摘 要
视频问答是深度学习中重要的问题之一,被广泛应用于安防、广告系统中,提高视频问答的准确率具有非常重要的意义。近年来,理解视频的内容是在现实世界中开发各种有用应用程序的核心技术之一,例如识别监视系统的各种人为行为或在自动商店中进行客户行为分析。然而,由于其庞大的数据量和时间结构,理解视频的内容仍然是一个具有挑战性的问题。近年来,自然语言处理中注意力机制方法得到了人们的关注,并迁移到视频问答任务上。但是,现有的方法仍存在四个方面的不足:一是使用对整个视频进行提取特征,这样虽然能够捕捉到视频的所有信息,但是由于视频本身的冗余性,训练代价巨大,得不偿失;二是部分现有方法采用了提取片段帧信息试图来描述视频,提取过多导致内容冗余,提取过少造成内容缺失;三是问题的处理比较粗糙,并没有停用词进行处理。四是现有的模型并没有考虑视频问答任务复杂性与逻辑性。以上的不足影响了模型的泛化性能,同时由于准确率低使得现有的视频问答模型不能够广泛应用于工业界。
本文在注意力机制框架下,提出了先验信息注意力机制 MASK 模型,并在这个基础之上提出了两种不同的视频问答方案:先验MASK的多注意力机制的视频问答方案和先验MASK的图注意力机制的视频问答方案。
先验MASK的多注意力机制的视频问答方案提出了3种注意力机制以及先验MASK方法,首先该方案采用帧特征提取视频的关键帧,然后通过Faster R-CNN以及残差网络提取视频中帧特征,以此得到特征以及视频关键帧中的对象标签,使用word2vec以及LSTM对问题进行编码,将抽取到的视频特征以及视频标签和问题文本特征融合输入到上述的先验MASK注意力机制模型中,最后得到问题的答案。本文模型在天池之江杯(ZJB)大赛中取得了冠军,同时通过大量的对比实验证明了本文的方法较现有的方法更有优越性。
而先验MASK的图注意力机制的视频问答方案则采用了图数据结构来表达视频种物体与物体之间的关系。使用Faster R-CNN提取出视频关键帧中物体的坐标以及所属类别,采用节点注意力机制以及边注意力机制将物体作为节点构建图,然后通过问题特征与图特征进行嵌入,得到的结果放入先验MASK中,得到模型的最终答案,实验结果表明,虽然图节点大大减少了网络模型的参数量,但是精度并没有损失很多,在某些对精度要求不是很高但对速度要求很高的场景该方案具有较大的应用价值。
关键词: 视频问答;深度学习;先验MASK;注意力机制;图注意力
Abstract
In recent years, understanding the content of videos is one of the core technologies for developing various useful applications in the real world, such as identifying various human behaviors in surveillance systems or conducting customer behavior analysis in automated stores. However, due to its huge amount of data and time structure, understanding the content of the video remains a challenging issue. In recent years, the attention mechanism method is an important method for researching video question answering. However, the current methods have the following four shortcomings: one is to use video for feature extraction, so although it can capture all the information of the video, but because of the redundancy of the video itself, the training cost is huge, and the gains are outweighed; the second is some methods The extracted frame information is used to describe the video, but there is still a lot of redundancy; the third is that the processing of the problem is relatively rough, and the stop words in the problem are not processed. Fourth, the complexity and logic of video question-and-answer tasks are not considered. The above-mentioned shortcomings greatly affect the generalization performance and accuracy of the model.
Based on deep learning, this paper first proposes a priori MASK attention mechanism model. Based on this, two different video question answering schemes are proposed, namely the video question answering scheme of multi-attention mechanism of prior MASK and the video question answering scheme of graph attention mechanism of prior MASK.
The video question answering scheme of multi-attention mechanism of prior MASK proposes three kinds of attention mechanisms and prior MASK method. First, the scheme uses frame features to extract the key frames of the video, and then extracts the video from Faster R-CNN and the residual network. Frame features to obtain features and object labels in key frames of the video, use word2vec and LSTM to encode the problem, and merge the extracted video features, video labels and problem text features into the above-mentioned prior MASK attention mechanism In the model, the answer to the question is finally obtained. The model in this paper participated in the Tianchi ZJB competition and finally won the championship. At the same time, it is compared with the existing methods at the end of this paper. From a large number of experiments, it can be proved that this method is more superior than the existing methods.
The video question answering scheme of graph attention mechanism of prior MASK uses graph data structure to express the relationship between video objects and objects. Faster R-CNN is used to extract the coordinates and category of the objects in the key frames of the video. The node attention mechanism and edge attention mechanism are used to construct the graph as nodes, and then the problem features and graph features are used to embed the results. In the prior MASK, the final answer of the model is obtained. The experimental results show that the graph nodes greatly reduce the amount of parameters of the network model, but the accuracy is not lost. In some scenarios where the accuracy requirements are not very high but the speed requirements are high this scheme can be used.
Keywords: video question answering; deep learning; prior MASK; attention mechanism; graph attention
目录
摘 要
Abstract
第1章 绪论
1.1 课题研究的背景以及意义
1.2 视频问答的国内外研究现状
1.3 课题的来源及研究内容
1.4 论文的组织结构与内容安排
第2章 深度学习基础知识
2.1 有监督学习
2.1.1 目标函数
2.1.2 优化算法
2.1.2.1 随机梯度下降(SGD)
2.1.2.2 Adagrad算法
2.1.2.3 RMSprop算法
2.1.2.4 Adam算法
2.2 卷积神经网络 CNN
2.2.1 卷积层
2.2.2 池化层
2.2.3 Dropout
2.3 递归神经网络 RNN
2.3.1 “Vanilla” RNN
2.3.2 LSTM
2.4 注意力机制
2.5 本章小结
第3章 视频问答相关技术概述
3.1 图像与视频
3.1.1 基本术语
3.1.2 视频消除冗余
3.1.2.1 视觉特性
3.1.2.2 时间上的重复
3.1.2.3 图像内的重复
3.2 视觉问答
3.2.1 图像问答
3.2.2 文本问答
3.2.3 视频问答
3.3 公开数据集
3.3.1 视频问答公开数据集
3.3.2 ZJB问答数据集
3.4 本章小结
第4章 先验MASK的多注意力机制的视频问答方案
4.1 对视频和问题进行处理(编码)
4.1 视频编码表示
4.1.1.1 关键帧
4.1.1.2 ResNet-101和Faster-RCNN
4.1.1.3 自底向上注意力
4.1.2 问题编码表示
4.1.2.1 对问题进行NLTK处理
4.1.2.2 对问题进行LSTM编码表示
4.2 评价标准
4.3 先验MASK的多注意力机制模型
4.3.1 视频与问题文本注意力temporal-attention
4.3.2 视频与视频对象标签注意力 attr-block-attention
4.3.3 问题与对象标签注意力 time-spatial-attention
4.3.4 先验MASK
4.3.5 答案生成
4.3.6 Loss函数选择
4.4 实验评估
4.4.1 3.6.1 模型参数以及环境设置
4.4.2 模型对比实验分析
4.4.3 实例展示
4.5 本章小结
第5章 先验MASK的图注意力机制的视频问答方案
5.1 相关工作
5.2 图注意力网络
5.2.1 节点选择
5.2.2 图构建
5.2.3 图嵌入
5.2.4 先验MASK
5.3 实验
5.4 本章小结
第6章 总结与展望
6.1 全文总结
6.2 未来工作与展望
致谢
参考文献
在读期间发表的学术论文与取得的研究成果
论文
竞赛成果
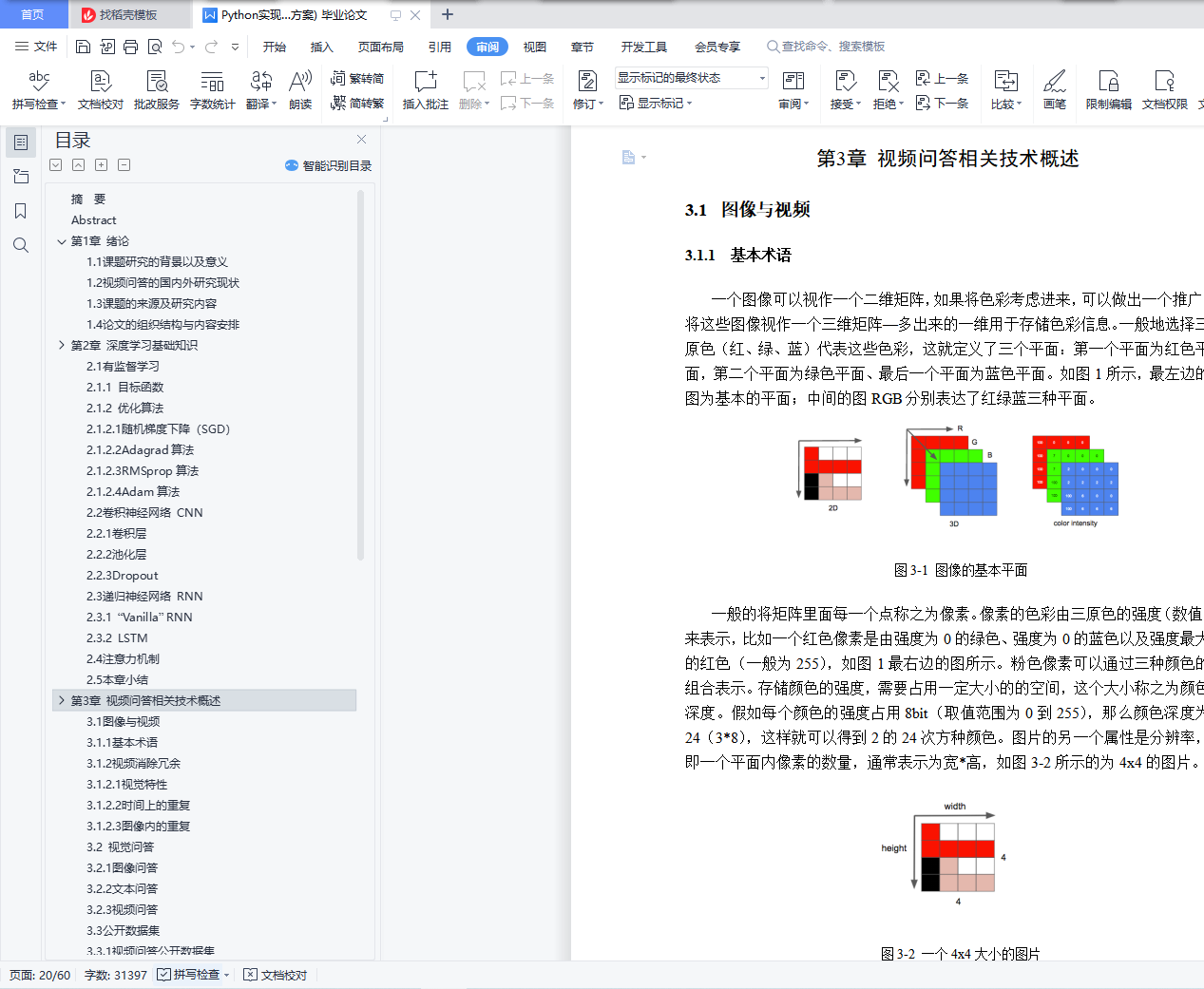
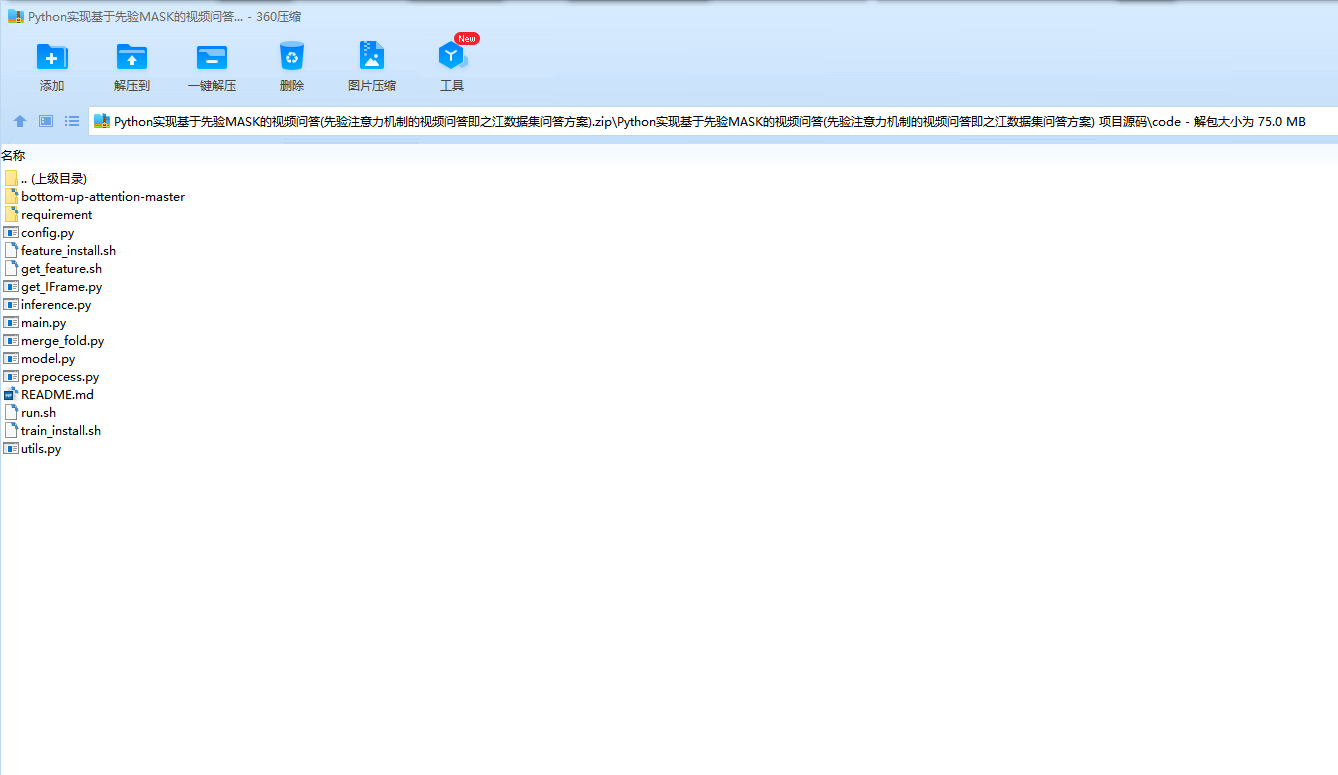
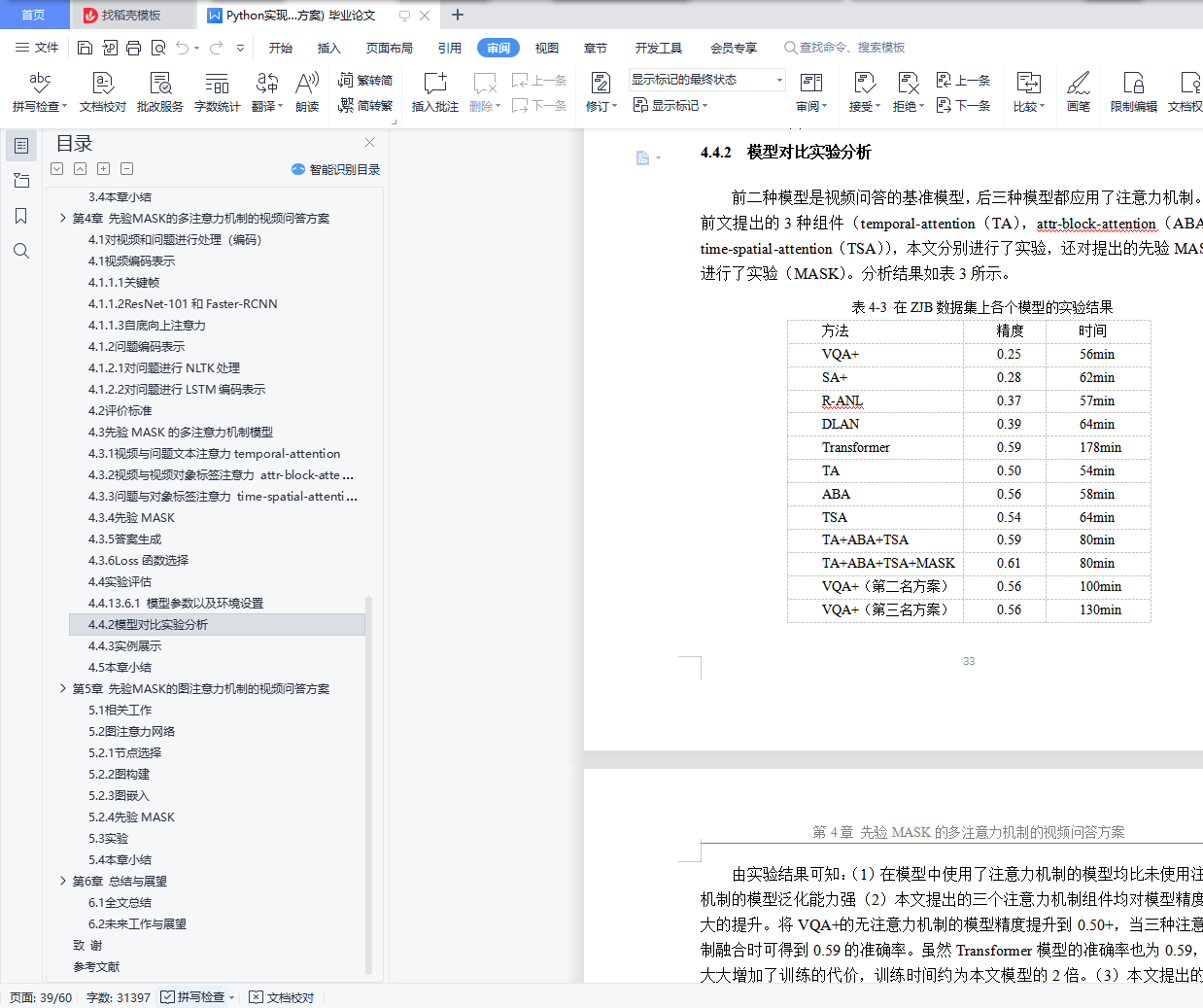
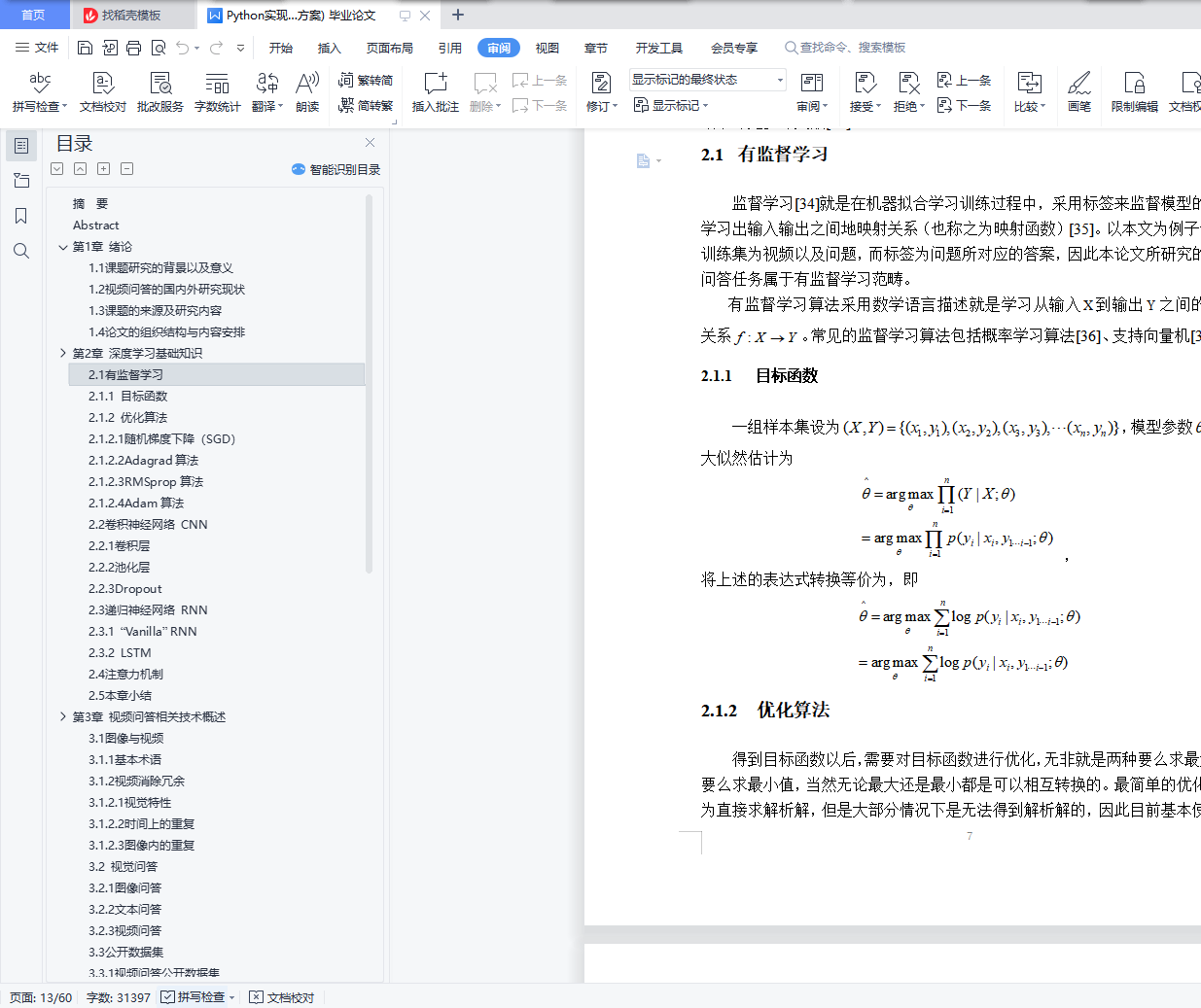
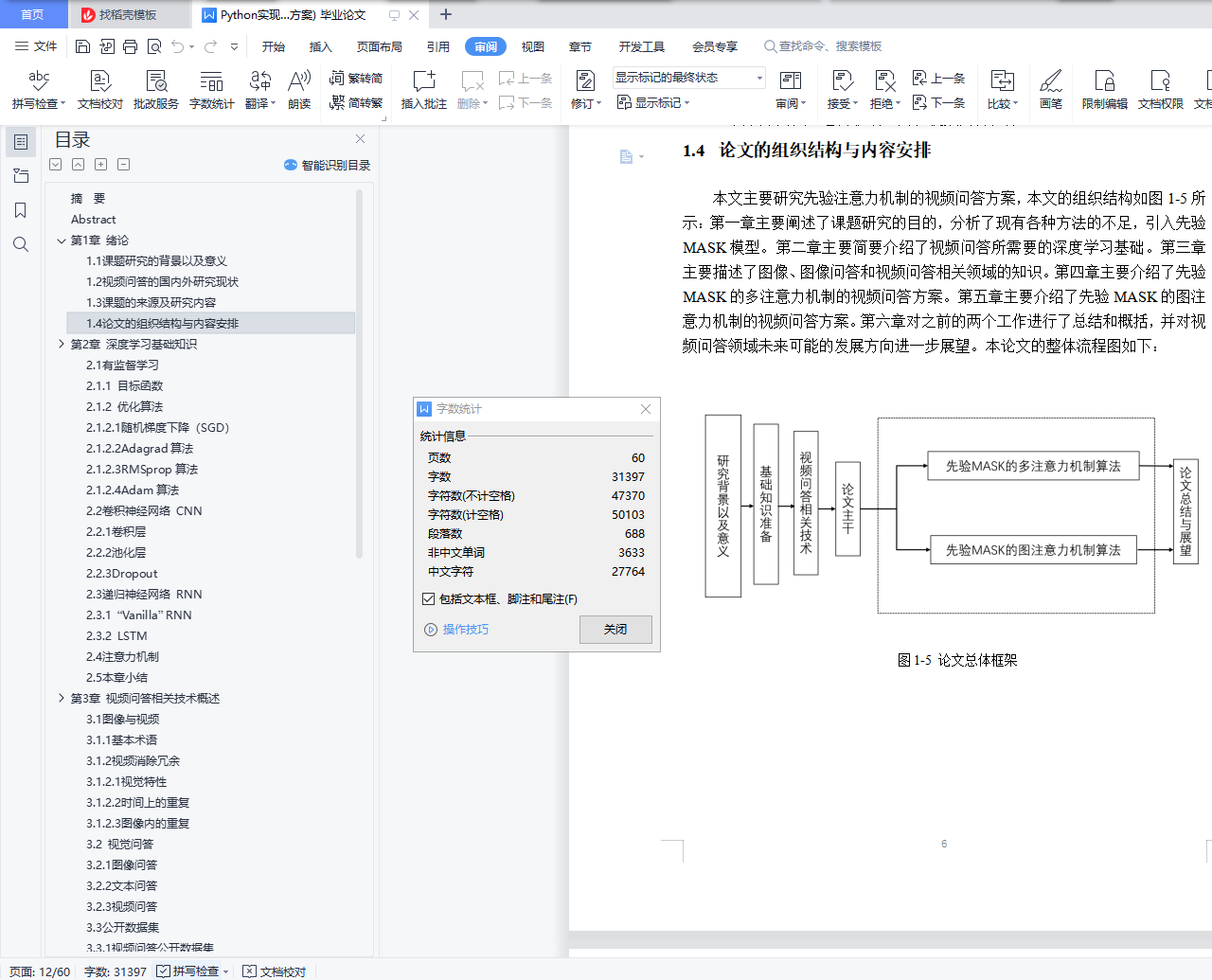
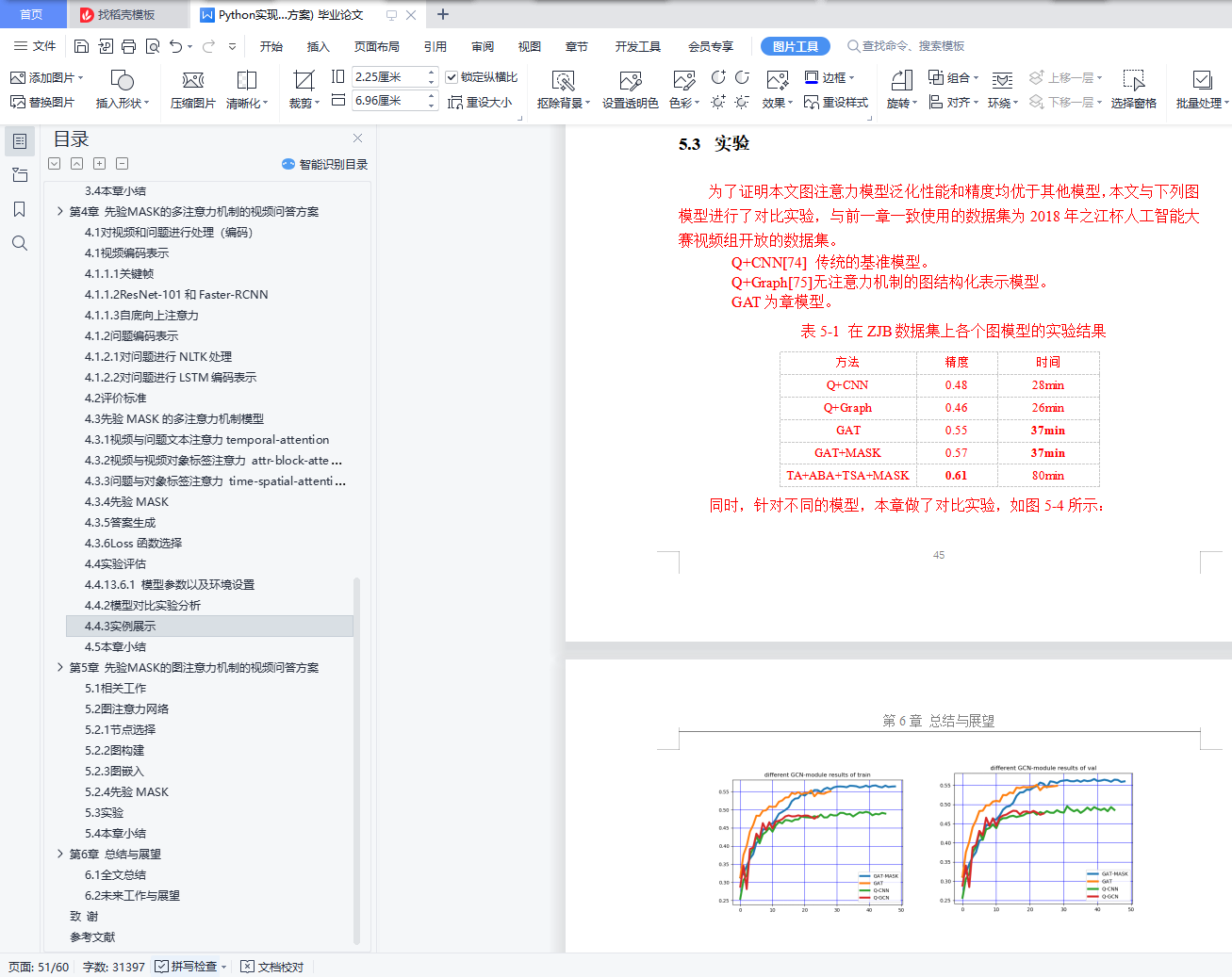
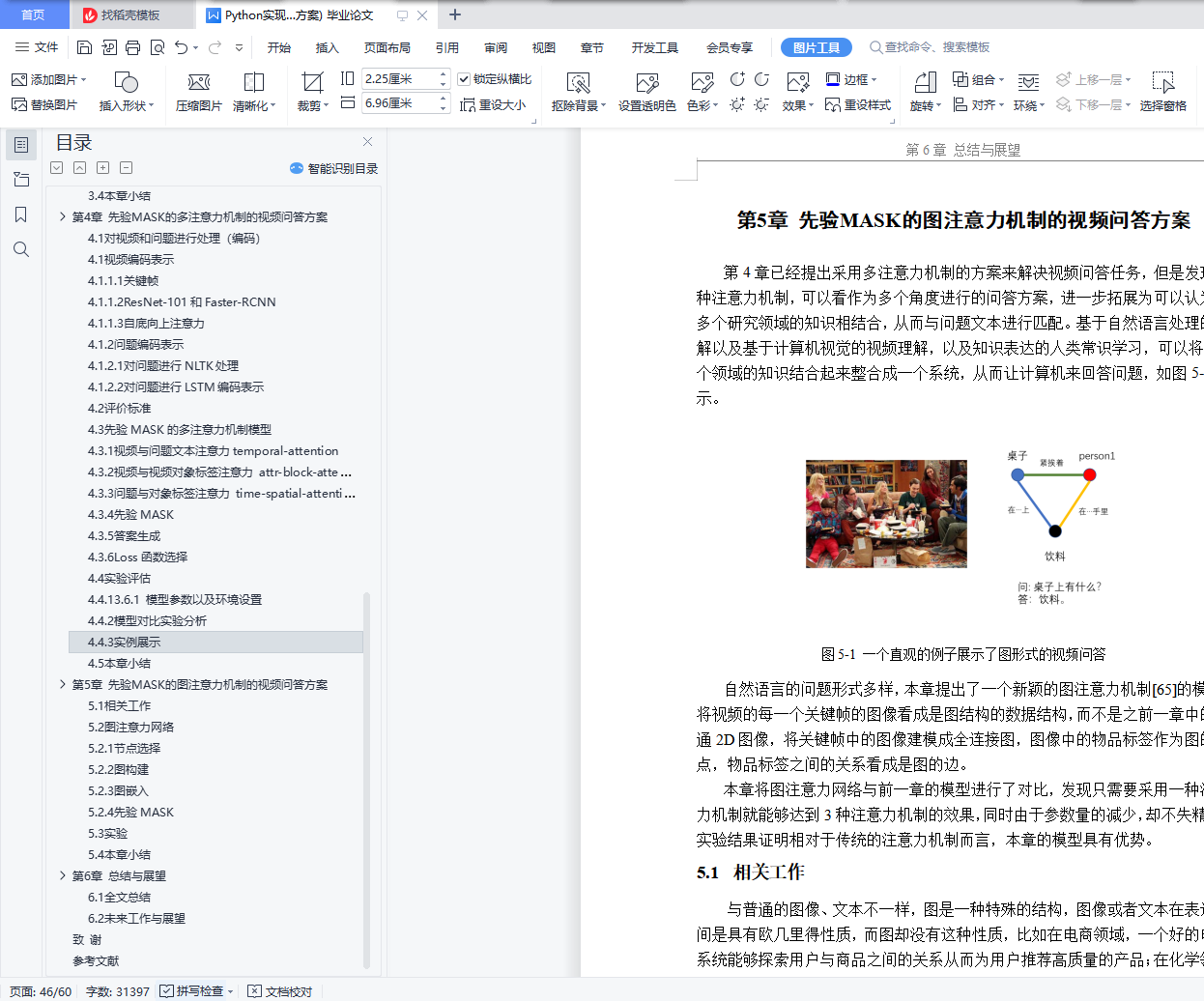
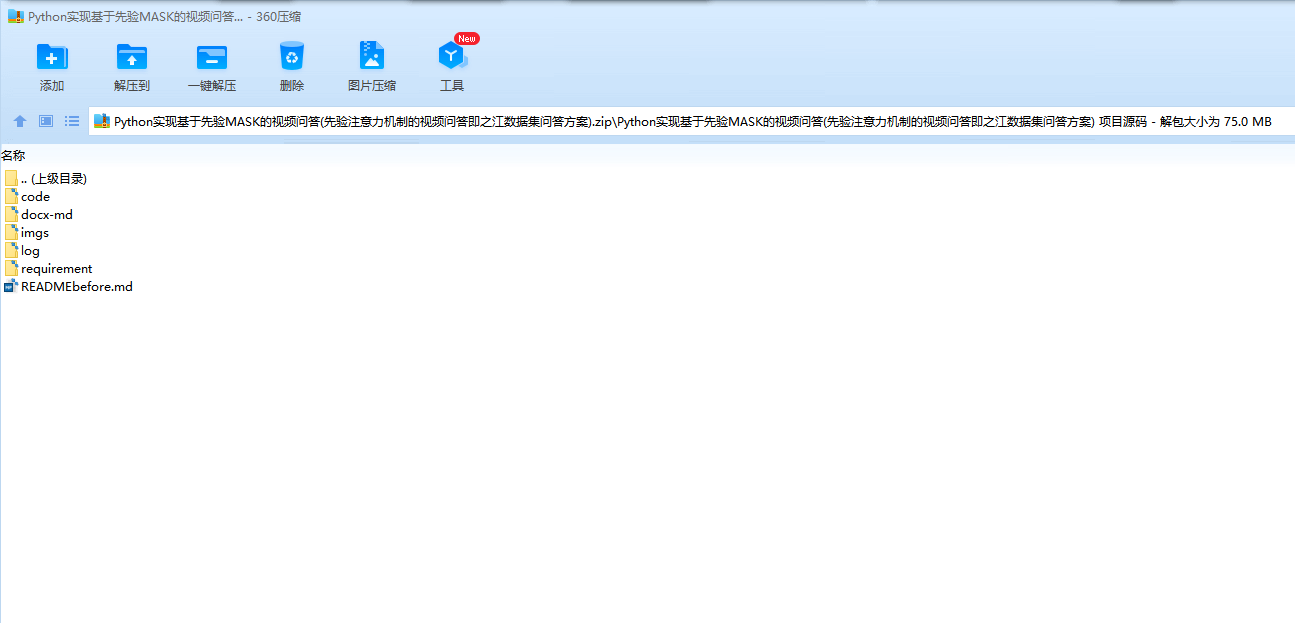
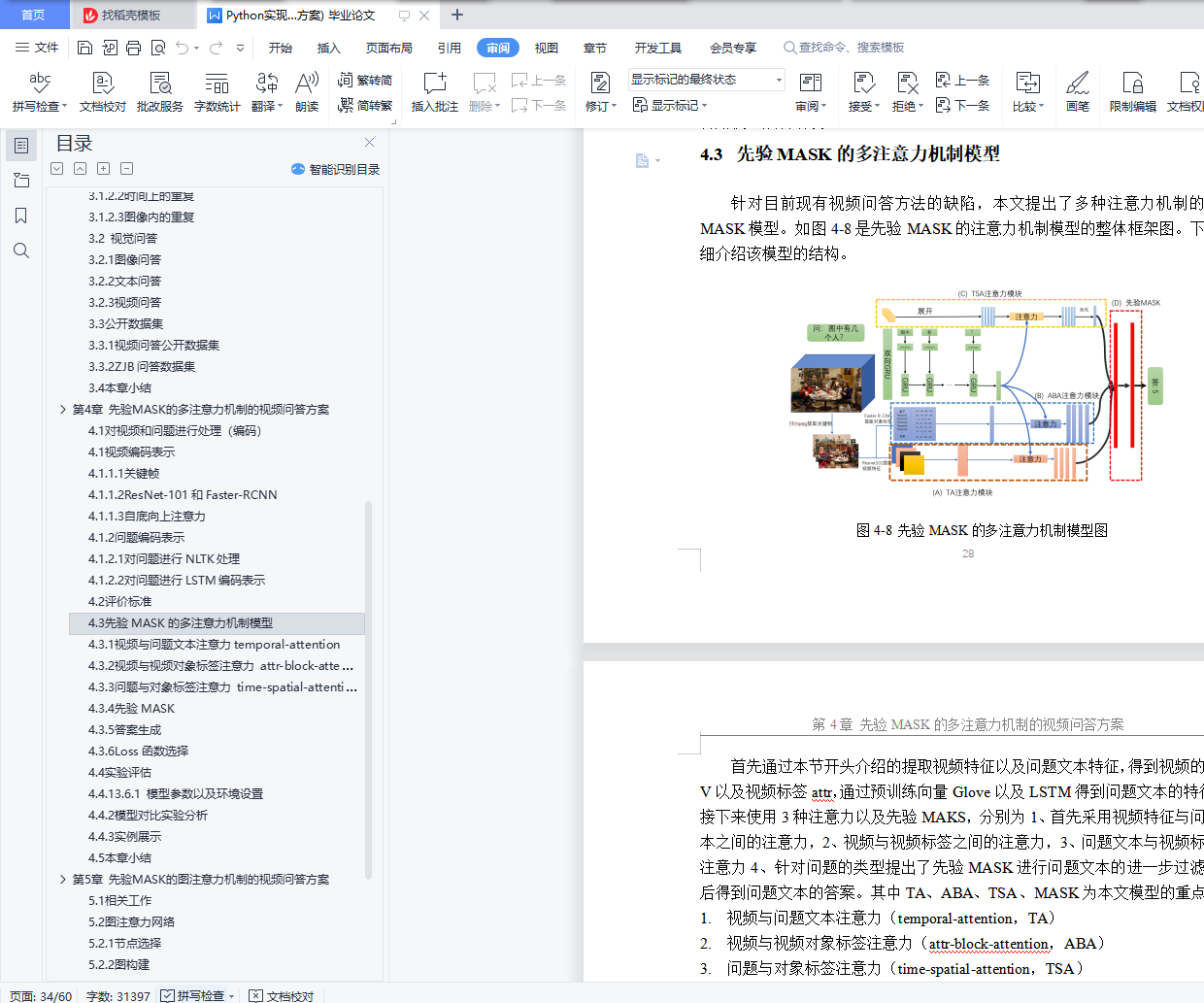
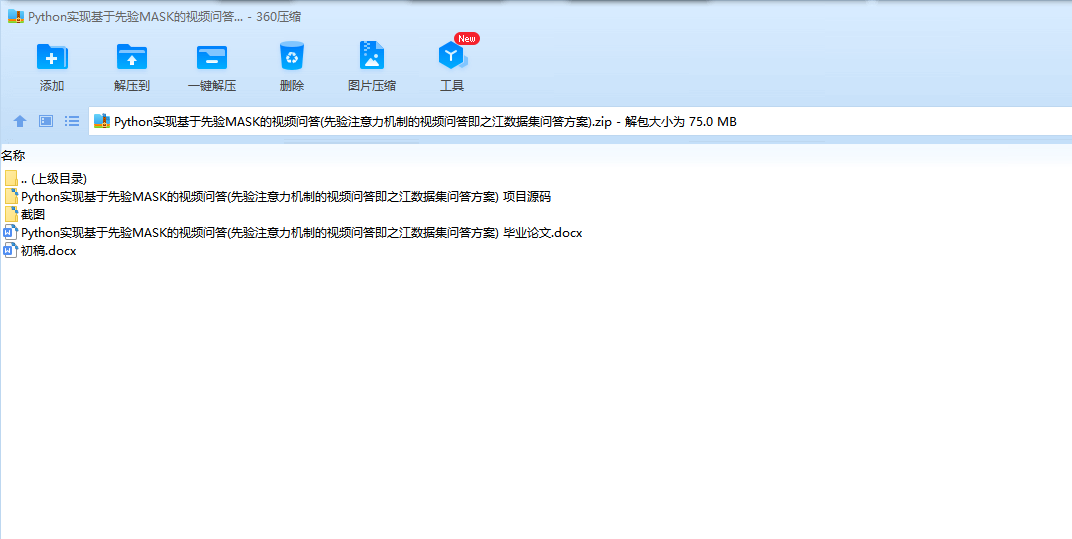
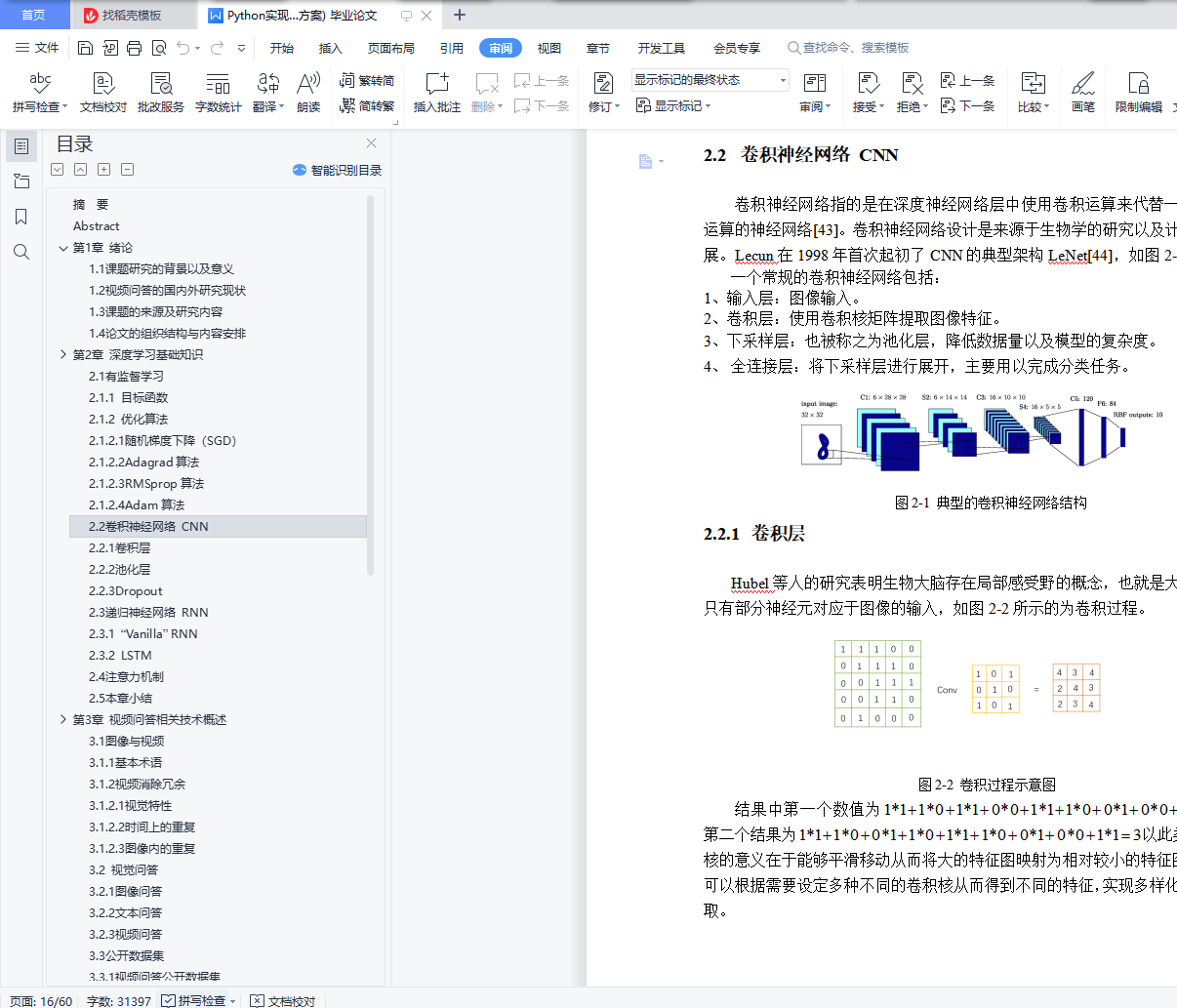
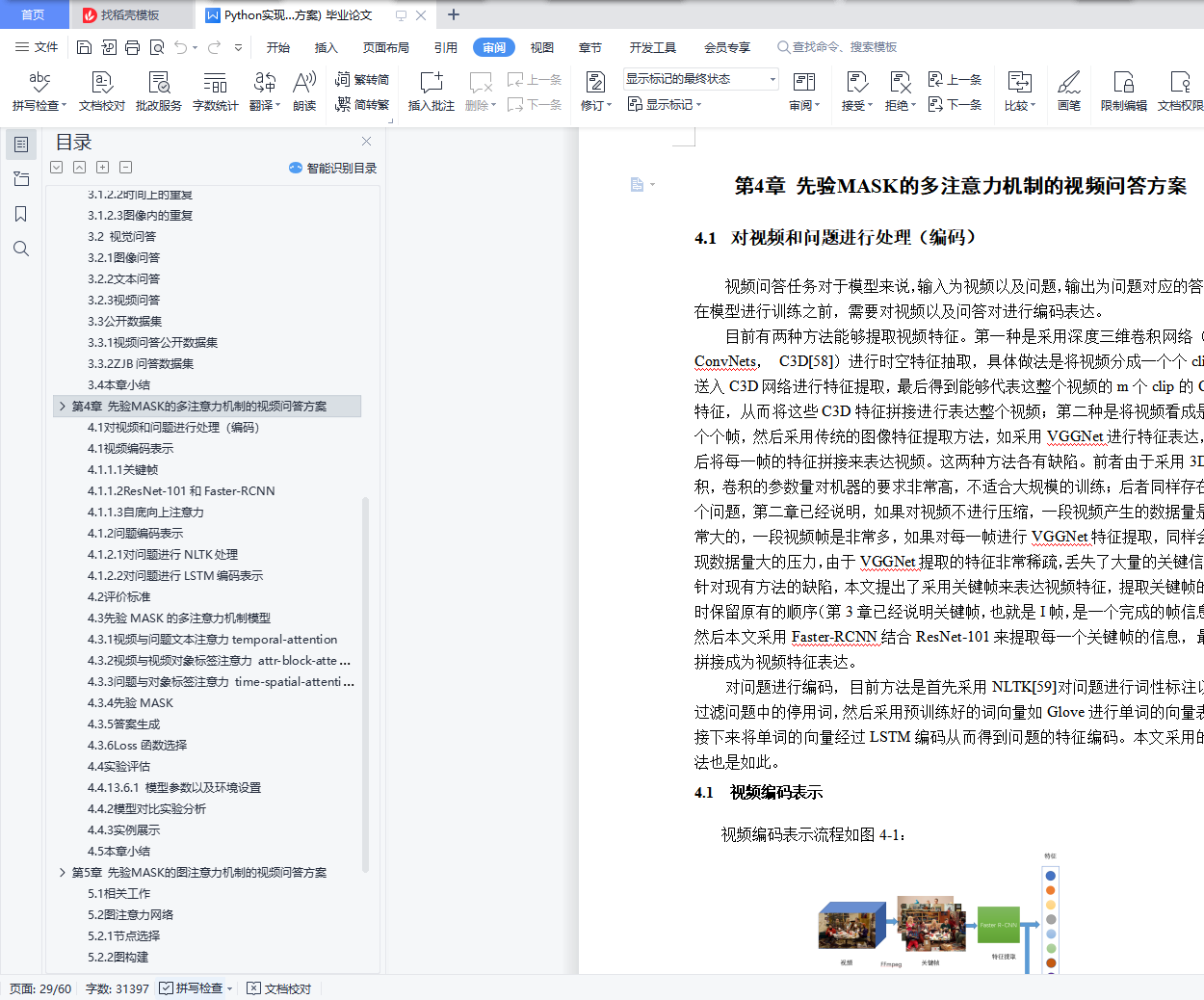
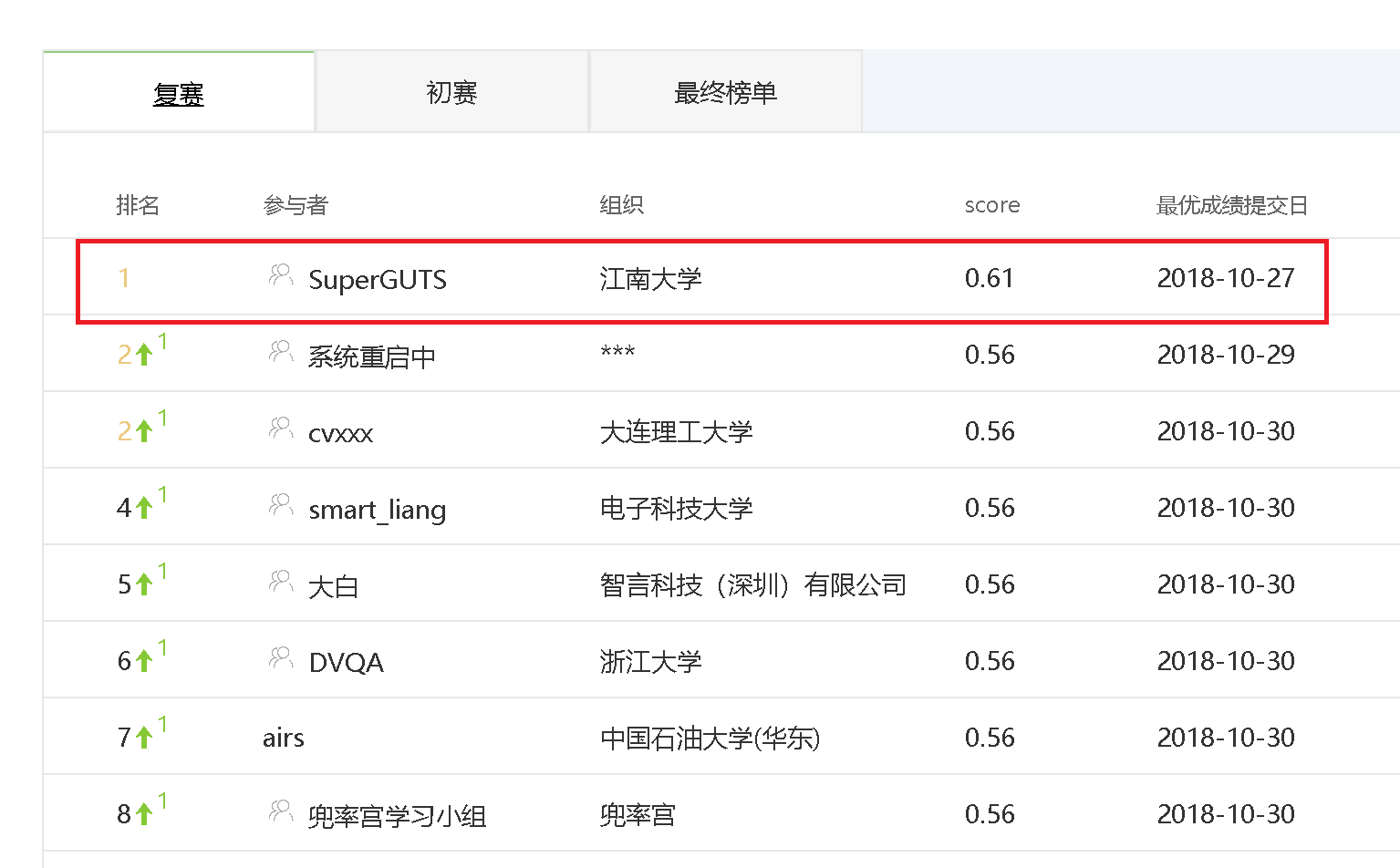
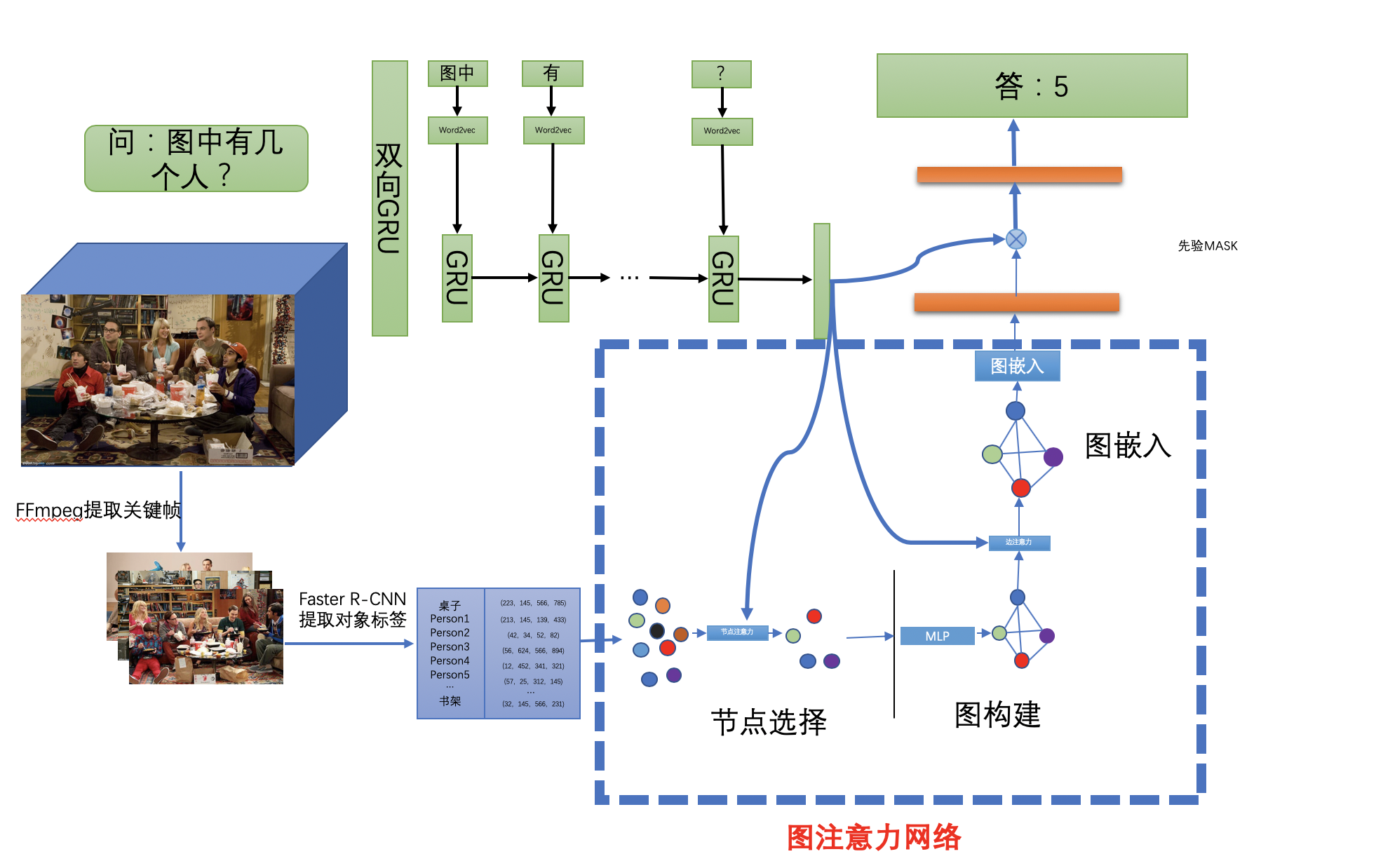
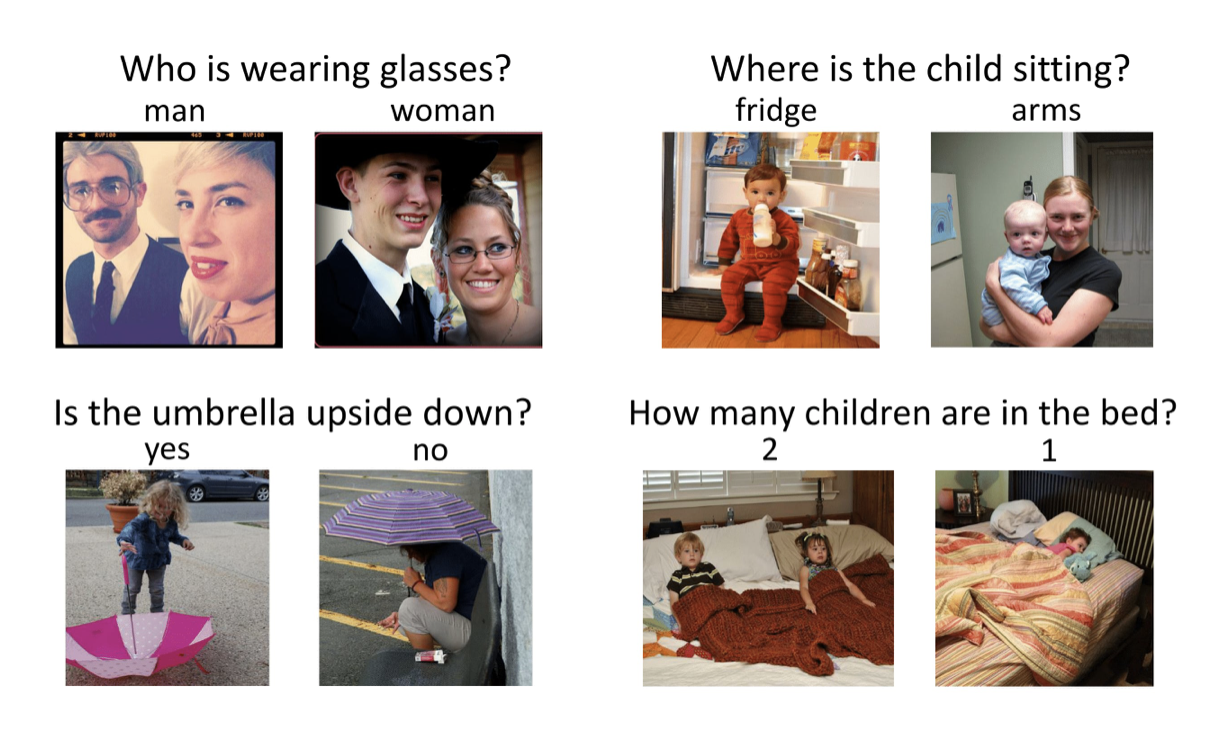
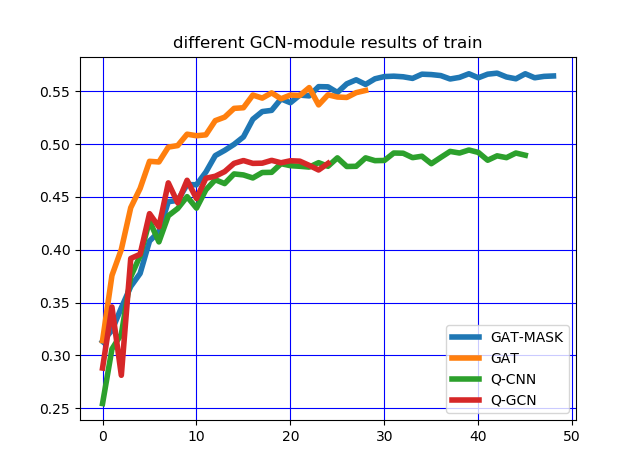
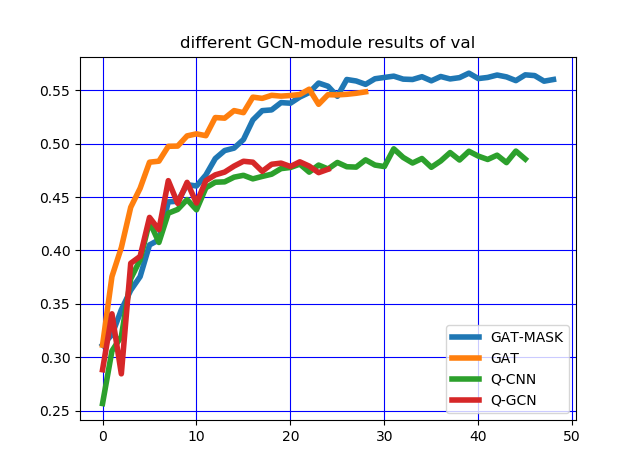