摘 要
随着全球金融市场的快速发展和信息技术的不断进步,投资者对股票价格预测的需求日益旺盛。准确的股票价格预测有助于投资者制定更为合理的投资策略,降低投资风险,提高投资效益。因此,研究股票价格预测问题具有重要的现实意义和应用价值。该选题也来源于机器学习领域的理论发展。近年来,深度学习技术在自然语言处理、图像识别等领域取得了显著成果,但在金融时间序列预测领域的应用仍处于不断探索和完善的阶段。LSTM作为深度学习中的一种重要模型,具有处理时间序列数据的优势,对于股票价格这类具有时序依赖性的数据预测具有天然的适应性。因此,利用LSTM进行股票价格预测,既能够丰富机器学习在金融领域的应用,也能够推动金融时间序列预测技术的发展。
本文旨在利用PyTorch这一基于Torch的Python开源机器学习库,构建基于长短期记忆网络(LSTM)的股票价格涨跌幅度预测模型。通过对股票信息进行多值量化分类,将股票预测问题转化为多维函数拟合问题。实验采用代号510050的上证股票历史交易数据作为数据集,将股票的历史基本交易信息作为特征输入,利用神经网络进行训练,并对股票的涨跌幅度进行分类预测。实验结果表明,该模型在单纯预测涨跌的情况下展现出较好的预测效果,为投资者提供了有价值的参考信息,有助于降低投资风险并优化投资策略。
关键词:PyTorch;长短期记忆网络(LSTM);股票价格预测;多值量化分类;深度学习;
Abstract
With the rapid development of global financial markets and the continuous progress of information technology, investors' demand for stock price forecast is increasingly strong. Accurate stock price forecast can help investors to develop more reasonable investment strategies, reduce investment risks and improve investment efficiency. Therefore, the study of stock price prediction problem has important practical significance and application value. This topic also comes from the theoretical development in the field of machine learning. In recent years, deep learning technology has made remarkable achievements in the fields of natural language processing and image recognition, but its application in the field of financial time series prediction is still in the stage of continuous exploration and improvement. As an important model in deep learning, LSTM has the advantage of processing time-series data and has natural adaptability for timing-dependent data prediction such as stock price. Therefore, using LSTM for stock price prediction can not only enrich the application of machine learning in the financial field, but also promote the development of financial time series prediction technology.
This paper aims to use PyTorch, a Torch-based Python, to build a prediction model of stock price rise and fall based on long-and short-term memory network (LSTM). The stock prediction problem is transformed into a multi-dimensional function fitting problem by conducting multi-value quantization classification of stock information. In the experiment, the historical trading data of Shanghai stock code 510050 is used as the data set, the basic historical trading information of stocks is taken as the characteristic input, and the neural network is used to train, and the rise and fall of stocks are classified and predicted. The experimental results show that the model shows a good prediction effect in the case of simply predicting the rise and fall, which provides valuable reference information for investors, and helps to reduce the investment risk and optimize the investment strategy.
Key words: PyTorch; long-and short-term memory network (LSTM); stock price forecast; multi-value quantitative classification; deep learning;
目 录
摘 要
Abstract
第一章 绪论
1.1 选题背景及意义
1.1.1 选题背景
1.1.2 选题意义
1.2 国内外研究现状
1.2.1 国外研究现状
1.2.2 国内研究现状
1.3 本课题研究主要内容
1.4 论文章节组织结构
第二章 理论基础与相关技术
2.1 Python简介
2.2 PyTorch简介
2.3 LSTM简介
2.4 当前股票预测的方法概述
2.5 本文所使用的模型
第三章 数据收集与处理
3.1 Tushare库与Talib库简述
3.2 历史行情数据的获取
3.3 实现代码
3.4 数据预处理
3.4.1 数据的归一化
3.4.2 训练集-测试集的分割
3.5 GUI界面设计与实现
3.5.1 设计思路
3.5.2 实现方法
3.5.3 主要功能
3.6 股票预测使用数据说明
3.7 特征工程处理
3.7.1 主成分分析方法(PCA)
3.8 输出数据说明
第四章 LSTM股票价格涨跌幅度预测模型构建
4.1 LSTM 模型概要
4.2 股票预测模型特征分析
4.3 LSTM结构的设计与实施
4.4 本次实验所采用的结构图
第五章 实验结果与分析
5.1 数据获取
5.2 Pytorch工具集概要
5.3 Pytorch 指令解析
5.4 搭建 LSTM 预测模型
5.5 GUI界面与模型集成的介绍
5.6 拟合结果评估方法概要
5.6 项目附件说明
5.7 项目使用说明书
第六章 总结与展望
6.1 总结
6.2 展望
致 谢
参考文献
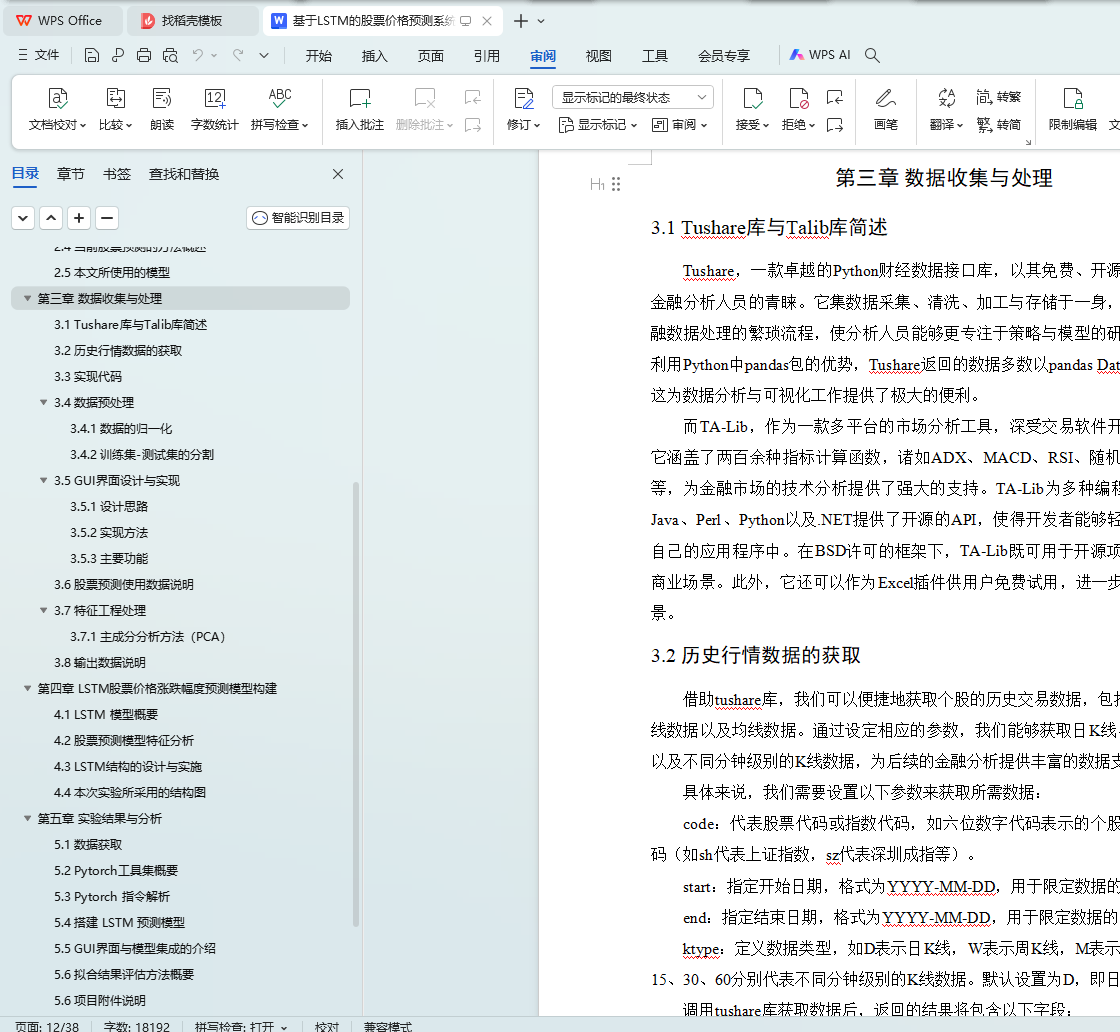
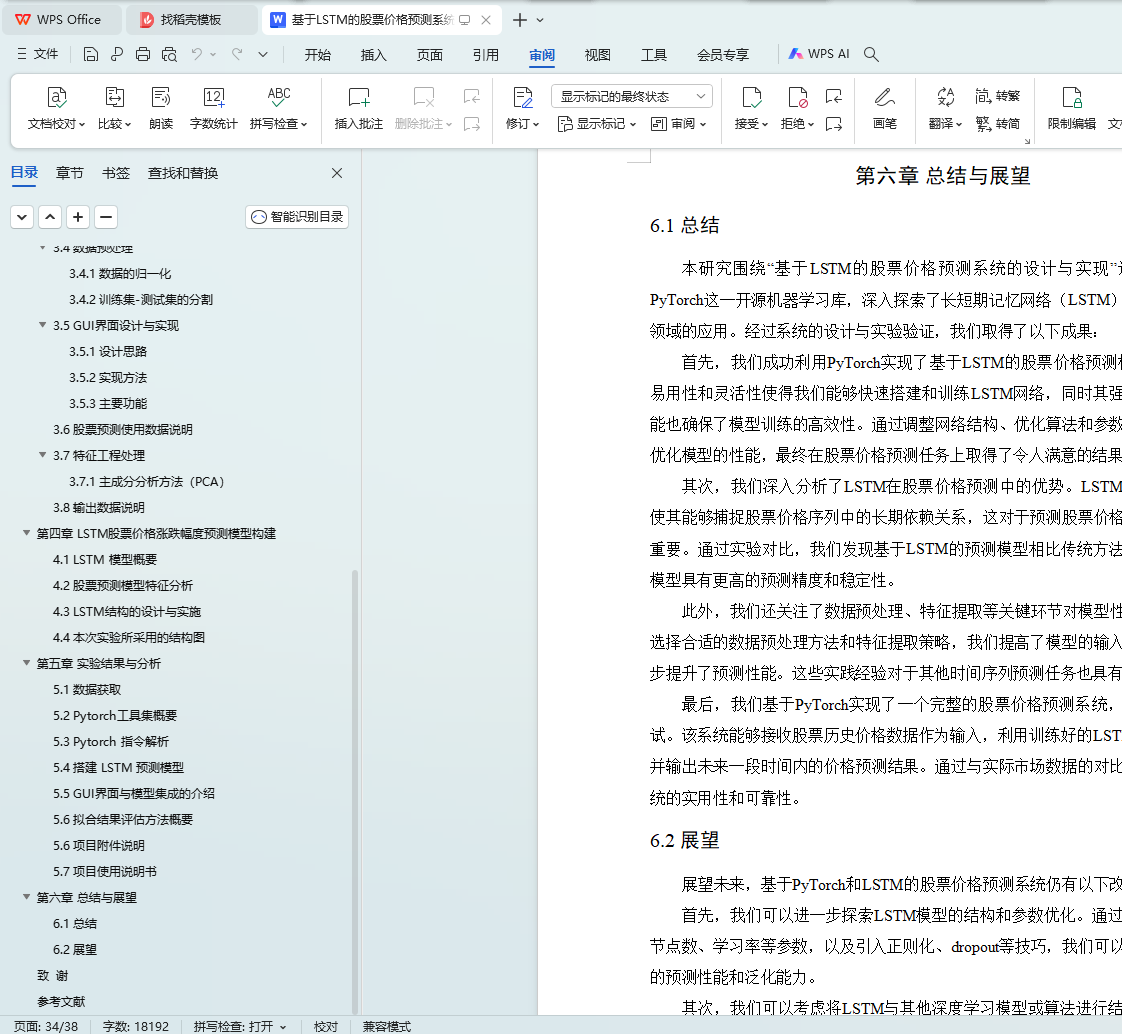
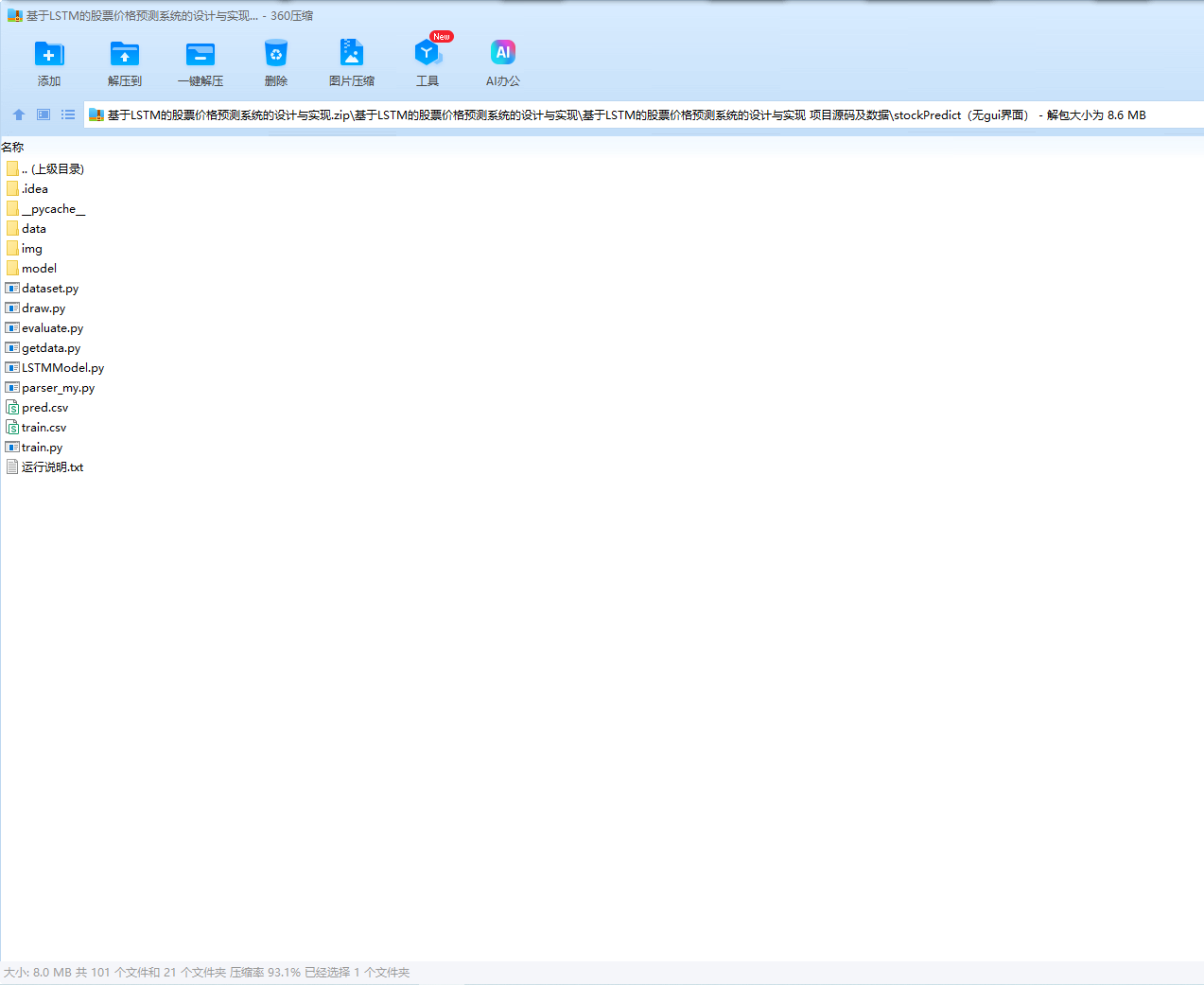
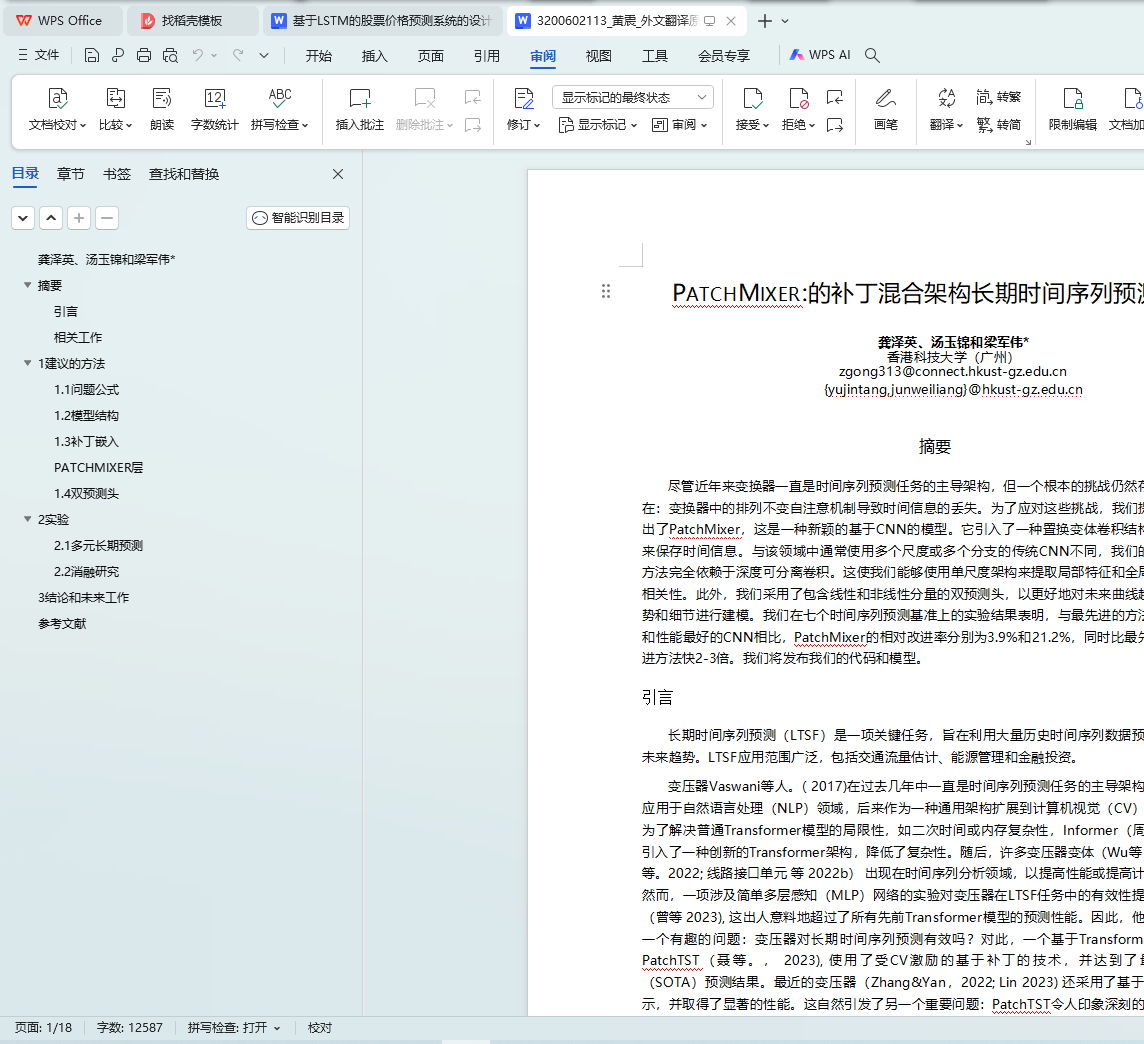
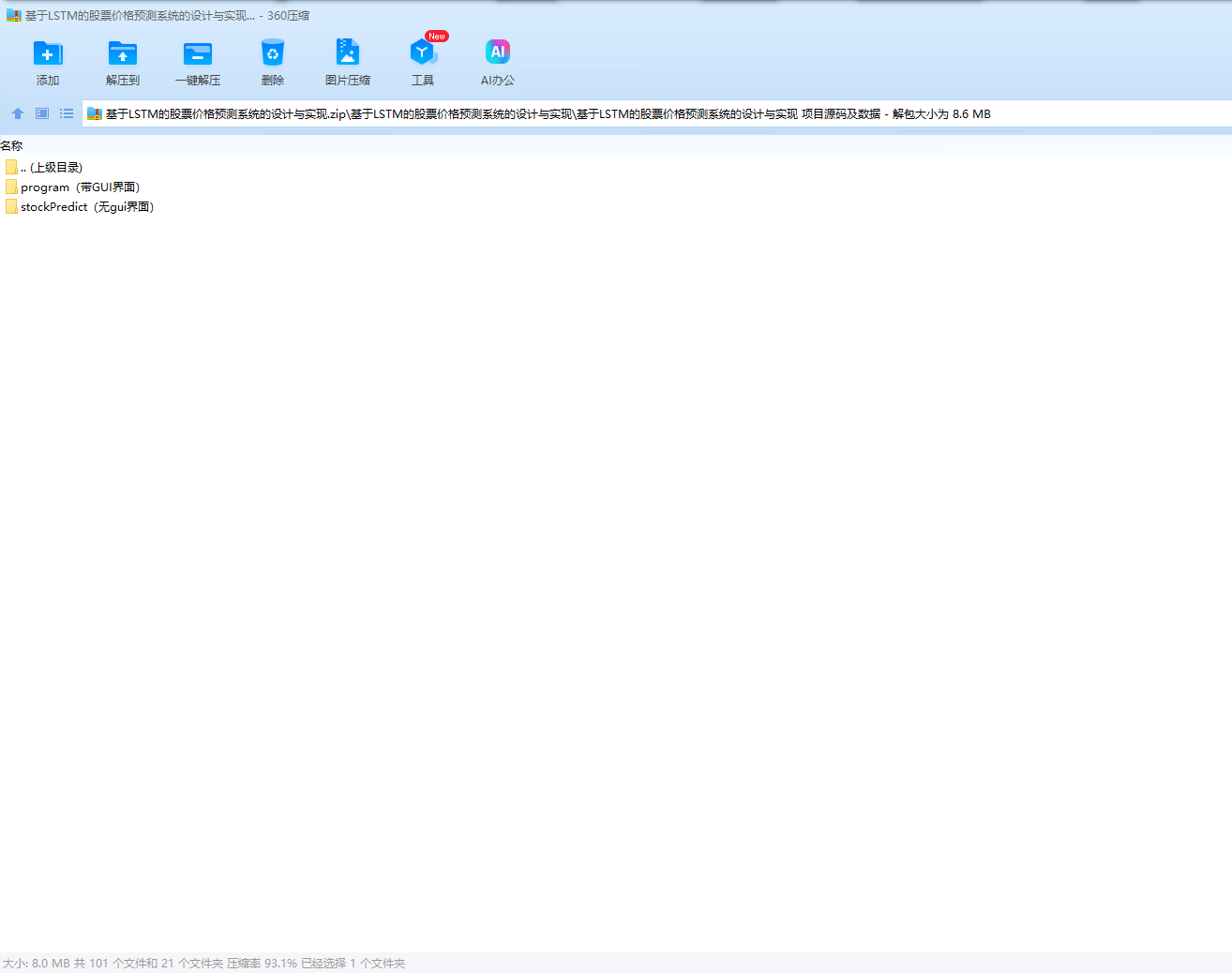
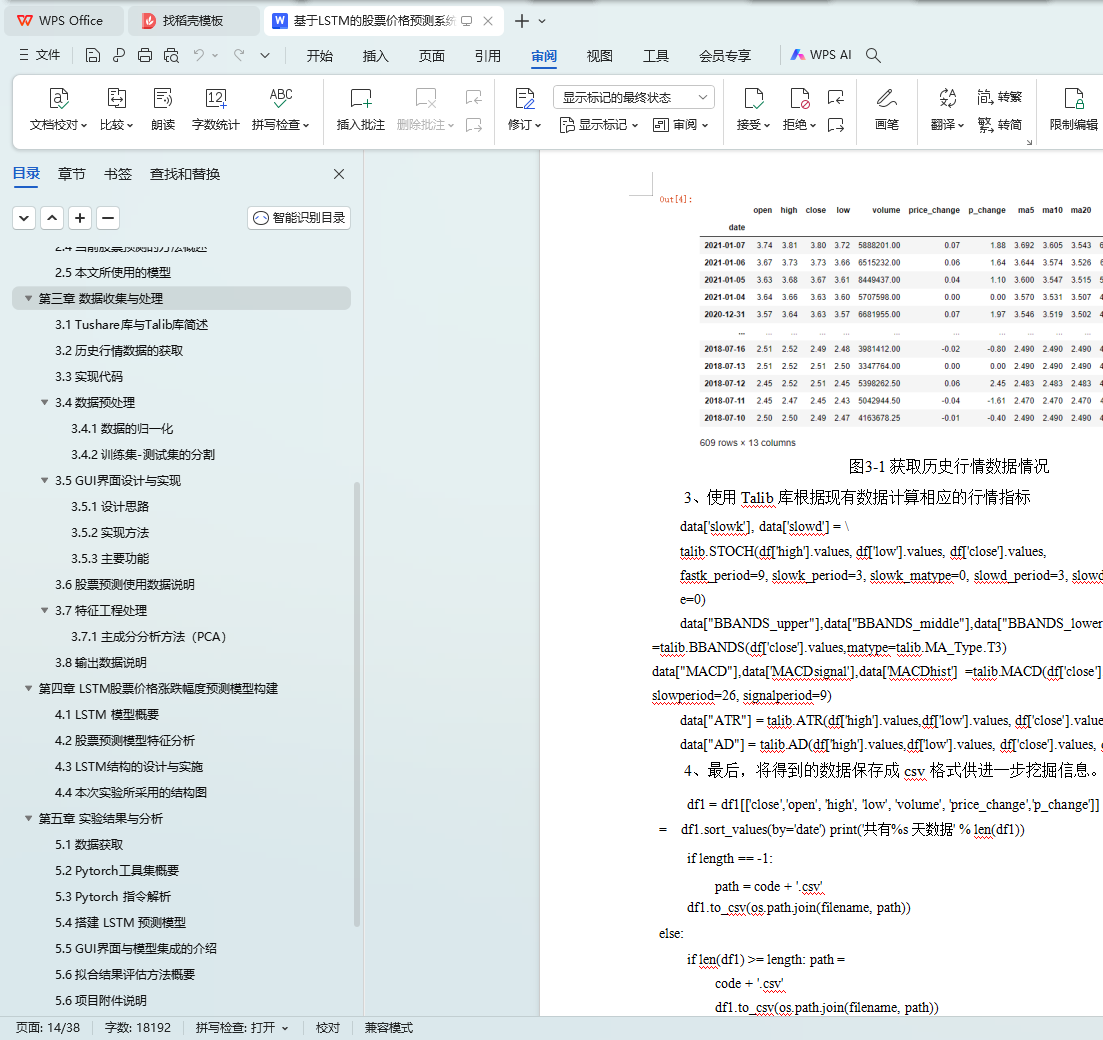
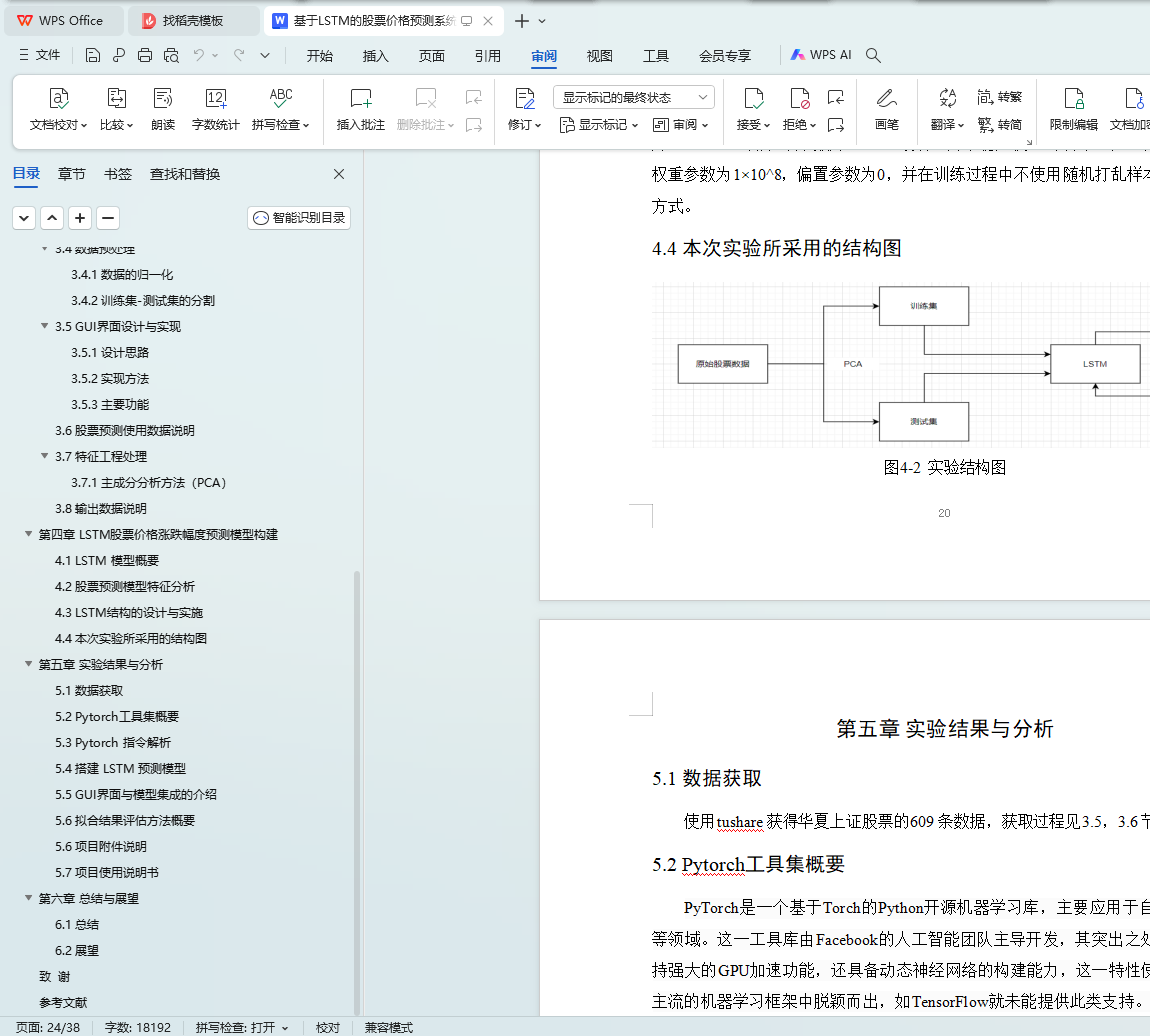
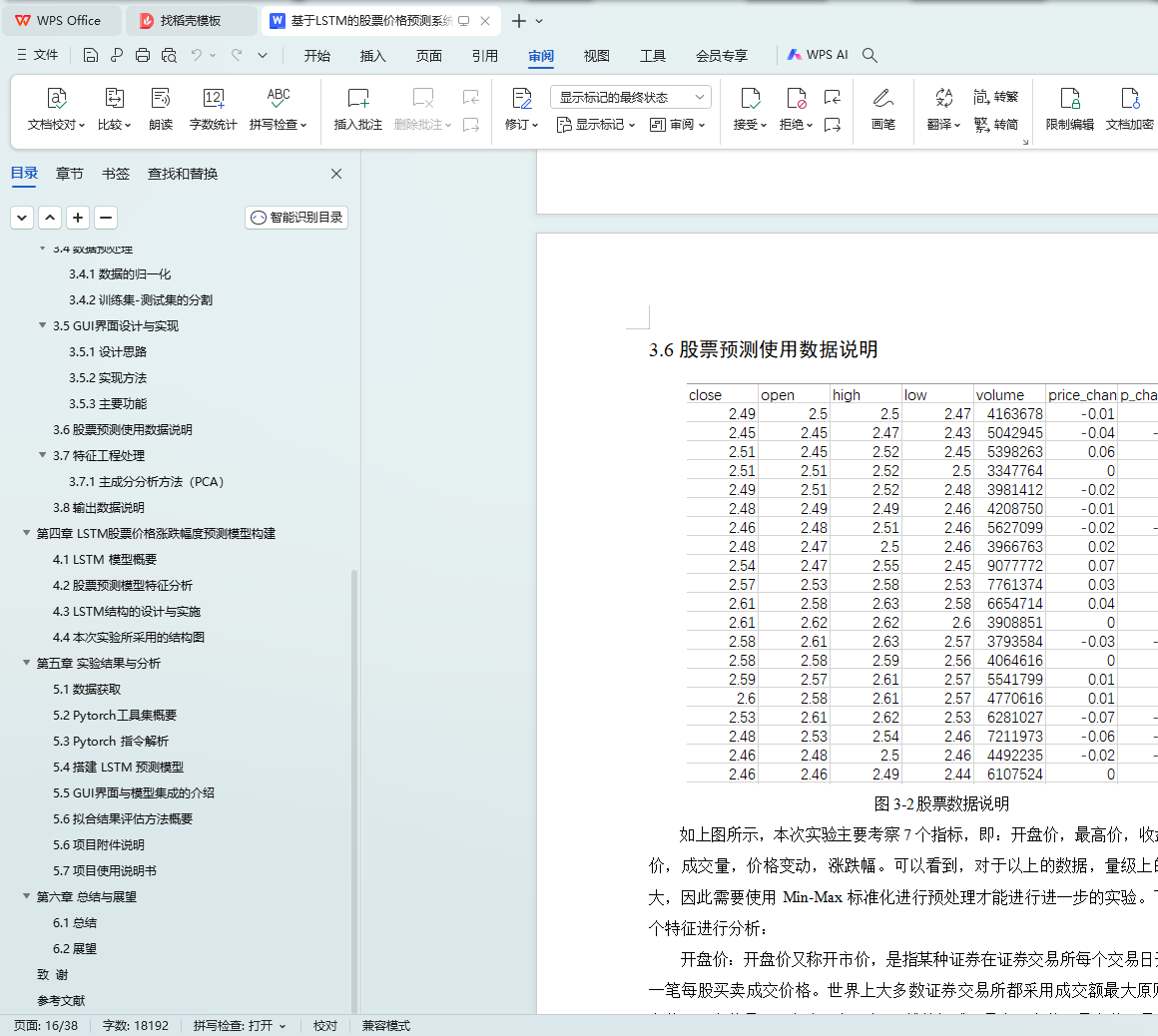
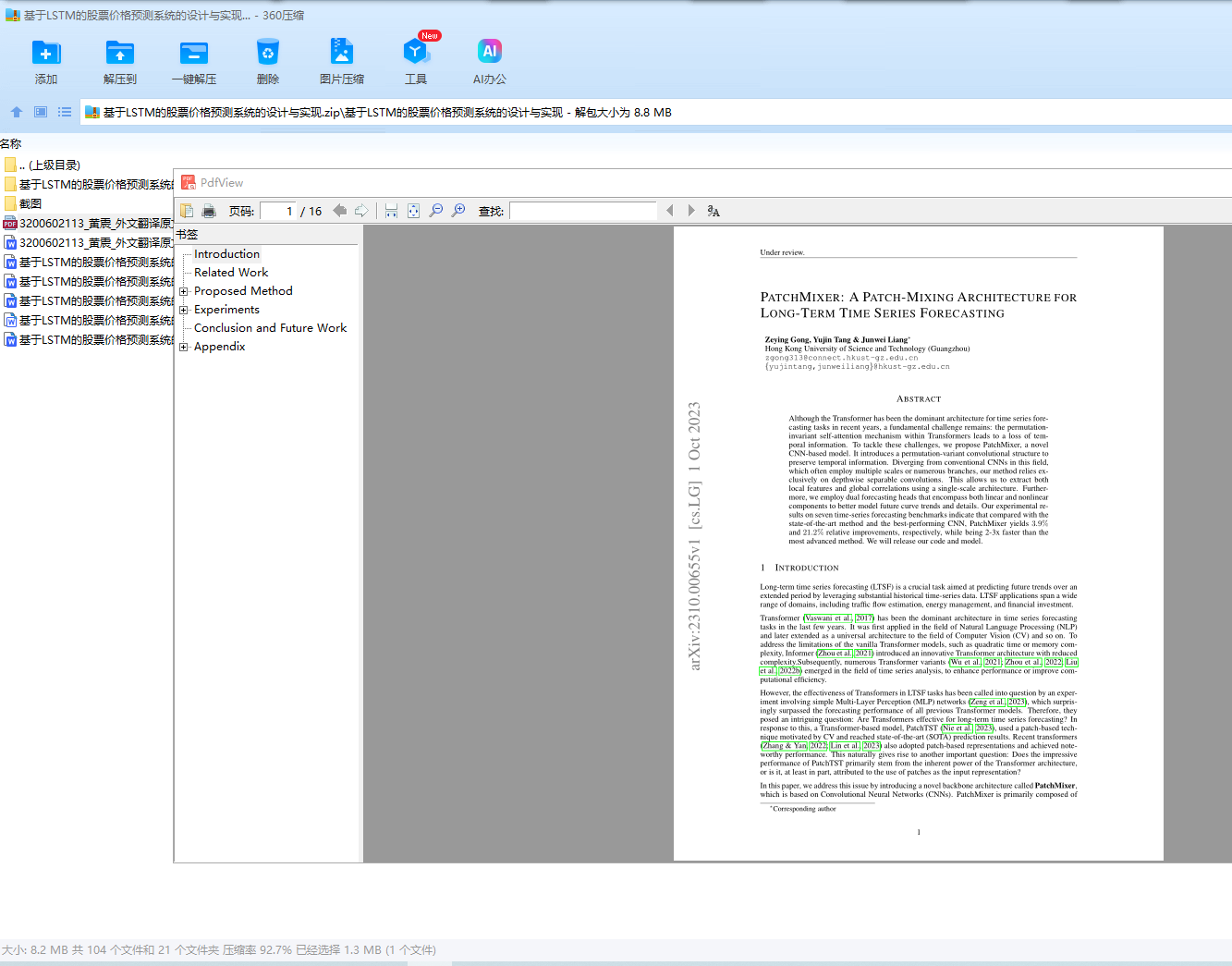
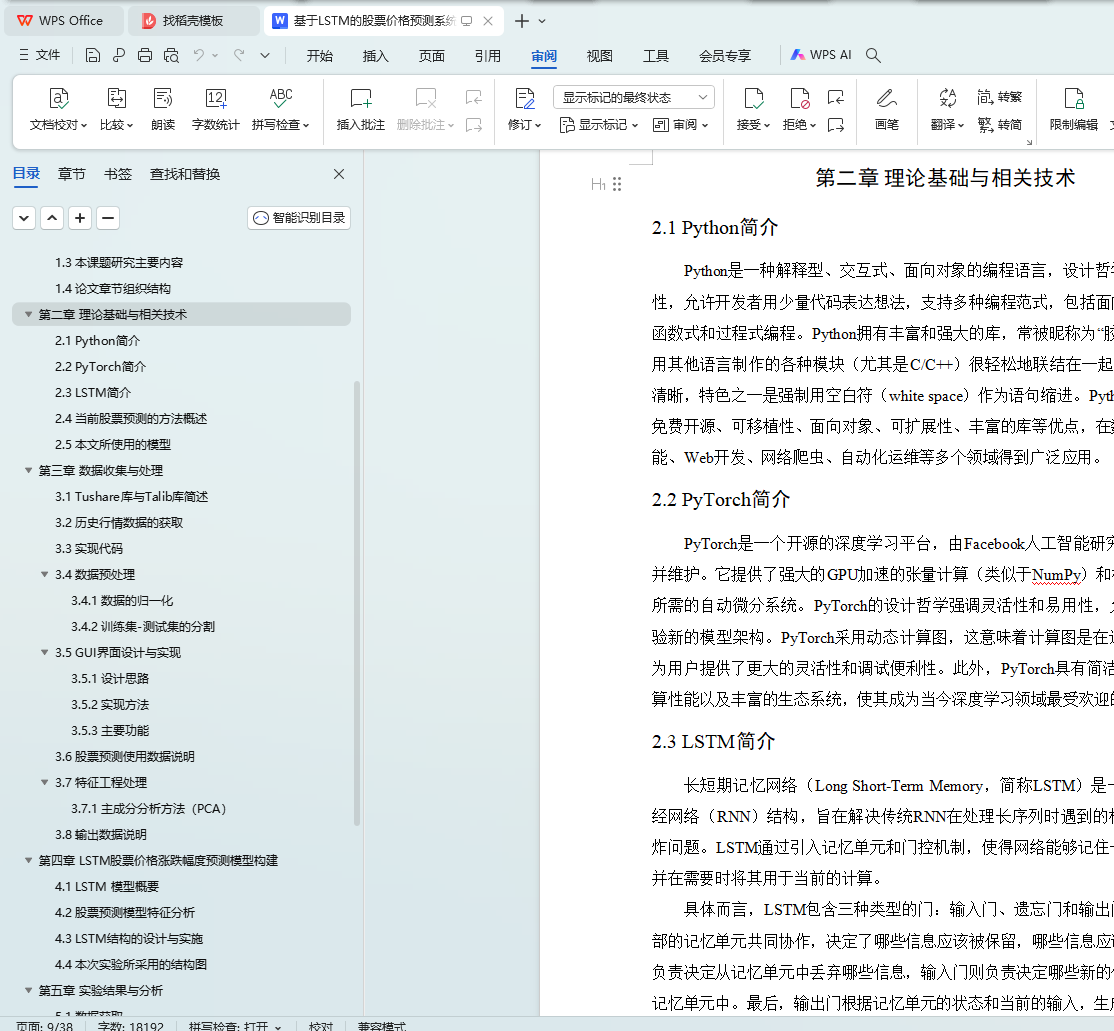
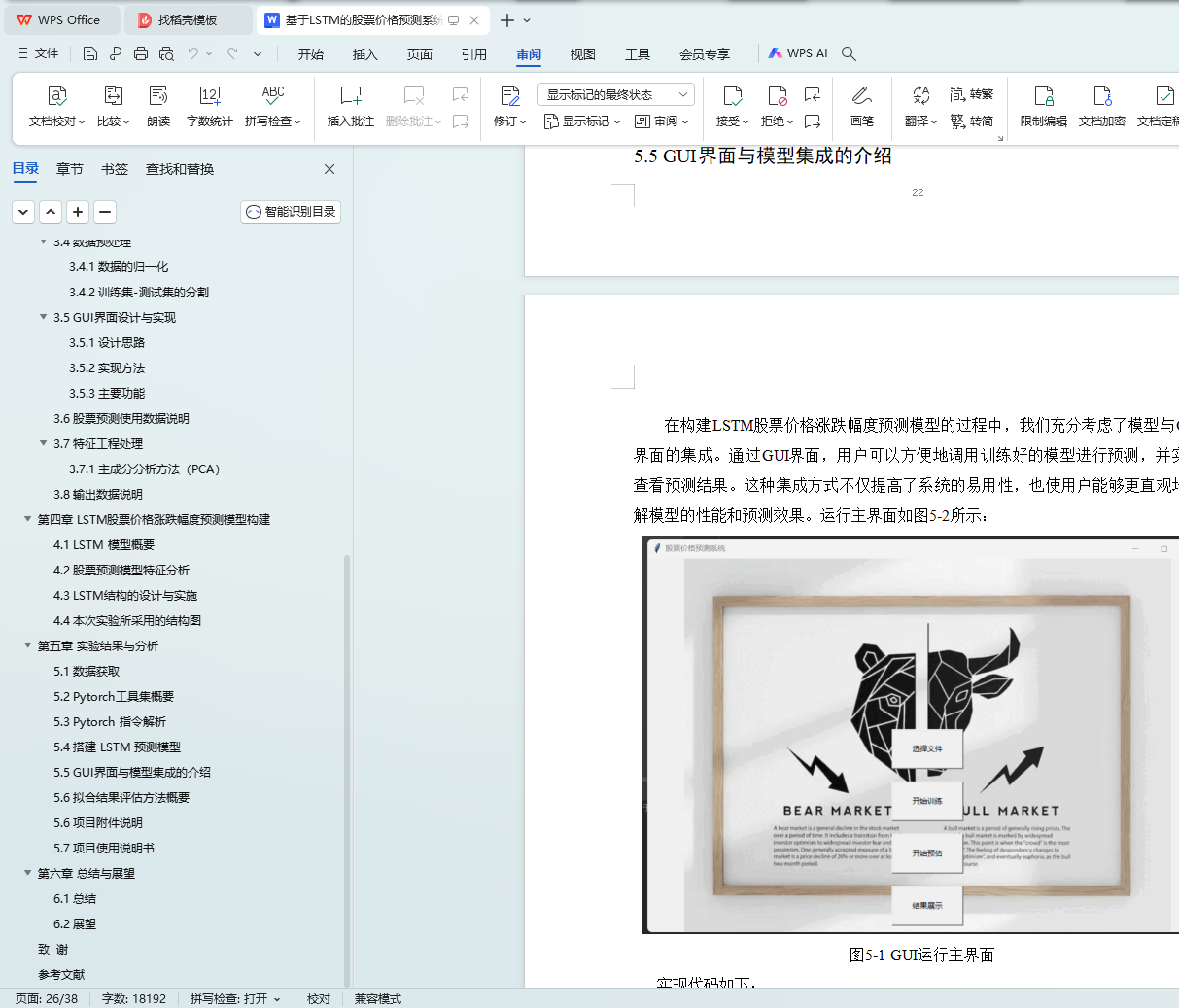
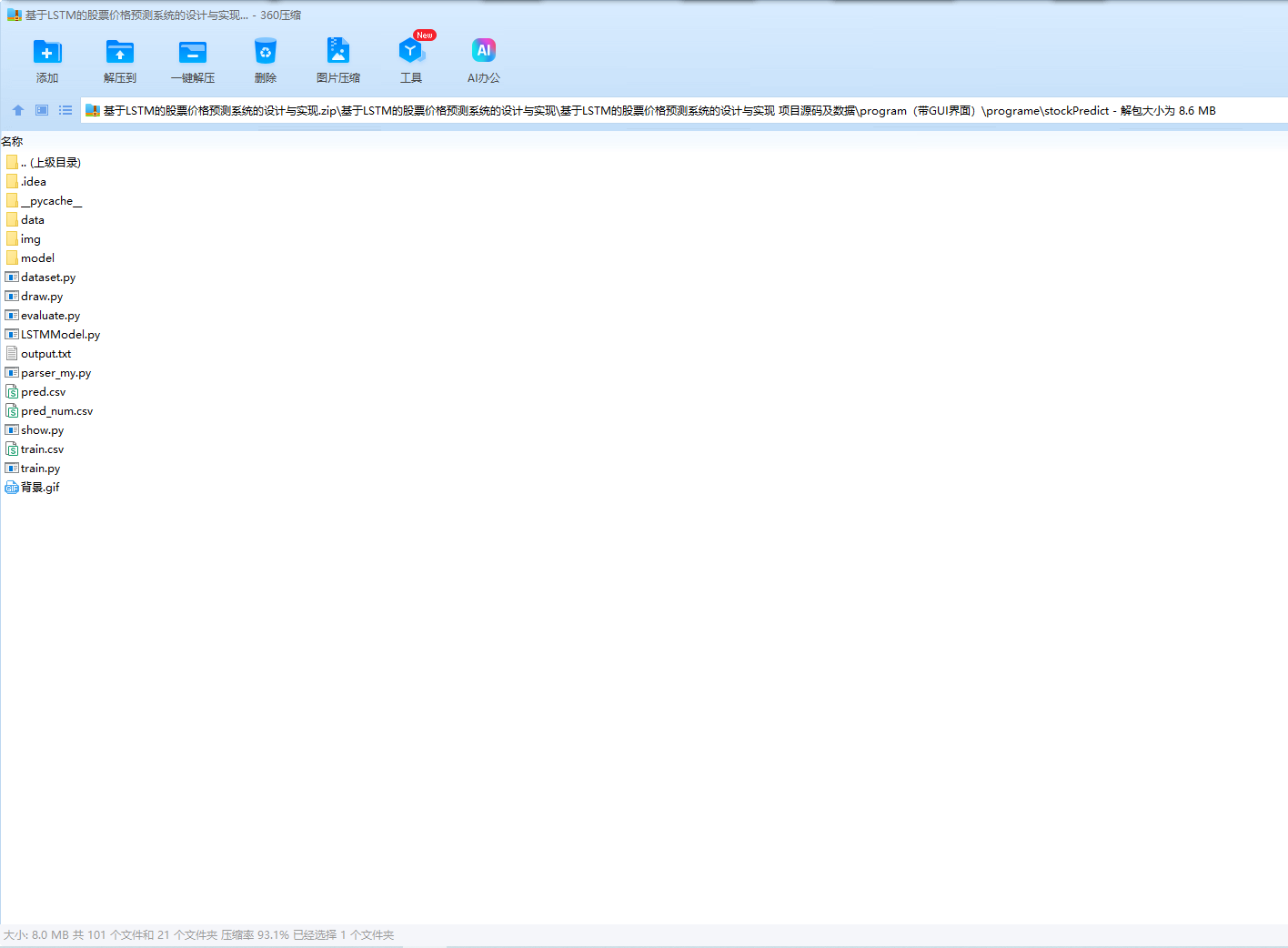
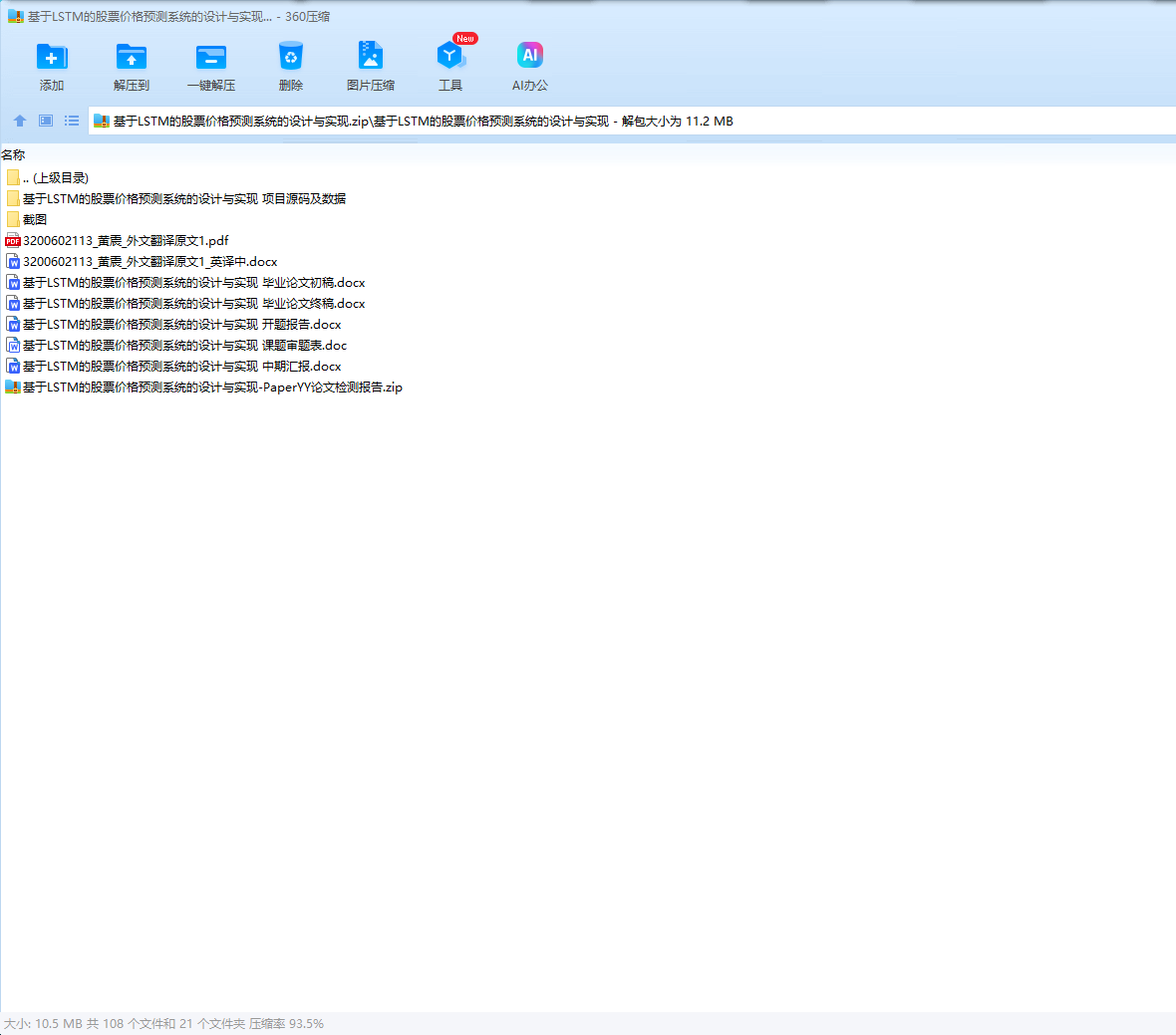
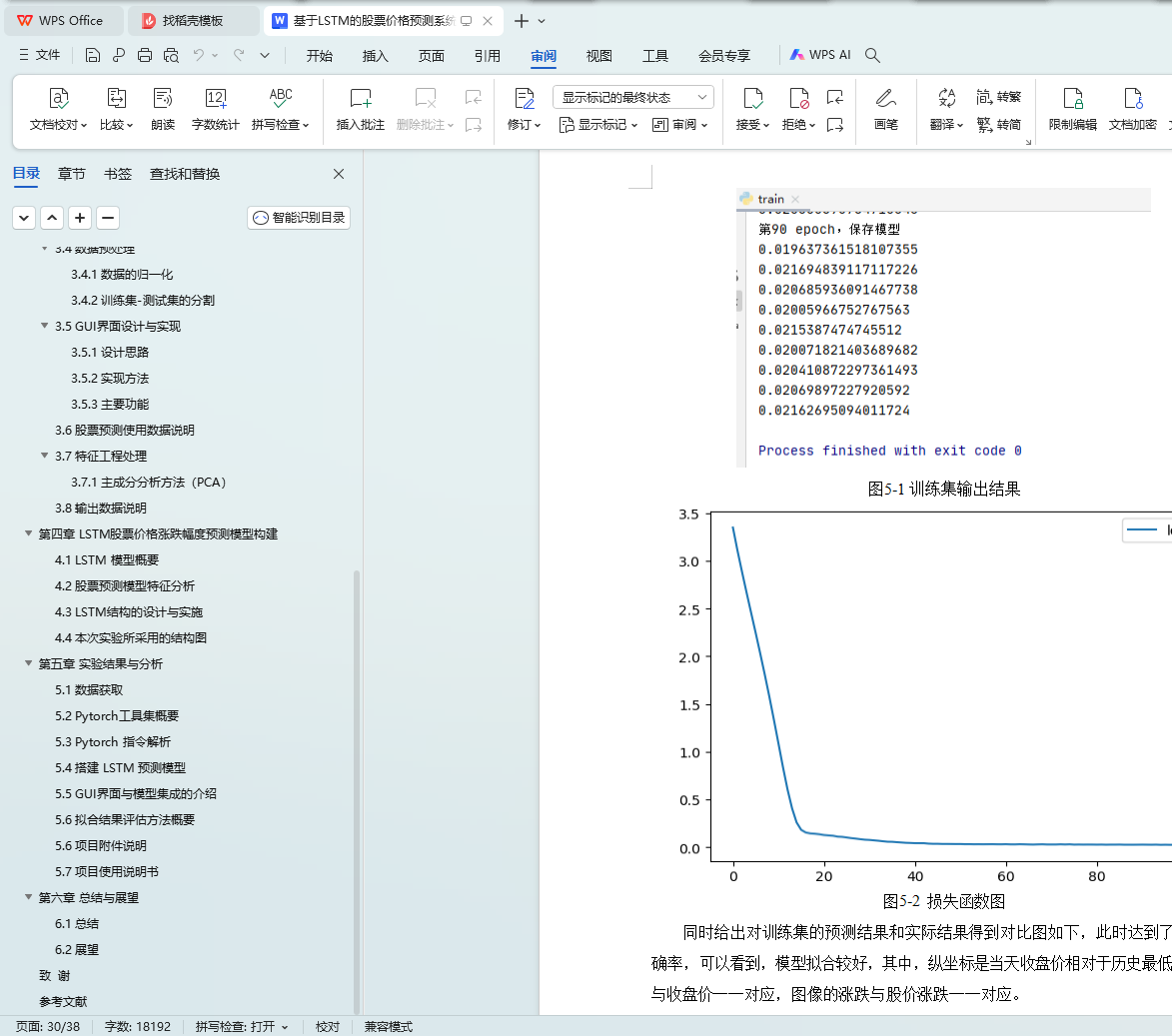
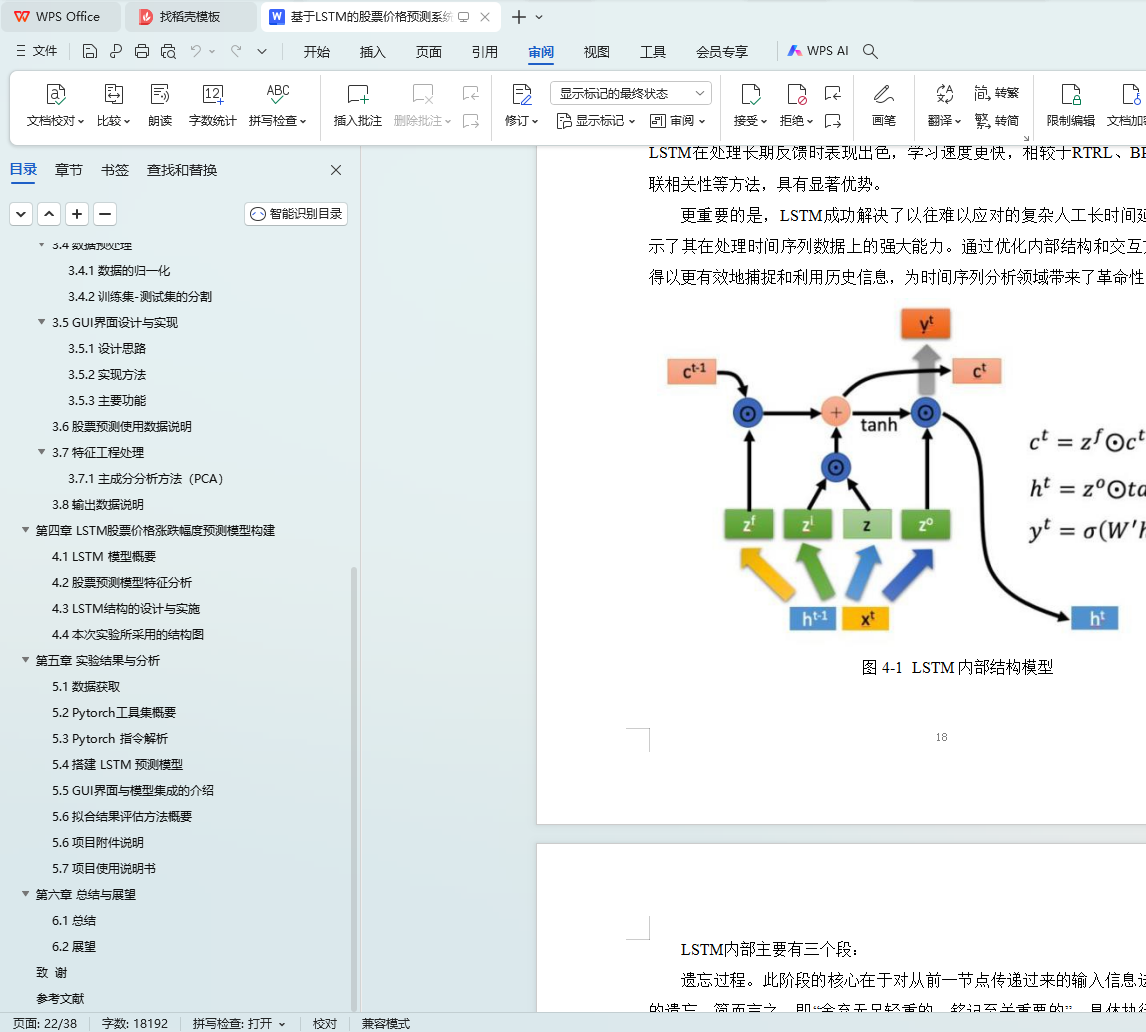
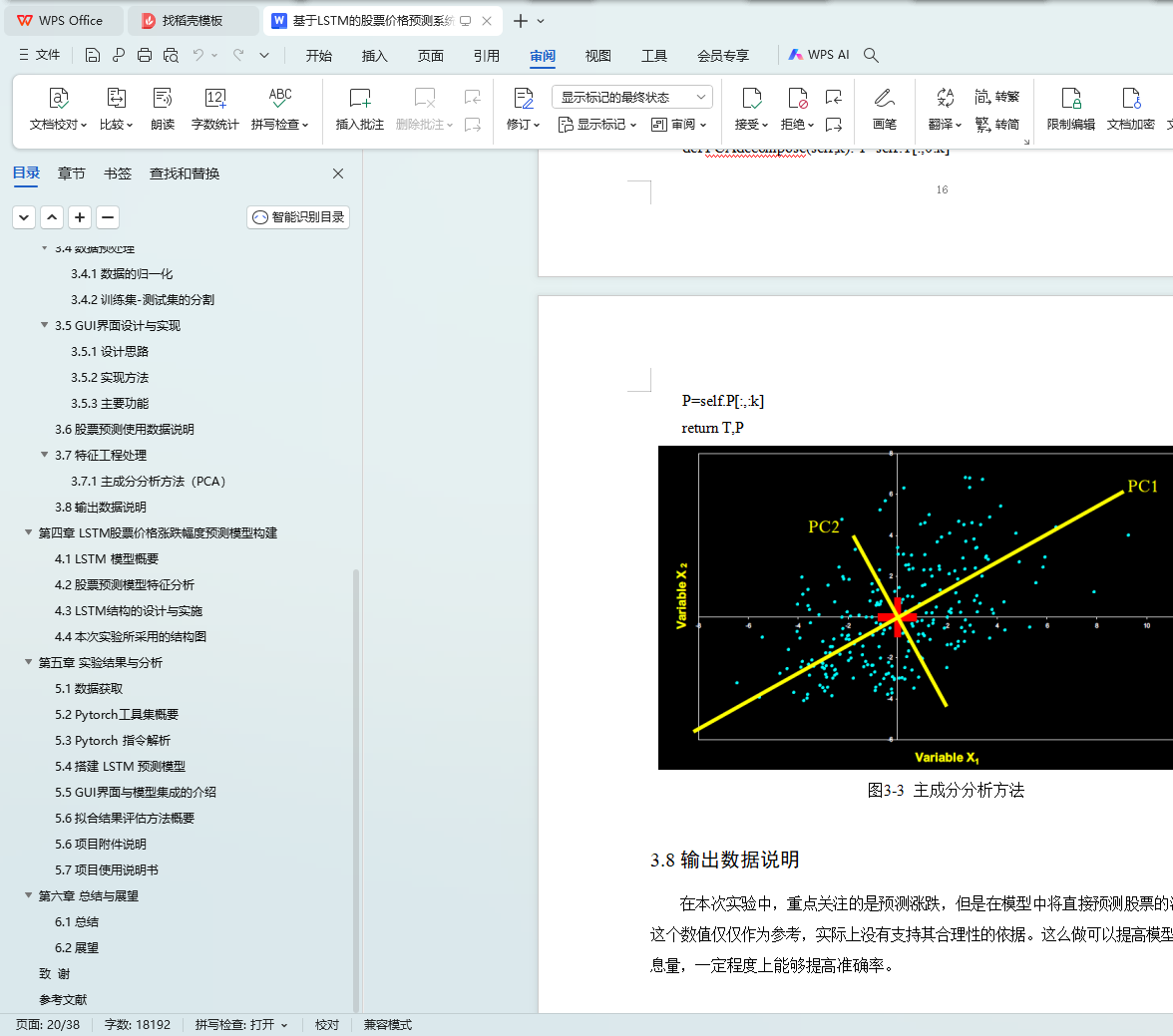
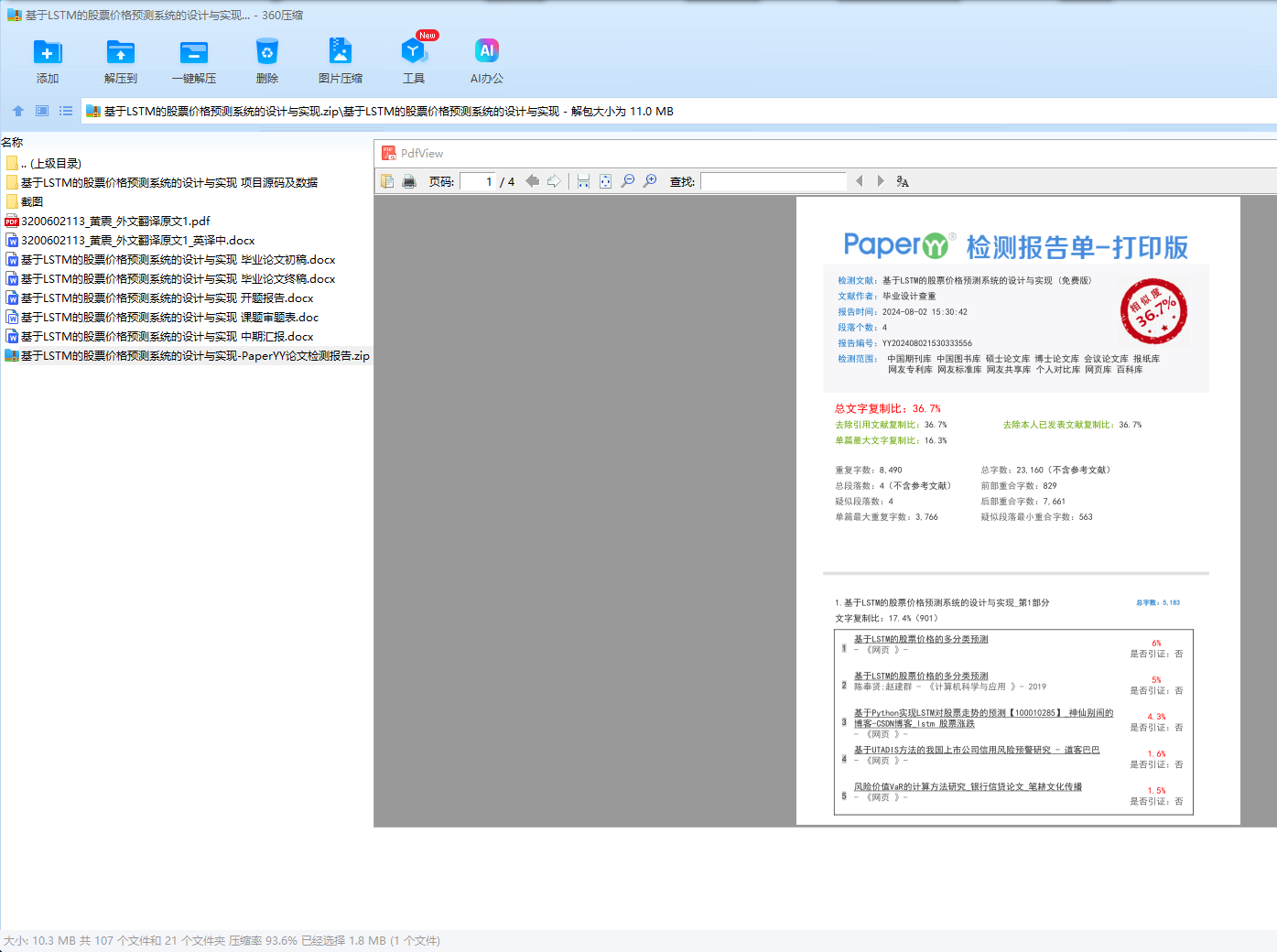
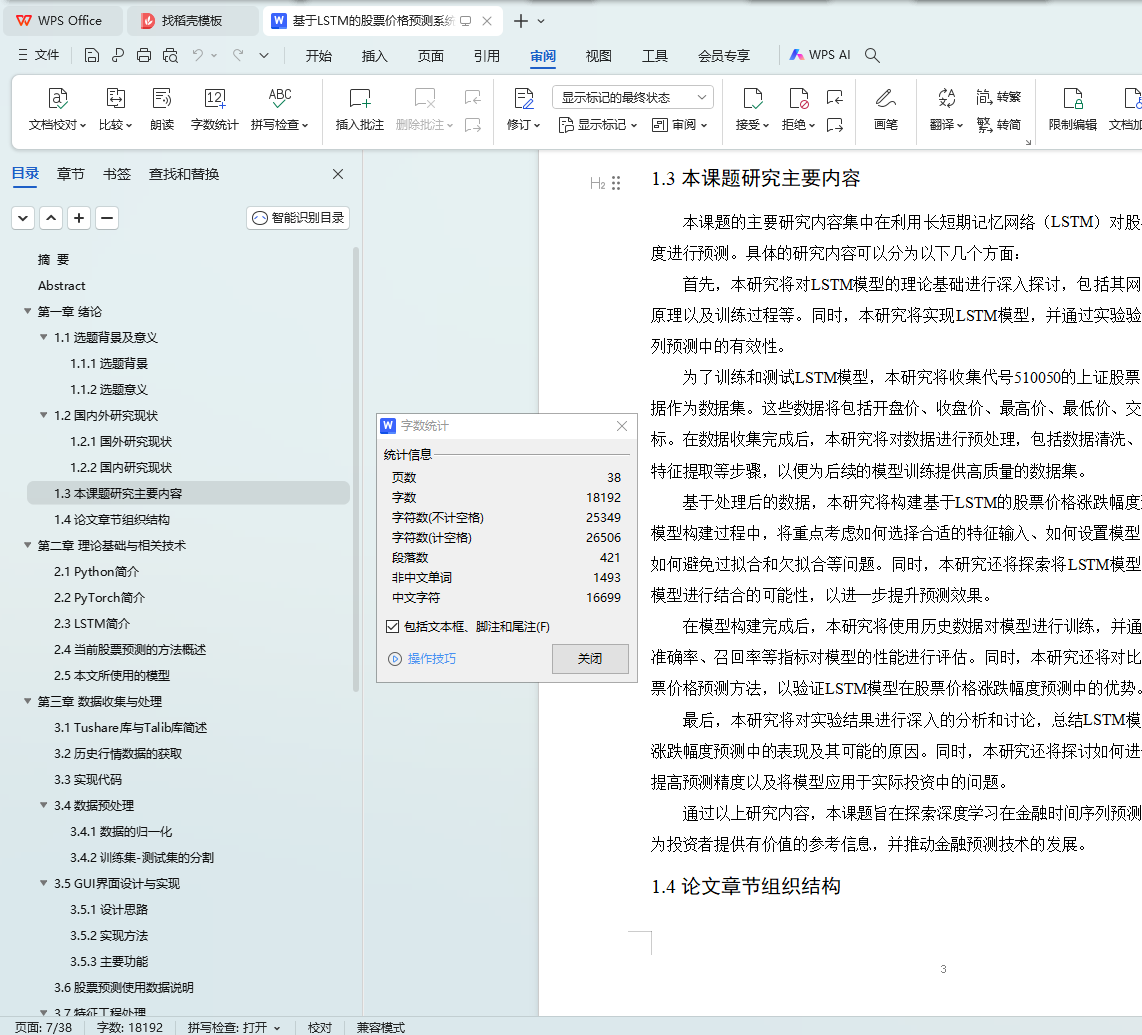
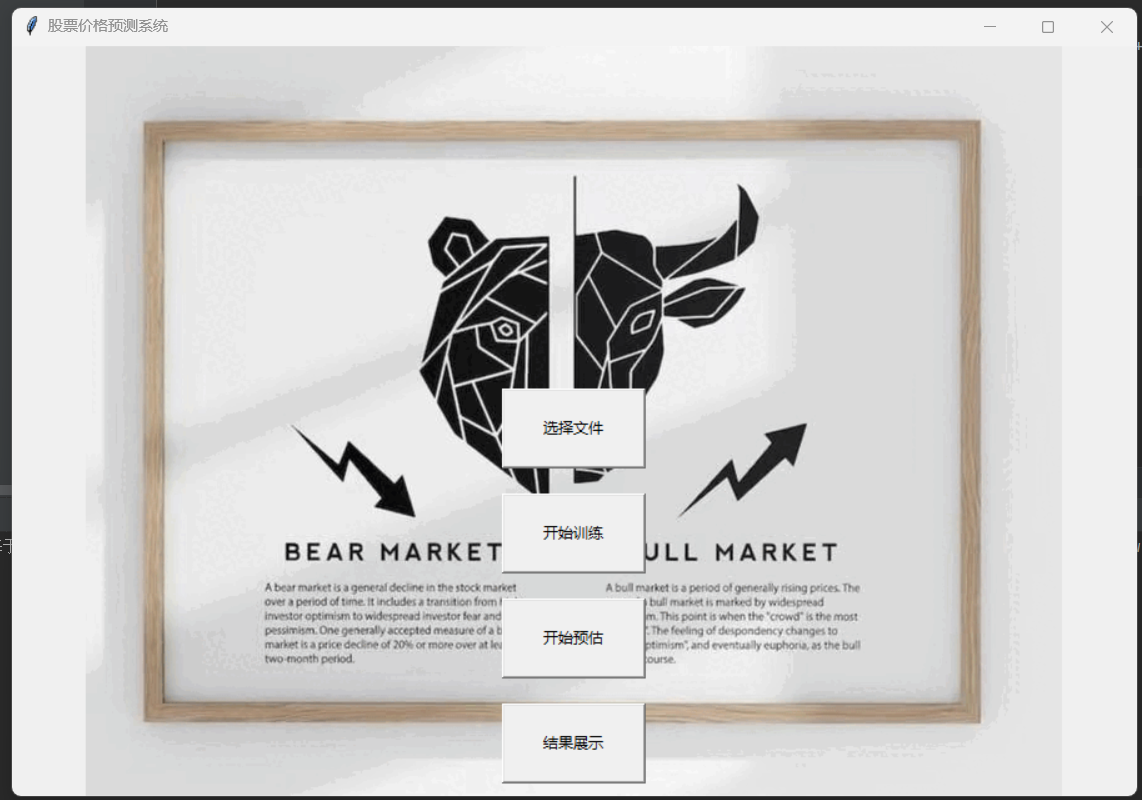
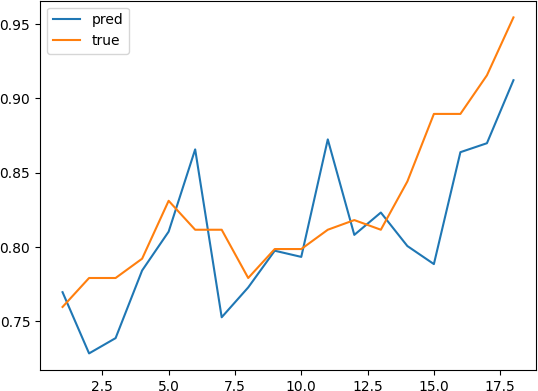
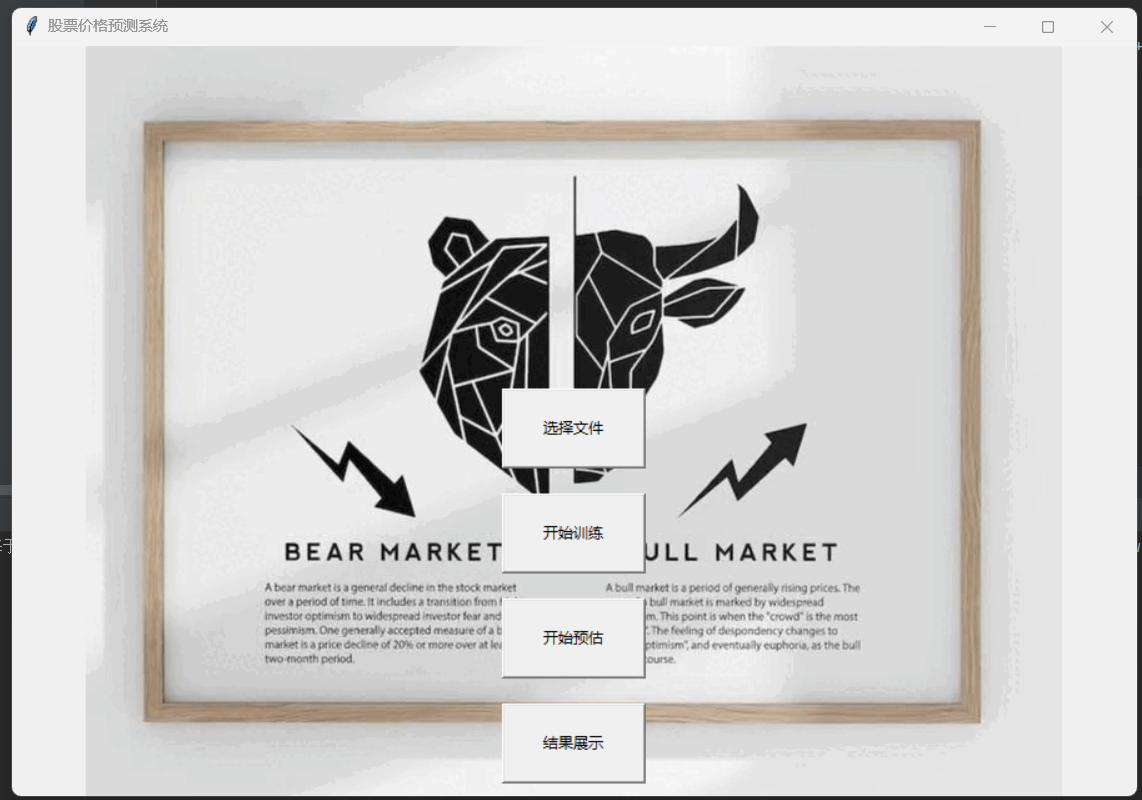
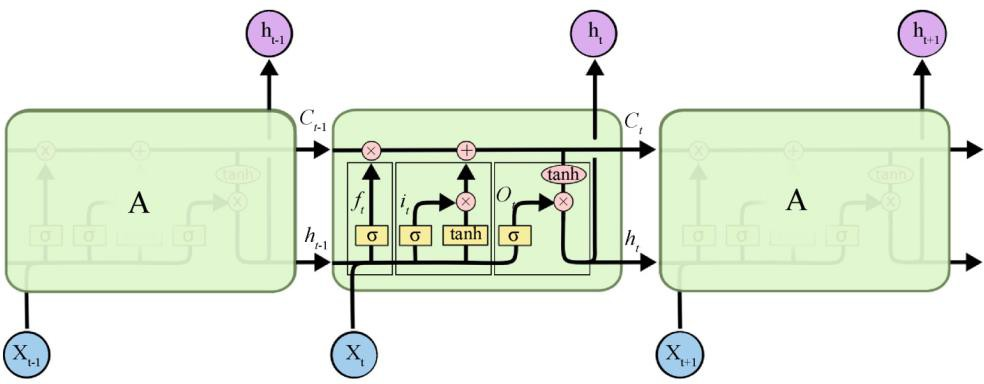
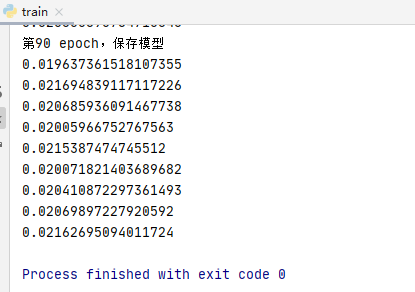
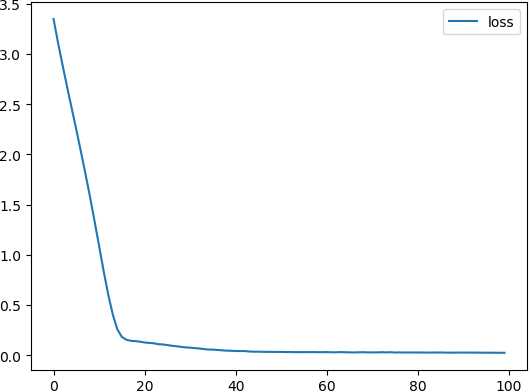
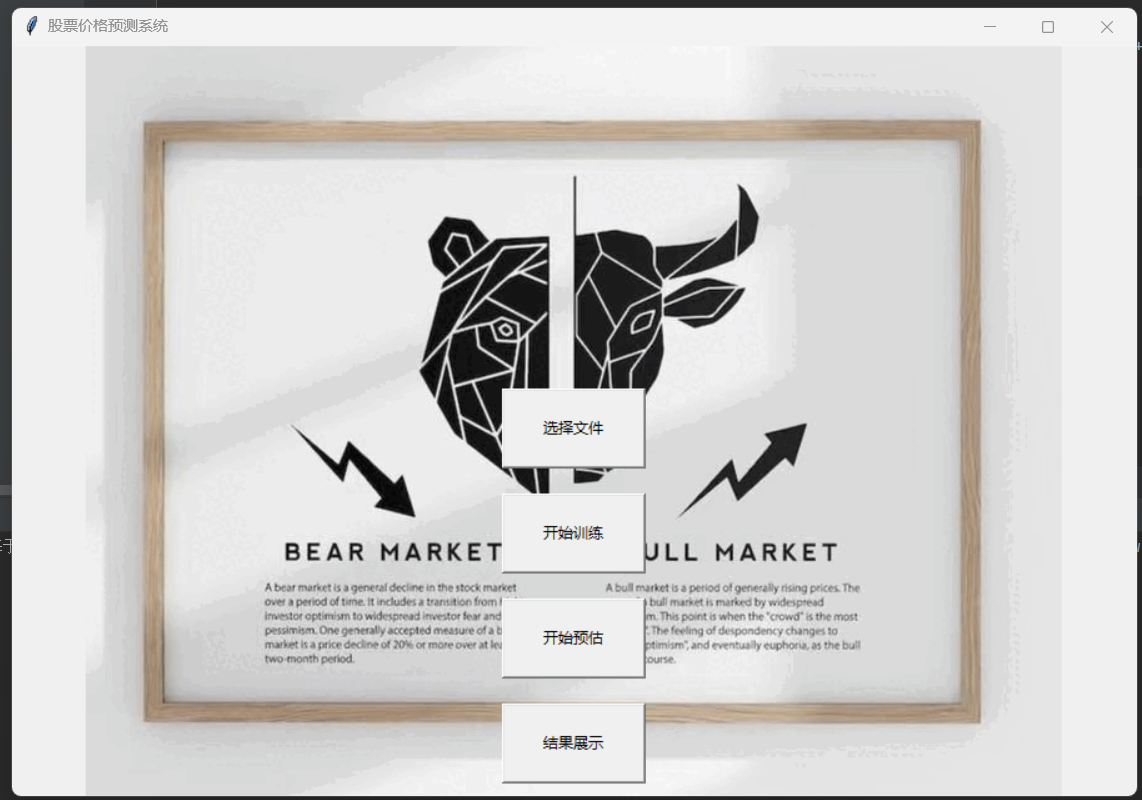
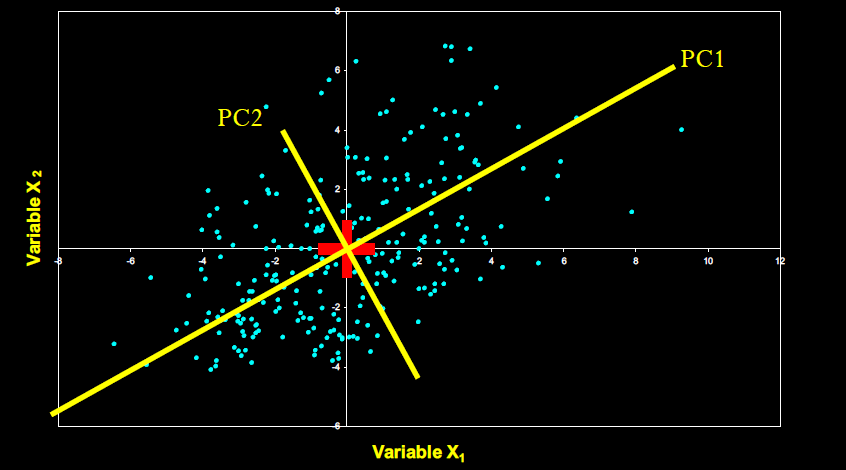
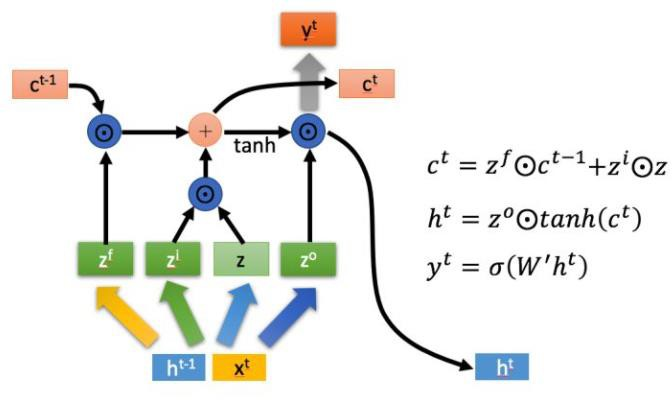
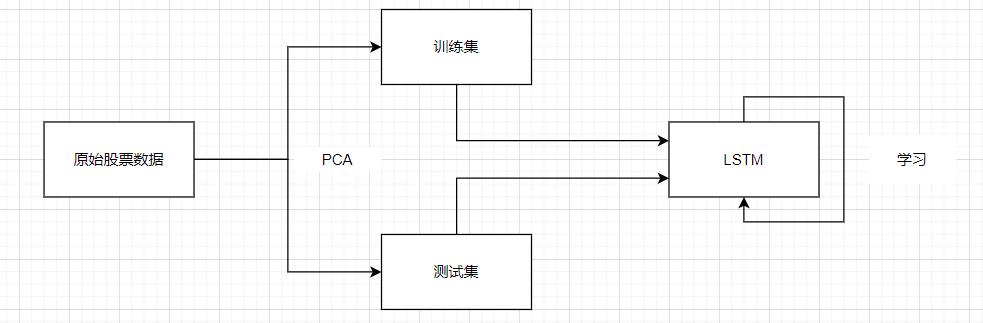
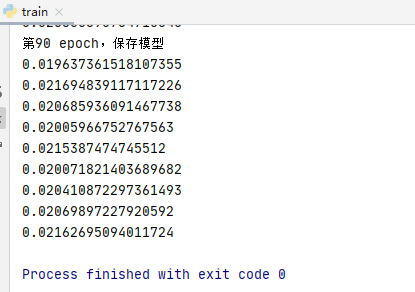
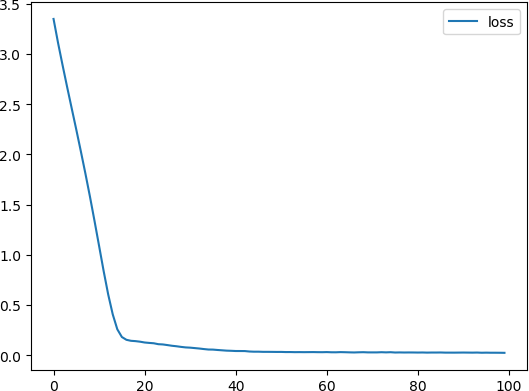
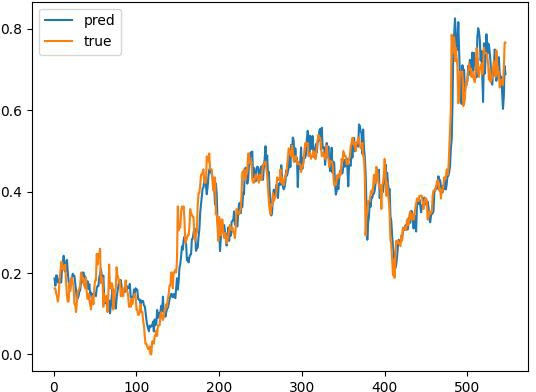