health care development. To respond to these issues, this paper analyzes the impact of medicare indicators on the medicare fund expenditures, and forecasts the monthly expenditure of medicare fund based on the method of time series forecasting, based on the historical data of medicare fund in the recent 5 years of Dalian, in order to prepare for policy adjustments and early warning. Eventually, it shows that medicare fund balances exist significant mean difference between the group of different medicare indicators. And forecasting error is 6.72%, which meets the actual demand.
With the relationship between the structure of the traditional database technology based on the widely used in various fields of the information age, a variety of complex database system have been established by the computer instead of manual operation, greatly improving the work efficiency of the people. With the relational database and the application of all kinds of information system over a long period of time, in the database system has accumulated a lot of business data, and because of the data service application amount is still increasing day by day. In the society increasingly fierce competition in the market, make the health care industry, the requirements for the database not only stay in the data processing level, and gradually shift to the deep data analysis and utilization direction. But if there is no data powerful analytical tool for mining analysis of existing data in the database, and by the analysis of query function of database and the ability of each business system, is unable to discover the rules and knowledge of these massive data in the. Therefore, in order to meet the growing need for deep utilization of data, data warehouse and data mining technologies emerge as the times require, they can on the data to extract knowledge and experience the deep summary, and obtain the useful information for management decision. At present, the technology is widely used in the financial industry (such as banking, insurance, retail) (supermarket) and the telecommunications industry and other commercial areas, but in the field of health but also to the exploration stage, we made a bold attempt, the researches on this field, in the hospital medical insurance cost analysis using data mining the technology to produce meaningful for Medicare cost management rules.
Key Words: Medicare Fund Expenditures, Medicare Fund Balances, Variance Analysis, Time Series Forecast.
1 INTRODUCTION
With the rapid economic growth in China, the Chinese health insurance has made tremendous achievements. As of 2010, the basic medical insurance system for urban employees has generally established nationwide with 237 million people insured. As of 2011, with the establishment of new rural cooperative medical care, there are 832 million people in the cooperative medical system, which accounts for 97.5% of the whole agricultural population[1,2]. As of 2012, the Chinese basic medical insurance has covered more than 95% of the whole population, which means China has initially built up the world's largest health care network.
With the introduction of some preferential health insurance policies, medicare fund expenditures increases. As of 2010, the general fund expenditures of the basic medical insurance for urban employees has achieved 3271.6 billion yuan, with the increase of 57% compared to 2008. Meanwhile, the general fund expenditures of new rural cooperative medical care has achieved 1187.8 billion yuan, with the increase of 79.4% compared to 2008. However, the growth of total GDP in 2010 is only 21.1% compared to 2008, which means the rate of increase in medical expenses is far more than the growth of GDP. The rapid increase in medical expenses has become an important issue in the field of medical services in China[3,4].
In recent years, the problem of medicare fund balance gradually emerges in various regions, and individual regions make ends meet. Taking the basicmedical insurance fund for urban employees as example, the growth rate of medicare fund stock has been declining year by year since 2005, from 91% down to 10.9% in 2010, and even negative growth happens in some regions[5,6]. It brings a great risk for the medical insurance system which finances based on a certain percentage of total wages. So the forecasting of fund expenditures is an important measure to maintain the medical insurance system in the premise of fund income relatively unchanged.
In order to ensure the smooth operation of medical insurance system, it is necessary to analyze influencing factors of medicare fund stock and expenditure trend, forecast medicare fund expenditures and adjust medical insurance policy. It has attracted the attention of domestic and foreign scholars. In the international, the esearch of medicare fund expenditures has become a hot topic. For example, Newhouse and Gerdtham in 1992, O’Connell in 1996 and Getzen in 2000 analyzed the data from different countries using time-series methods to ensure the trend of total expenditures[7]. Also, George and Karatzas in 2000 used time-series methods based on the medicare fund expenditures data from 1962 to1989 of America, in order to analyzed the impact of medicare indicators[8].
2 CHANGE ANALYSIS OF MEDICARE FUND BALANCE VARYING WITH MEDIC ARE INDICATOR
Medicare indicator, also known as average payment standard, is the expense that medicare fund management institution pays for treating one patient to medicalinstitutions. The exact amount is different due to different regions and years, but gradually increases in general. As shown in the Table. 1 and Fig. 1, it shows the change of medicare fund Balance varying with medicare indicator from 2008 to 2010.
After analyzing the difference of medicare fund balance varying with medicare indicator based on the method of variance analysis via SPSS[9], it shows that medicare fund balances exist significant mean difference between the group of 4760 and 5610, and the fund balance of relatively lower medicare indicator is relatively higher. It also happens between the group of4760 and 6060.
In fact, the adjustment of medicare indicator is increasing the payment standard of medical institutions under the premise of medicare fund having slight surplus. It aims to reduce the operating pressure of medical institutions. But in practice, medical institutions enhance the diagnostic criteria, which aims at increasing medical income. Meanwhile, medical institutions expand the operation area, increase open beds and patients admitted, stimulated by benefits. All these acts will lead to the increase of medicare fund expenditures.
Table. 1. Data of Medicare Fund Balance Varying with Medicare Indicator from 2008 to 2010
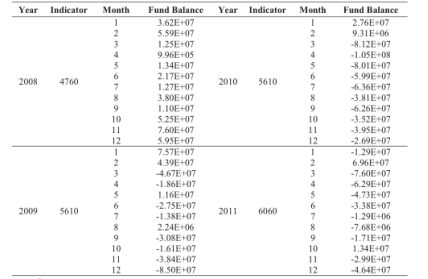
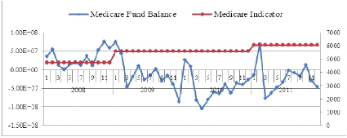
Fig. 1. Trend of Medicare Fund Balance Varying with Medicare Indicator from 2008 to 2011
3 TIME SERIES FORECASTING OF MEDICARE FUND EXPENDITURES
With the improvement of medical technique, thechange of people’s awareness of social medicare and the influence of favourable policies, social medicare fund expenditure increases. The revenues and expenditures ofmedicare fund is imbalance. Taking Dalian as example,as shown in the Fig. 2, medicare fund balance declined significantly in recent years(Assuming medicare fund balance is zero before 2008). So it is necessary to forecasts medicare fund expenditures for early warning based on the historical data.
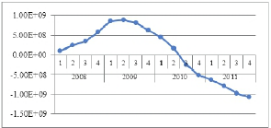
Fig.2. Trend of Medicare Fund Balance from 2008 to 2011
Time series forecasting and causality forecasting both are widely used to describe the laws of change about variables varying with time. The difference is that time series forecasting bases on lots of historical data of target variable varying with time [10] , and causality forecasting bases on the variables that has causal relationship with target variable.
Due to only medicare indicator can be quantified in the influence factors of medicare fund expenditures, it means there are only 5 values of medicare indicator for the past five years. The data size is too small to forecast accurately based on causality forecasting. Also, there is a certain trend in the change of medicare fund expenditures over time. So time series forecasting is selected to forecast medicare fund expenditures.
The forecasting starts with establishing a data stream using time series node via Clementine. The next is to set the node by selecting the predictive model [11] . As shown in the Fig. 3, it shows the data stream.
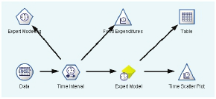
Fig.3. The Data Stream of Time Series Forecasting Model via Clementine
Table 2. Fitting Data of Monthly Medicare Fund Expenditures
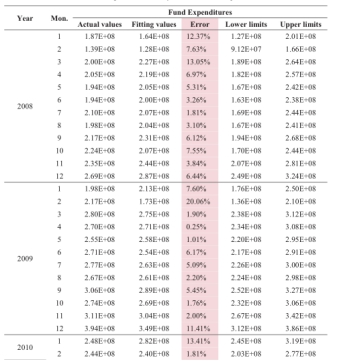
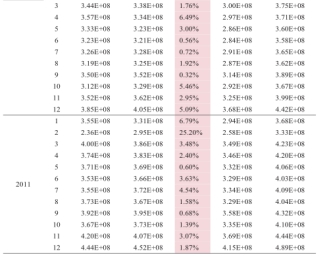
Table 3 Forecasting Data of Monthly Medicare Fund Expenditures
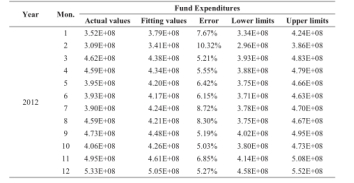
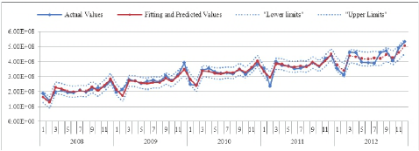
Fig. 4 Fitting and Predicted Curve of Monthly Medicare Fund Expenditures
As shown above, Table 2 shows the fitting values. It provides sample data to establish the model and fitting results, that monthly medicare fund expenditures from 2008 to 2011. Column 1 and 2 represent the time period,from 2008 to 2011 . Column 3 provides actual values of monthly medicare fund expenditures. These three columns provide the sample data. Then the fitting results are shown in the Column 4 to 7. Column 4 provides fitting values of monthly medicare fund expenditures.Column 5 provides the relative error of fitting resultsbased on Column 3 and 4. Column 6 and 7 provides the confidence interval of fitting values. And the confidence level is 95%, which means the probability that the fitting value occurs in this interval is 95%. Table 3 shows the predictive values. It provides inspection data to text the model and forecasting results. And the meaning of columns is similar to Table 2. Fig. 4 shows the fitting and predicted curve.
In fitting results, the goodness of fit is 0.772. And the randomness test of residuals sequence shows there is no structure unexplainable in the sequence of observations, which means the established model is ideal. Besides, the average fitting error is 5.05% and the variance of these errors is 0.25%, which means the model provides a better fit with small fluctuation and can be used to forecast as shown in the Table 3.
From forecasting results listed in Table 3, the forecasting error is 6.72%, which means the forecasting accuracy meets the actual demand.
As shown in the Fig. 4, the fitting and forecasting effect can be observed visually.
From the analysis above, it is reasonable and effective to apply time series forecasting to forecast medicare fund expenditures. And it is necessary.
4 CONCLUSION
Through the research found that the data mining tool is applied to medical insurance settlement work, will not only help management conveniently and flexibly quota standard, but also can accelerate the speed of analysis and feedback; the most important is that it can help to find out the possible causes problems when problems arise. With such an analysis tool, not only can greatly improve the efficiency and accuracy of medical insurance, but also provides an effective means for the management of medical insurance and Medicare data, for further in-depth analysis provides a useful attempt, provides the analysis of flexible management department.
In conclusion, the adjustment of medicare indicator produces an effect on medicare fund expenditures with certain amplification, and fund balance of relatively lower medicare indicator is relatively higher. Such favorable policies can more or less stimulate the growth of medicare fund expenditures. Since there is large amount of fund balances in previous years, medicare agencies paid little attention to medicare fund balances. In recent years, the annual deficit of fund happens and fund stock decreases. It has affected the sustainable development of medical insurance. So forecasting the medicare fund contributes to early warning and policy adjustments.
REFERENCES
[1]Xu W B.Flourishing Health Work in China [J]. Social Science and Medicine,1995,41(8):1043-1045.
[2] 2012 China Health Statistical Yearbook.
[3] Tu Tingyong. Development Research of Chinese MedicalInsurance System[D]. Dalian: Dongbei University of Finance and Economics, 2012.
[4] Wang K M. Health care expenditure and economic growth: Quantile panel-type analysis[J]. Economic Modelling, 2011,28(4): 1536-1549.
[5] Zheng Xiaoyu. Brief Analysis of Causes and Countermeasuresabout Medicare fund loss[J]. China Collective Economy.2011(2):126-127.
[6] Shen Shuguang, Wu yubin. The Critical Issues of theIntegration of Urban and Rural Medical Insurance in China[J]. China Health Insurance, 2013(6): 33-35.
[7] Meijer C, O’Donnell O and Koopmanschap M. Health expenditure growth: Looking beyond the average through decomposition of the full distribution [J]. Journal of Health Economics, 2013,32(1):88-105.
[8] GEORGE KARATZAS.On The Determination of The US Aggregate Health Care Expenditure[J]. Applied Economics 2000, 32, 1085-1089.
[9] Wu Minglong, Statistical analysis of the questionnaire practice-SPSS operation and applications. Chongqing: Chongqing University Press,2010.
[10] Zhang Meiying, He Jie. Summary on Time Series Forecasting Model[J]. Mathematics in Practice and Theory,2011, 41(18), 189-195.
[11] Xiong Pin. Data mining algorithms and Clementin practice[M]. Beijing: Tsinghua
University Press, 2011
[12]M.J.Zaki,N.Lesh,and M.Ogihara,andW.Li.Parallel algorithm for discovery of association rules.Data Mining and Knowledge Discovery,1 997
[13]Randy Crane, A Simplified Approach to Image Processsing , Prentice Hall, 1997.