摘 要
遥感影像变化检测是指根据同一物体或现象在不同时间的观测来确定其不同的处理过程。其研究的目的是找出感兴趣的变化信息,滤除作为干扰因素的不相干的变化信息。变化检测的结果可以用于国土资源利用、土地覆盖类型监测、农作物提取和获取作物播种面积,高效获取准确、客观的土地利用情况,监测国土变化情况。随着遥感、传感器技术的发展,特别是多时相高分辨率遥感图像数据的普及,使我国遥感领域已步入了高分辨率影像的快车道,对遥感数据的分析应用服务的需求也与日俱增。传统方式对高分辨率卫星遥感图像的对特征刻画能力差且依赖人工经验工作量巨大。随着人工智能技术的兴起,特别是基于深度学习的图像识别方法获得了极大的发展,相关技术也推动了遥感领域的变革。相对于传统基于人海战术的目视解译方法,基于深度学习的遥感影像识别技术可以自动分析图像中的地物类型,在准确率和效率方面展现出极大的潜力。
本文通过对BIT模型进行改进,并应用于求解遥感影像变化检测任务。主要的工作包括:对原数据集进行数据增强以提高训练模型的泛化能力;针对样本不平衡问题对样本进行重采样;在计算交叉熵损失的时候加入权重因子,使模型更多的关注变化样本以及难区分样本的损失;使用正交试验将不同的超参数进行组合,并将得到的结果进行极差分析,确定对模型影响最大的因素和最佳的超参数组合方案;在原来BIT的骨干网络中加入空洞卷积池化金字塔模块(ASPP)用以提取多尺度图像特征,改善模型对多尺度目标的漏检和边界粗糙问题;同时,在ResBlock中加入双注意力机制(CBAM)用以提高模型的对变化像素的关注;最后,通过将AC-BIT模型与其他的模型进行对比分析,从实验结果中可以得出AC-BIT模型的综合效果要优于其他模型。
关键词:双时相遥感影像变化检测,深度学习,BIT,正交试验,注意力机制
Abstract
Remote sensing image change detection refers to determining the different processing processes of the same object or phenomenon based on the observation of the same object or phenomenon at different times. The purpose of its research is to find interesting change information and filter out irrelevant change information as interference factors. The results of change detection can be used to monitor the use of land resources and land cover types, efficiently obtain accurate and objective land use, and monitor land changes. With the development of remote sensing and sensor technology, especially the popularization of multi-temporal high-resolution remote sensing image data, my country's remote sensing field has stepped into the fast lane of high-resolution images, and the demand for remote sensing data analysis and application services is also increasing. Traditional methods have poor ability to characterize high-resolution satellite remote sensing images and rely heavily on manual experience. With the rise of artificial intelligence technology, especially image recognition methods based on deep learning have achieved great development, related technologies have also promoted changes in the field of remote sensing. Compared with the traditional visual interpretation method based on human sea tactics, the remote sensing image recognition technology based on deep learning can automatically analyze the types of objects in the image, showing great potential in terms of accuracy and efficiency.
This paper improves the BIT model and applies it to solve the task of remote sensing image change detection. The main work includes: enhancing the original data set to improve the generalization ability of the training model; resampling the samples for the problem of sample imbalance; adding weight factors when calculating the cross entropy loss, so that the model pays more attention to changes Loss of samples and indistinguishable samples; use orthogonal tests to combine different hyperparameters, and perform range analysis on the obtained results to determine the factors that have the greatest impact on the model and the best hyperparameter combination scheme; in the original BIT The Atrous Convolution Pooling Pyramid Module (ASPP) is added to the backbone network to extract multi-scale image features and improve the model's missed detection and rough boundary problems for multi-scale targets; at the same time, a dual attention mechanism (CBAM) is added to ResBlock In order to improve the model's attention to the changing pixels; finally, by comparing the AC-BIT model with other models, it can be concluded from the experimental results that the comprehensive effect of the AC-BIT model is better than other models.
Key words: Bi-temporal Remote Sensing Image Change Detection, Deep Learning, BIT, Orthogonal experimental design, Attention Mechanism
目 录
第一章 绪论
1.1问题背景及意义
1.2国内外研究现状
1.3论文主要研究内容
1.4论文组织结构
第二章 相关技术
2.1遥感图像变化检测
2.2 孪生网络
2.3 残差网络
2.4 注意力机制
第三章 基于BIT的遥感影像变化检测
3.1 Transformer模型
3.2 BIT模型
3.3 AC-BIT模型
第四章 实验及结果分析
4.1 实验环境
4.2 实验数据
4.3 网络配置
4.4 正交实验
4.5 对比测试
第五章 总结与展望
5.1 总结
5.2 展望
参考文献
致 谢
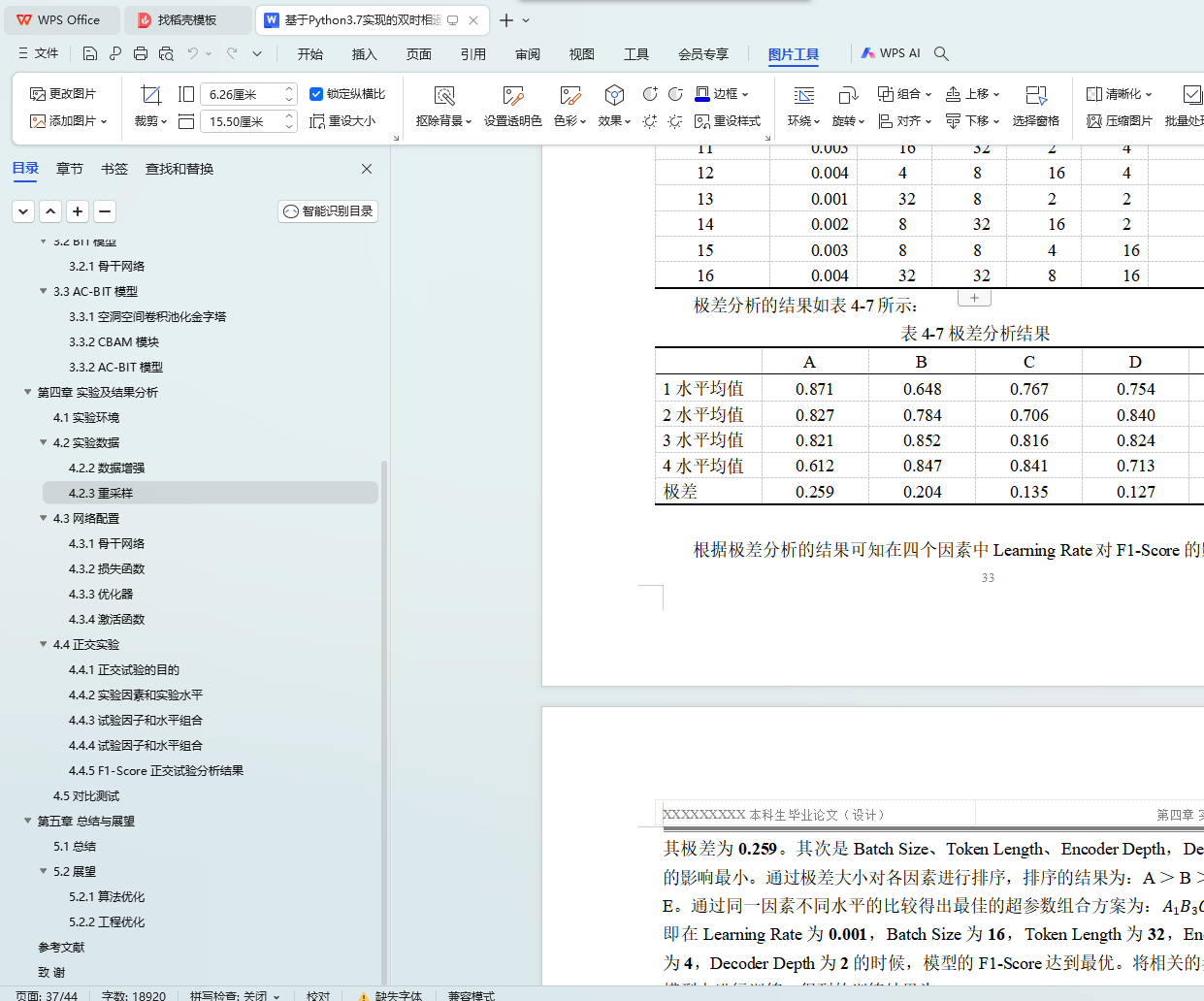
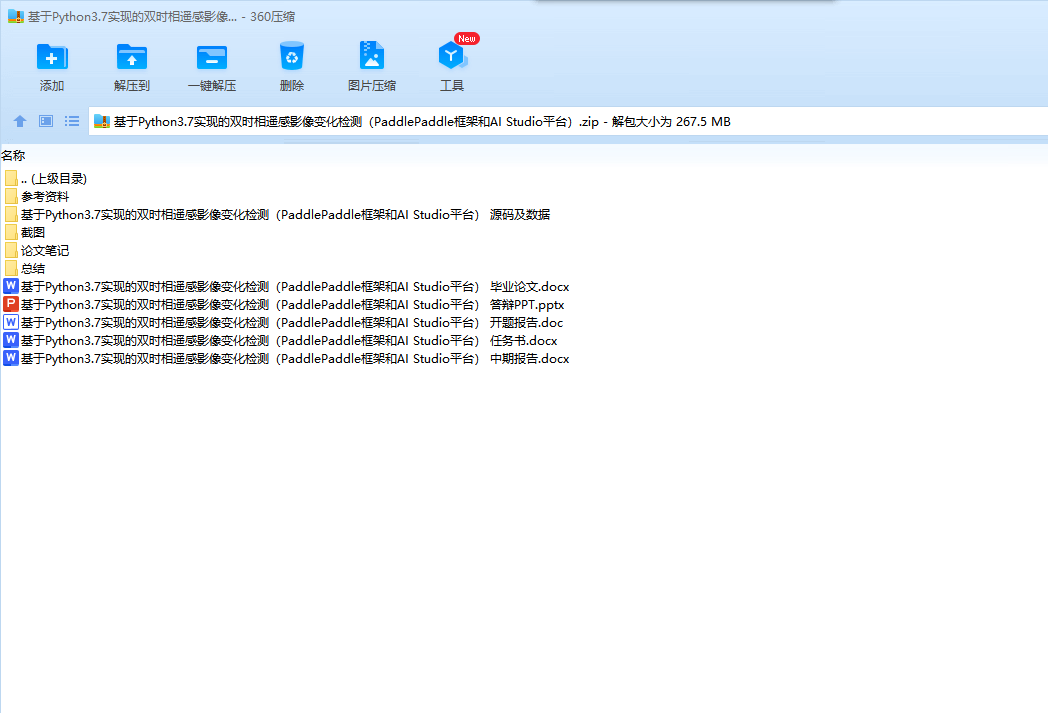
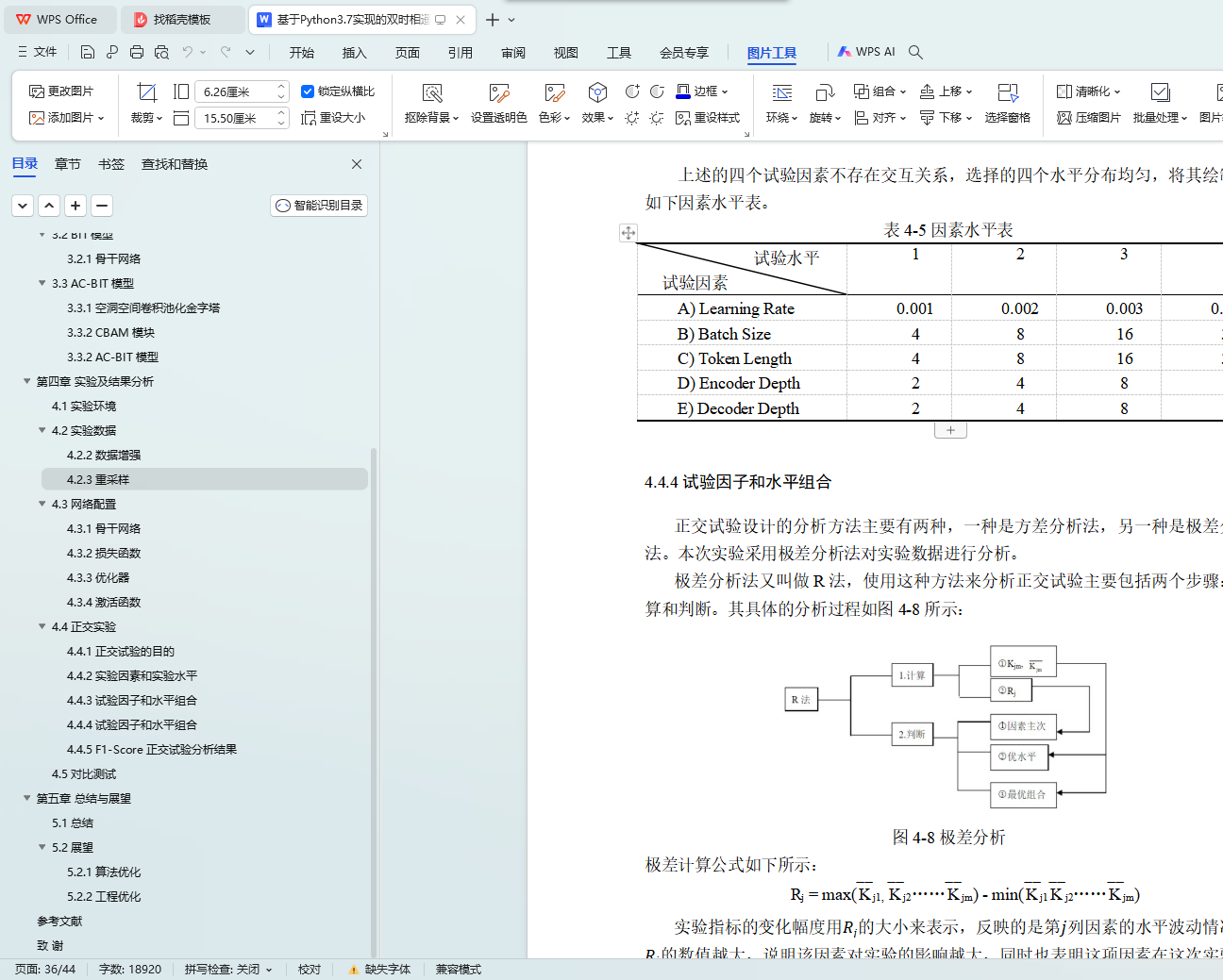
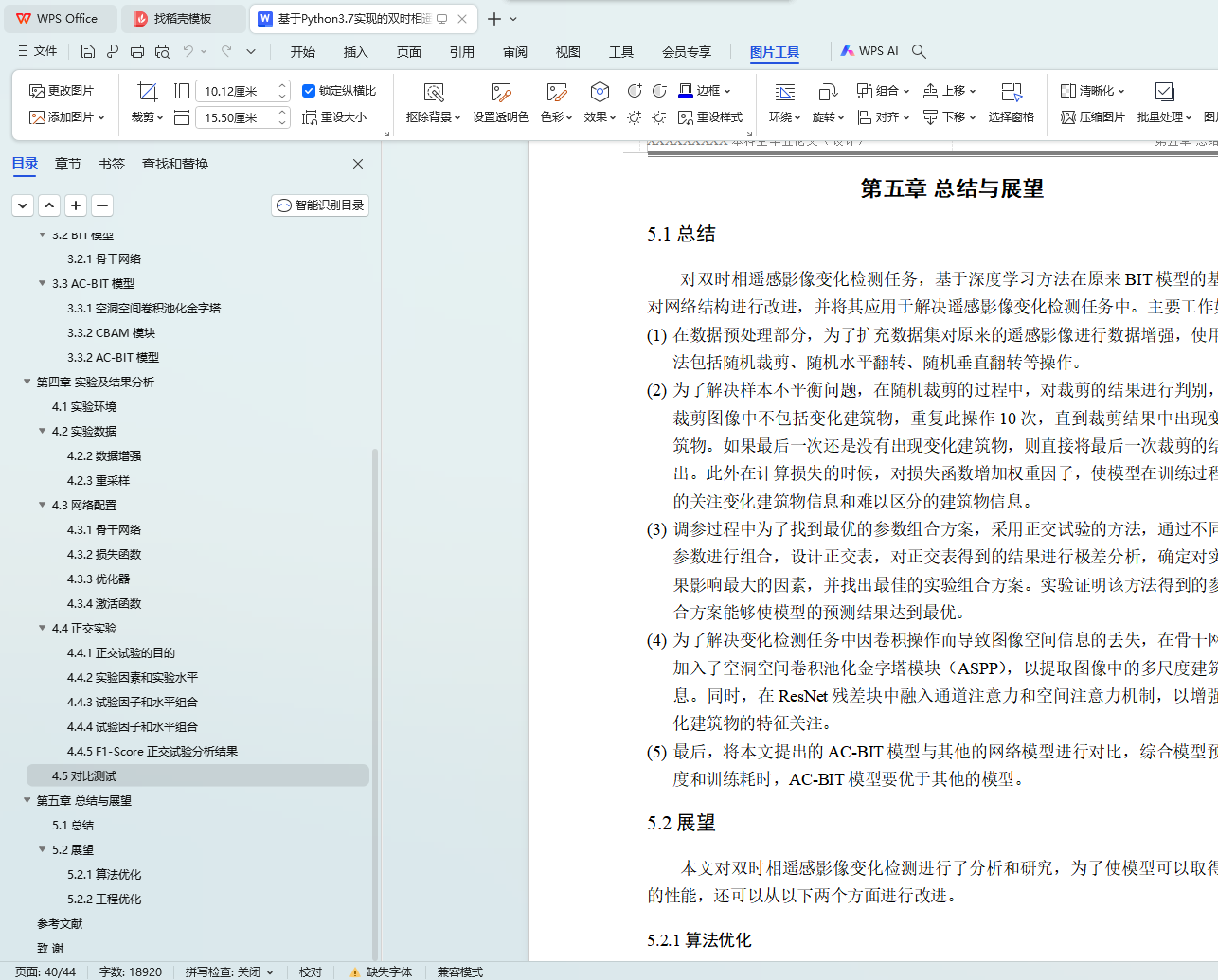
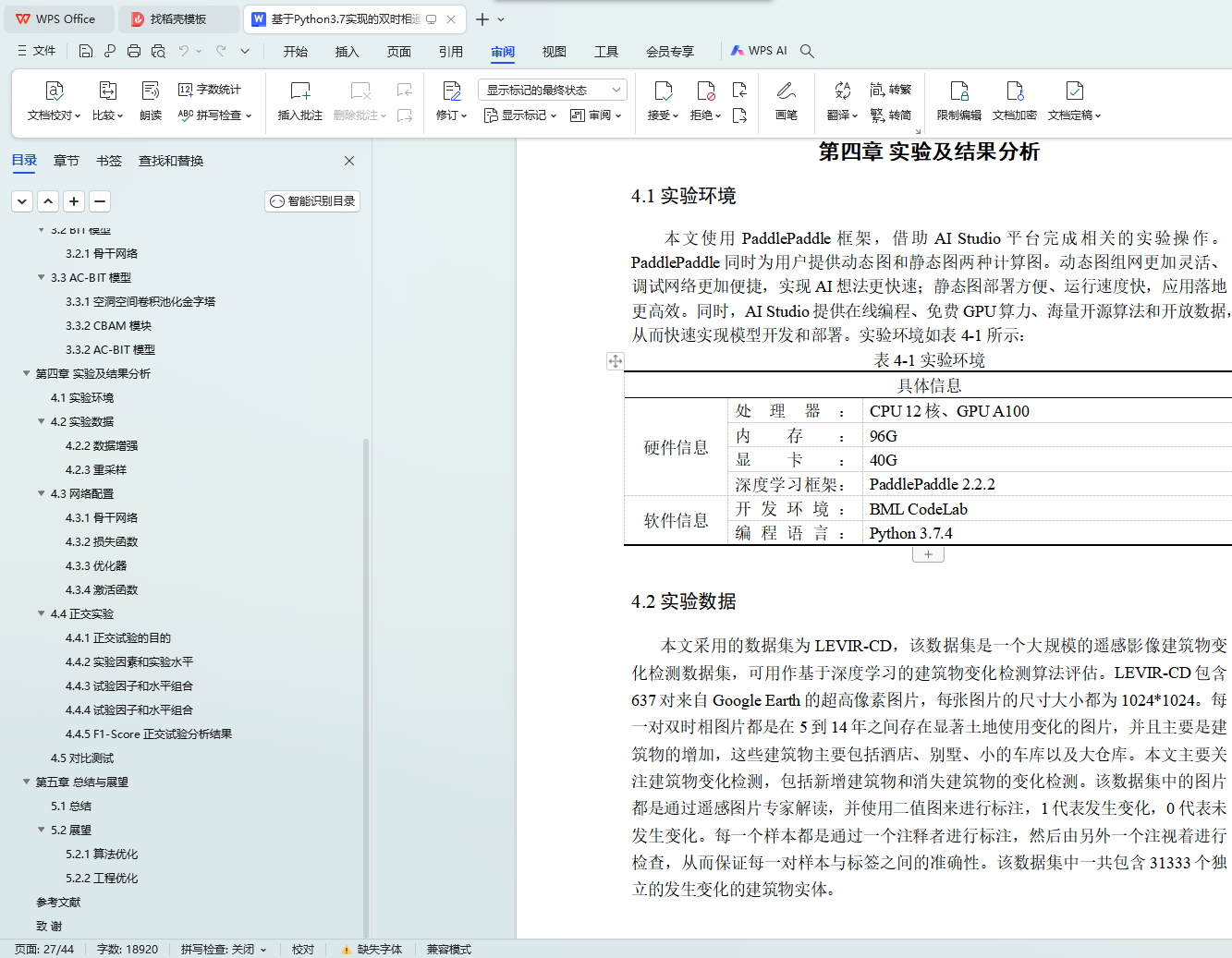
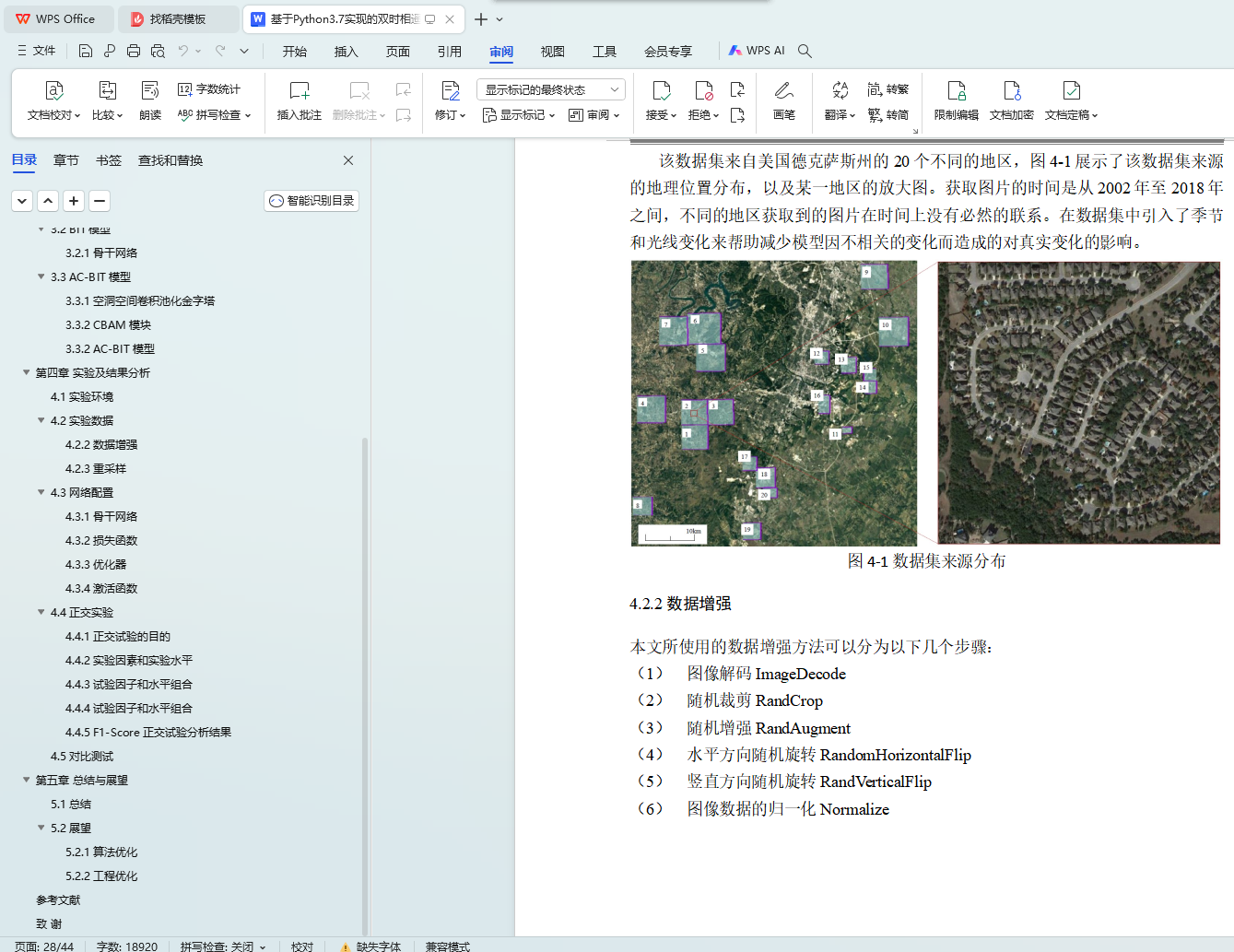
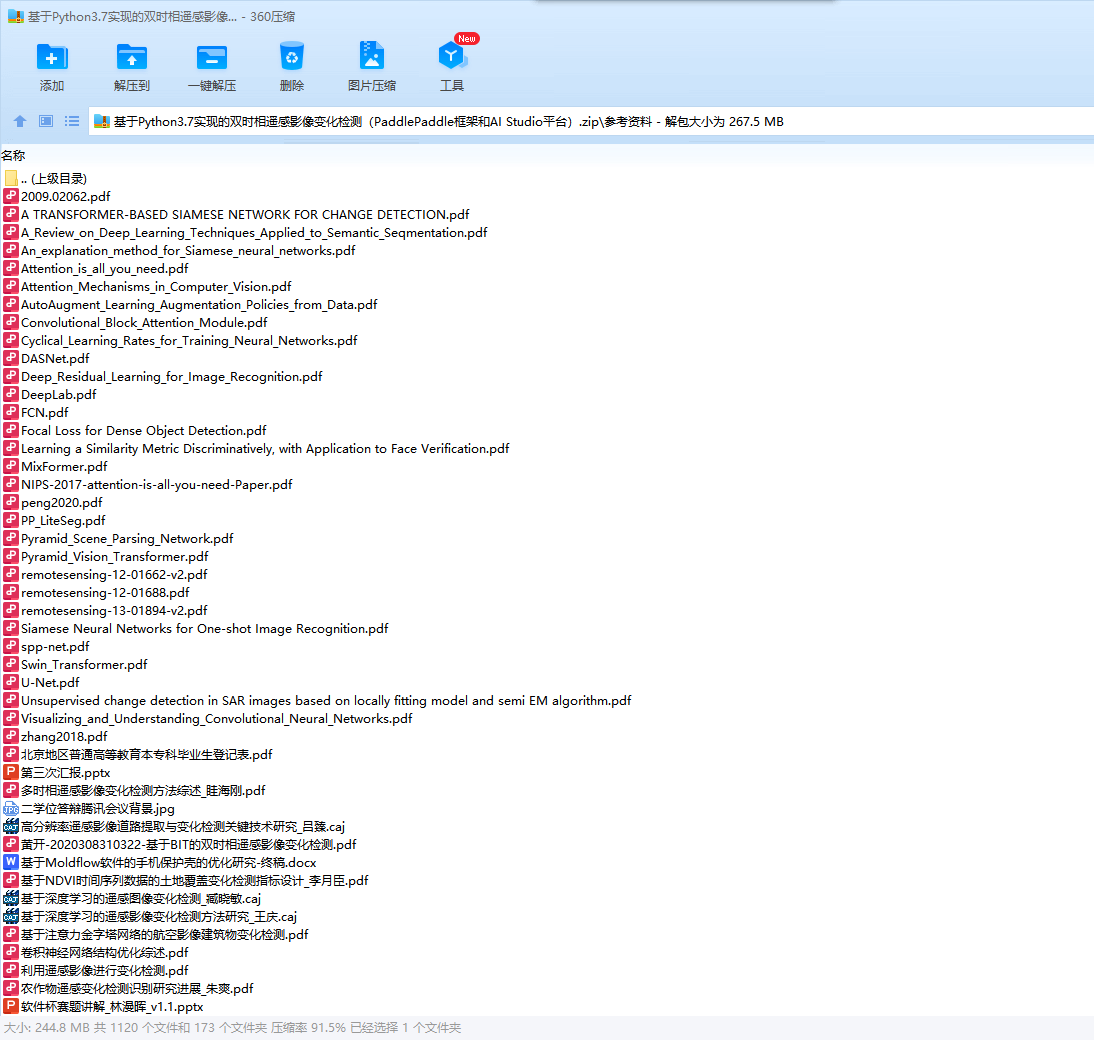
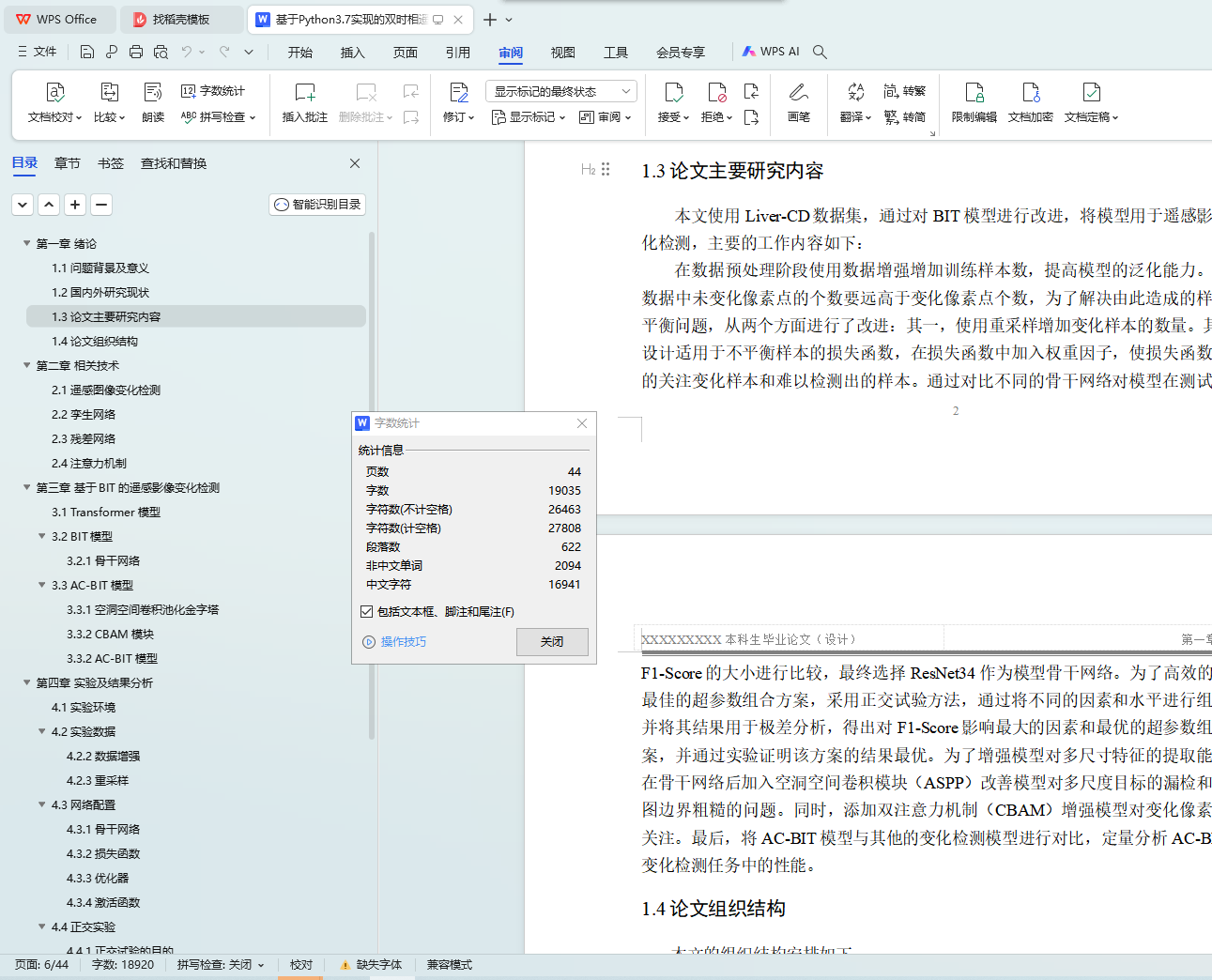
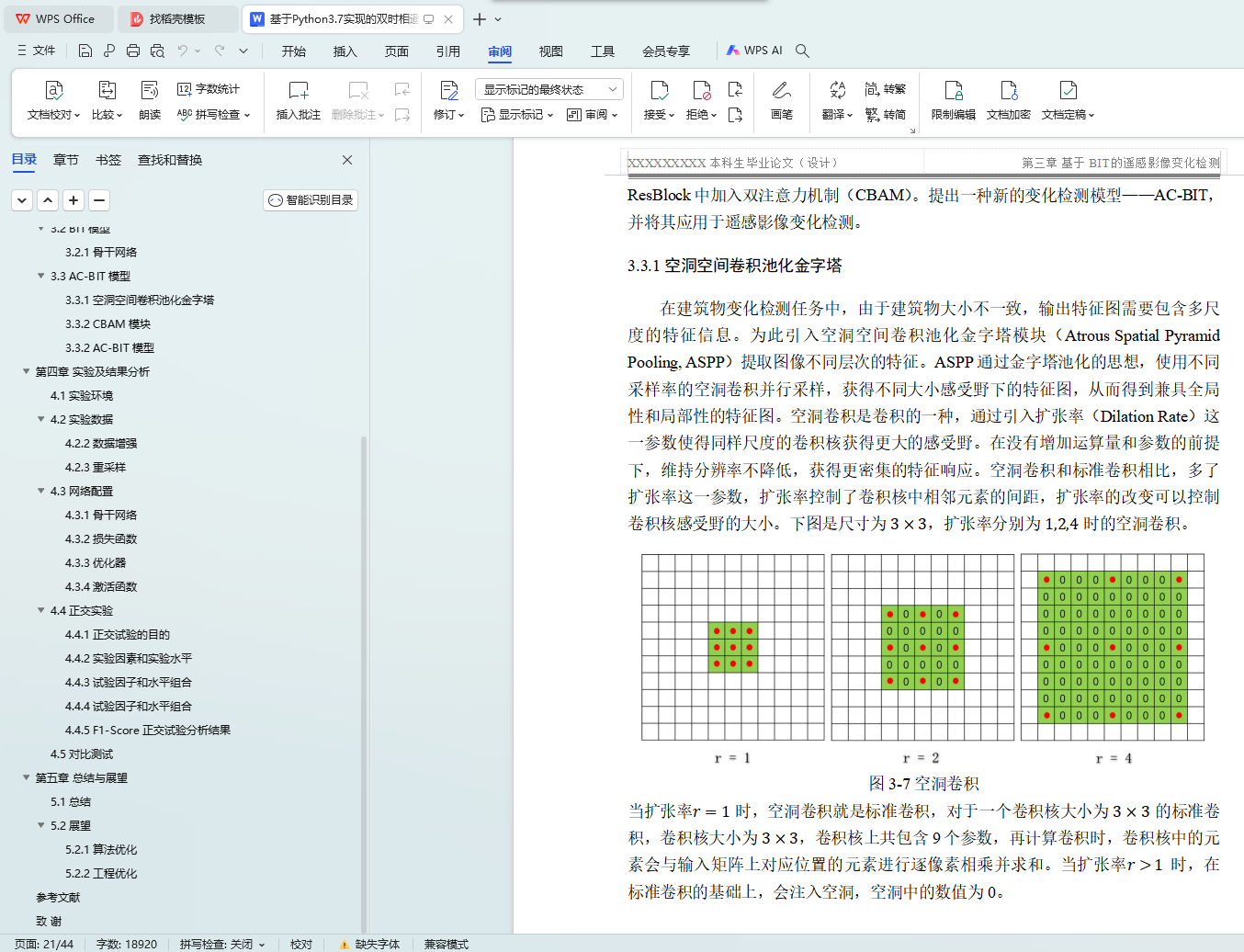
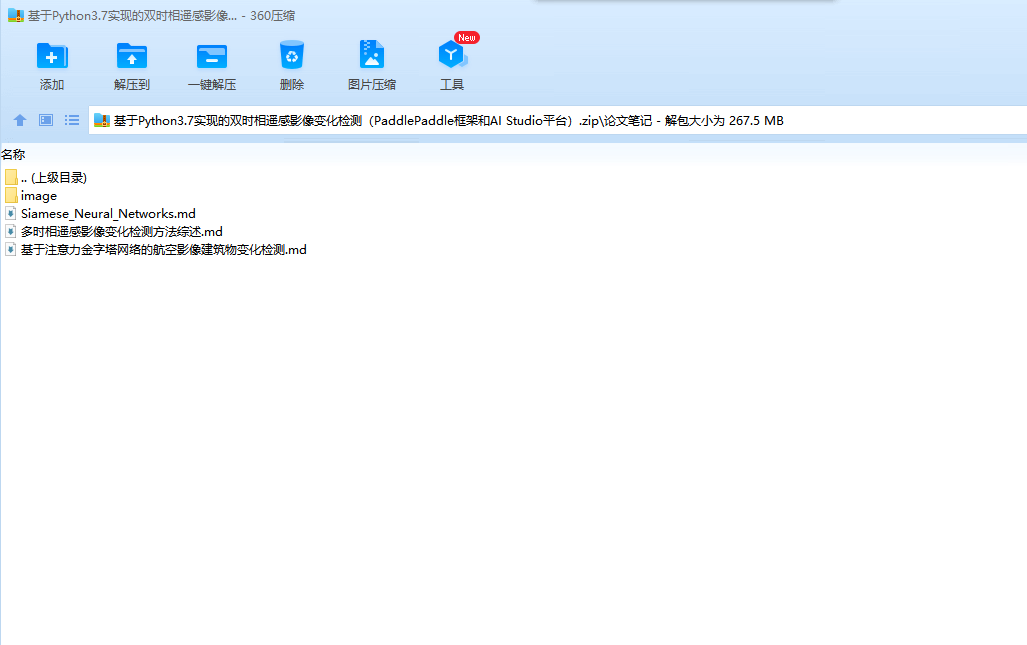
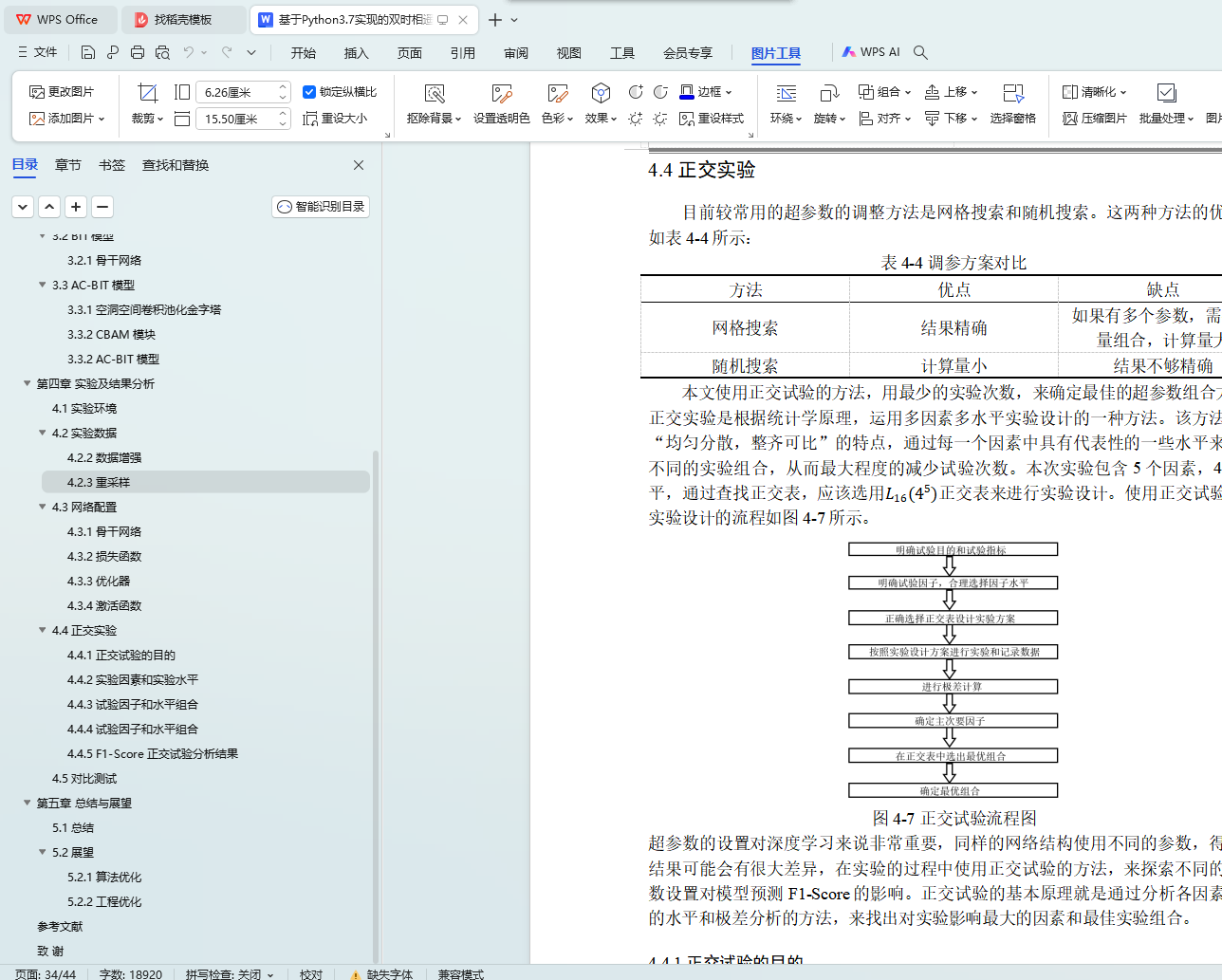
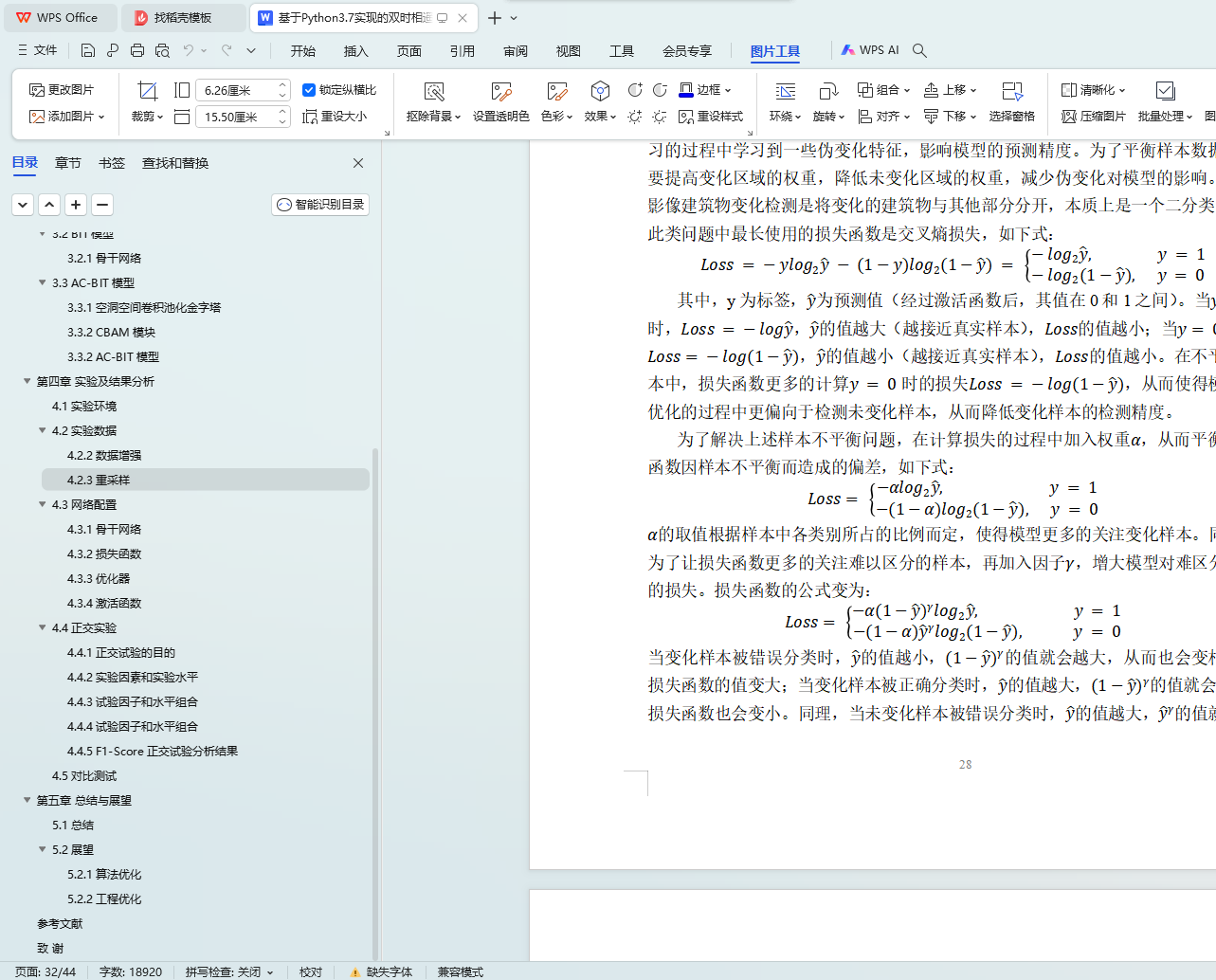
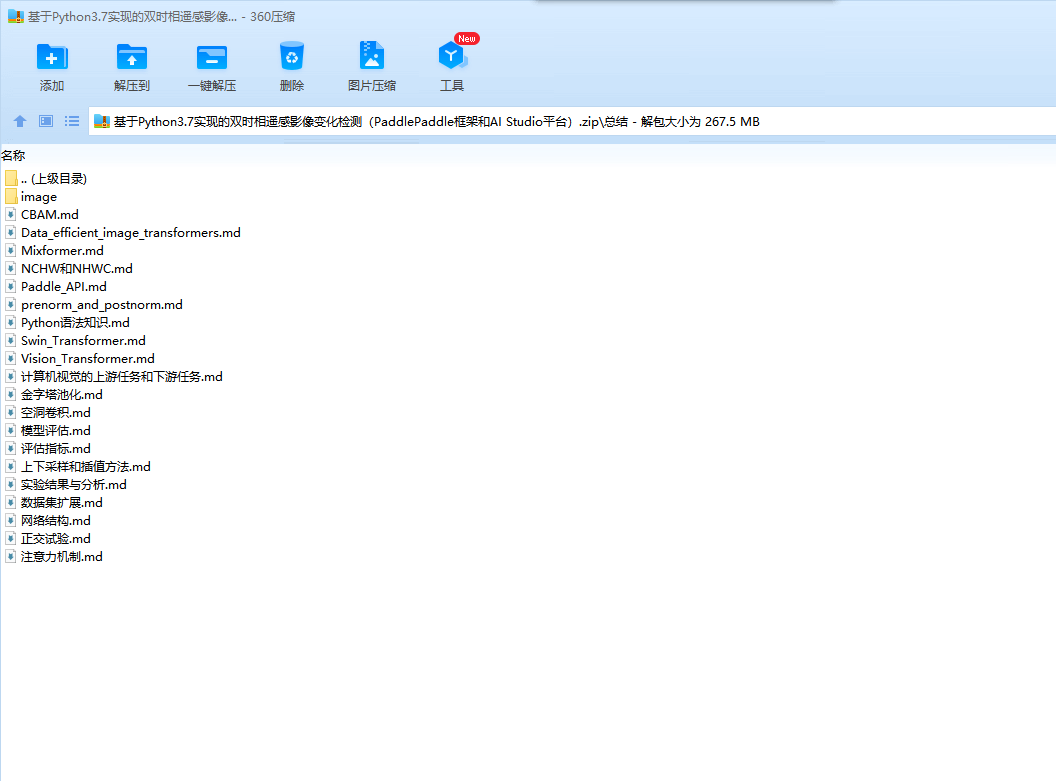
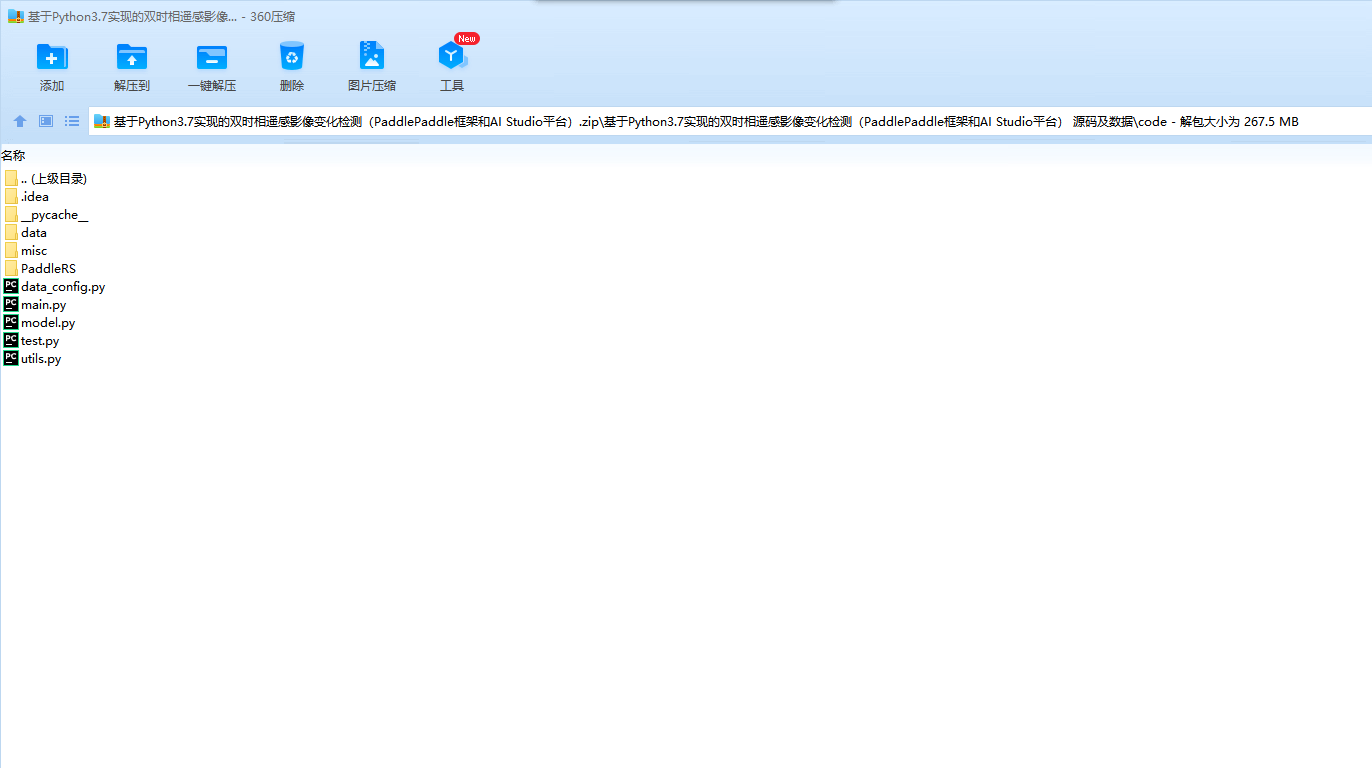
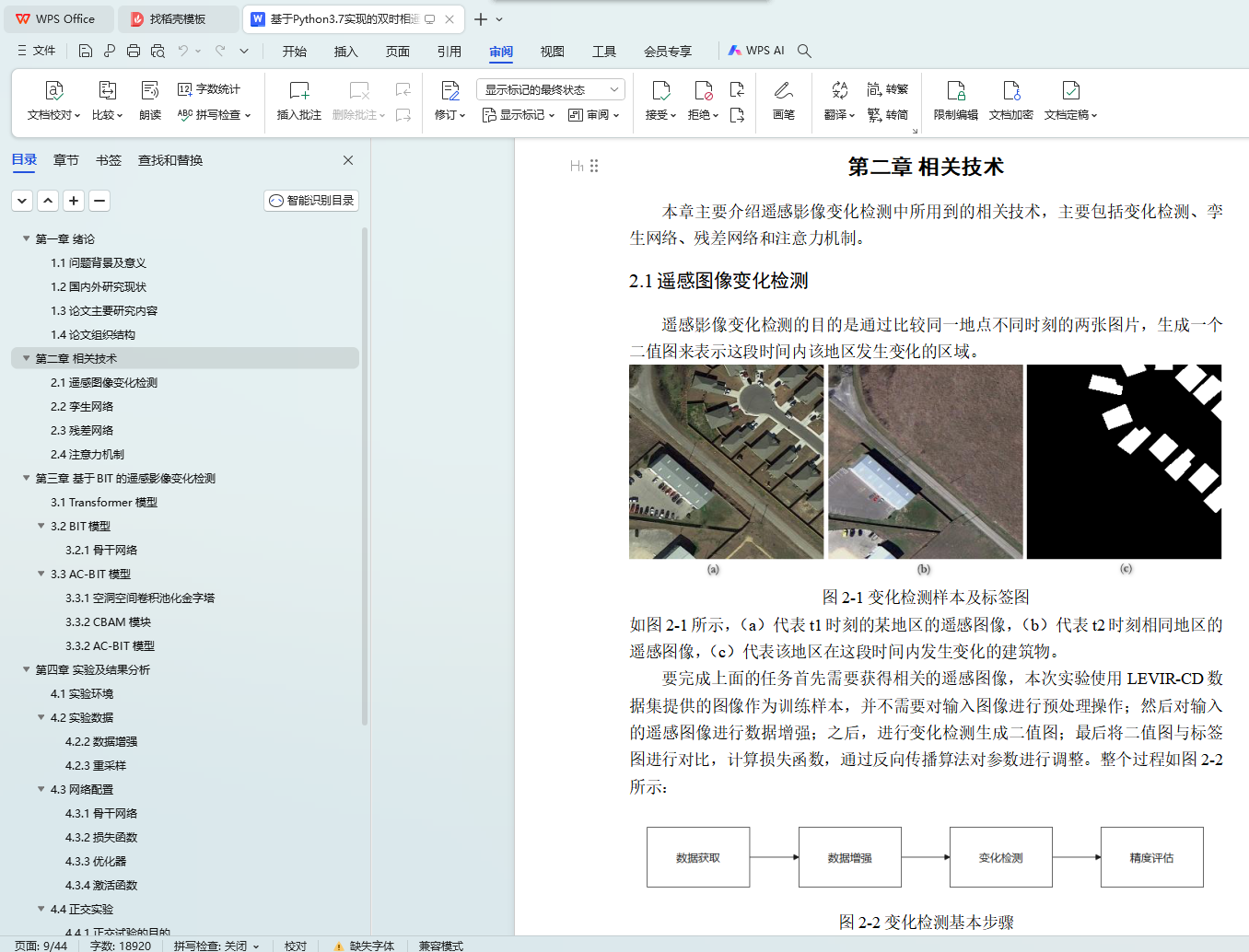
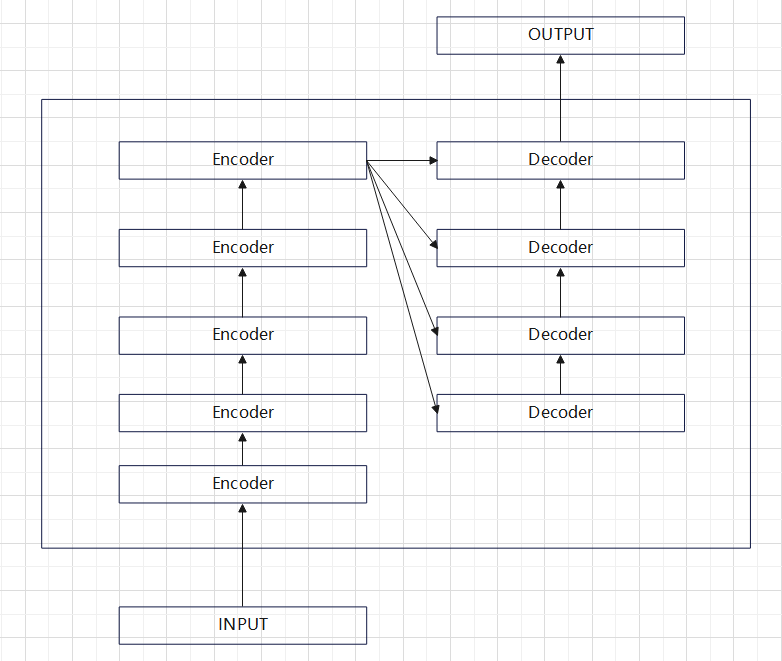
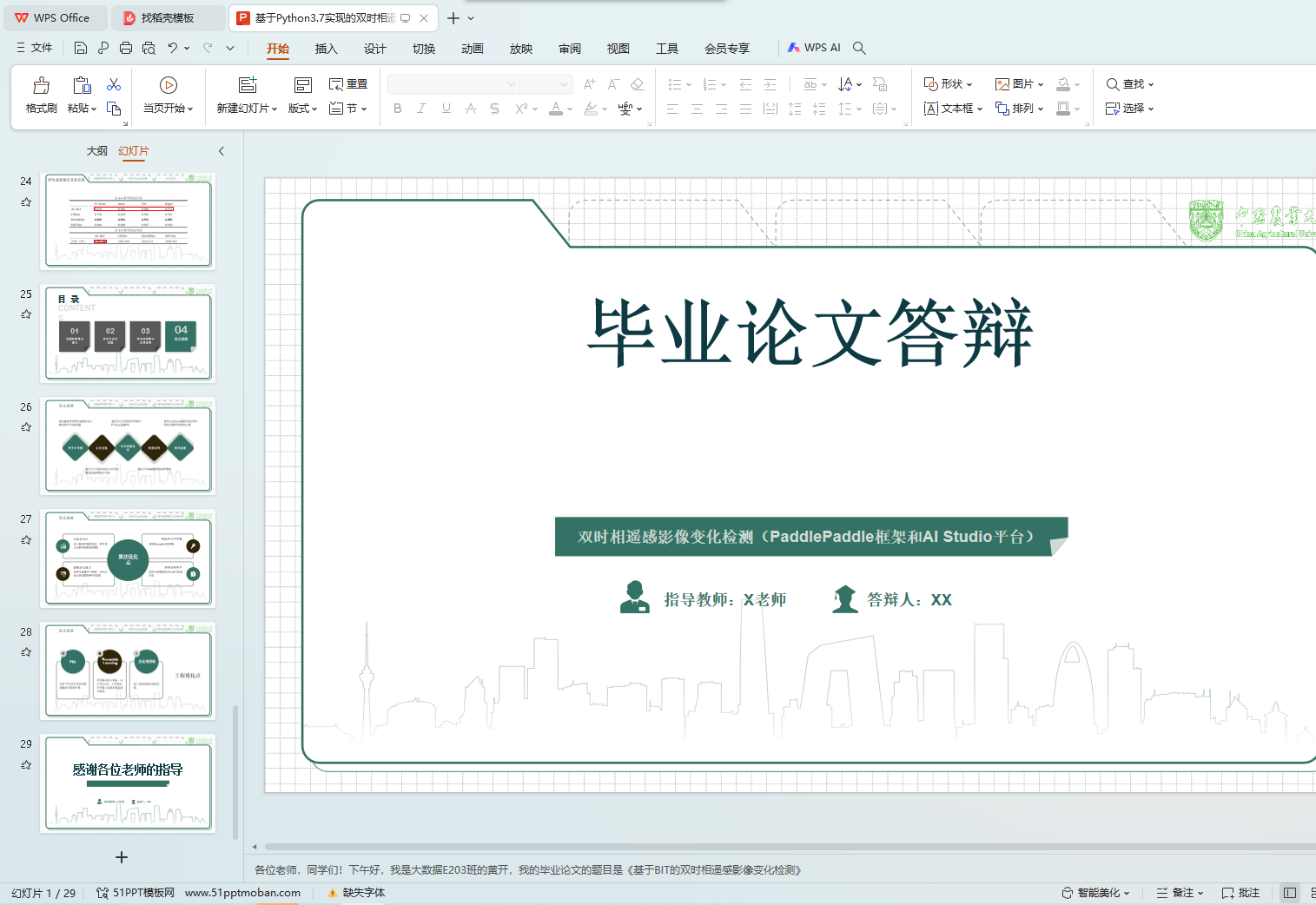
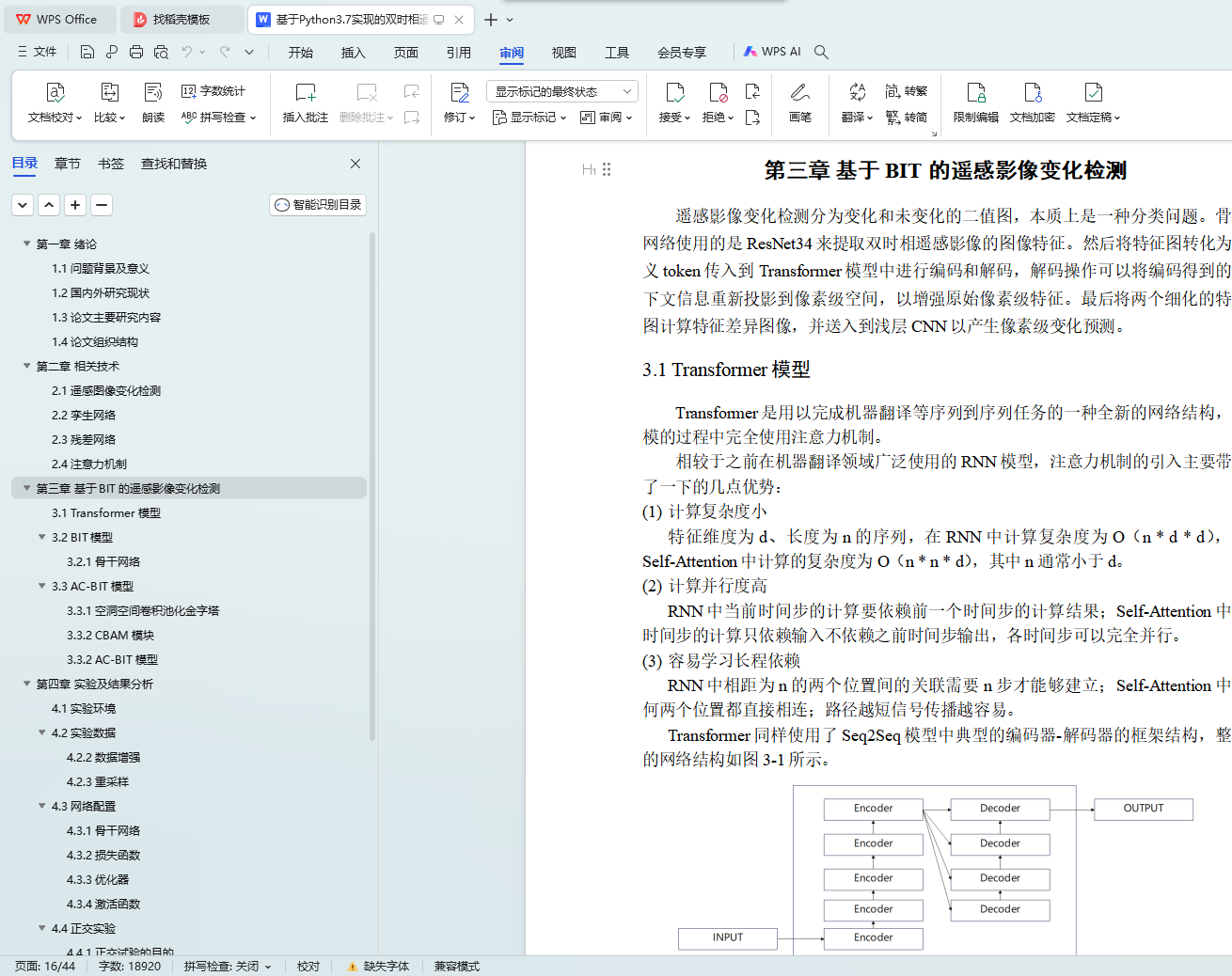
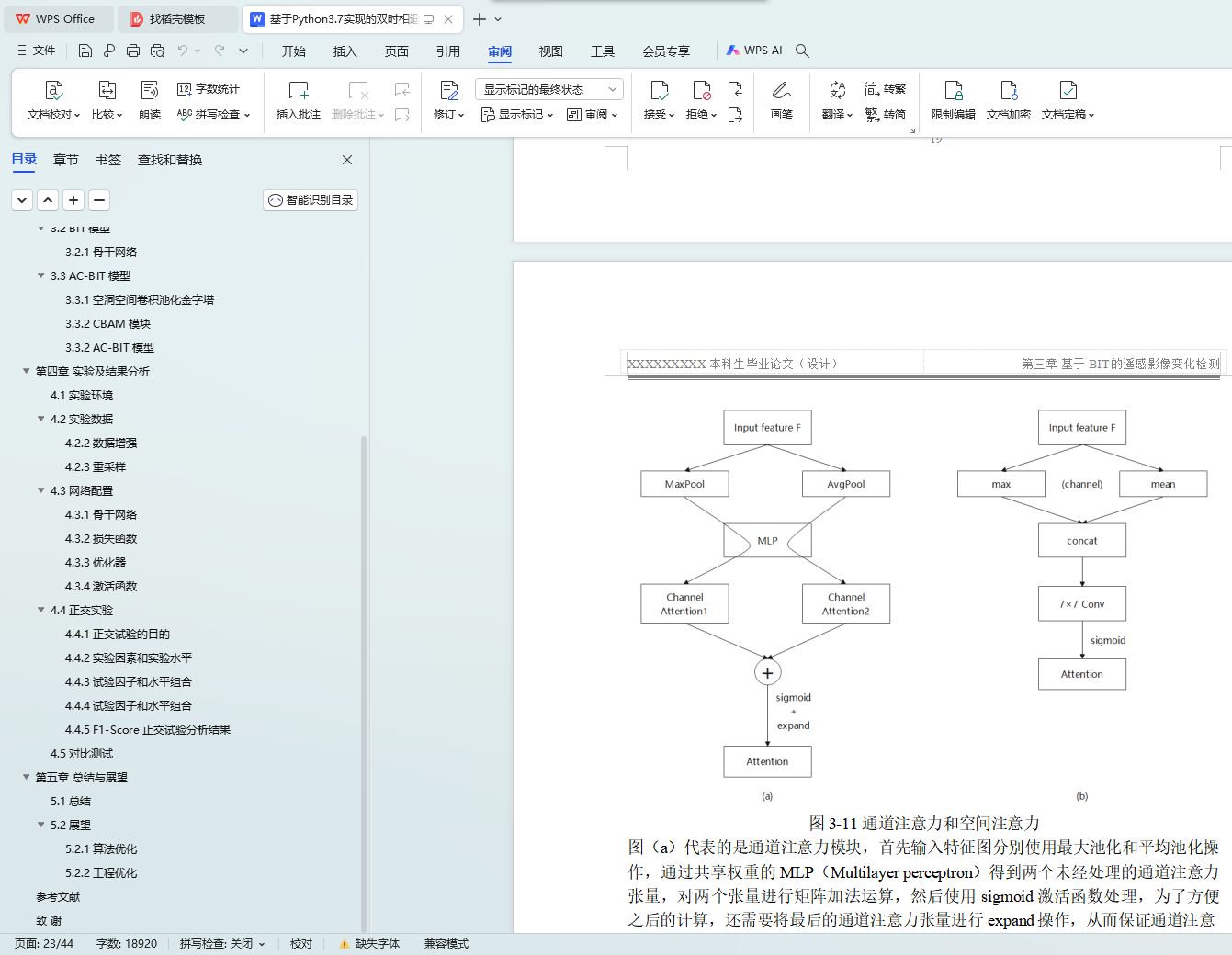
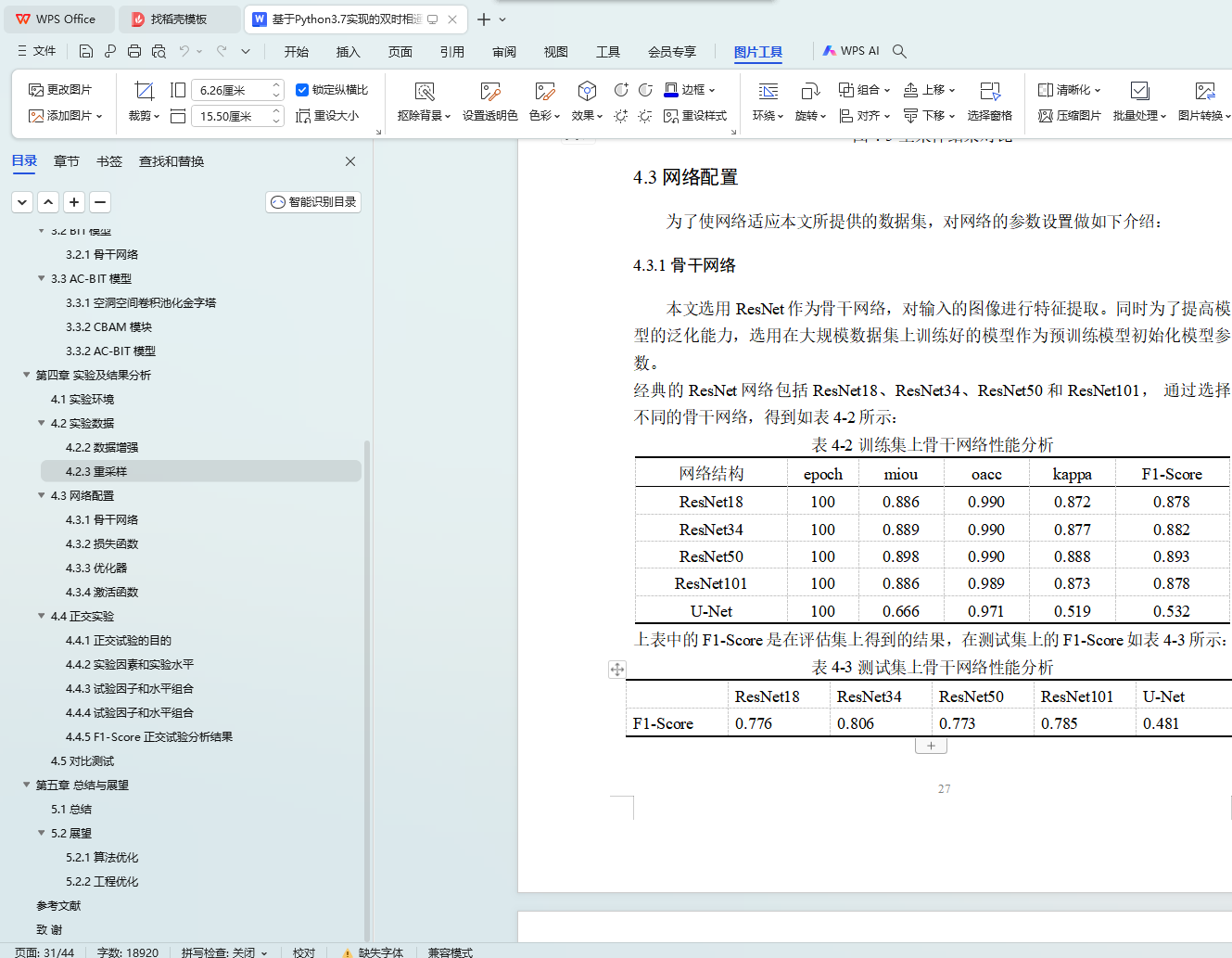
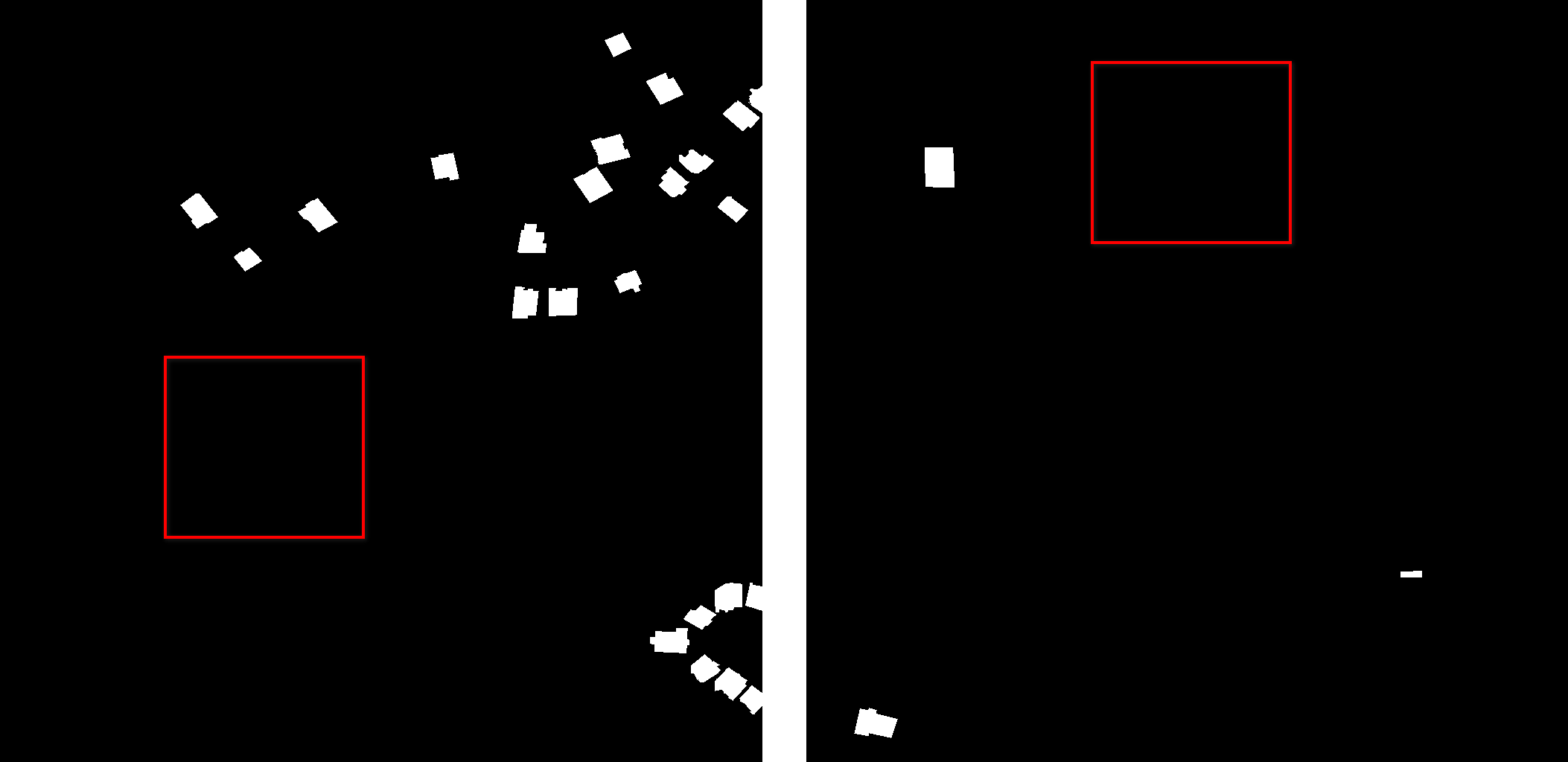
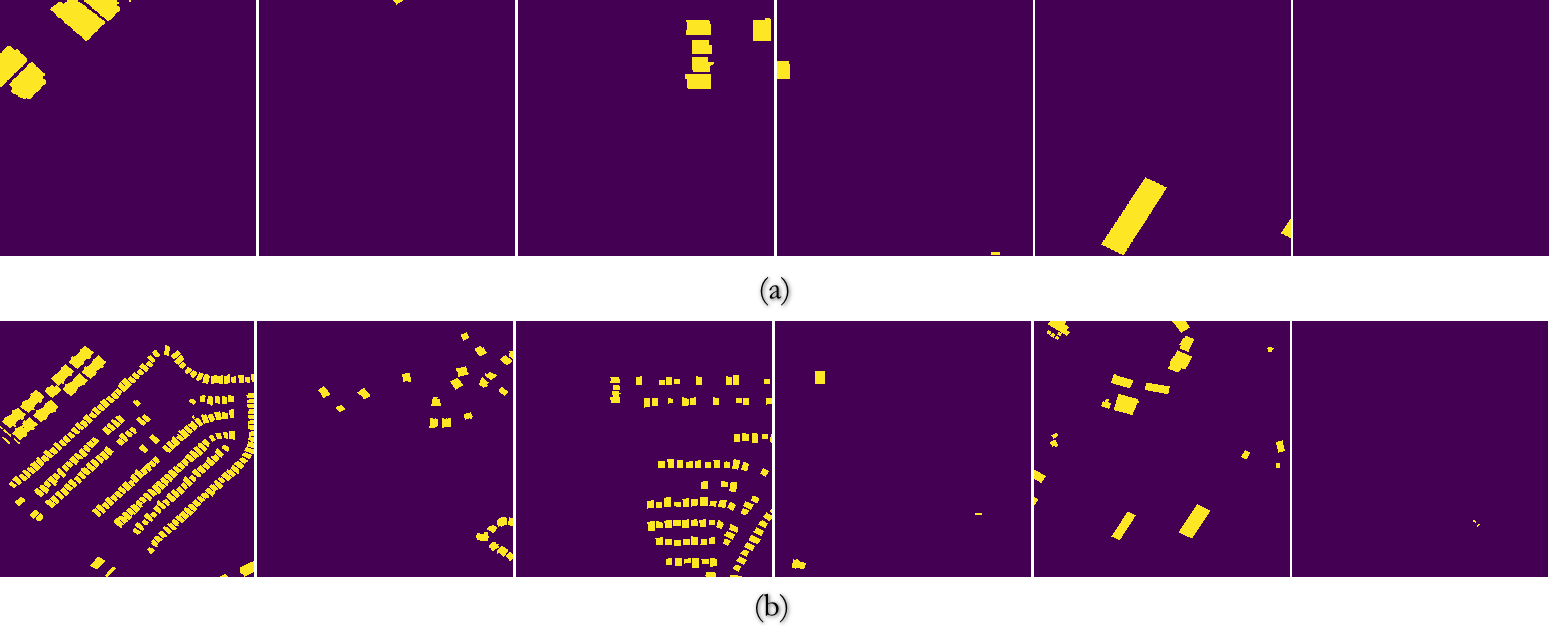
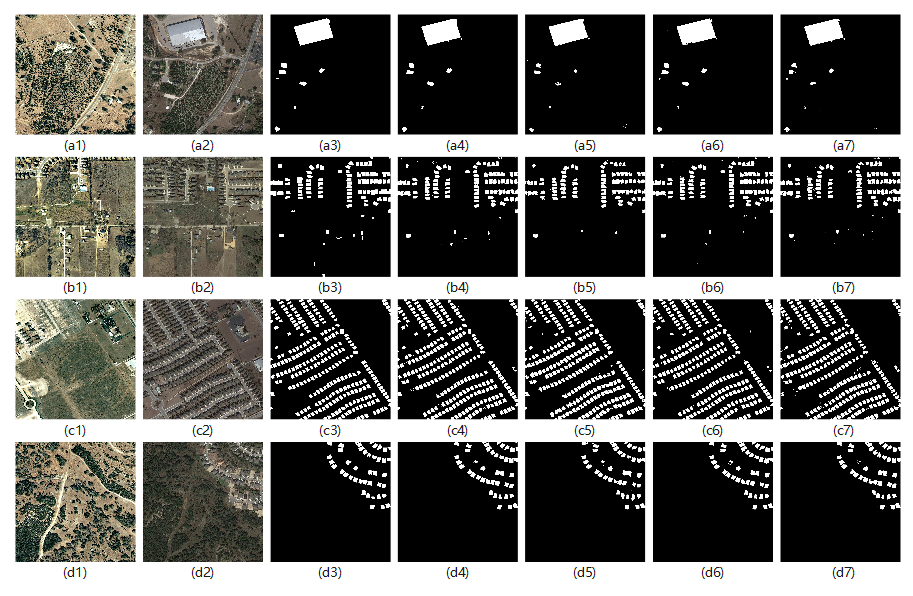
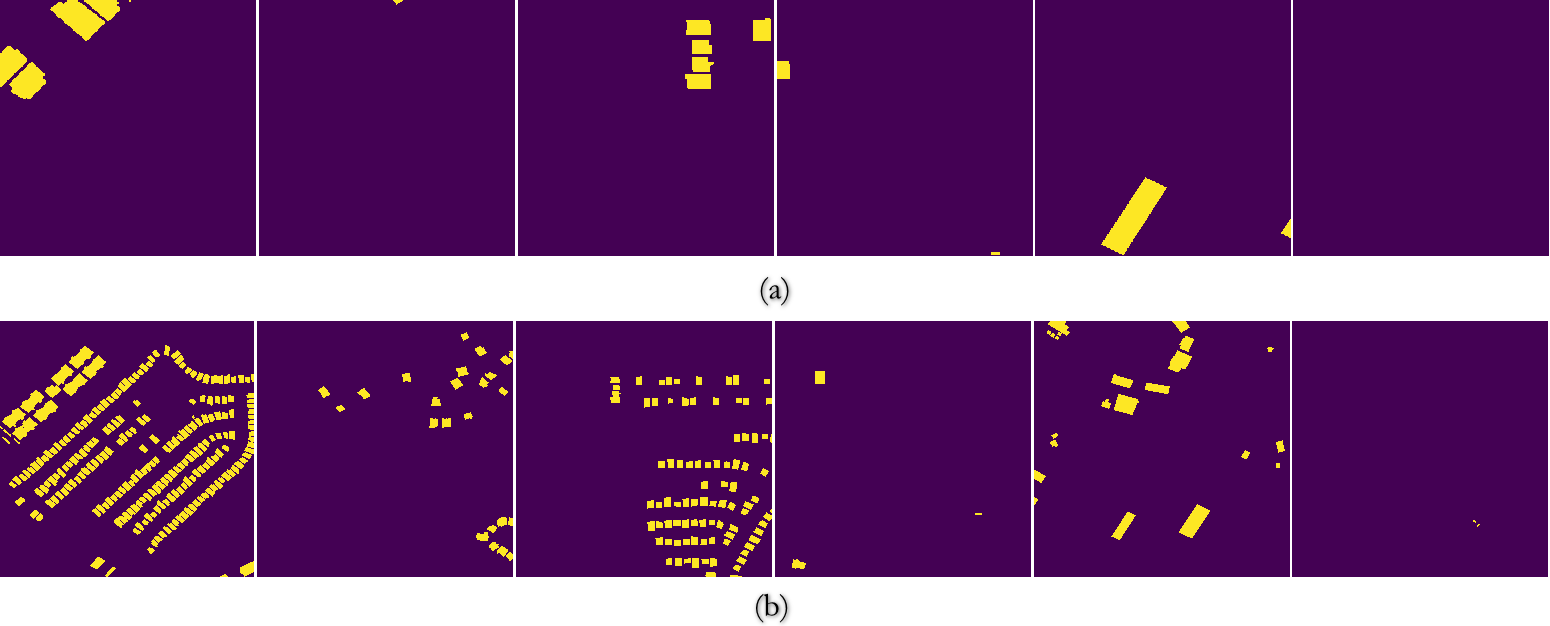