基于 CRF 和 Bi-LSTM 的命名实体识别
摘要
命名实体识别任务主要是识别文本中的人名、地名、机构名等实体。基于 CRF 的命名实体识别是传统的识别方法,而随着深度神经网络的发展,深度学习也逐渐应用到实体
识别中。本文将首先介绍命名实体识别的相关工作,然后介绍 CRF 模型原理,以及用于
命名实体识别的深度神经网络 Bi-LSTM 网络。之后实现基于 CRF 和 CRF+Bi-LSTM 的两种命名实体识别模型,并使用 BioNLP 数据集进行实验分析。实验中主要分析了
CRF 模型的特征选取和组合对模型的影响,同时进行了参数调整以获取最优 CRF 实体识别模型。之后分析 CRF+Bi-LSTM 模型各参数对模型性能的影响并做相关调整以获得最优性能,最后将 CRF 模型和 CRF+Bi-LSTM 模型的命名实体识别效果进行对比。实验结果表明,CRF+Bi-LSTM 模型相对于 CRF 模型有更好的识别效果。
关键字:CRF;Bi-LSTM;命名实体识别
Named Entity Recognize Based On CRF and Bi-LSTM
Abstract
The named entity recognition task mainly identifies entities such as person names, place names, and institution names in the text. CRF-based named entity recognition is a traditional recognition method, and with the development of deep neural networks, deep learning is gradually applied to entity recognition. This article will first introduce the current state of named entity recognition, and introduce the CRF model, as well as the deep neural network Bi-LSTM network for named entity recognition.Two named entity recognition models based on CRF and CRF+Bi-LSTM were then implemented and experimental analysis was performed based on the BioNLP data set. It mainly
analyzes the influence of feature selection and combination of CRF model on the model, and adjusts the parameters to obtain the optimal CRF entity recognition model. After that, the influence of each parameter of CRF+Bi-LSTM model on the performance of the model is analyzed to obtain the optimal performance. Finally, the CRF model and CRF+Bi-LSTM model are compared for the performance of named entity recognition. The experimental results show that the CRF+Bi-LSTM model has a better recognition effect than the CRF model.
Keywords:CRF;Bi-LSTM;NER
目录
基于 CRF 和 Bi-LSTM 的命名实体识别
摘要
Abstract
0 引言
1 相关工作
2 基于 CRF 的命名实体识别
2.1 CRF
1 2 n
(2)
2.2 特征提取
2.3 CRF 模型框架
3 基于 CRF 和 LSTM 的命名实体识别
3.1 Bi-LSTM
3.2 CRF+Bi-LSTM 模型框架
4 实验
4.1 实验环境
@ 3.40GHz(2 核) 内存:13G
4.2 实验数据
4.3 评价指标
正确识别的命名实体个数
(5)
4.4 实验结果及分析
4.4.1 CRF 模型
4.4.2 CRF+Bi-LSTM 模型
4.4.3 模型对比
5 结束语
参考文献
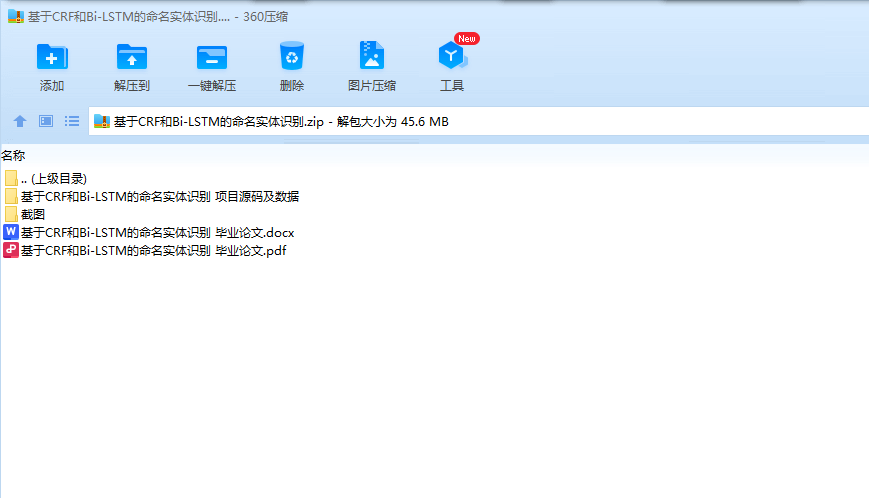
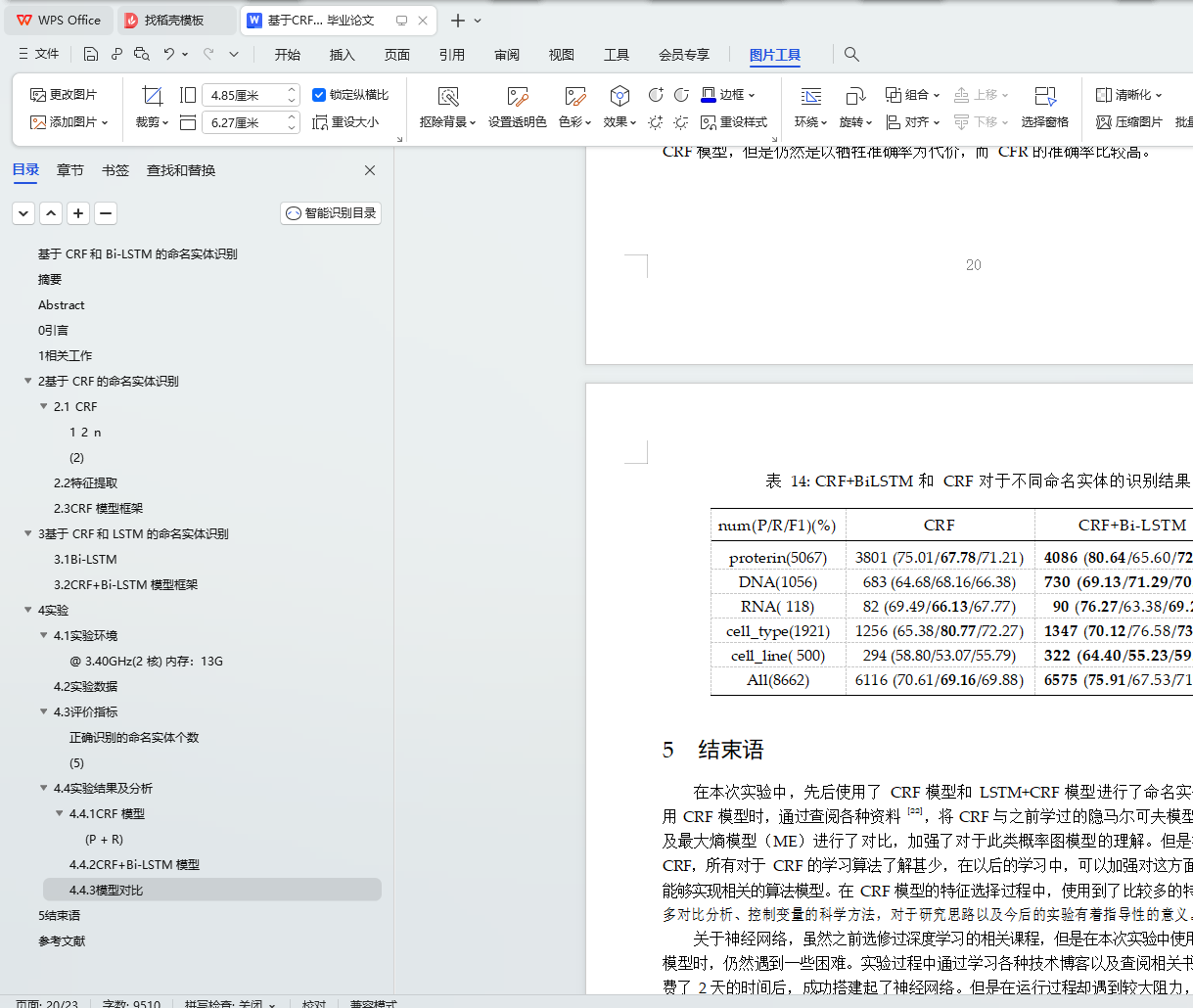
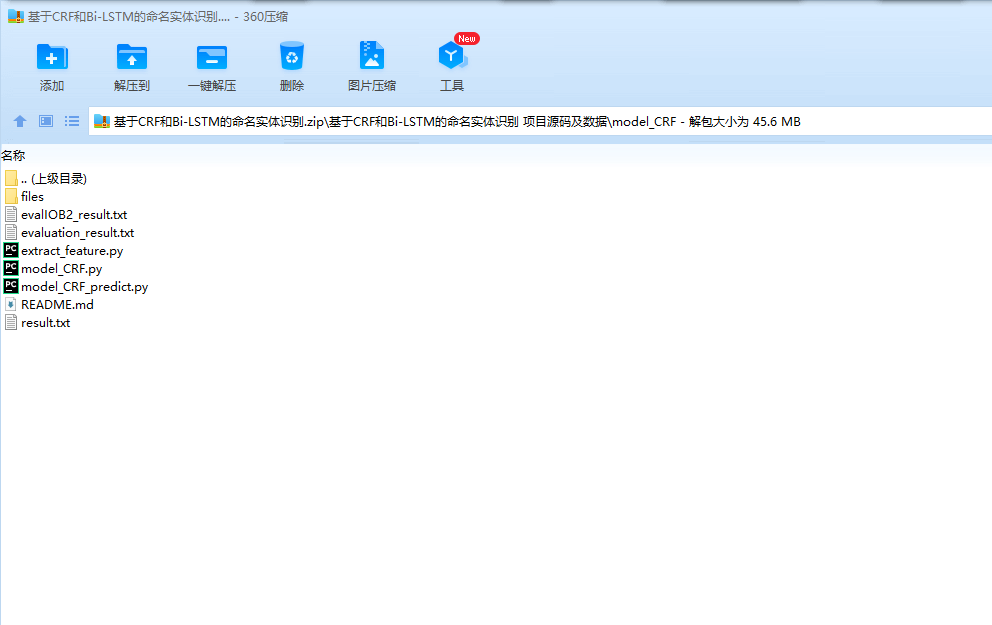
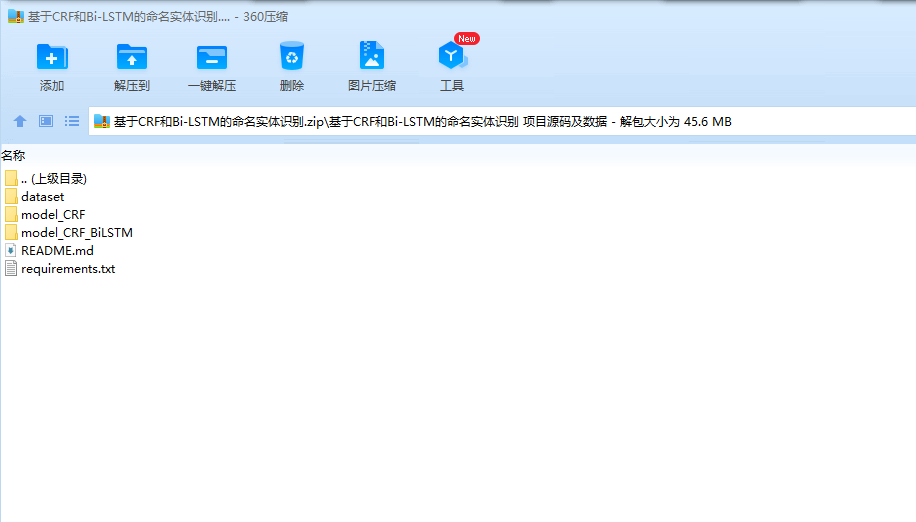
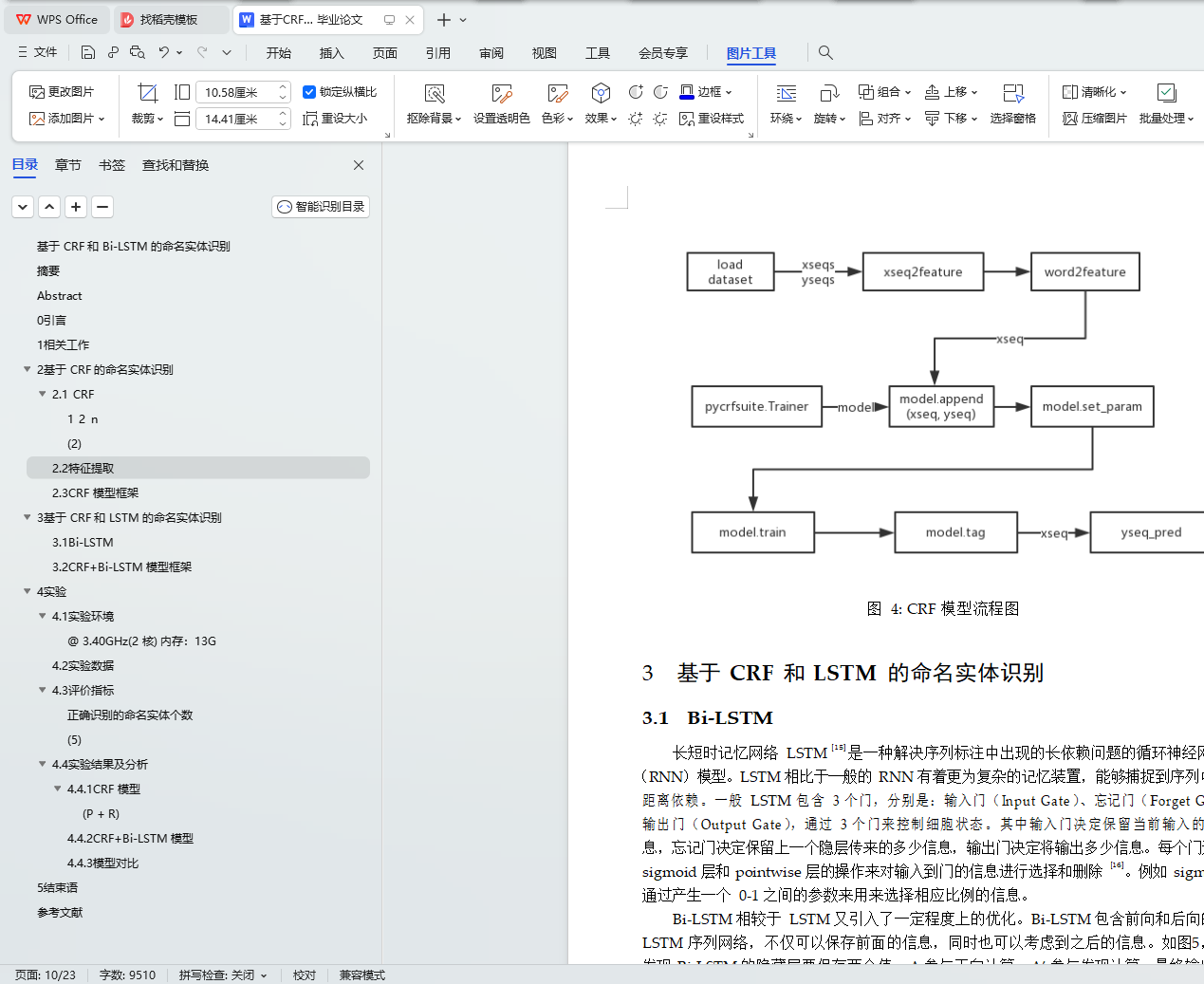
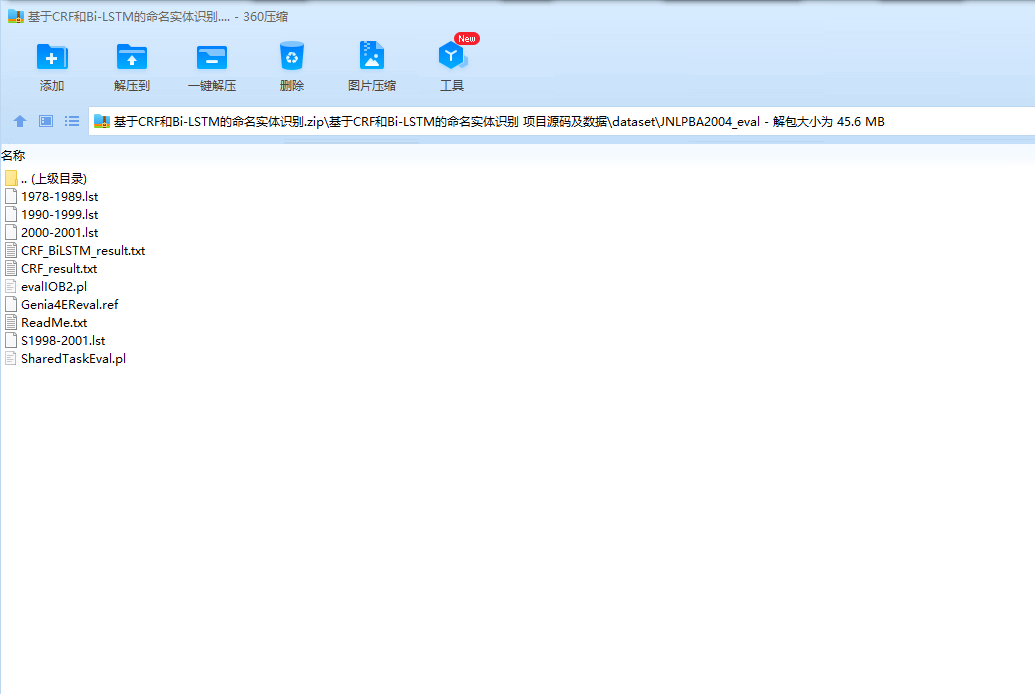
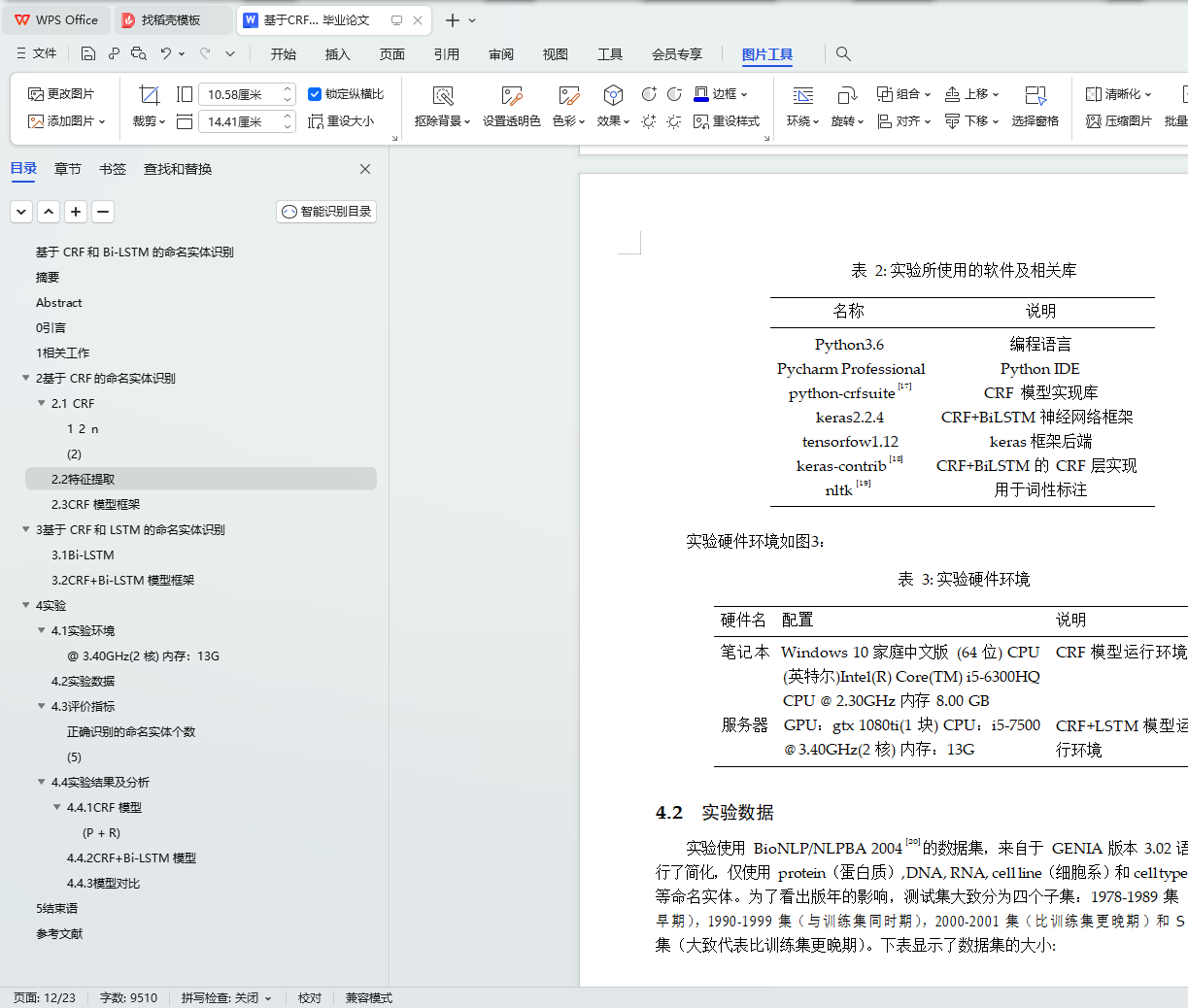
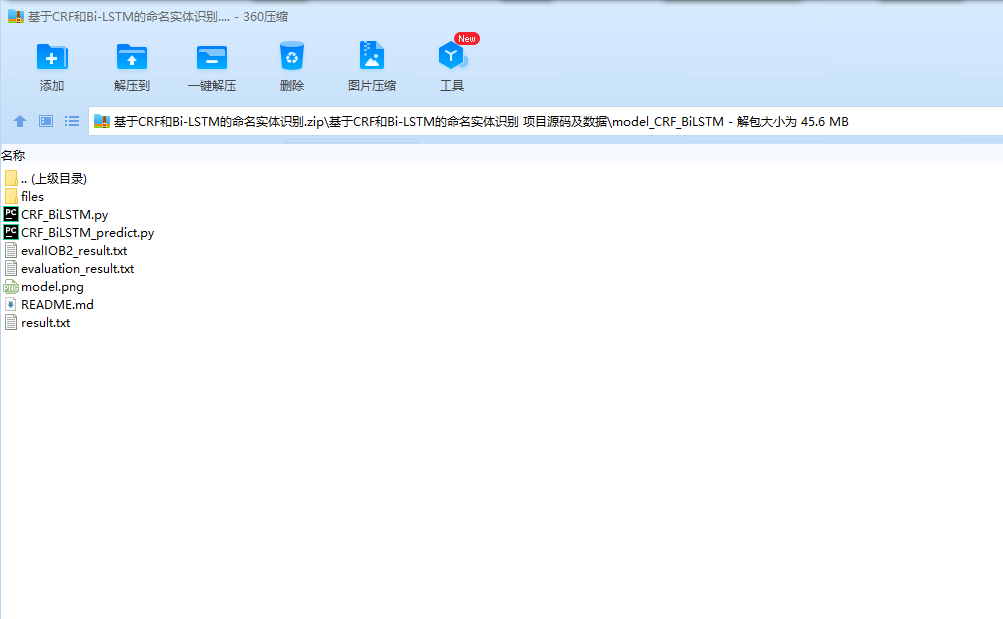
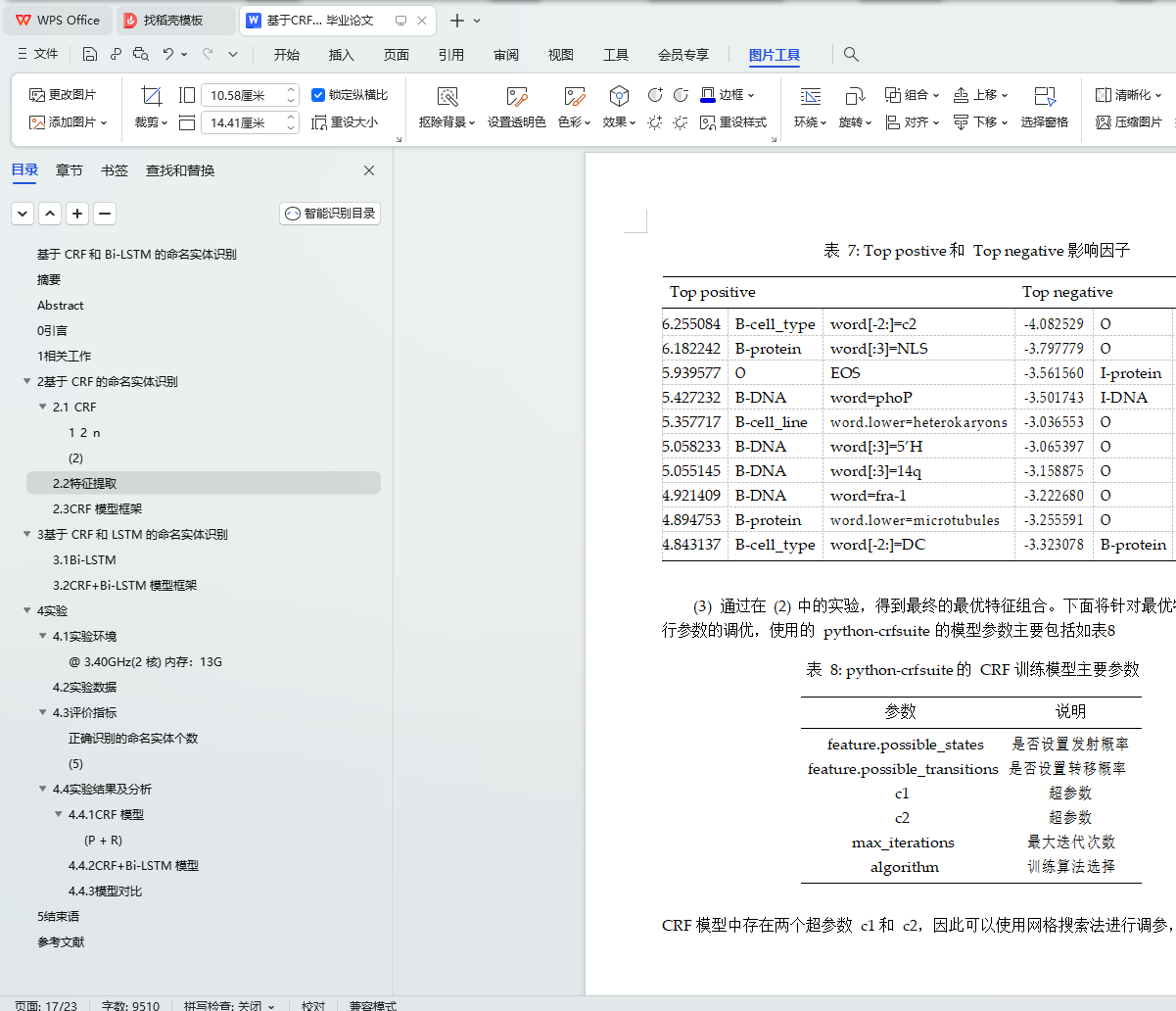
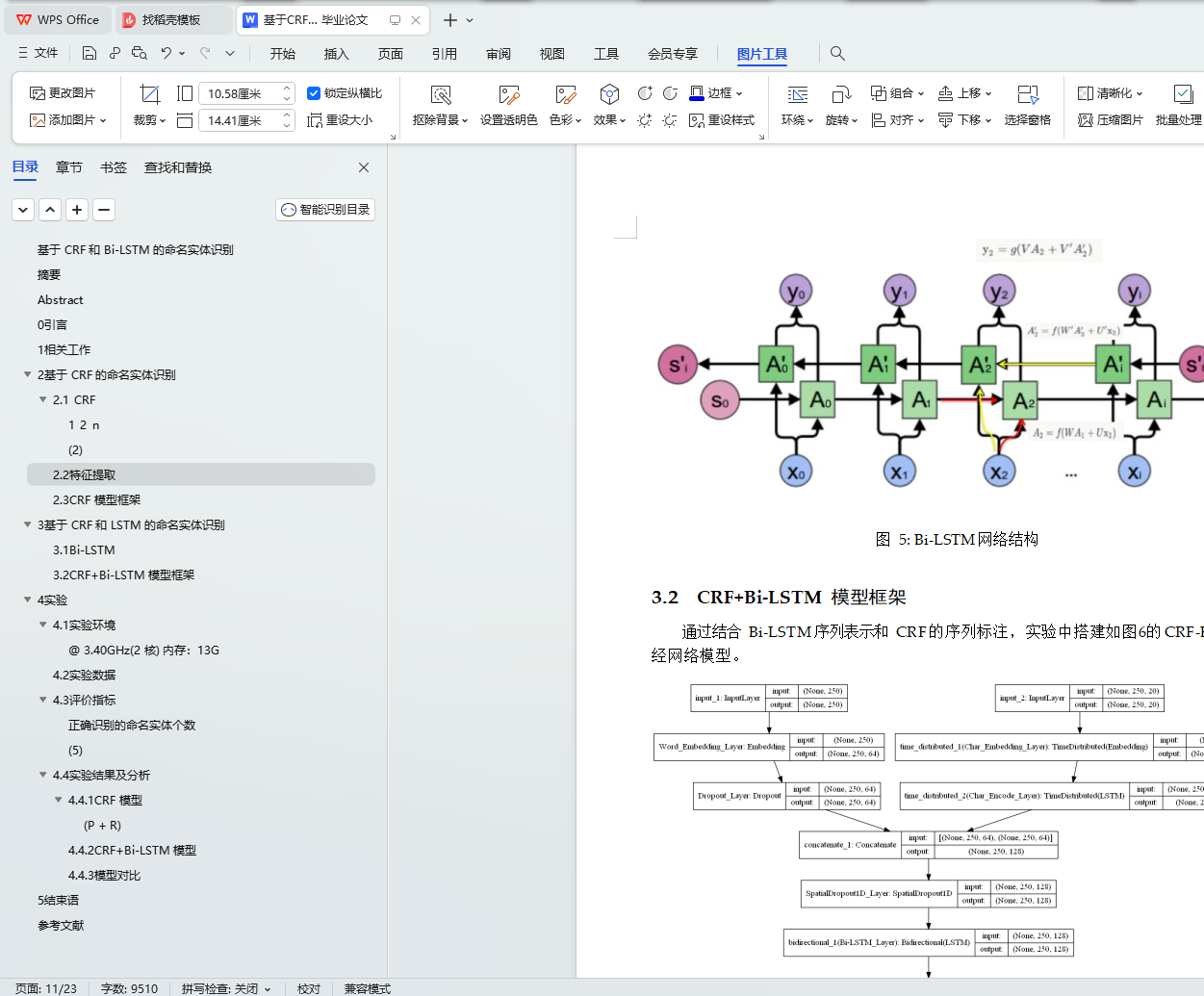
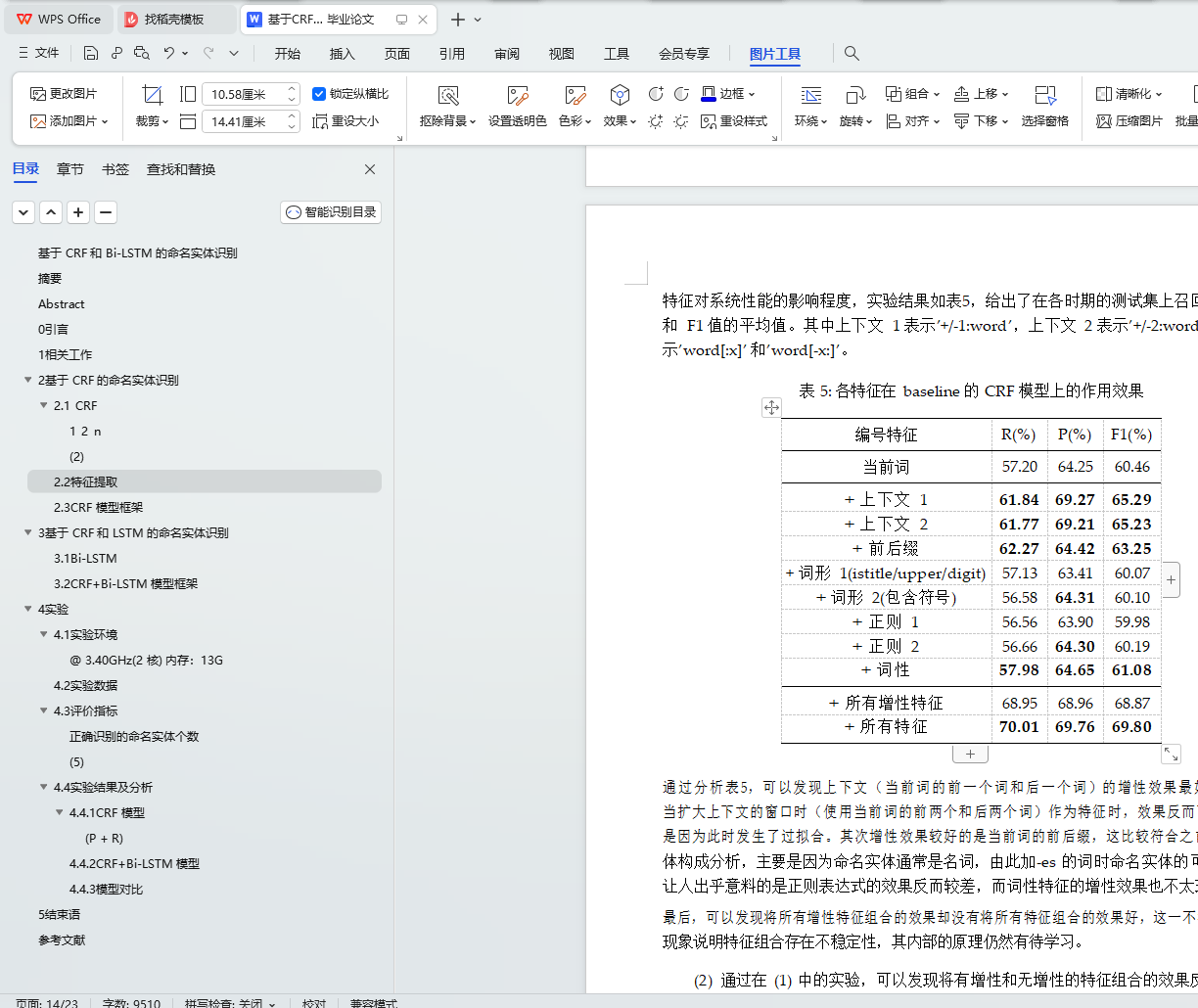
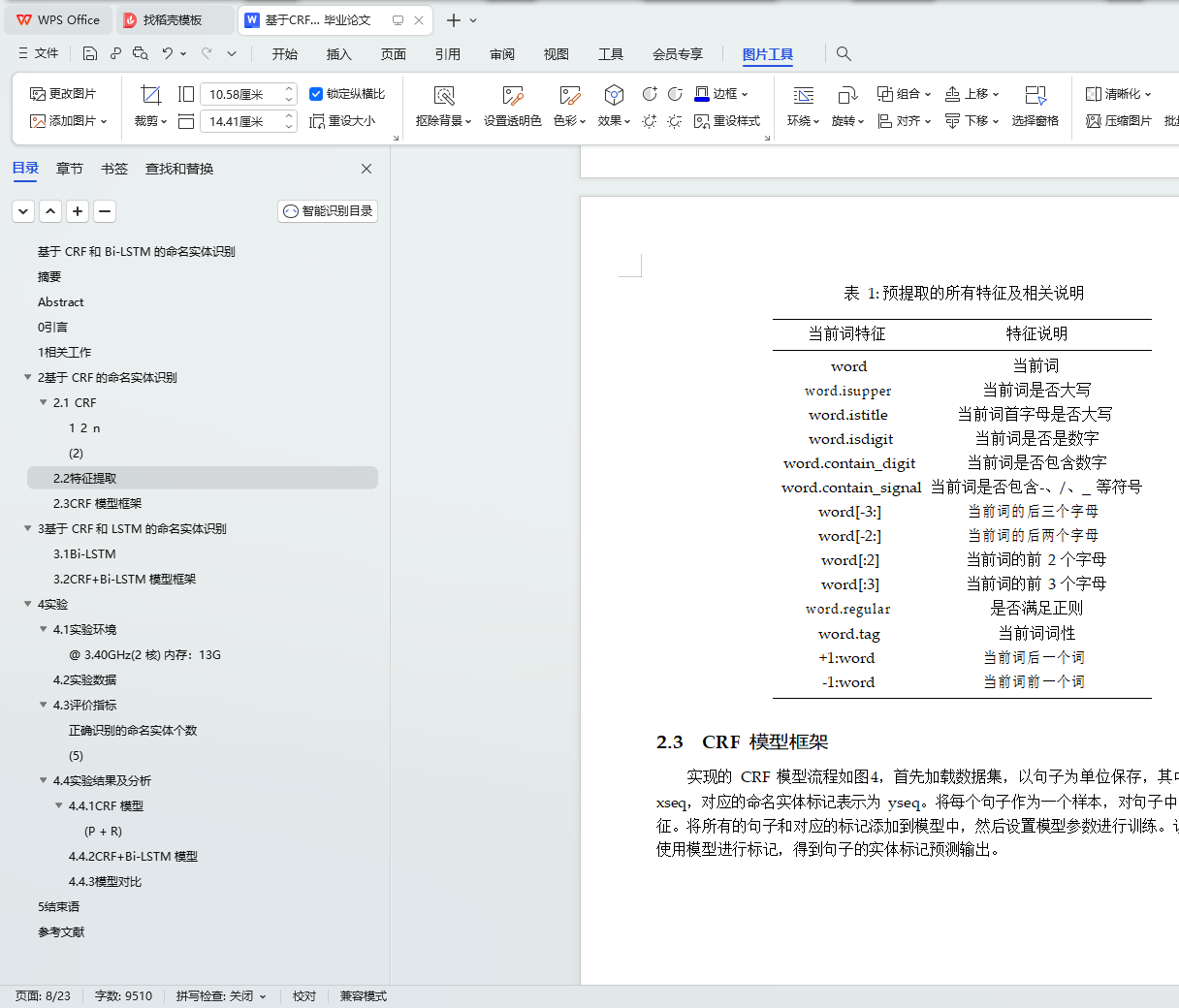
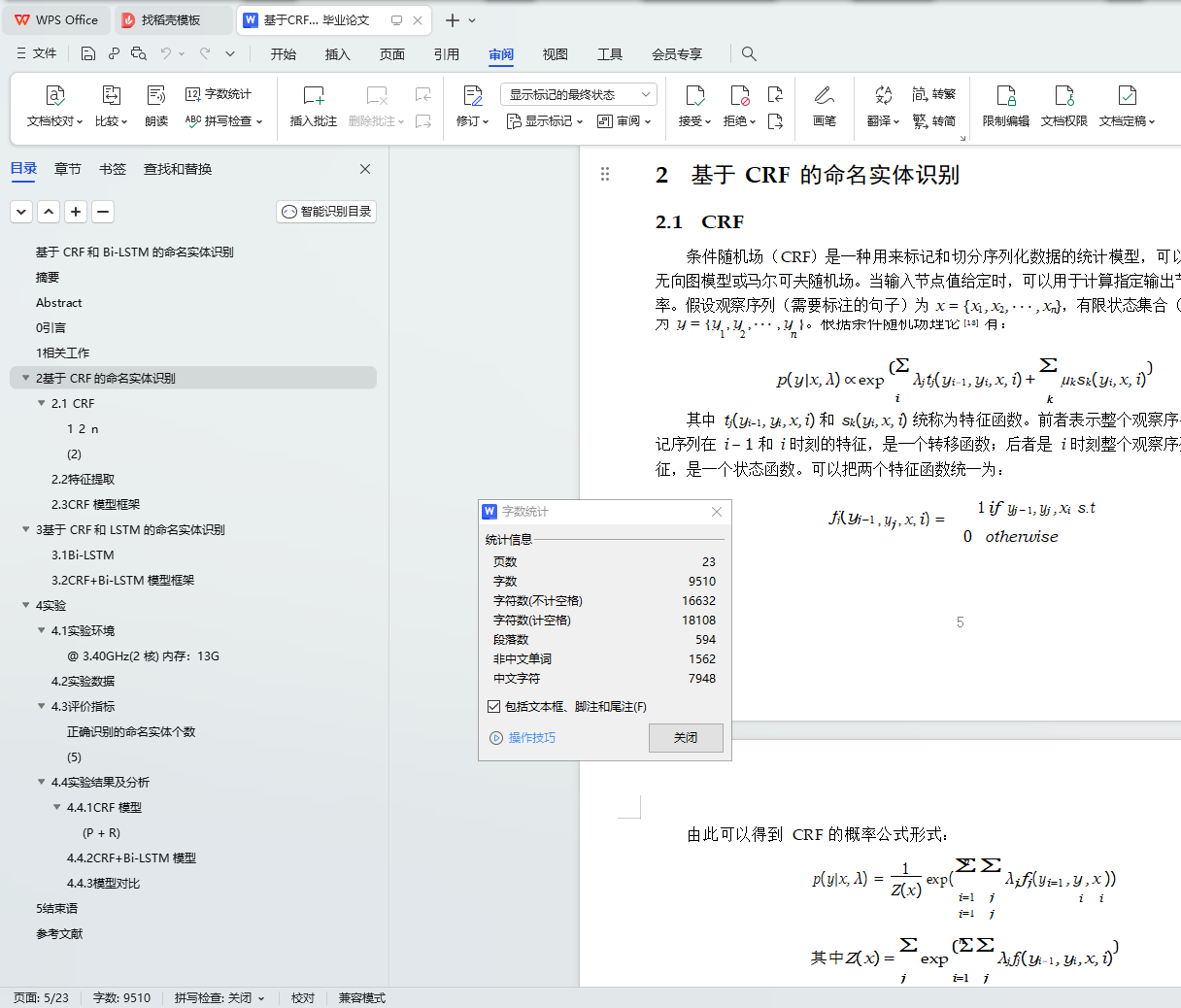
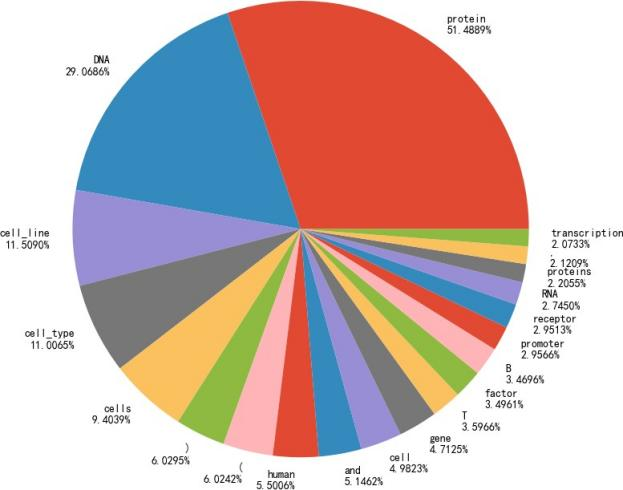
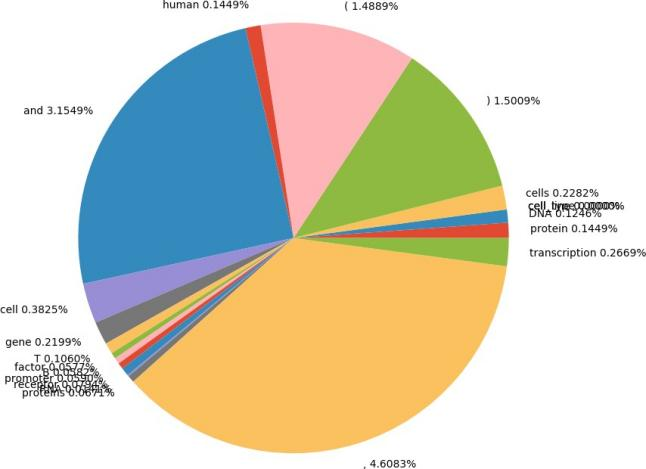
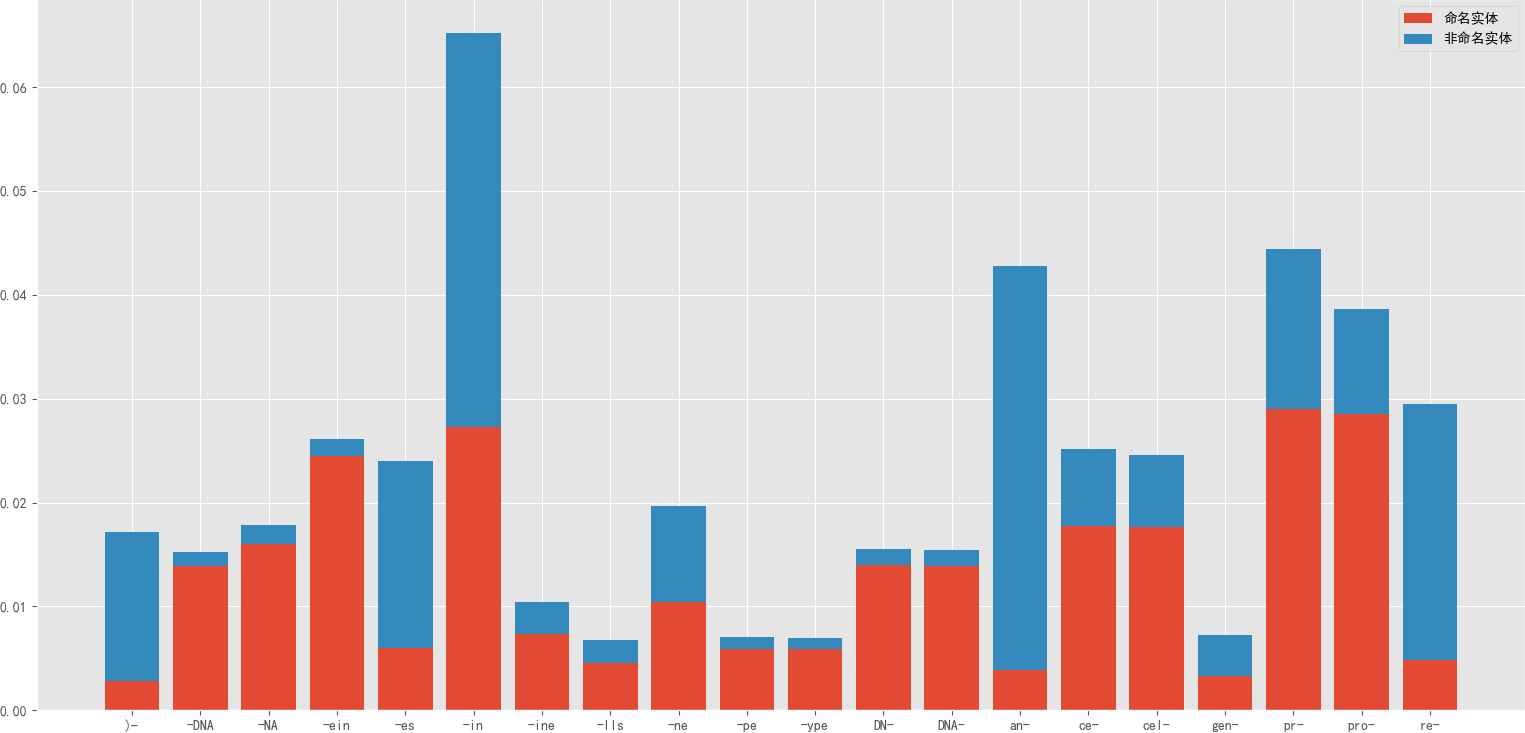
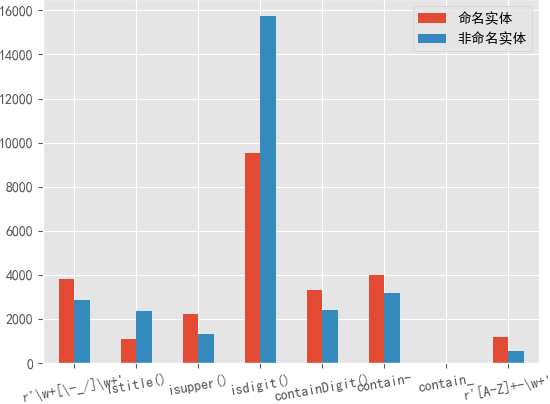
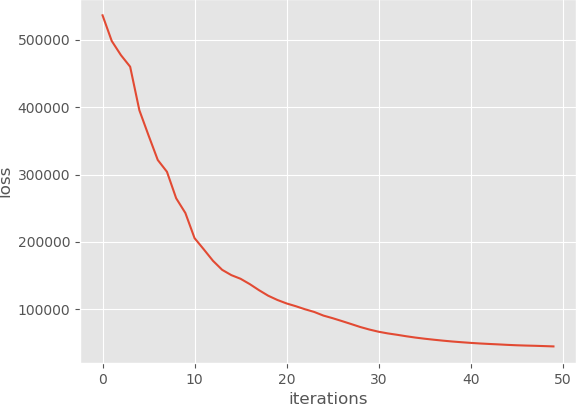
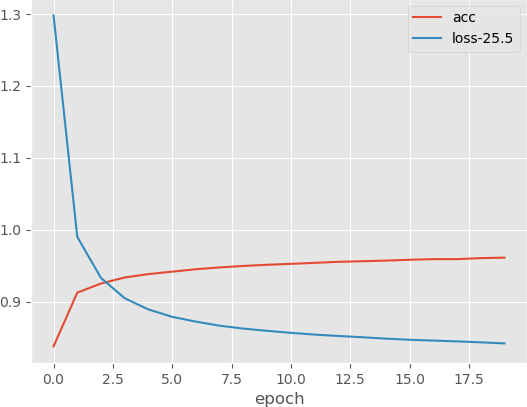