中文摘要
近年来,三维场景重建与定位是计算机视觉领域中重要的研究方向。随着自动驾驶技术与工业机器人技术的不断发展,对于场景重建精度与定位准确度的要求也不断提高。如何利用各种传感器采集到的数据,完成对场景的精确重建与定位,是非常有价值和应用前景的研究方向。
目前这一领域中存在着许多挑战:在重建方面,使用传统方法对大场景一次性建图会产生漂移误差,同时效率较低,而采用分区域重建的方法又依赖于准确的融合技术;在定位方面,由于光照环境的变化,定位时刻的环境细节特征与地图重建时刻存在差异,在匹配上存在难度;同时,基于传统单目相机的视觉定位的视场较小,有时无法捕捉到足够多的特征进行定位。这些挑战共同限制了建图的精确性与定位的鲁棒性。
针对以上挑战,本文提出了一种重建与定位流程:首先利用传感器数据,生成场景局部点云,然后对局部点云进行拼接融合,合成整体场景高精度点云,最后利用全景相机实现在场景中的定位。
本文主要的研究内容包括:
1.采用了三个平行的局部重建算法:基于视觉的SfM算法、基于激光雷达的LOAM算法与基于图像激光扫描仪的方法,完成对清华园局部场景的重建任务,为针对不同环境的重建任务在设备和算法选择上提供了参考;
2.提出一种由粗到精的点云配准算法,对局部场景点云进行融合,生成全局高精度点云。该算法融合了传统特征提取与神经网络的方法,能够在不依赖于初始转移矩阵的情况下具有较为鲁棒的配准结果。
3.提出一种跨模态鲁棒匹配的视觉定位算法,完成了基于先验点云场景中的定位任务。该算法通过将2D-3D匹配问题转换为2D-2D匹配问题,能够在仅使用全景相机,且不预先对场景进行任何布置的情况下实现定位。
关键词:三维重建;定位;点云配准;神经网络;高精度地图
ABSTRACT
Inrecentyears,3Dscenereconstructionandlocalizationisanimportantresearchdirectionincomputervisionfield.Withthecontinuousdevelopmentofautonomousdrivingtechnologyandindustrialrobottechnology,therequirementsfortheaccuracyofreconstructionandlocalizationarealsoincreasing.Therefore,howtousethedatacollectedbyvarioussensorstoaccuratelyreconstructthesceneandlocateinit,isaveryvaluableandpromisingresearchdirection.
Atpresent,therearemanychallengesinthisfield.Intheaspectofreconstruction,thetraditionalmethodwillproducedrifterrorandlowefficiencyinthereconstructionoflargesceneinonetime,whilethemethodofsubregionalreconstructionreliesonac-curatefusiontechnology.Intheaspectoflocalization,duetothechangeofilluminationenvironment,thedetailedfeaturesofthelocationtimearedifferentfromthoseofthemapreconstructiontime,soitisdifficulttomatchthem.Futhermore,thevisuallocal-izationtechonologybasedontraditionalmonocularcamerahasasmallfieldofview,sosometimesitcannotcaptureenoughfeaturesforlocalization.Thesechallengestogetherlimittheaccuracyofmappingandtherobustnessoflocalization.
Aimingattheabovechallenges,areconstructionandlocationprocessisproposedinthisthesis.Firstly,localpointcloudsaregeneratedfromsensordata,andthenthelocalpointcloudsaresplicedandfusedtosynthesizehigh-precisionpointcloudsofthewholescene.Finally,thepanoramiccameraisusedtorealizethelocationinthescene.
Themainresearchcontentsofthisthesisinclude:
1.Threeparallellocalreconstructionalgorithmsareadopted:visualbasedSFMalgorithm,lidarbasedLOAMalgorithmandimage-laserscannerbasedmethod,tocom-pletethereconstructiontaskofthelocalsceneofTsinghuaUniversity,whichprovidesareferencefortheselectionofequipmentandalgorithmforreconstructiontasksindif-ferentenvironments;
2.ACoarse-to-finepointcloudregistrationalgorithmisproposedtointegratethelocalpointcloudsandgeneratetheglobalhigh-precisionpointcloud.Thisalgorithmcombinesthetraditionalfeatureextractionandneuralnetworkmethods,andcanachieverobustregistrationresultswithoutrelyingontheinitialtransfermatrix.
3.Across-modalrobustmatchingvisionlocationalgorithmisproposedtoaccom-plishthelocationtaskbasedonaprioripointcloudscene.Bytransformingthe2D-3Dmatchingproblemintoa2D-2Dmatchingproblem,thealgorithmcanrealizepositioningusingonlythepanoramiccameraandwithoutanylayoutofthesceneinadvance.
Keywords:3Dreconstruction;localization;pointcloudregistration;neuralnet-work;highprecisionmap
目 录
第1章 引言 1
1.1研究背景及意义 1
1.2研究现状 3
1.2.1视觉三维重构 3
1.2.2点云配准 8
1.2.3图像特征描述 12
1.2.4视觉定位 14
1.3本文工作 15
1.4论文结构 17
第2章 局部三维场景重建 18
2.1 引言 18
2.2基于视觉的SfM三维重构 18
2.2.1算法简介 18
2.2.2COLMAP简介 19
2.2.3实验及结果 20
2.3基于激光雷达的LOAM三维重构 21
2.3.1算法简介 21
2.3.2实验设备 22
2.3.3实验及结果 22
2.4基于激光扫描仪的三维重构 23
2.4.1LeicaBLK360 23
2.4.2扫描结果 24
2.5本章小结 25
第3章 基于由粗到精的点云配准 26
3.1 引言 26
3.2点云粗配准 26
3.2.1局部特征描述子 26
3.2.2基于点特征的全局粗匹配 28
3.3基于深度学习的点云精配准 30
3.3.1模型结构 31
3.3.2损失函数 32
3.4实验与结果 33
3.4.1数据采集 33
3.4.2全局点云配准实验 34
3.4.3算法评价 35
3.5本章小结 40
第4章 基于2D-3D的图像-激光跨模态匹配定位 42
4.1 引言 42
4.2点云-平面映射 43
4.2.1虚拟视点图像生成 43
4.2.2实验结果 45
4.3全景畸变校正 46
4.3.1全景成像原理 46
4.3.2畸变校正原理 47
4.3.3实验结果 48
4.4基于SOSNet的图像特征提取与匹配 49
4.4.1网络结构及损失函数 50
4.4.2特征提取与匹配结果 51
4.5基于对极约束的定位算法 53
4.5.1对极约束 53
4.5.2定位实验 54
4.6本章小结 55
第5章 总结与展望 57
5.1工作总结 57
5.2工作展望 58
插图索引 59
表格索引 61
参考文献 62
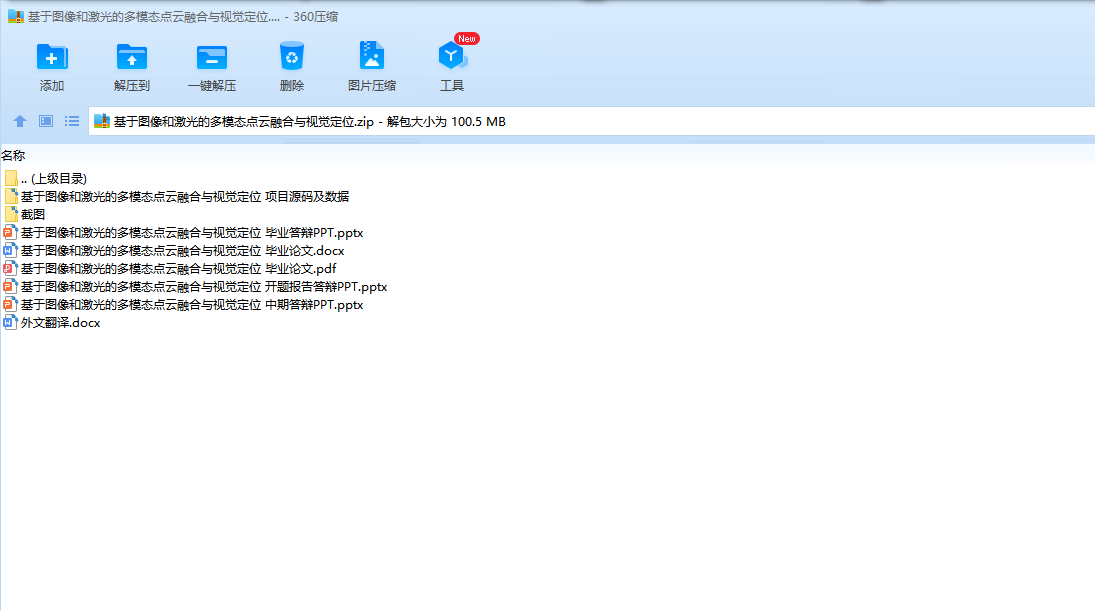
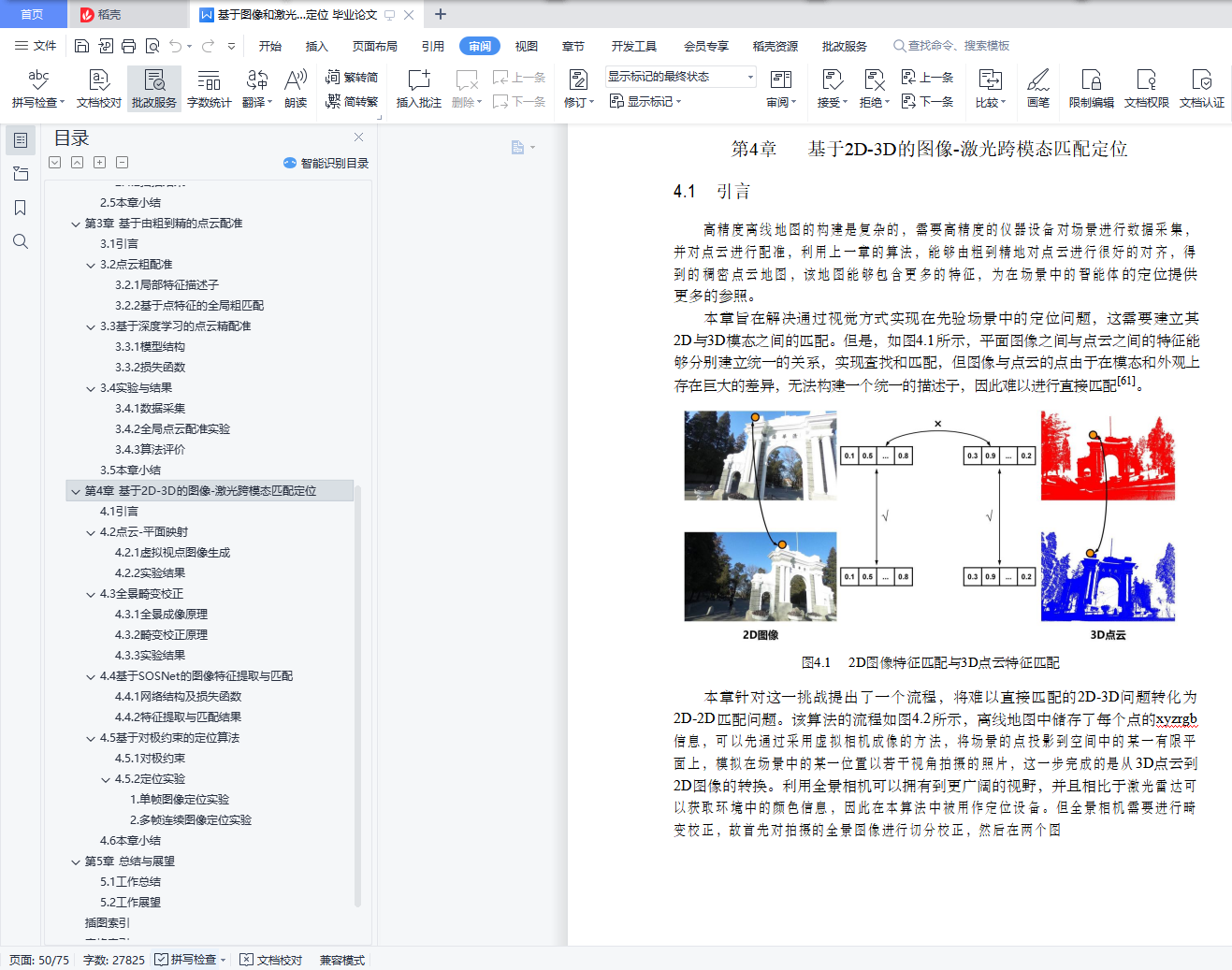
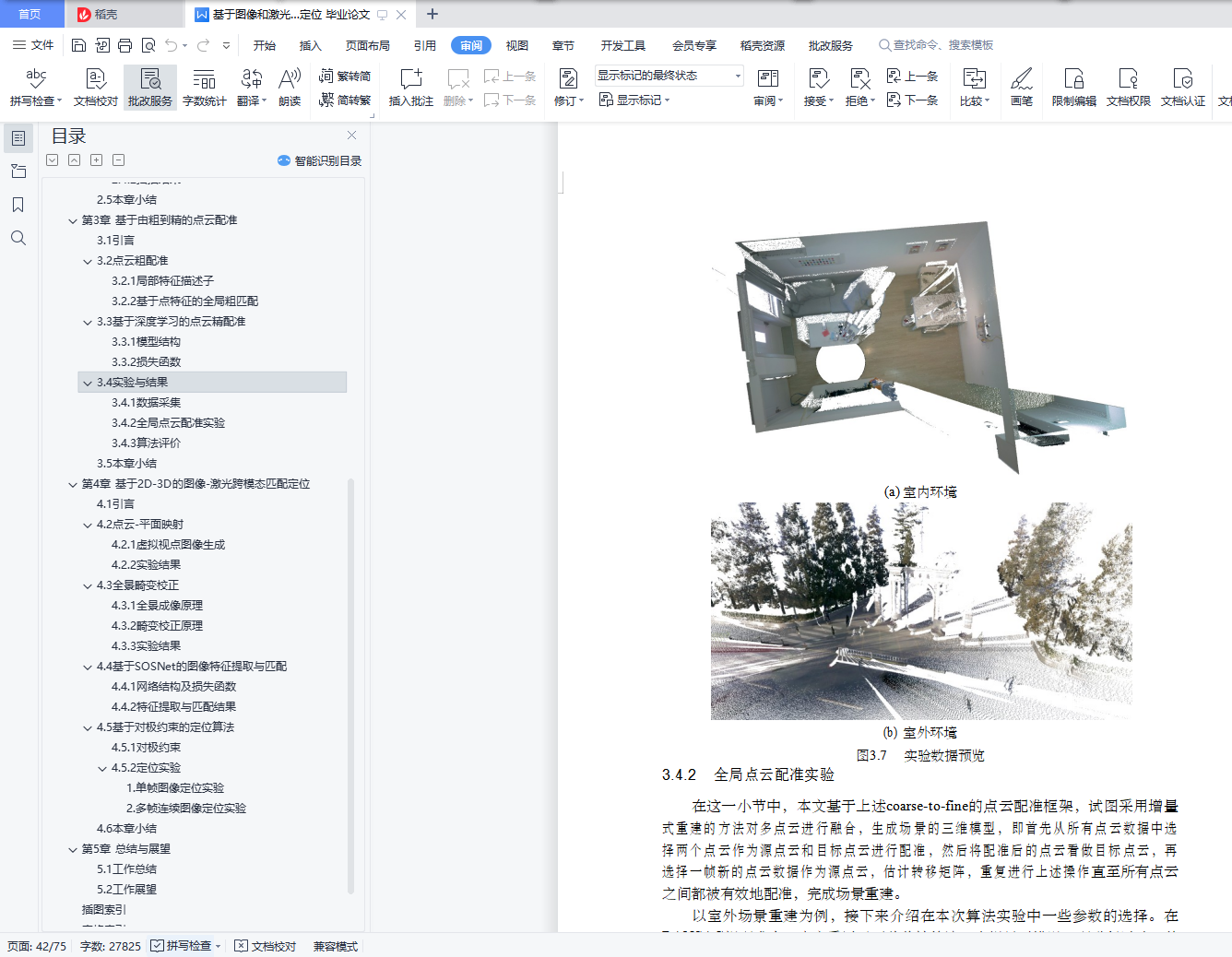
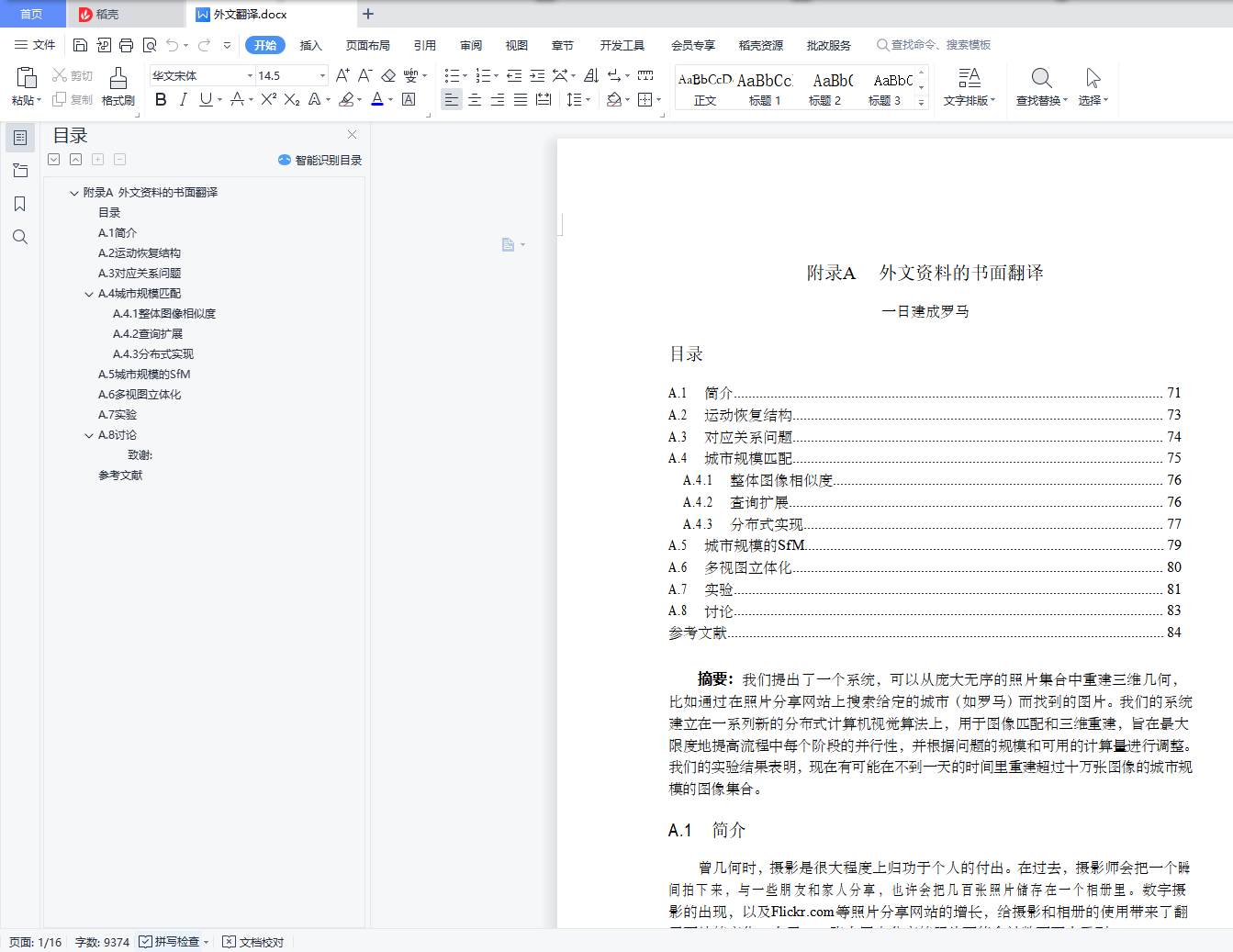
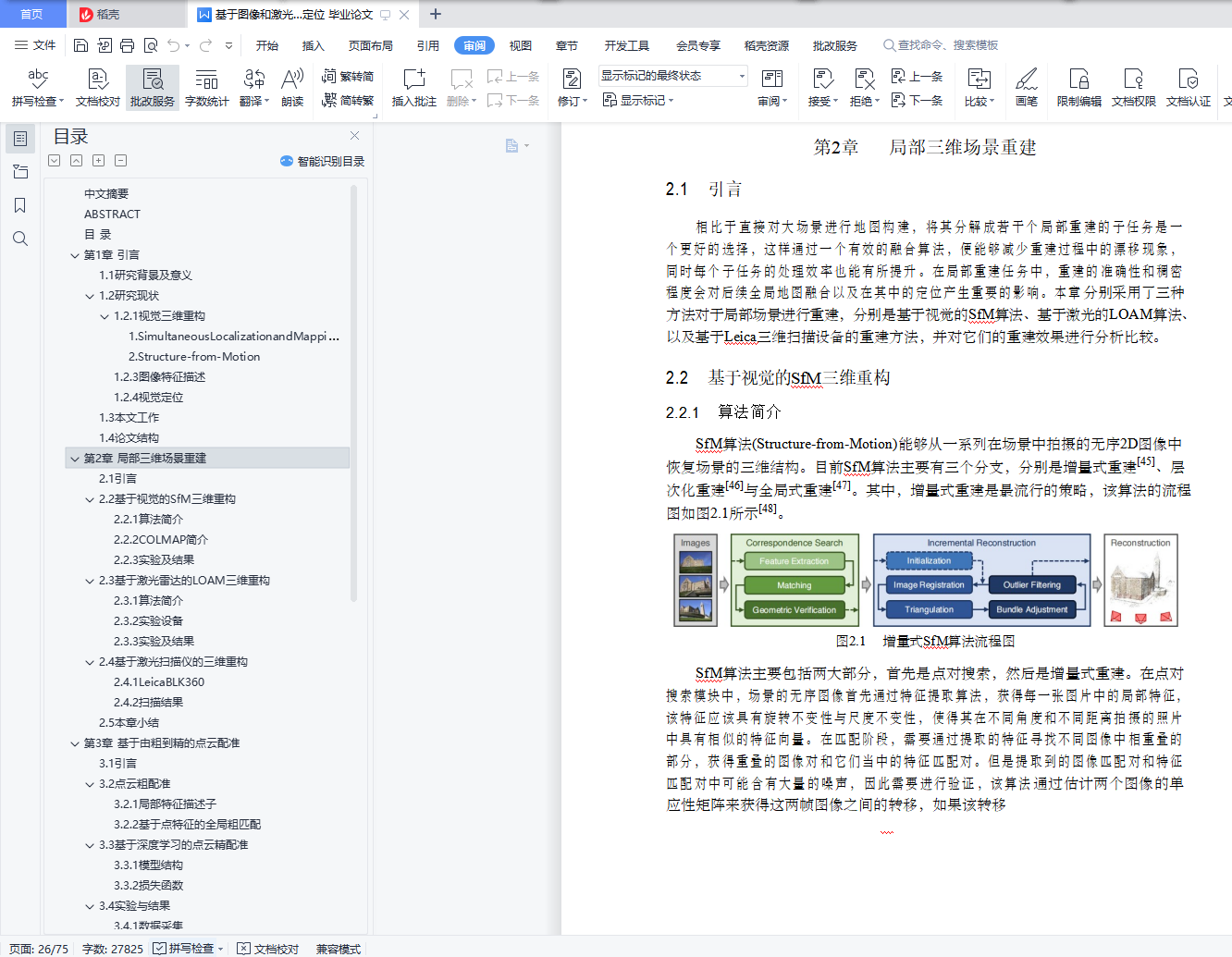
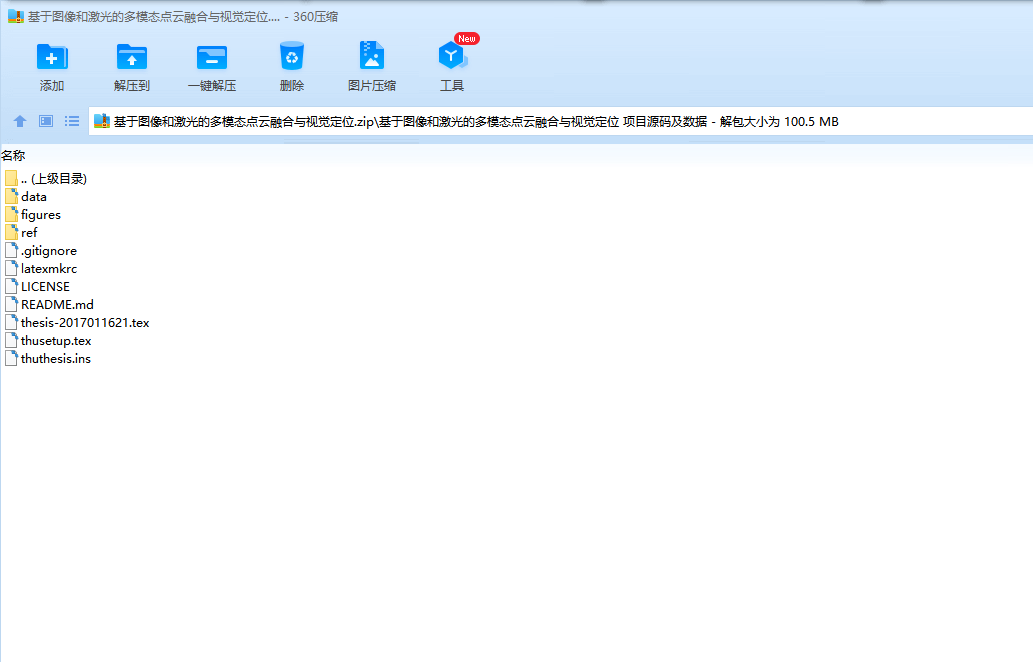
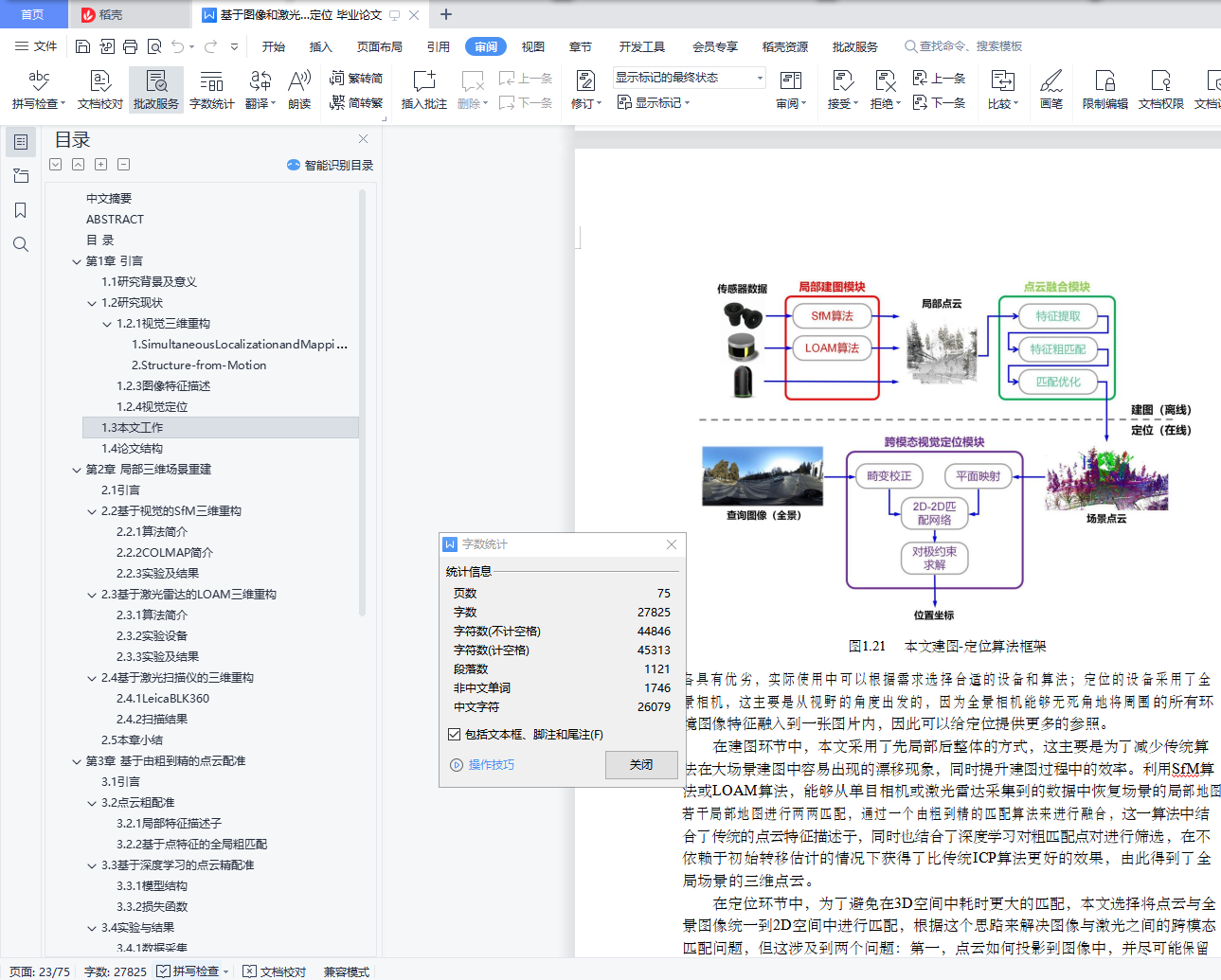
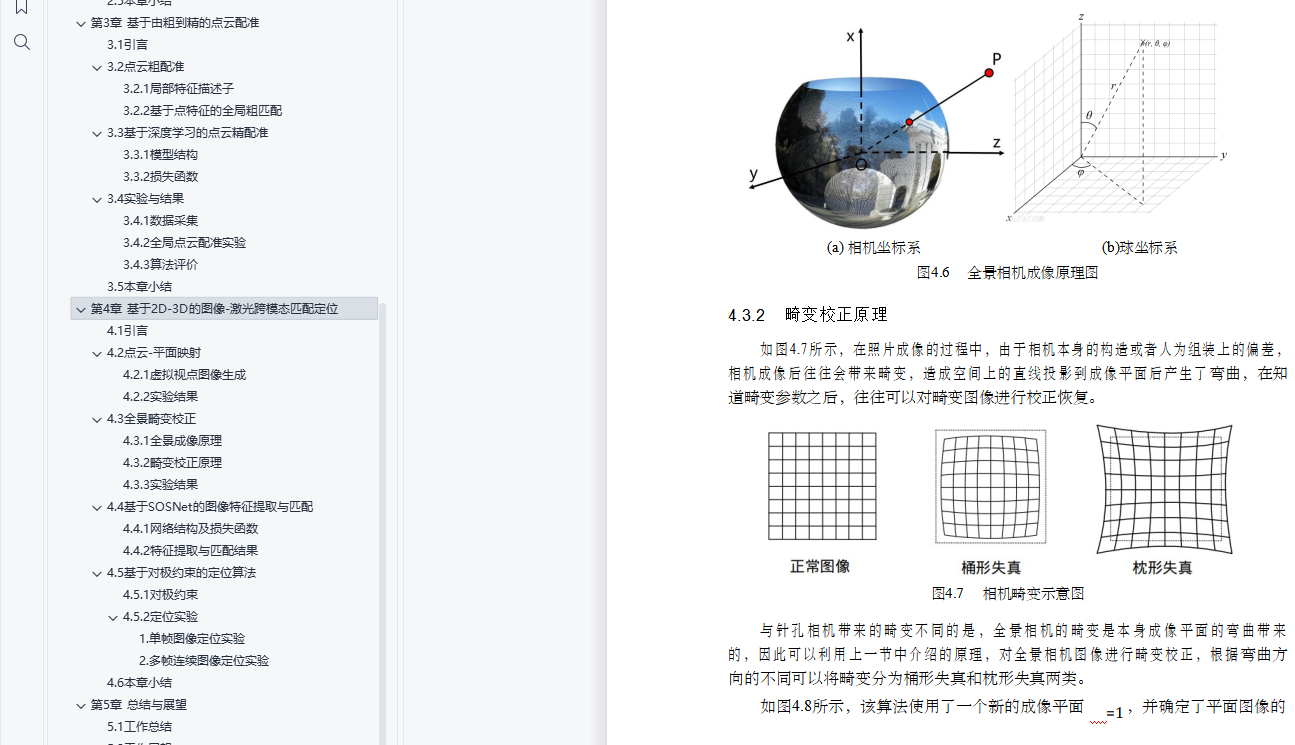
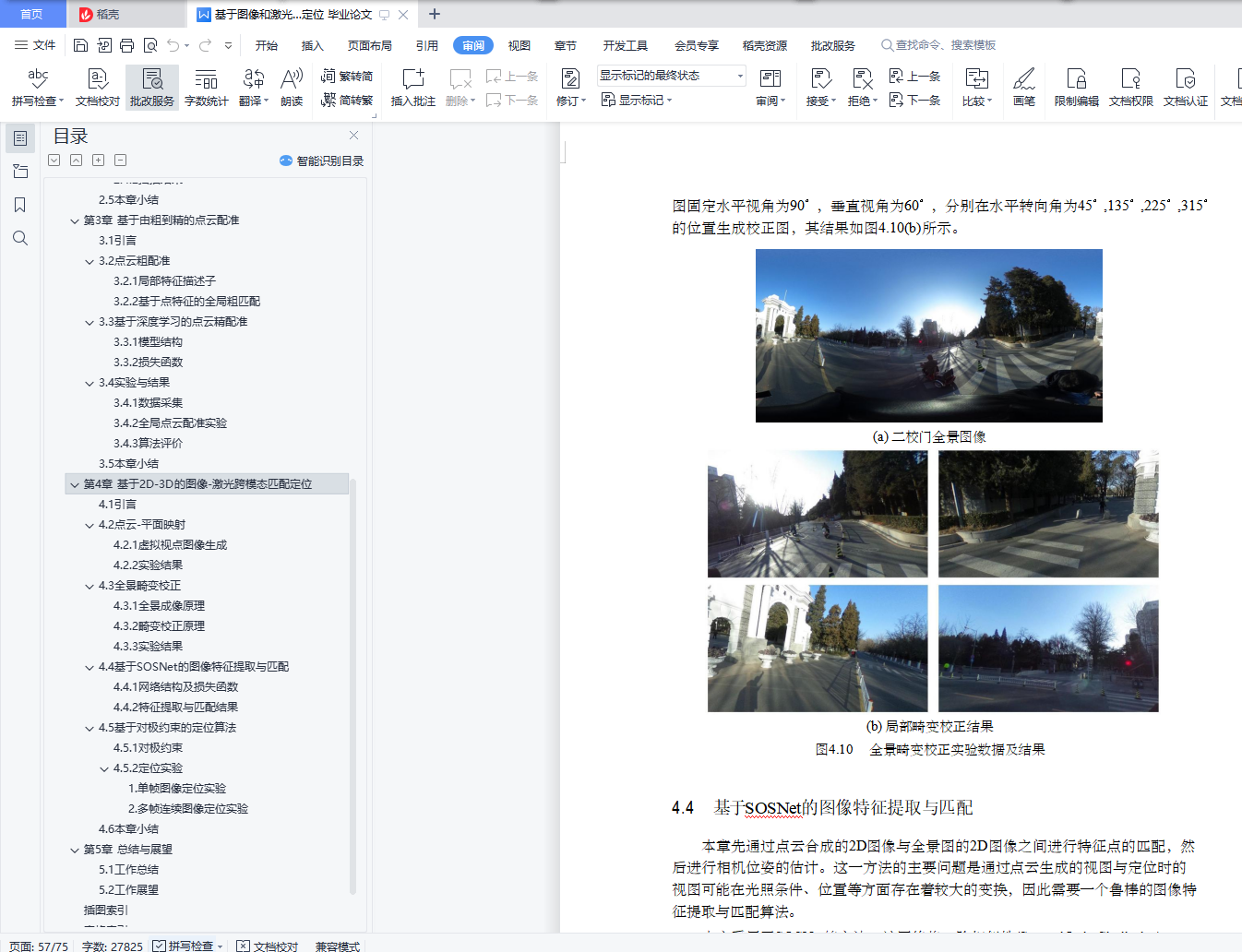
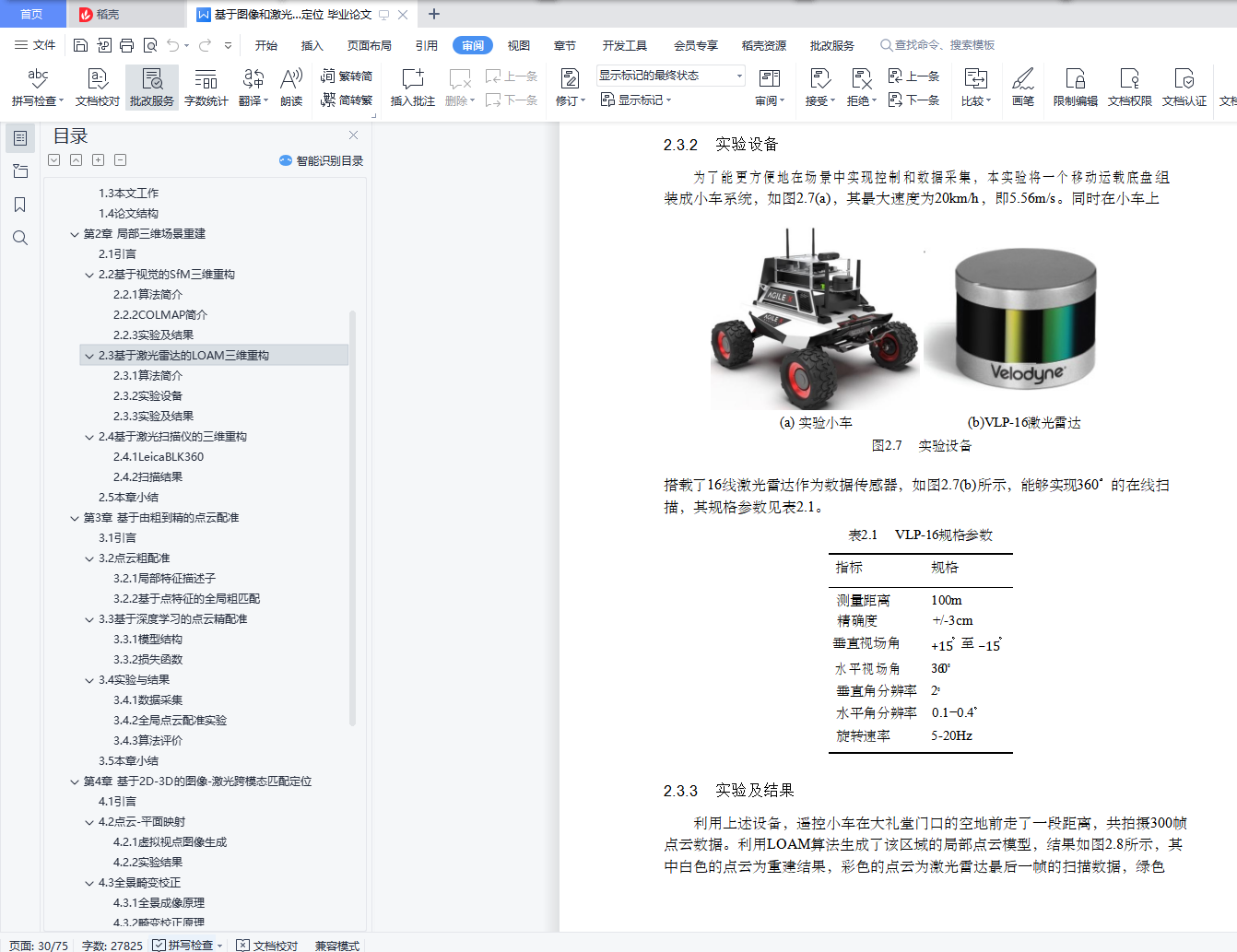
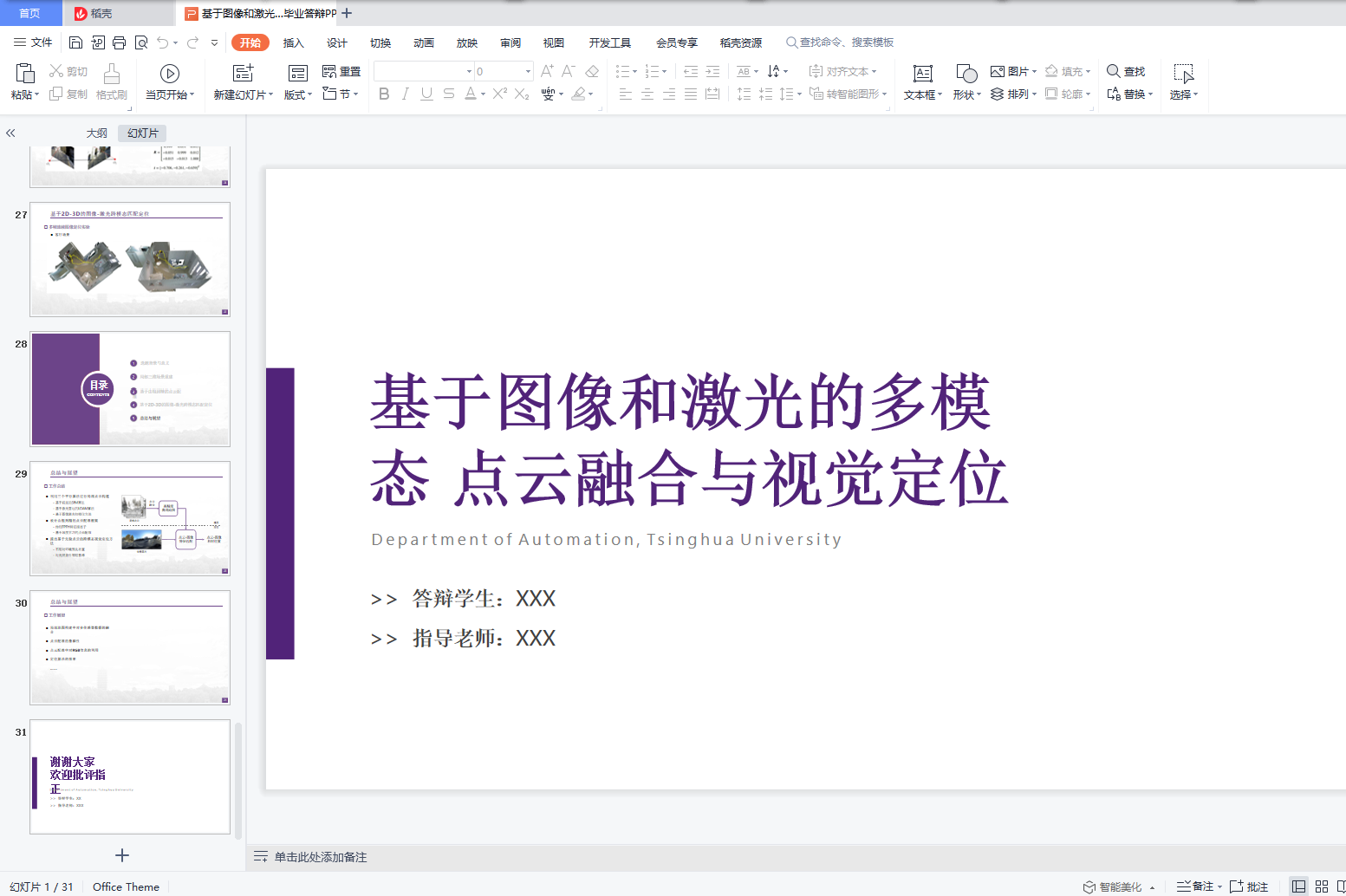
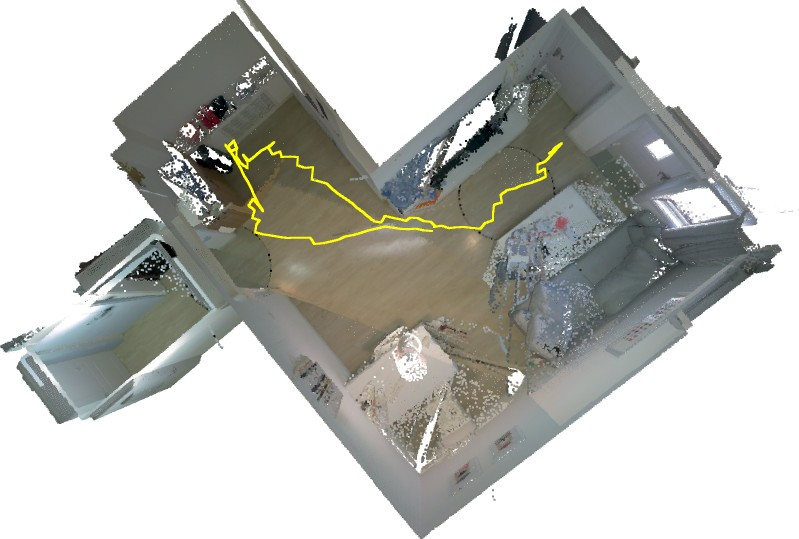
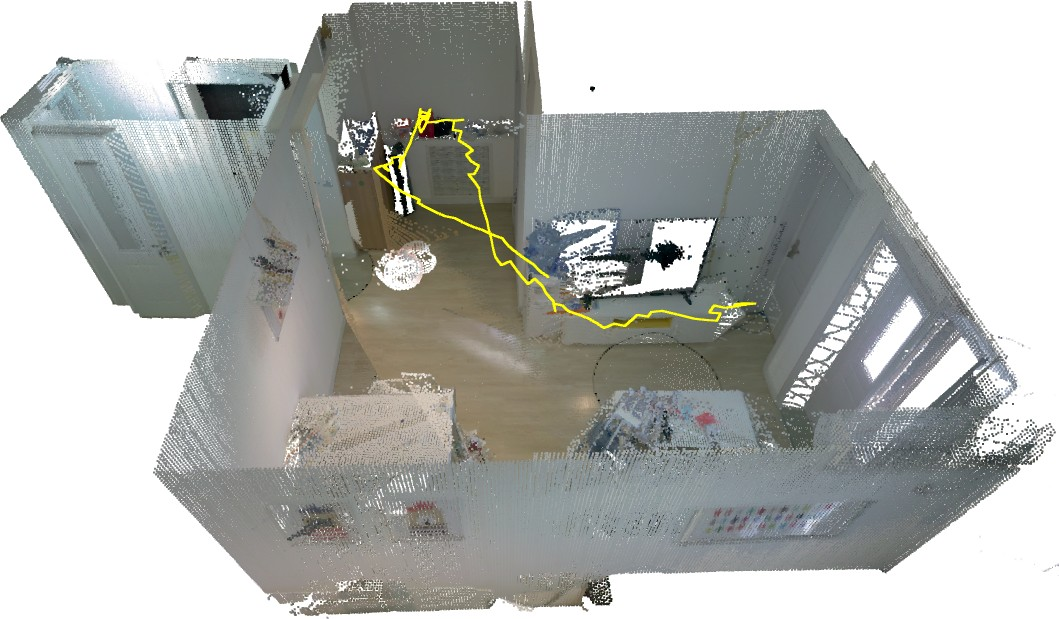
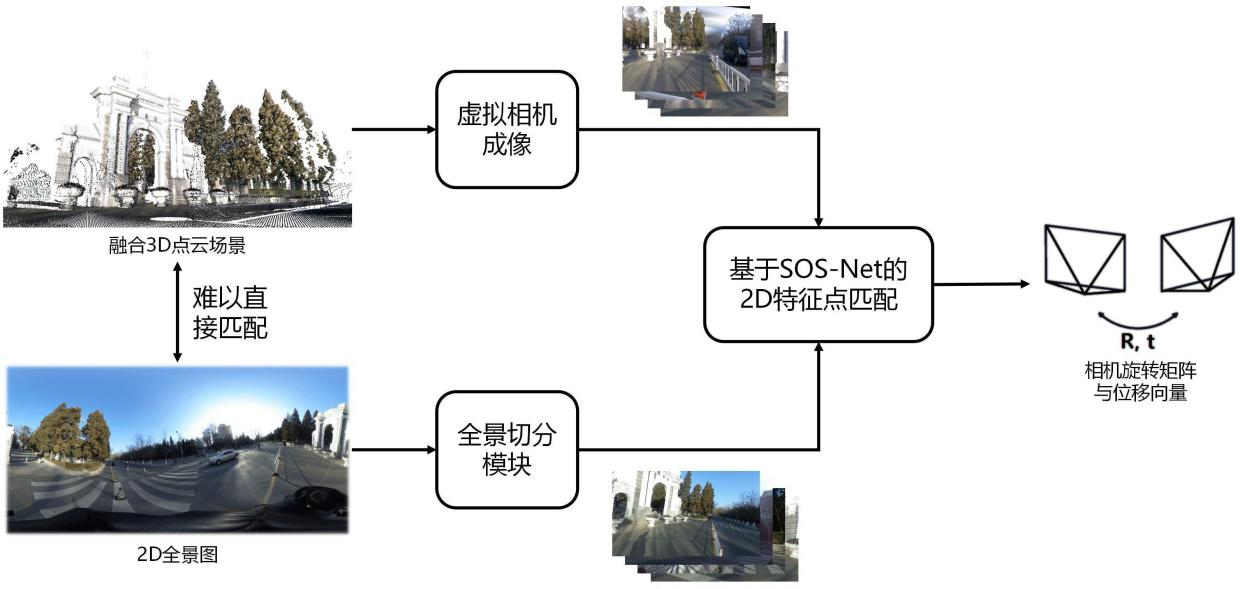
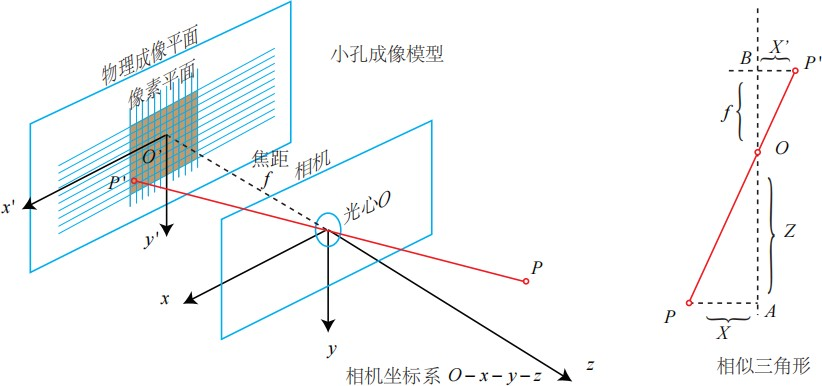
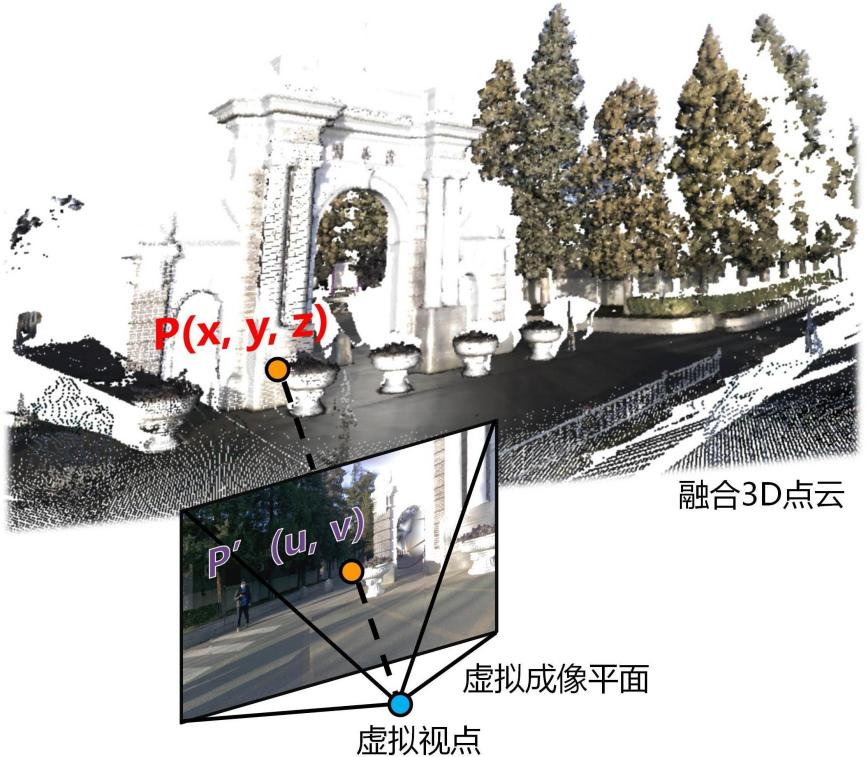
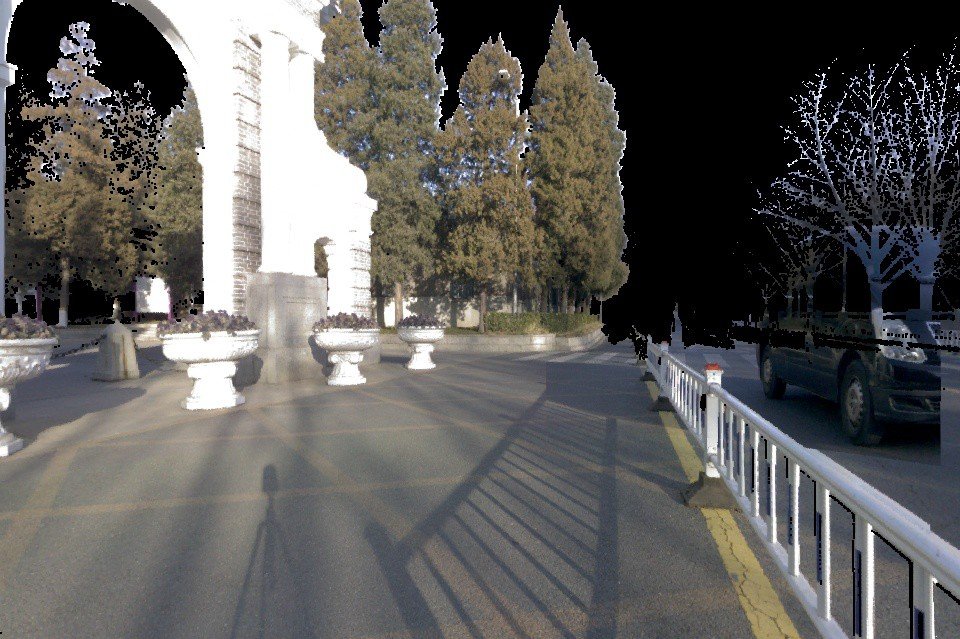
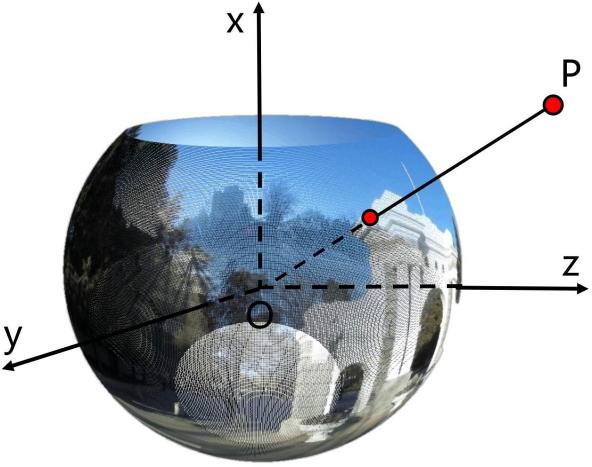
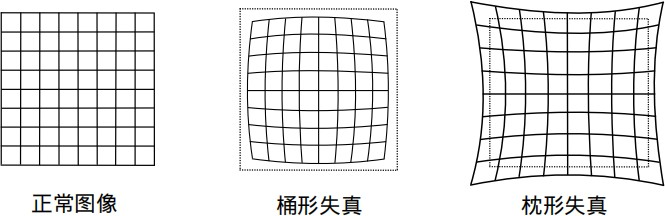
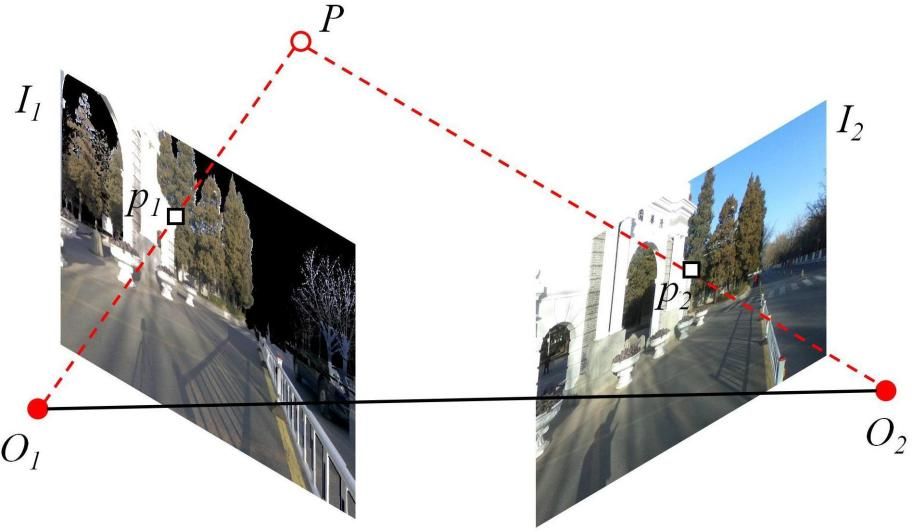