基于ARIMA模型对邮政业务总额的预测
【摘要】 本文利用Eview 7建立了中国邮政业务总额的ARIMA模型,并通过数据挖掘技术对2000-2017年中国邮政业务数据进行预测。许多非平稳序列差分后会显示出平稳序列的性质,我们对其进行差分处理,不能直接使用ARMA模型,ARIMA模型基于原始序列是否平稳以及回归过程的不同部分,包括移动平均过程(MA)、自回归过程(ARA)、自回归移动平均过程(ARMA)和ARIMA过程。
通过邮政业务总额中各分支的数据观察,快递业务影响最大。快递业务占邮政业务总量的很大比例。得出了一个结论,即邮政业务的发展状况只能通过快递业务的表现来预测。本文采用时间序列分析法对邮政业务总量进行了分析。预测2018年10月到2019年12月共15个月的邮政业务总量,并分析其发展趋势。
【关键词】 总额的预测,ARIMA模型,Eviews7
Prediction of Postal Service Total Volume Based on ARIMA Model
【Abstract】 In this paper, we use Eview 7 to build the ARIMA model of China's total postal business, and forecast the data of China's postal business from 2000 to 2017 through data mining technology. Many non-stationary sequences show the properties of stationary sequences after differential processing. We can not use the ARMA model directly. The ARIMA model is based on whether the original sequence is stationary or not and different parts of the regression process, including the moving average process (MA), the autoregressive process (ARA), the autoregressive moving average process (ARMA) and the ARIMA process.
Through the observation of the data of each branch of the total postal business, express business has the greatest impact. Express business accounts for a large proportion of the total postal business. It draws a conclusion that the development of postal service can only be predicted by the performance of express service. At the same time, this paper uses time series analysis method to analyze the total amount of postal business, and finds that the fluctuation of the total amount of postal business has seasonal trend. Therefore, this paper uses seasonal time series model to fit the total postal service volume curve, forecasts the total postal service volume in the 12 months of 2019, and analyses its development trend.
【Key Words】 Total Postal Service,ARIMA model, Eviews7
图目录
图3.1 ARIMA的模型流程图 8
图3.2原始数据y的自相关图 9
图3.3原始数据y的偏相关图 9
图3.4原始数据y的ADF检验图 10
图4.1原始数据y的一阶差分时序图 11
图4.2原始数据y的一阶差分序列图 11
图4.3 dy的ADF检验图 12
图4.4 dy的的自相关图 13
图4.5 dy用ar(12)ma(12)定阶图 13
图4.6 dy用ar(24)ma(24)定阶图 14
图4.7残差检验图 14
图4.8 RESED03的自相关图
图4.9 模型预测图
图5.1 y1一阶差分时序图
图5.2 dy1用ar(12)ma(12)定阶图
图5.3 模型预测图
图5.4 快递业务邮政业务对比图
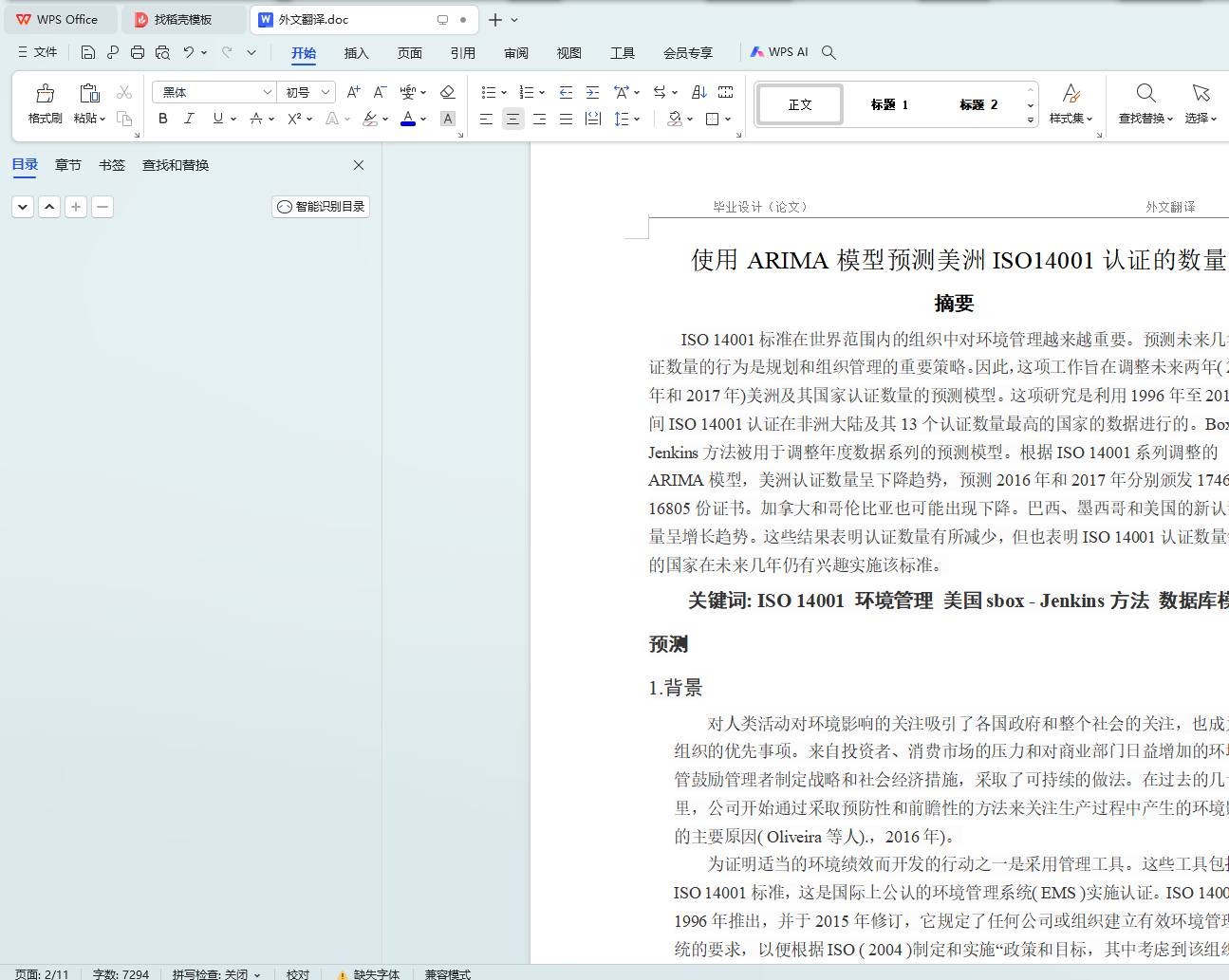
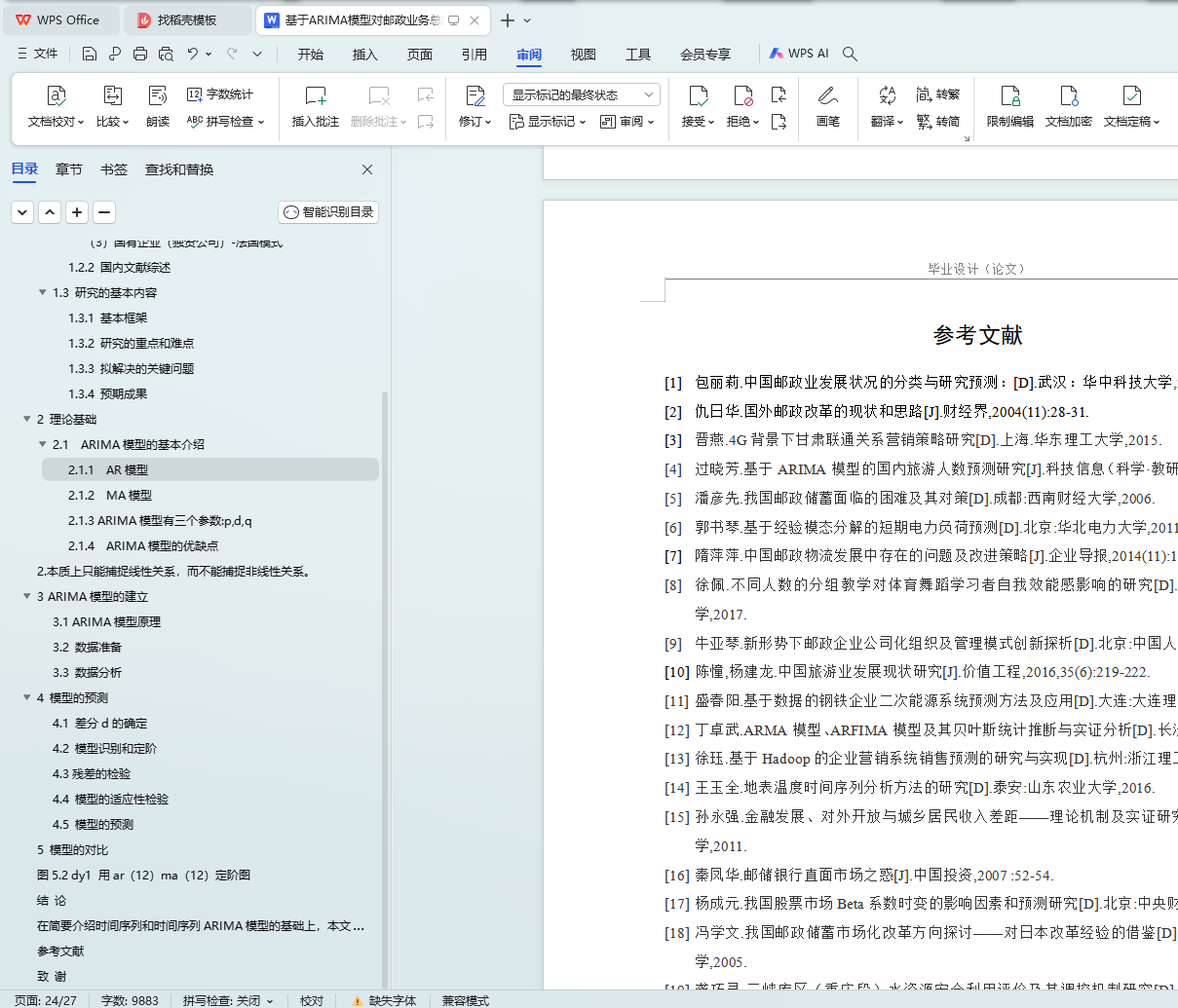
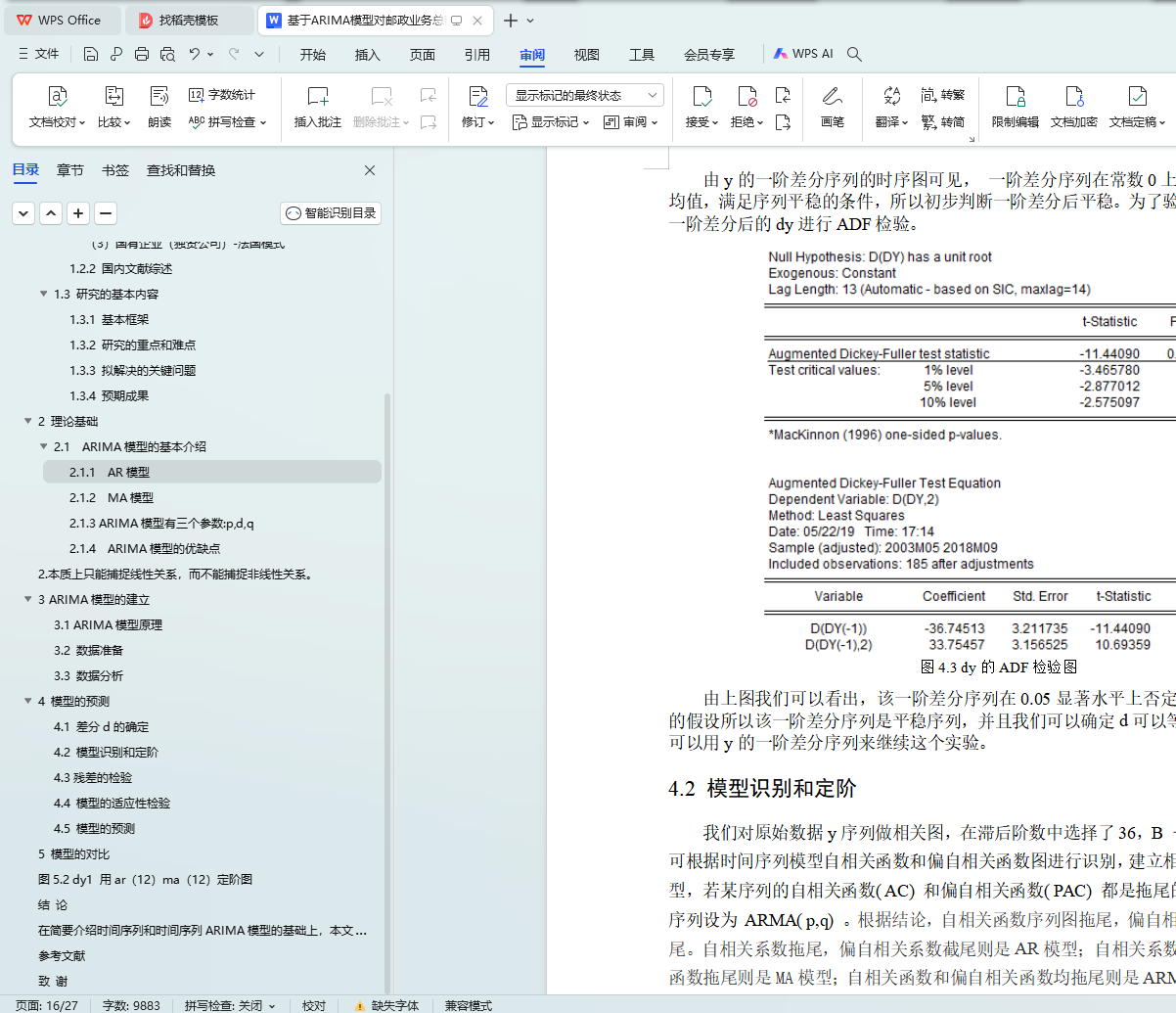
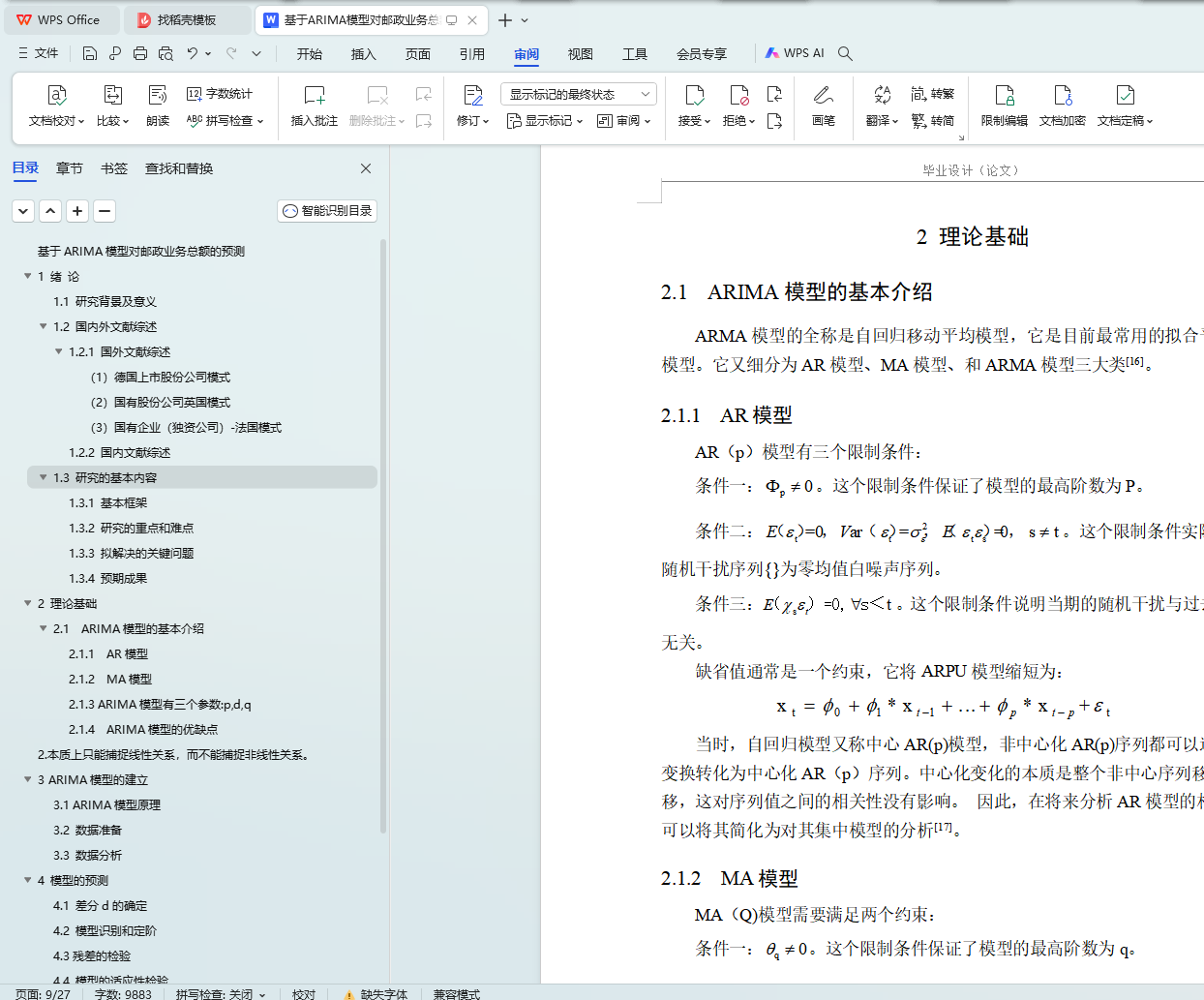
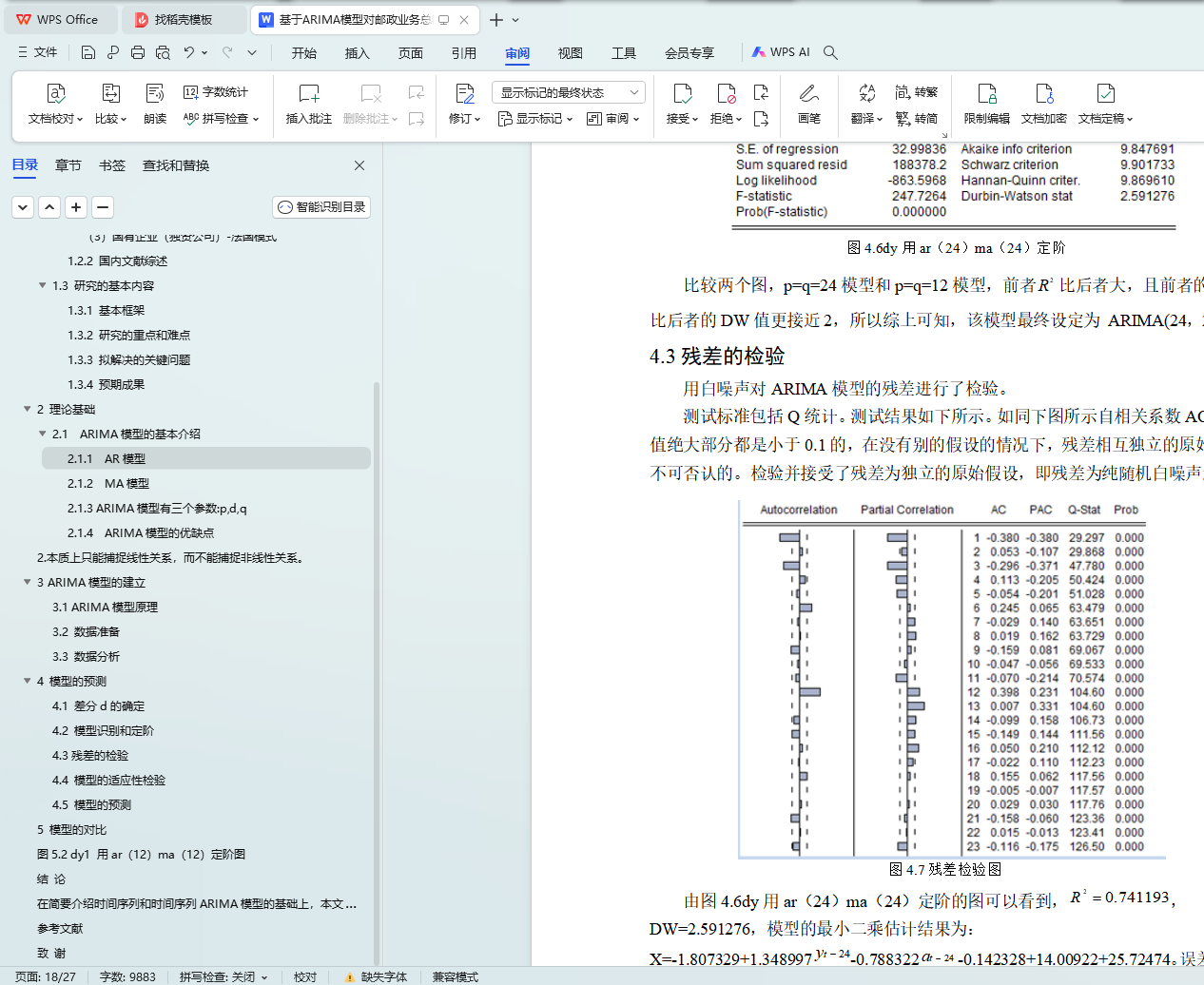
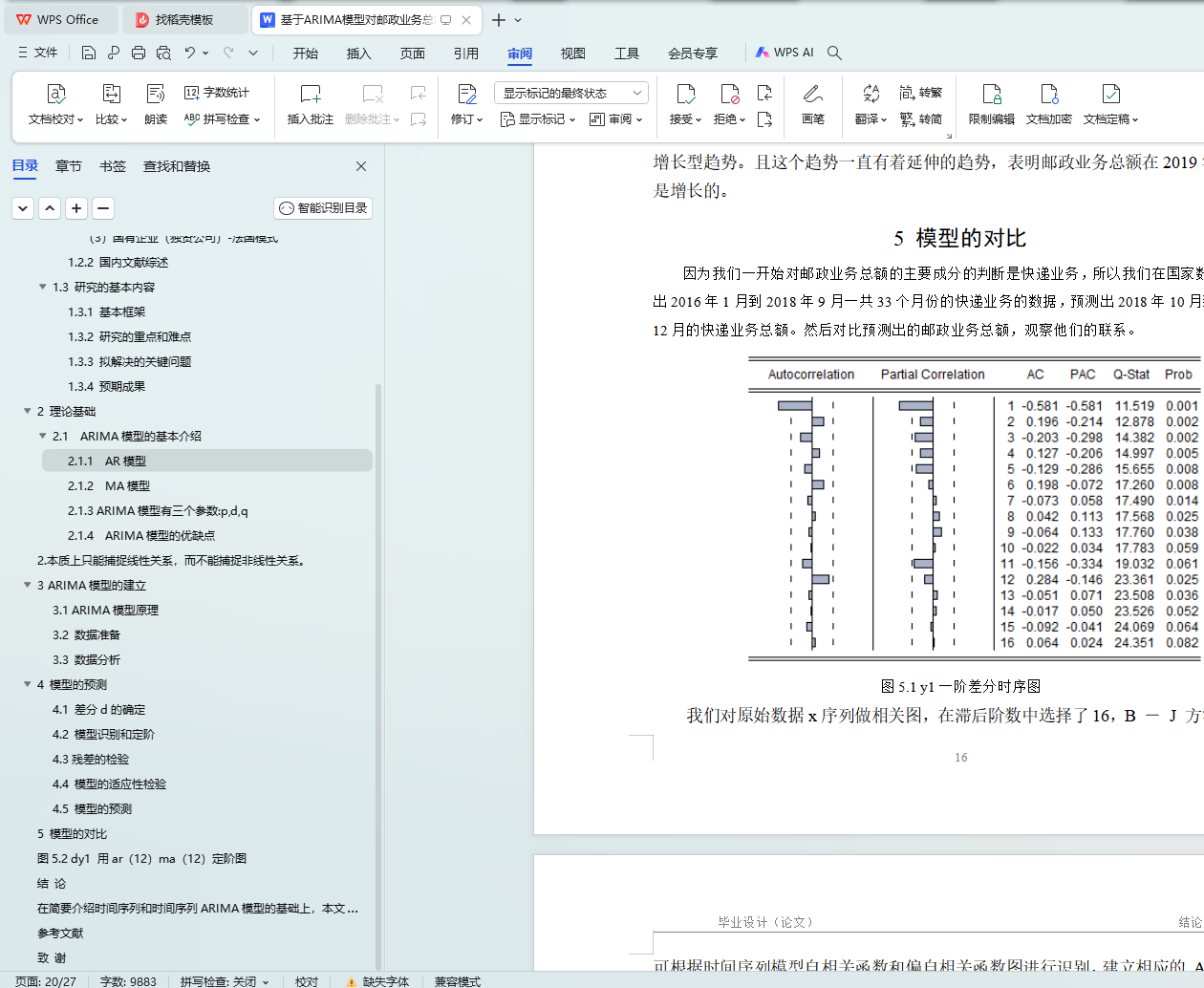
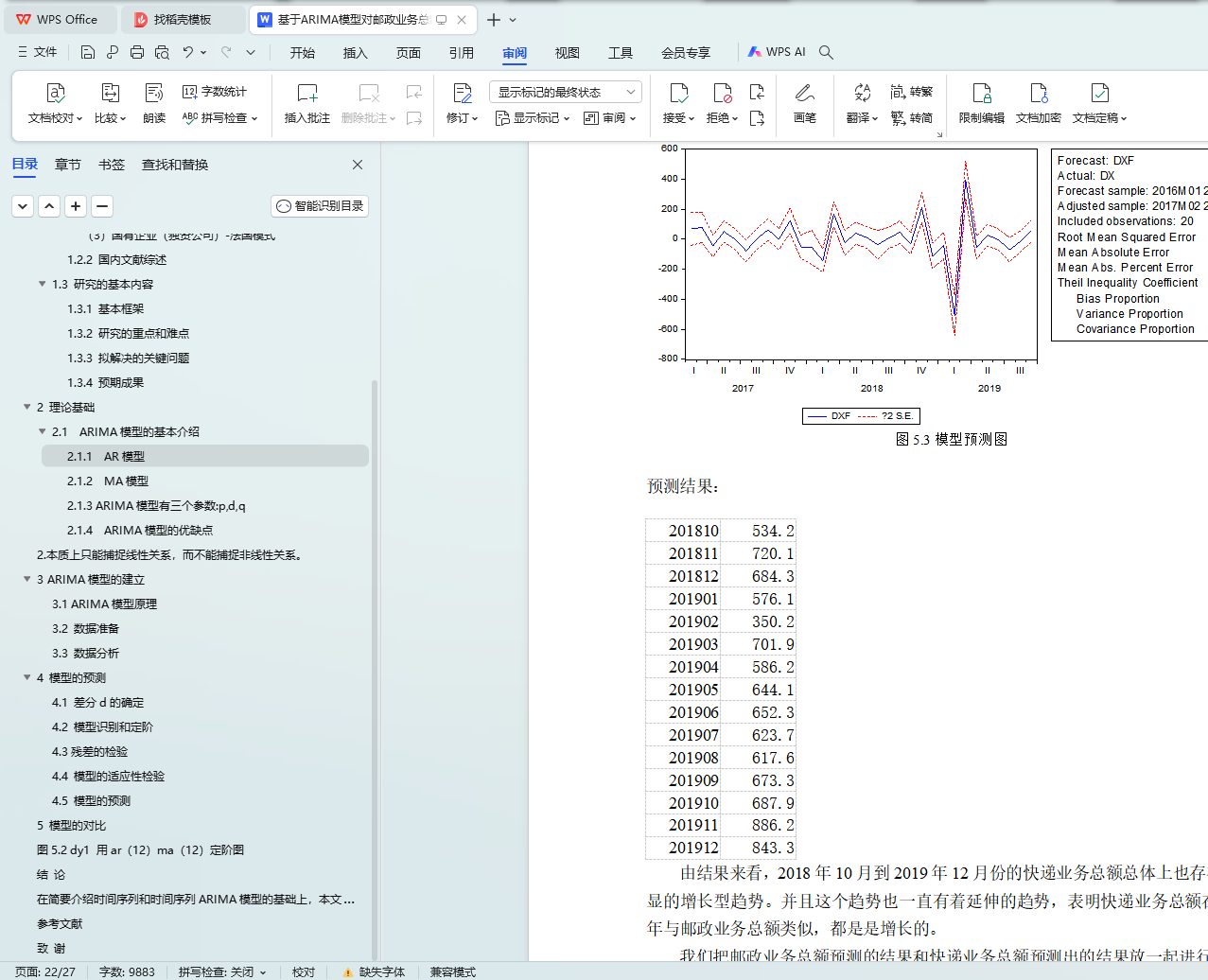
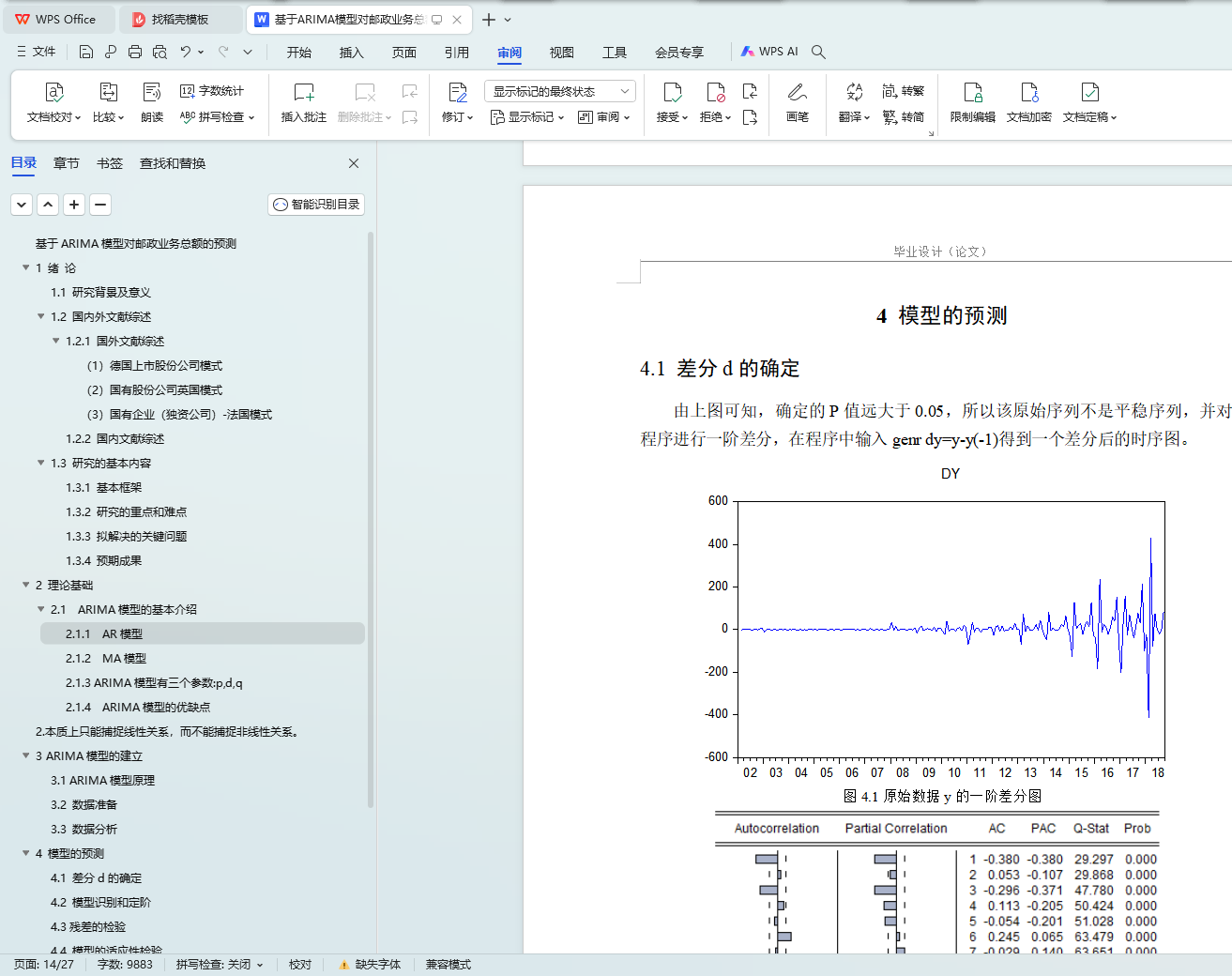
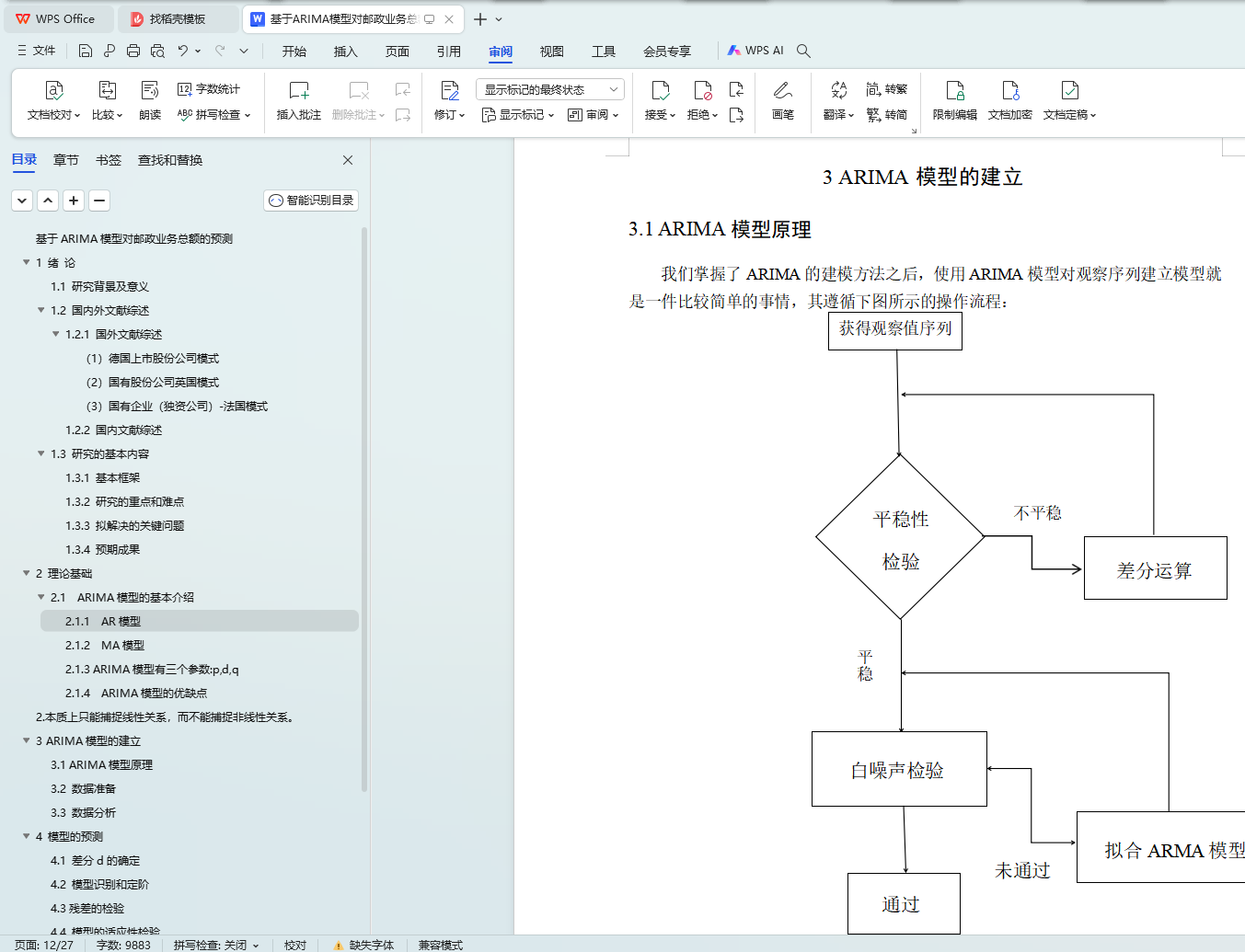
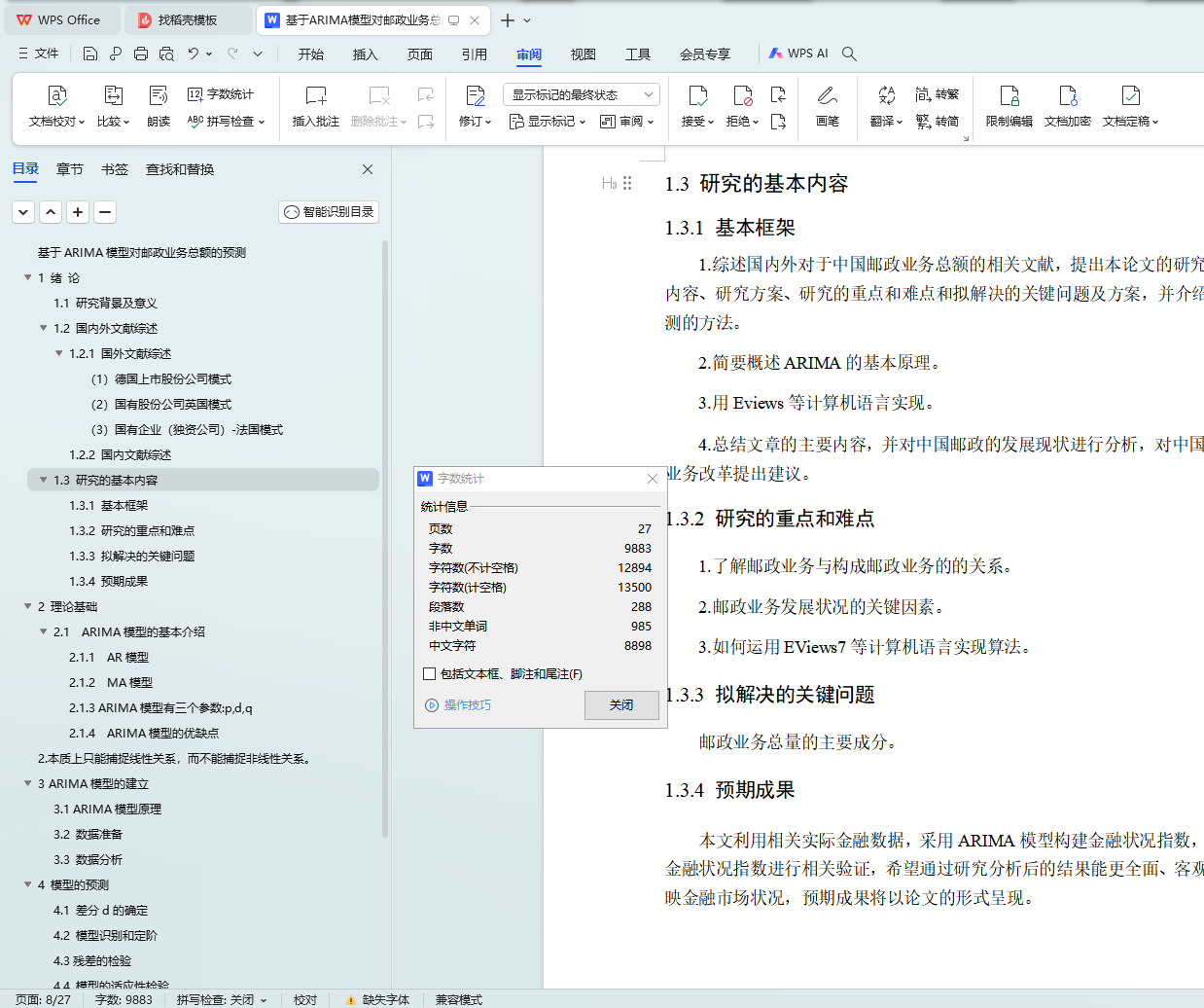
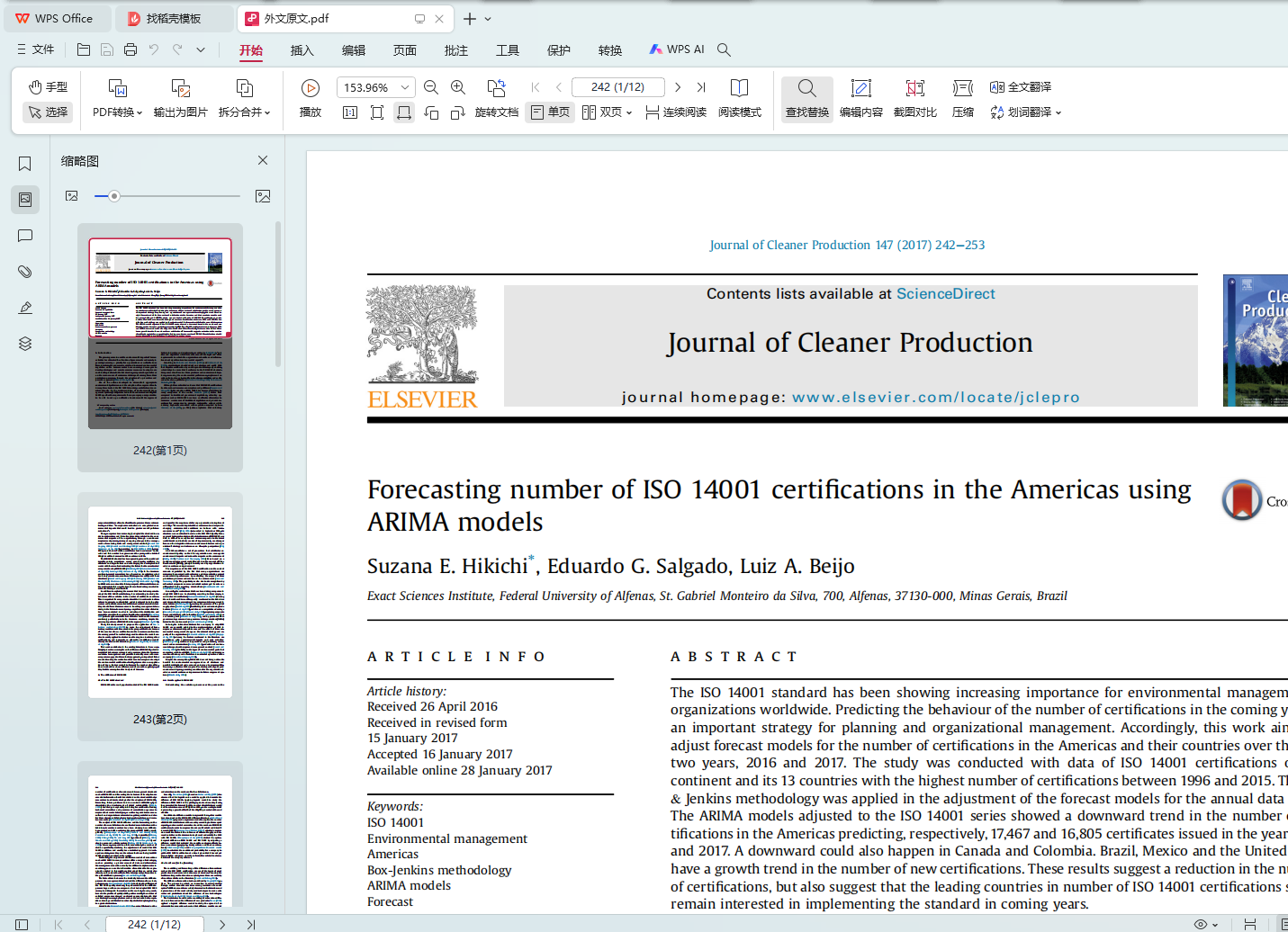
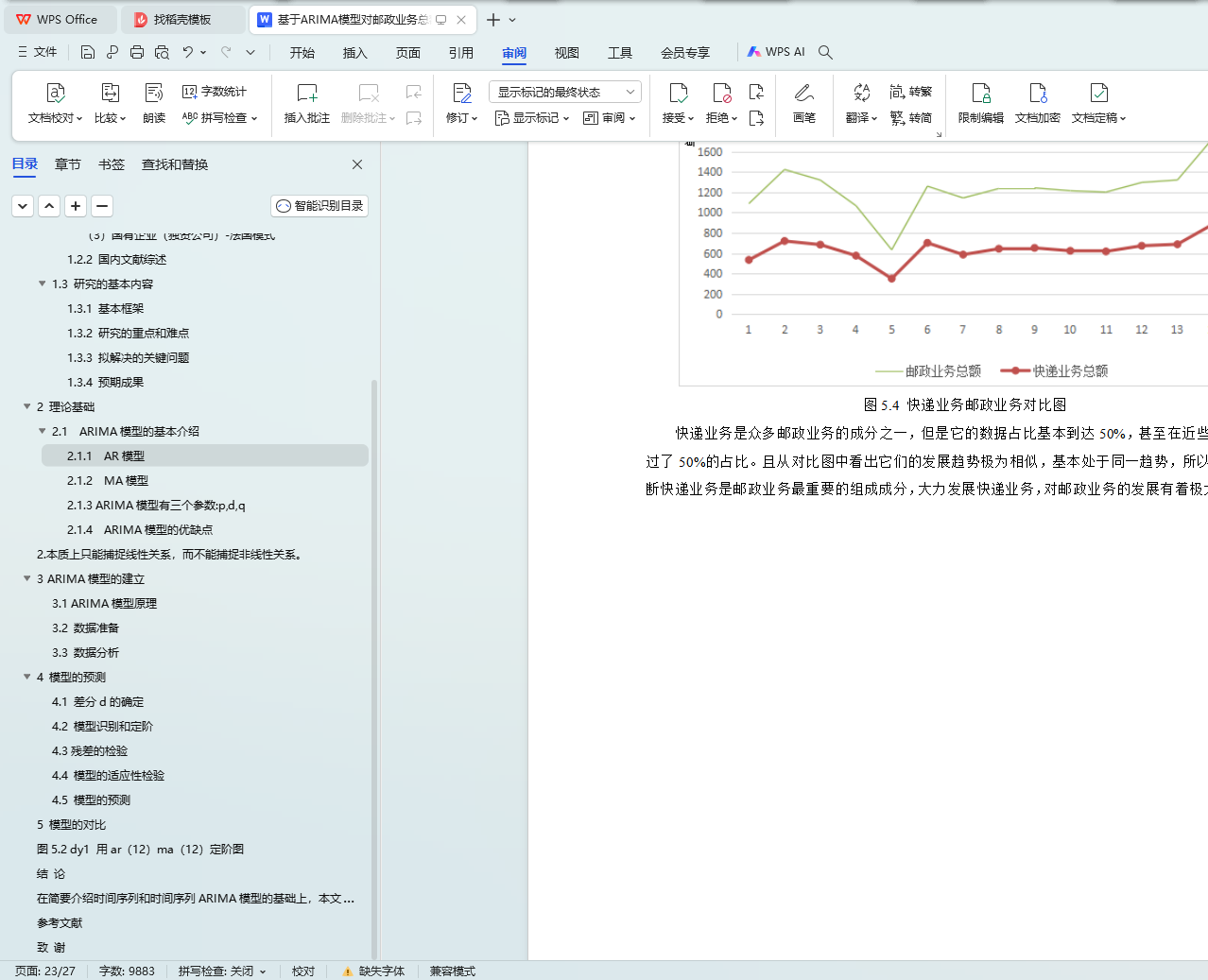
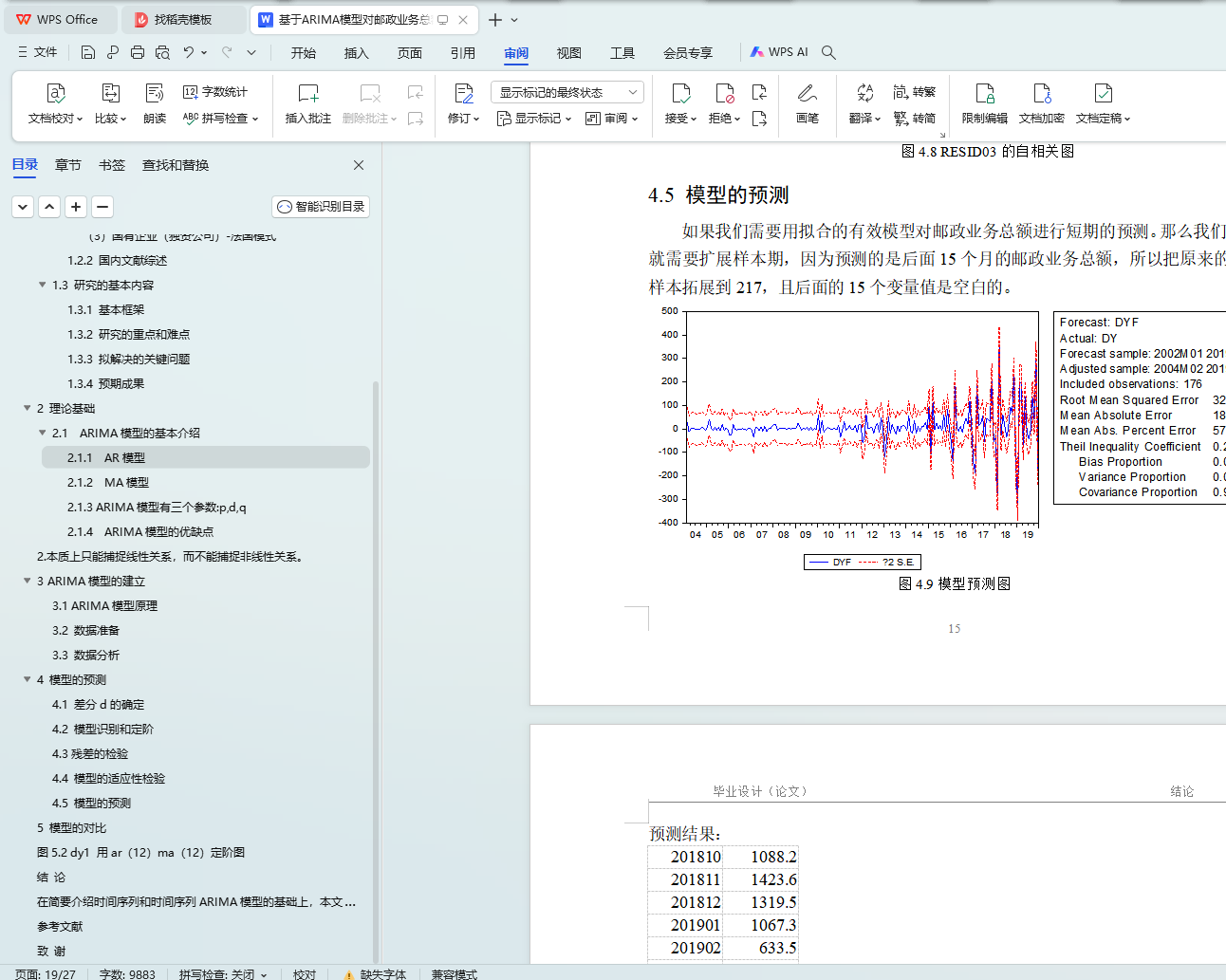
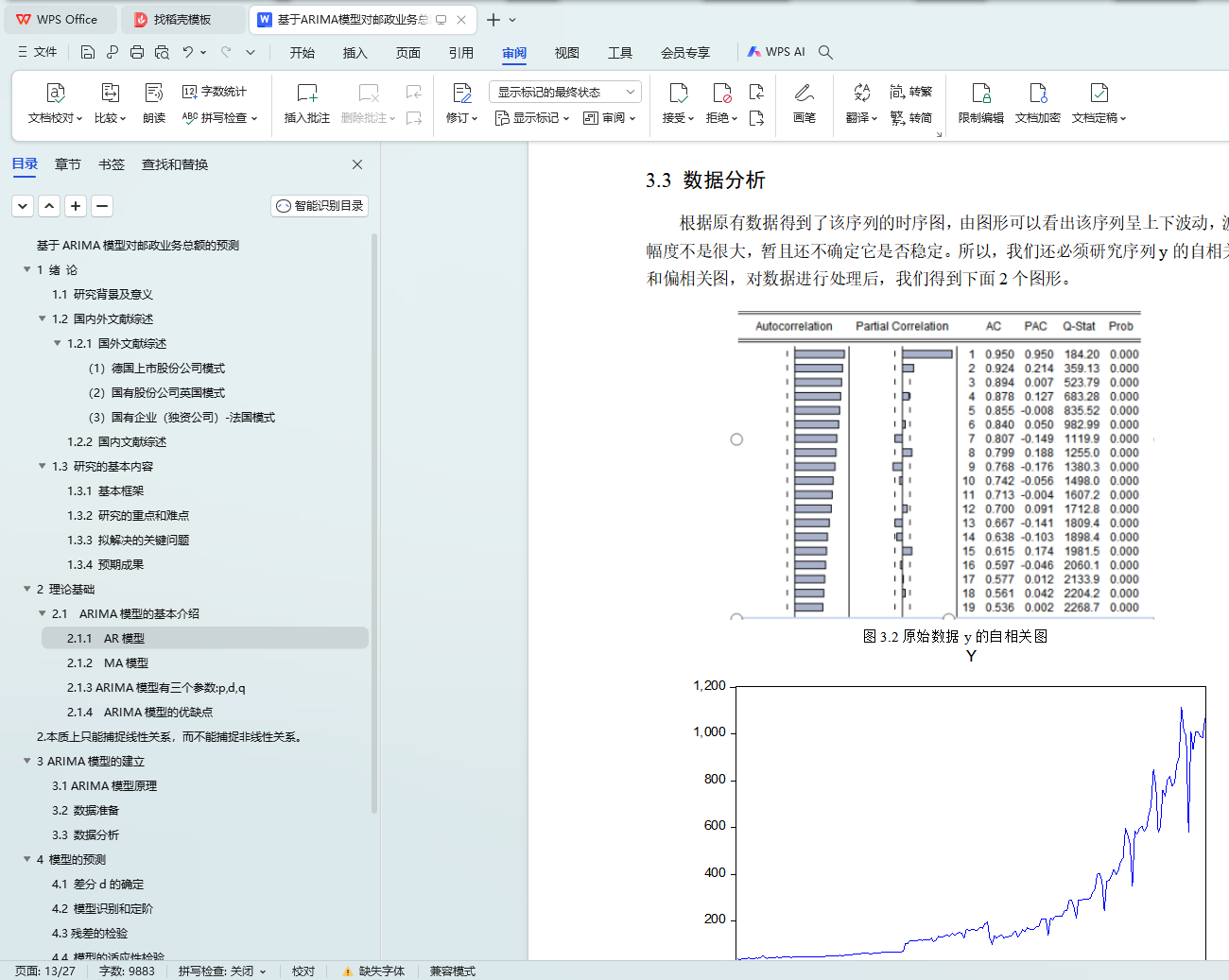
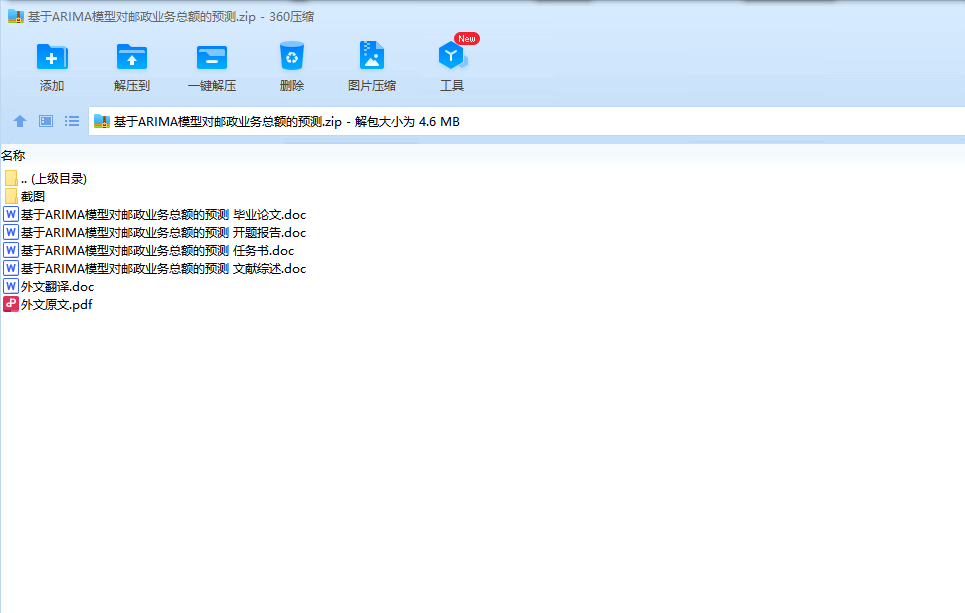