n-bottom: 0px; -ms-text-justify: inter-ideograph;">as reported in the official TRECVID2002 submissions for those feature/site combinations are presented in Table 1 below.
Table 2. Average Precision of the features used
Feature
|
Average Precision
|
Monologue
|
0.0086
|
Speech
|
0.7103
|
Music
|
0.2221
|
Indoor
|
0.3348
|
Outdoor
|
0.6091
|
Landscape
|
0.1337
|
Cityscape
|
0.3479
|
People
|
0.1543
|
Face
|
0.2707
|
Text Overlay
|
0.4181
|
The accuracy of the feature evidences donated by groups, including ourselves, which we incorporated into our search system in many cases was disappointingly low, though at the time when we did use them these performance figures were not available. In addition, many features were not good discriminators between shots or between videos. In section 3 we outlined our use of an inverse document frequency factor to address these problems, however because of the poor discrimination power this would not have been sufficient in some cases. For example, the following timeline shows the top ranked video as a result of querying “Face” and “Indoor” segments.

Figure 8. The 1st video programme’s timeline, when the query was Face & Indoor
As can be seen, the SVG display of the combined Face and Indoor scores (top half of the timeline) is nearly flat indicating very similar scores across the entire video, and almost all content was detected with Indoor (second line from top spanning from the beginning to near the end), which not very useful for navigation into this video other than indicating that this video is full of indoor scenes. On the other hand, Figure 9 shows the timeline of the top- ranked video as a result of query for all “Face” features and the ASR term “Abraham Lincoln” (for Topic #4 “find shots with a depiction of Abraham Lincoln”):
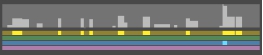
Figure 9. The 1st video programme’s timeline, when the query was Face & term: “Abraham Lincoln”
The timeline in Figure 9 clearly highlights parts of the video where the query was matched and directs the user to a few video
segments. The area on the top half of the timeline which is highest (where both the top line (yellow), and second from bottom line (blue) appear at the same column in Figure 7) is where the Face was detected with the ASR text for “Abraham Lincoln”
4.2.2 User Issues
Figure 6 suggested that ‘System A’ had two users from the sample of six, who had very different searching abilities compared to the others. The ability of users has to be taken into account in building systems for video navigation. We assessed each of these users’ logs further and noticed that the user returning the most relevant documents was not using features in its original query whereas the rest of the users valued features much more highly. This brings us to the issue of whether features were of benefit to the user in formulating queries at all. It also suggests that given the facility to use features and the option to use either ASR only or ASR and features, the users made use of both the ASR and features in generating a query 75% of the time.
Let us again consider (Topic 18): - “ Find shots with one or more sailboats, sailing ships, clipper ships, or tall ships - with some sail(s) unfurled”. A typical query generated for this topic is:
Text= sailing ship boat
Location= OUTDOOR
Location Scape = LANDSCAPE.
When a user clicks on a video title from the ranked video listing, the first browsing screen presented to the user is the overview of that video, with a short textual description and thirty keyframes. The thirty keyframes were selected from throughout the video’s content at certain time intervals and were intended to provide a single-glance view of the video content.
Observed user behaviour during the experiments showed that users would often discard a video based on the keyframes of the overview without actually examining the shots within that video. While this thirty-keyframe overview of the video serves well to show the rough content of the video, it could mislead the user if keyframes relevant to the user’s query did not appear in the overview, thus causing users to miss relevant shots. We noticed that when users searched for Topic #4 (“find shots with depiction of Abraham Lincoln”), the video concerned showed Abraham Lincoln’s face as one of the thirty keyframes, and users instantly clicked this to go to that shot. However, if that keyframe had not been selected among the thirty keyframes, the user might simply move on to the next video programme, completely missing the relevant shot. This example is repeated in other topics also. We consider that the overview of the video should show a more query-based overview [16] rather than the general overview as at present, that is, we should select thirty keyframes based on the scores of the shots, rather than simply by time length as currently.
5. CONCLUSION
In this paper we have examined the variations between two systems for video information retrieval which are almost identical in nature and design, except that one incorporates feature-based as well as ASR based searching. The systems have been tested in a laboratory setting, with twelve users conducting search tasks on TRECVID topics. We were interested in the level of effectiveness the system provided for users when searching for a particular topic in a video collection. We obtained ratings and user comments as part of laboratory testing sessions from the test users, to analyse users’ opinions and ideas on the system and its various features. Our user interaction logs indicate that given the opportunity to use features, users of ‘System A’ (features-based) incorporated features into seventy-five per cent of their queries. However, although features were an important aspect of query formulation, the inclusion of these features did not improve retrieval performance to any notable extent.
Due to the large amount of variability in the reported accuracy of the features we used, along with the fact that there is a high variability among users, it is necessary to devise an evaluation strategy that reduces user variability and facilitate inter-system comparisons. This evaluation strategy will measure and compare the effectiveness of two system variants (A and B), which allows one user to use both systems while at the same time never letting a user see a given topic more than once. In order to do this the user must alternate between the two systems, progressing through the topics. For future experiments we strongly believe that even when perceived user variance is regarded as being minor, that within- subject testing of the two system variants should be performed in preference to between-subject testing of two system variants. If nothing else, our results illustrate that there is high subject variance on the same system over the same topics, between a group of users with similar background and age profile.
The entire evaluation of our Físchlár systems has provided us with a qualitative and query based overview from which we have been able to identify the strengths, problems, and issues with the evaluation for our digital video retrieval system. The evaluation has also allowed us to do comparisons among variations of our own system, but comparisons with other systems at other sites. Although this is a stated aim of TRECVID, the fact that this was not possible in the 2002 TRECVID is because the activity of the track has not yet matured to that state. TRECVID has not yet established a benchmark that can be used across sites to support cross-site system comparison. At this point, the TRECVID activity has the requisite data, has an understanding of the issues involved in cross-site comparison of video IR when the search is interactive, and has the momentum to make progress in this area. Many aspects of interactive video navigation such as combining text and features-based search as we have reported in this paper, local browsing among temporally close shots within a single video, and following information links which span across videos, these are aspects for which TRECVID is not yet mature enough to facilitate in within-site or cross-site comparisons. Progress in this can be expected in the next few years.
Acknowledgement: Part of this material is based upon work supported by the IST programme of the EU in the project IST- 2000-32795 SCHEMA. The authors would like to thank the Informatics Research Initiative of Enterprise Ireland and the Irish Research Council for Science, Engineering and Technology for support. We also thank MSR Asia and IBM for providing feature detection output to DCU and all the TRECVID participants.
7. REFERENCES
[1] TREC2002 Video track. Available online at URL: http://www-nlpir.nist.gov/projects/t01v/t01v.html (last visited May 2003)
[2] Lee, H. and Smeaton, A.F. Designing the user interface for the Físchlár Digital Video Library. Journal of DigitalInformation, Special issue on Interactivity in Digital Libraries, 2(4), 2002.
[3] Lee, H. and Smeaton, A.F. Searching the Físchlár-NEWS Archive on a mobile device. Proc. 25th International ACM on R&D in Information Retrieval, Workshop on Mobile Personal Information Retrieval (SIGIR 2002), Tampere, Finland, 11-15 August, 2002.
[4] The Internet Archive. Available online at URL: http://www.archive.org/movies/ (last visited June 2003)
[5] The Open Video Project. Available online at URL: http://www.open-video.org/ (last visited July 2003)
[6] McDonald, K., Smeaton, A.F., Marlow, S., Murphy, N. and O’Connor, N. Online television library: organisation and content browsing for general users. Proc. of the SPIE Electronic Imaging – Storage and Retrieval for Media Databases, San Jose, CA, 24-26 January, 2001.
[7] Savoy, J. and Picard, J. Report on the TREC-8 experiment: searching on the Web and in distributed collections. The 8th Text Retrieval Conference (TREC-8), Gaithersburg, MD, 1999.
[8] Dimitrova, N., McGee, T. and Elenbaas H. Video keyframe and filtering: a keyframe is not a keyframe to everyone. Proc. of the 6th ACM International Conference on Information and Knowledge Management (CIKM '97), Las Vegas, NV, 10-14 November, 1997.
[9] Wactlar, H., Kanade, T., Smith, M. and Stevens, S. Intelligent access to digital video: Informedia project. Computer, 29(5), 1996.
[10] Smith, J.F. Searching for images and videos on the World- Wide Web. Technical report #459-96-25, Center for Telecommunications Research, Columbia University, 1996.
[11] Zhang, H., Low, C., Smoliar, S. and Wu, J. Video parsing, retrieval and browsing: an integrated and content-based solution. Proc. of 3rd ACM International Conference on Multimedia (MM ’95), San Francisco, CA, 5-9 Nov, 1995.
[12] Wolf, W. Keyframe selection by motion analysis. Proc. of the International Conference on Acoustics, Speech, and Signal Processing (ICASSP '96), Atlanta, GA, 7-10 May, 1996.
[13] Aoki, H., Shimotsuji, S. and Hori, O. A shot classification method of selecting effective key-frames for video browsing. Proc. of the 4nd ACM International Conference on Multimedia (MM '96), Boston, MA, 18-22 November, 1996.
[14] Uchihashi, S., Foote, J., Girgensohn, A. and Boreczky, J. Video Manga: generating semantically meaningful video summaries. Proc. of the 7th ACM International Conference on Multimedia (MM '99), Orlando, FL, 30 Oct - 5 Nov, 1999.
[15] TREC Interactive track. Available online at URL: http://www-nlpir.nist.gov/projects/t11i/t11i.html (last visited July 2003)
[16] Christel, M., Winkler, D. and Taylor, R. Improving access to a digital video library. Proc. of the 6th IFIP Conference on Human Computer Interaction, Sydney, Australia, 14-18 July, 1997.
,
Design, Implementation and Testing of an Interactive Video Retrieval System
Georgina Gaughan
Centre for Digital Video Processing Dublin City University
Ireland 353 1 700 8448
ggaughan@computing.dcu.ie
Alan F. Smeaton
Centre for Digital Video Processing Dublin City University
Ireland
asmeaton@computing.dcu.ie
Cathal Gurrin, Hyowon Lee, Kieran McDonald
Centre for Digital Video Processing Dublin City University
Ireland
ABSTRACT
In this paper we present and discuss the system we developed for the search task of the TRECVID 2002, and its evaluation in an interactive search task. To do this we will look at the strategy we used in designing the system, and we discuss and evaluate the experiments used to determine the value and effectiveness of one system incorporating both feature evidence and transcript retrieval compared to a transcript-only retrieval system. Both systems tested are built on the foundation of the Físchlár System developed and running for a number of years at the CDVP. The system is fully MPEG-7 compliant and uses XML for exchange of information within the overall architecture.
Categories and Subject Descriptors
H.3.3 [Information Storage and Retrieval] Information Search and Retrieval – search process, query formulation.
General Terms
Algorithms, Measurement, Performance, Design, Experimentation, Human Factors.
Keywords:Video Retrieval, Video Analysis, Video Browsing, System Evaluation, Usability Testing, Interface Design.
1. INTRODUCTION
Multimedia information retrieval has significantly evolved over recent years with the development of many digital libraries and the WWW allowing browsing and retrieval of multimedia content. Each year research groups from all over the world get an opportunity to evaluate their progress in developing and enhancing information retrieval systems using the TREC (Text Retrieval Conference) guidelines and common evaluation procedures. NIST (National Institute of Standards and Technology), the organising body behind TREC, provide a set of documents and a set of search topics in electronic form to all participating groups. Groups then run their own information retrieval applications using the provided topics against the corpus,
Permission to make digital or hard copies of all or part of this work for personal or classroom use is granted without fee provided that copies are not made or distributed for profit or commercial advantage and that copies bear this notice and the full citation on the first page. To copy otherwise, or republish, to post on servers or to redistribute to lists, requires prior specific permission and/or a fee.
MIR’03, November 7, 2003, Berkeley, California, USA. Copyright 2003 ACM 1-58113-778-8/03/00011…$5.00.
and send their results back to NIST. The returned results are manually assessed for relevance thus creating a ground truth and enabling comparative assessments among all the submitted results. Use of a single common corpus and a single evaluation strategy creates a consistent basis for benchmarking different systems’ performance. TREC supports experiments into different aspects of information retrieval with different tracks introduced since the establishment of TREC in 1992 e.g. the Interactive track introduced in 1997 assessing interactive elements of IR systems and the Web track introduced in 1999 assessing retrieval performances from hyperlinked web page corpus.
The Centre for Digital Video Processing (CDVP) in Dublin City University participated in the Video track known as TRECVID [1] in 2002, conducting both the Feature Detection and Search tasks. TRECVID was first introduced in 2001 and has the same underlying goals of the overall TREC activity but focuses on content-based retrieval from digital video information. More than twenty groups worldwide, from university research groups to industry research groups participated in the second year of Video track 2002, and 35 are participating in 2003. As in all other tracks in TREC, NIST provided the participating groups with a corpus – a total of about 70 hours of digitised video documents in MPEG-1 format. This corpus was divided into three subsets to be used for 3 different tasks within TRECVID:
• The Shot Boundary Detection (SBD) task was an exercise to evaluate how accurately a system can automatically detect camera shot boundaries in video content.
• The Feature Detection task was introduced for the first time in the 2002 Video track. The aim of the track is to evaluate system effectiveness, of identifying simple semantic features within video content.
• The Search task was introduced to evaluate the performance of a retrieval system, by analysing the users ability to effectively and efficiently search through the large video corpus. Each participating group developed either an interactive or automatic video retrieval system with a front- end interface allowing access to the video corpus. The users within each group then used the twenty-five topics or queries provided by NIST to search through the video collection.
In 2003, a subsequent task on story bound detection in broadcast TV news has been added. For the Search task, we developed an interactive video search/browse system and evaluated it with real test users in a laboratory environment using the TREC topics. The system is a variation of the Físchlár Digital Video System [2], purposefully designed for conducting the Search task in the Video
track in TRECVID2002 [2]. The Físchlár system is a web-based video recording/indexing system that allows its campus-wide users to browse online TV schedules and request recording of a particular TV broadcast programme. The user can then browse and select a programme she or some other user has recorded to receive streamed playback from any point in the video. The system has been very popular, and at the beginning of 2003 had more than 300 hours of content online and 2,500 registered users within the University campus, accessing the system for studying, teaching and research purposes.
This paper sets out to examine the hypothesis that a system incorporating features derived from visual and audio aspects of video improves the overall retrieval performance in searching through a large video collection. To prove this we require users to search through a large collection of videos using both ASR (automatic speech recognition) only and a combination of ASR and feature-based systems. In the following sections we describe the systems designed for conducting the search task in TRECVID
– their architecture, search mechanism and the user interface (sections 2 and 3). We outline how the developed systems have been user-tested in a lab experiment before presenting our findings from this experiment (section 4).
2. SYSTEM DESCRIPTION
For the purpose of evaluation and system variant comparisons we developed two almost identical systems for TRECVID 2002 with a common underlying architecture from previous Físchlár systems (Figure 1). The two variants are referred to as ‘System A’ (Figure
2) which incorporated both features and ASR transcript searching (described later in this section) and ‘System B’ which incorporated ASR transcript searching only. ‘System B’ has a similar interface to that of System A, however we replaced the features panel by a text search box allowing users to search for a relevant shot using the ASR transcript.
2.1 System Architecture
The system has an XML-based architecture with its internal video description complying with the MPEG-7 standard. Figure 1 shows the overall architecture of the system with the internal XML description. When a user submits a query via the web- based interface, the web application processes it and sends the query to the search engine. More details of the search engine can be found in Section 3. The search engine sends back the retrieved results of both individual and combined scores to the XML generator which generates the necessary XML descriptions dynamically, to be used by appropriate XSL (extensible Stylesheet Language) stylesheets in order to render HTML and SVG (Scalable Vector Graphics) for display back on the user’s web browser.
2.2 The User Interface
One of the goals of designing a user interface to any system should be the provision of simple, straightforward and easy interaction that does not confuse the user. However a sophisticated multimedia system such as a video search system requires sophisticated interface elements for searching and displaying of results. Having an internal XML-based architecture allowed us to clearly separate the presentation of data in the interface from how the system operates internally, significantly helping the system development process where software
engineering and interface design can happen separately. To facilitate the search system’s display on a web-based interface, XSL and SVG have been extensively used along with XML descriptions.
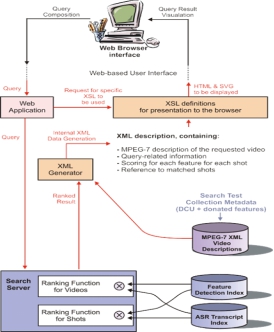
Figure 1. Architecture of Físchlár-TREC2002
2.2.1 Design
We designed the interface to accommodate all ten features that were donated to the TRECVID participants (see Figure 2) as this was essential for the hypothesis presented. We grouped the ten individual features into four conceptual, higher-level features groupings, as shown in Table 1.
People:
|
Face, Group of People
|
Location:
|
Outdoor, Indoor, Cityscape, Landscape
|
Audio:
|
Speech, Instrumental Sound, Monologue, ASR transcript search
|
Text:
|
Text Overlay
|
Table 1. Groupings of the features used
Each group has a distinct tab on the panel in order to save screen space while allowing gradual exposure to the user, thus preventing “too much” effect (top left in Figure 2). Once these groupings were established, we used them throughout the visualisation and interaction rather than using ten individual features separately. Each of the four groups had a distinctive colour consistently used throughout the interaction stages. Small icons were designed for
each of the ten features using one of the four colours designated according to the group they belonged to, providing a low but distinctive and consistent cue on the kind of feature the user is interested in. These icons were attached to the video shots that had those features with a high confidence value.
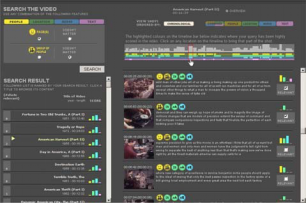
Figure 2. User interface for querying & browsing
2.2.2. Querying
During querying, a user is initially presented with the query panel on the top left of their screen (see top left Figure 2). The query panel displays one of the four feature groups in a tab arrangement, with Figure 2 showing the People group containing two features (Face, Group of people) The user specifies a query by selecting a relevant feature’s radio. All features have default radio buttons labelled “DOESN’T MATTER”. To select features other than Face or ‘Group of People’, the user must select one of the other tabs at the top of the query panel e.g. to enter a text query the user must select the ‘Audio’ tab containing the search box. When the query has been constructed the retrieval tool is activated returning
intuitive way. The initial screen a user sees when selecting a video programme from the search result display is an overview of the selected video with a subject description, and about thirty small keyframes automatically selected from throughout the video. At the top of the content browser the user has five options for viewing the shots list of the relevant video (see Figure 2). These include:- ‘Combined’, ‘People’, ‘Location’ ,‘Text’ and ‘Chronological’. Each of these buttons returns the ranked list of shots in that video by overall feature score, people score, location score and text overlay score, respectively. The ‘Chronological’ button displays the shot list, but in additions a timeline is displayed at the top of the list visualising the status of the user’s query matching within the video (see Figure 3).

Figure 3. Timeline visualisation of search result within a video
The top half of the timeline shows the combined score for each shot by the relative heights of the lighter grey bars, while the bottom half shows the individual 4 feature group matching status, highlighted where the query matched the shot over a threshold.
Below the timeline is a chronological shot listing corresponding to the horizontal progression of the timeline. Clicking on any part of the timeline brings up that part of the shot content in the shot listing below. Each shot entry in the shot listing presents the following information on the shot, as Figure 4 summarises.
Feature icons indicating that this shot contains Face, Indoor, and Speech
Score bars
representing the
a ranked list of relevant videos.
2.2.3 Viewing the Search Result
The initial search result is presented in the form of video programmes ranked in order of aggregate scores against the user’s query just below the query panel (see Figure 2). Individual
Keyframe representing the content of this
shot
Relevance checkbox
relative scores for 4 conceptual feature groups
feature detection scores are combined to form a single value for each video (explained in Section 3), thus allowing us to present a
ASR Transcript
displaying the portion of text spoken during this shot
For the user to indicate whether
this shot answers the query
list of ranked videos rather than a list of individually ranked shots from across different videos. Displayed adjacent to each video are the visual scores of the four feature groups in the form of bars, indicating the individual weighting of each feature group and which features were more influential in ranking that video highly. A number displayed on the left of each ranked video indicates how many shots the user has defined as relevant in that particular video program.
2.2.4 Browsing Video Content
When a user selects one of the video programmes on the ranked video listing, a content browser on the right side of the screen (see right side of Figure 2) supports browsing the video content. The video listing on the left side of the screen is used for visualising query result scores among the video programmes, but once a user moves into one particular video programme the issue becomes visualising query result scores among the shots within that video and presenting the individual shots’ contents in efficient and
For each shot there is the following information presented
• A representative keyframe – a keyframe selected from each shot by the system’s automatic keyframe extraction process.
• Icons for features detected in the shot – with confidence levels and the shot entry shows this by attaching icons for features whose confidence level are over a threshold. Restrictions are in place to ensure contradictory features are not selected simultaneously, i.e. indoor and outdoor.
• ASR transcript text – below the set of feature icons the dialogue spoken within that shot is displayed.
• Score bars – on the right side of each shot entry, 4 coloured bars are displayed indicating relative scores for each of the 4 feature groups with respect to the user’s query.
• Relevance checkbox – the user checks this box when she thinks this shot answers the given topic updating the number of relevant shots displayed on the left of each ranked video.
2.3 The TREC Video corpus
The video corpus was a closed set and thus all content-based analysis and indexing was done off-line. The data provided through NIST and available for the system to use was:
• 176 video programme files, total about 40 hours in total ( MPEG-1 format) varying from 1 to 28 minutes each.
• Mark-up data for pre-determined and agreed shot boundaries for all videos (in XML format).
• Mark-up data for ten features for each shot in the Video programmes (in XML format).
• Automatic Speech Recognition (ASR) transcript of all videos.
The video programmes were mostly 1940-70’s American government advertisement, campaign, and various documentaries on nature, history and society. Although all videos were of sufficient quality to be recognised and watched comfortably by a human eye, the relatively dated source of some material (‘40-‘70s) meant that the visual and audio quality of the videos were below the level which the Físchlár system has been tuned to handle, and so we had to go through a set of parameter adjustments for each of the system’s indexing modules for this particular video collection.
Each of the videos also had one paragraph of textual description provided by the content providers, the Internet Archive and Open Video Projects. In addition to the video corpus, common Shot Boundary Detection data was provided and available for all TREC participants to use, which in theory allowed easier reference to segments within the videos when comparing cross participant system results. The common SBD data has also been used in the Feature Detection task as a unit of referring to a video segment where a particular feature has been detected. Interactive systems from participating groups in the Search task used the shot units defined by this common SBD result as a basis for searching, browsing, displaying retrieval results to the users, selecting segments that answers the topics, and final submission to NIST.
Feature Detection data was also used in the Search task, provided in XML by three participating groups (Face, Music and Speech by DCU and other features by Microsoft Research Asia and IBM), giving a total of ten features. This feature data contained information on the appearance of a certain feature (e.g. faces within shots) in the video collection. For each shot, each of the ten features were scored in the range [0..1]. Participating groups were able to integrate these “donated” Feature Detection outputs into their search systems.
Also available to each participating group was the transcript of all videos in the corpus, generated and provided from automatic speech recognition (ASR) techniques developed by some of the participating groups (Microsoft Research Asia, Carnegie Mellon University and LIMSI France), who applied their ASR engines to all video programmes in the corpus. Transcripts were also marked up in XML format.
The video programmes in MPEG-1 format went through an off- line process in the Físchlár system to allow proper alignment with the above-mentioned Feature Detection data and ASR transcript
text, all previously segmented by the shot-level units defined by the common SBD.
3. SUPPORTING SEARCH & RETRIEVAL
In our Físchlár-TRECVID2002 system, a user composes a query consisting of zero or more required features and (if required) a text query for matching against the ASR transcript. Recall that a user’s search session is essentially a two-phase process, and the first phase generates a ranked list of videos where each of the 176 videos are scored and ranked before being returned in decreasing rank order to the user. In the second phase the user may select one of the videos (usually the highest ranked) for shot-level examination. Shot-level examination results in the retrieval tool producing a ranked listing of shots from within the selected video that match the user’s query.
Recall from our experimental hypothesis under examination here that we required users to search using ASR-only and using a combination of ASR and features. Hence, we developed a specialised retrieval tool, which was designed to support all required search tasks:
• ASR-only querying over 176 full video ASR descriptors;
• ASR-only querying over shot ASR descriptors from any one video;
• ASR + feature querying over 176 full video ASR descriptors and feature listings, and
• ASR + feature querying over shot ASR descriptors and feature listings from any one video.
For System B (ASR-only), the retrieval process only required the processing of text queries, however for System A the user could search through an index of ten automatically generated feature evidences, along with searching through the ASR transcript. We examine the retrieval methodologies employed for each separately.
3.1 ASR-only Searching (System B)
Search and retrieval functionality for System B was simply based on a conventional text retrieval engine. Each video was represented by the ASR transcript associated with all shots which comprise that particular video and each shot was represented by the ASR transcript portion associated with that shot. This resulted in 176 documents for video retrieval and 14,524 documents for shot retrieval. This required the use of two conventional (text- only) search engines, one for the 176 video descriptors and another for the shot descriptors, which had to support index partitioning. This was more efficient than ranking all shots and post-processing the ranked output to remove all shots not from a particular video.
Prior to indexing, the ASR transcript for each shot was pre- processed to remove stopwords (words that occur too frequently to aid the search process, “the”, “of”, “and” etc.) and then stemmed using Porter’s algorithm. The ranking algorithm we chose to employ for searching the ASR transcripts was the popular BM25 algorithm, which has proved its value in TREC experiments over a number of years. Our BM25 ranking was based on the following parameter values which were set according to the best performance achieved on the WT2g collection from TREC-8 [7] whereby advl = 900, b = 0.75, k1 = 1.2 and k3 =
1000. We note that additional experimentation would be beneficial to tune these parameters to best-fit ASR content.
3.2 ASR and Feature Searching (System A)
Search and retrieval functionality for System A was based on a conventional text retrieval engine (as outlined in section 3.1), but had to incorporate all of the 10 features detectors available in TRECVID 2002 into the retrieval process. Clearly the order in which the ranked shots and ranked videos are presented to the user will have a large effect on whether the user will find relevant shots. In order to provide as accurate a ranking of videos and shots as possible, we approached the ranking of shots and videos differently, as the bottom part of Figure 1 indicates. We will now examine our search and retrieval methodology for both videos and shots. Our algorithms were developed without using TREC topics and thus were not developed specifically to provide high retrieval performance on this particular corpus and associated queries.
3.2.1 Search and Retrieval of Video Units
Each of the 176 videos was represented by an overall feature weight for each of the ten features calculated from all shots within that video and then dividing these aggregate scores by the total number of shots in the video. In this way, we obtained an overall weight for each of the ten features within each video that was used in the video ranking process.
Without an exhaustive sampling of the accuracy of the feature detection we were using and given that our features originated from three separate participating groups we felt it best to normalise the weights of each feature so that no one feature would outweigh any other feature because of differences in confidence levels alone. Had we ignored these differences, the top weighted features would actually be weighted up to 5 times the higher than lower weighted features and this would obviously have influenced retrieval performance. In addition, we added one final weighting to each feature’s influence based on its usefulness as an aid to distinguishing between different videos. For this we adapted the conventional text-ranking technique called idf (inverse document frequency) which allowed us to increase the weighting of features that better support distinguishing between relevant and non- relevant videos. Letting FWtvf be the feature weight of feature f in video v, N be the number of videos in the system and vff be the video frequency of feature f, our idf calculation was based on the following formula:
In response to a user’s query, a ranked list of videos is then returned to the user for further consideration. The overall rank for each video was based on the summation of required (as specified in the query) feature influence along with the ASR search score (which had been normalised to be in the range of [0..1]). The influence of the ASR transcript in the video retrieval phase was weighted at 4 times that of any one feature. In this way, a user who selects a large number of features is illustrating the fact that features are important for a particular query and thus our ranking algorithm reflects this by allowing overall feature influence to outweigh the influence of the ASR text.
3.2.2 Search and Retrieval of Shot Units
When the user selects a ranked video from the first phase, the initial query (that has generated the video listing) is then sent to the retrieval tool in order to rank shots from within that video. The ranking algorithm used to rank shots within a selected video is similar to that used to rank the videos except that the normalisation of feature weights for shots was calculated at a shot level as opposed to the video level and the regulation of feature influence (based on idf) was also calculated at the shot level as opposed to the video level. This resulted in our weighting certain features, such as face and monologue, higher than others due to the fact that they are better discriminators between shots. In addition, our weighting of the ASR transcript score was less than that of the video ranking (at twice that of any one feature) because we felt that features would be of more benefit in ranking shots given that the video under examination is already considered relevant.
4. SYSTEM EXPERIMENT
The purpose of this experiment was to evaluate and analyse the effectiveness of ‘System A’ over ‘System B’. We carried out formal experiments following TREC guidelines that allowed us to compare the effectiveness of both systems for searching a video collection. The experiments enabled us to evaluate whether incorporating feature evidence (System A) into the search process improved retrieval performance over text-only searching (System B).
4.1 Experimental Procedure
Twelve people participated as test users, ten postgraduate students and two summer intern staff from the School of Computing within the University with the overall aim that individual differences between users would be relatively small enabling us to compare ‘System A’ and ‘System B’ more effectively, i.e. with less user variability. All users had advanced levels of computer knowledge and familiarity with web-based searching, each conducting some form of online searching daily. Twenty-five query topics were provided by NIST after our system had been developed. For the user experiment, we partitioned the twelve users into two groups; group A who used System A (features + ASR transcript) and group B who used System B (ASR transcript searching only) with six users in each group. To avoid the learning effect of the users as they progressed through twenty-five topics, for both group A and group B, three users conducted the twenty-five topics in forward order, and the other three used the same topics but in reverse order. Each user was given a four-minute time limit for searching a topic (including reading the topic and preparing the initial query). When the users had completed twelve topics they were given a short break before moving on to the next set of topics.
Each user was required to complete the TREC questionnaire, which has been developed over the past number of years by the TREC interactive track divided into pre-test, post-test and twenty- five post-topic questions. After a brief introduction, each test user was given a series of web pages presenting each individual topic, containing the audio/image/video/text examples that formed part of the topic descriptions.
A typical TRECVID2002 query is Topic 18. This query contained a text description “find shots with one or more sailboats, sailing ships, clipper ships, or tall ships - with some sail(s) unfurled”, three pictures of sailboats and two video clips. The user prepared an initial query using whatever aspects of the TREC topic they felt useful and proceeded to conduct their search. When a shot was located that the user thought answered the topic query, they indicated this by checking a small box immediately beside the shot (see Figure 4). At the end of the four minutes, the user filled in a short post-topic questionnaire. On completion of all the topics the user filled in the post-test questionnaire. All individual user interactions were logged on the system’s server, and the results were collected, processed and submitted to NIST for evaluation.
4.2 Results of the experiment
We present a comparison of the combined results of all six users for each of the video retrieval tools in an effort to address the issue of user variability. Each of the results below represents the interleaved union of the shots identified by the six users for each system variation. In comparing the precision and recall graph for all six users by combining the results together we can see that systems performance is roughly equal as shown in Figure 5.
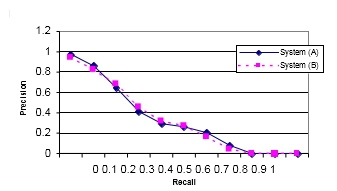
Figure 5. Precision-Recall graph, six users of each system
In Figure 6 we present an examination of the results of all six users for each system showing the number of relevant and non- relevant shots submitted by each (of six) users for each of two system variants. The graph shows that there is a much higher variance among the users of System A than System B (see Figure 6).
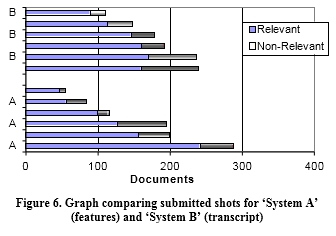
From Figure 6 we can clearly see that ‘System B’ (transcript-only) is far more consistent in returning a high percentage of relevant documents compared to ‘System A’ (all features). It is clearly visible that there is a greater variance with the incorporation of features into a retrieval system with ‘System A’ returning a standard deviation of 79 as opposed ‘System B’ returning a standard deviation of 46. This suggests that System A would highlight differences in user ability more easily than System B, and from Figure 6 we notice that clearly the best and worst performing users (in terms of number of shots found) were using
System A. However, we cannot discount that the ability of our users was different and that the users assigned to System A did
Examining the submitted results in more detail shows that the users of System A found 382 relevant shots over all 25 queries, whereas the users of System B found 388 relevant shots. The mean average precision of the aggregate users of System A is 0.3164 while the mean average precision for System B is 0.3105. This suggests that both systems are reasonably comparable, with no significant statistical difference in the results based on average precision for the aggregate results of all six users. Given that this is an interactive experiment involving the user actively making judgements as to which shots are relevant and should be submitted for assessment, this means that users will have already judged shots as relevant before submitting their results, and this explains the artificially high precision figures. Another reason why these results are somewhat misleading in that they represent the artificial scenario of a team of 6 users collectively working on a single search task, but they allow us to conform to TRECVID guidelines in our submissions and mean that all of the results we have submitted have been assessed. This in turn means that in our post-TRECVID analysis we can “untangle” our official submitted results and examine the performance of the two systems on a per- user basis to present a set of new performance results and a new analysis which is included here.
not perform as well as the users of System B.
Our experience of user testing and post-testing interviews highlighted important qualitative issues on the system usage when used by users undertaking a search task within a pressured time limit of only four minutes. One such issue is how much emphasis did users of System A place on features when generating their queries. Our user interaction logs indicate that features were incorporated into seventy-five per cent of all queries submitted by users of ‘System A’. In using the full feature system (System A) most users made use of ASR transcripts with 89% of all queries containing a text element within their query. One comment by User #7 in the post-test questionnaire sums up most of the test users’ opinions:
“I think the system relies on the spoken words. The most important feature for me was spoken words and the result on that was better than others.”
4.2.1 Accuracy of Feature Indexing
One possible reason for disappointing performance of feature- based video retrieval in our experiments could be that the quality of the donated features in terms of accuracy was not sufficient to adequately aid in the retrieval process. Examination of the average precision scores for the features that we used in our experiments
全套毕业设计论文现成成品资料请咨询微信号:HWFJ5828 QQ:1015083682
返回首页
如转载请注明来源于www.biyezuopin.vip