改进的用户协同过滤推荐算法的对比与实现
摘要
随着互联网的普及和发展,人们越来越难以从海量信息中获取自己所需要的个性化信息。推荐系统的产生使得这个问题得以解决,它通过用户的历史信息和访问行为,为用户推荐符合用户需求的个性化信息。其中,协同过滤推荐技术是推荐系统中应用最早、最广泛,也是最为成功的技术之一。本文根据亚尔迪(厦门)科技有限公司的实际需求,研究改进基于用户的协同过滤推荐算法的对比与实现。
首先,在对算法进行深入理解和阐述后进行了实现,并利用推荐算法常用的四个指标:召回率、准确率、覆盖率和流行度对结果进行分析。在实现过程中,利用了余弦相似度计算用户的相似度,使用Movielens100K数据集,找到推荐效果最好时的K值(最近邻数)为50左右。
其次,针对协同过滤推荐算法存在的稀疏性问题,本文建立物品-用户的倒查表,只计算有相同评分项的用户之间的相似度,避免了传统方法两两用户计算相似度的复杂工作量,减少了约10%的运行时间。
再次,本文还根据John S. Breese提出的结论,利用“惩罚”用户共同兴趣列表中热门物品对他们相似度的影响这一方法,改进了用户相似度的计算,通常为了保证评测指标不是过拟合的结果,利用十折交叉法进行交叉验证,使得召回率由原来的最高19.523%提升到19.550%,准确率由最高22.842%提升到22.874%、覆盖率由最高31.084%提升到32.711%。
最后,本文对比了在两个不同规模数据集Movielens100K和HetRec 2011数据集下的运行结果,前者推荐效果最好的K值为50,而后者为150;还实现了计算用户相似度的其他方法:Jaccard系数、欧式距离、皮尔逊系数,并与余弦相似度方法进行对比,得出余弦相似度方法更合适做相似度量的结论。
本文仅涉及到推荐算法的理论研究,进一步需要将经过改进的、并在标准数据集和实际电子商务交易数据上验证之后的推荐算法开发成一个即插即用的模块,集成到《智能电子商务数据综合分析系统》中。完成智能电子商务数据分析平台的集成测试和系统测试后,投入试产运营,并取得社会经济效益。
关键词: 推荐算法;协同过滤;基于用户;最近邻数;稀疏性;倒查表;相似度计算;十折交叉法
Abstract
With the popularization and development of the Internet, people are becoming more and more difficult to obtain the personalized information they need from huge amounts of information. Recommendation system has solved this problem, and it recommends personalized information that meets users’ requirements referring to users’ historical information and access records. Collaborative filtering recommendation is one of the earliest and most widely used technologies. According to the actual demand of Yardi (Xiamen) science and technology co., LTD, this paper discusses the contrast and implementation of improved User based collaborative filtering recommendation algorithm.
First of all, the algorithm is implemented after the deep understanding and expounds of the algorithm. And the commonly used four indicators: recall ratio, accuracy, coverage and popularity are used to analysis the results. In the process, Cosine similarity was used to calculate the similarity of users, based on the Movielens100K data set, and the best recommend effect of K value (number of nearest neighbors) was about 50.
Secondly, aiming at the existing data sparseness of collaborative filtering recommendation algorithm, this paper establish items – user inversion table, just calculate with the users similarity which users have same rating items. In this way, the complicated traditional method and calculation of users’ similarity was avoided, and about 10% run time was saved.
Moreover, according to John S. Breese’s conclusion (reduce the influence of hot items for users’ similarity), user similarity computing is improved. The recall ratio enhance from 19.523% to 19.523%, accuracy from 22.842% to 22.842%, coverage from 31.084% to 32.711%.
Finally, the results from two different scale data sets: Movielens100K and HetRec
2011 were compared. The former best recommend effect of K value is 50, while the latter is 150. Other methods of computing the user similarity (Jaccard coefficient, Euclidean distance, Pearson coefficient) were implemented, and compared with Cosine similarity, concluding Cosine similarity is more suitable for similarity measures.
As a module, there works finally integrated into the Intelligent Electronic Commerce Data Comprehensive Analysis System.
Keywords: recommendation algorithm, collaborative filtering, user based, K value, inversion table, similarity calculation
目录
摘要 - 2 -
Abstract - 3 -
1 绪论 - 7 -
1.1研究背景与意义 - 7 -
1.2国内外研究现状 - 9 -
1.3论文的内容及结构 - 10 -
1.3.1论文的主要内容和组织结构 - 10 -
1.3.2论文的创新点 - 11 -
2 电子商务个性化推荐系统 - 12 -
2.1电子商务推荐系统概述 - 12 -
2.2电子商务推荐系统的结构 - 13 -
2.3电子商务推荐系统的作用 - 15 -
2.4推荐系统技术 - 16 -
2.4.1协同过滤推荐技术 - 16 -
2.4.2基于内容的推荐技术 - 17 -
2.4.3基于关联规则的推荐技术 - 19 -
2.4.4基于知识的推荐技术 - 20 -
2.4.5混合推荐技术 - 20 -
2.4.6主要推荐技术对比 - 20 -
3 基于用户的协同过滤算法及其改进 - 21 -
3.1算法简介 - 21 -
3.2算法步骤 - 23 -
3.3几种常用的计算相似度的方法 - 24 -
3.3.1欧几里德(欧氏)距离 - 24 -
3.3.2皮尔逊相关系数 - 25 -
3.3.3 Cosine(余弦)相似度 - 25 -
3.3.4 Jaccard 系数 - 26 -
3.4算法存在的主要问题 - 26 -
3.5改进的用户协同过滤算法 - 27 -
4实验验证和结果分析 - 30 -
4.1测试数据集简介 - 30 -
4.2算法评价指标 - 31 -
4.3编程实现语言Python - 32 -
4.3.1Python综述 - 32 -
4.3.2Python特点概述 - 33 -
4.4实验环境参数介绍 - 34 -
4.5实验结果 - 35 -
4.5.1不同K值对推荐效果的影响 - 35 -
4.5.2 Movielens100K数据集的运行结果数据用Matlab描点绘图、拟合 - 36 -
4.5.3采用传统计算用户相似度的方法与采用倒查表方法运行时间的对比 - 38 -
4.5.4采用用户相似度改进方法后的对比 - 39 -
4.5.5使用Movielens100K数据集与HetRec 2011数据集对比 - 39 -
4.5.6采用不同计算相似度方法的结果对比(Jaccard系数,欧式距离,皮尔逊系数) - 42 -
4.6实验结果的分析与比较评价结果 - 45 -
4.6.1不同K值对推荐效果的影响 - 45 -
4.6.2 Movielens100K数据集的运行结果曲线图 - 45 -
4.6.3传统计算用户相似度的方法与倒查表方法运行时间的对比 - 45 -
4.6.4采用用户相似度改进方法后的对比 - 46 -
4.6.5使用Movielens100K数据集与HetRec 2011数据集对比 - 46 -
4.6.6采用不同计算相似度方法的结果对比(Jaccard系数,欧式距离,皮尔逊系数) - 46 -
5 结论与展望 - 47 -
5.1本文主要内容总结 - 47 -
5.2进一步研究方向 - 47 -
参考文献 - 48 -
致谢 - 50 -
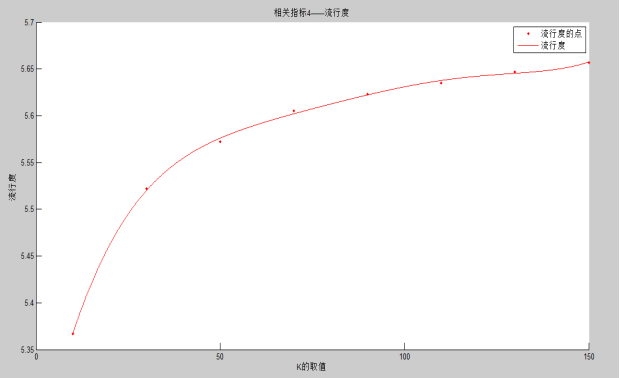
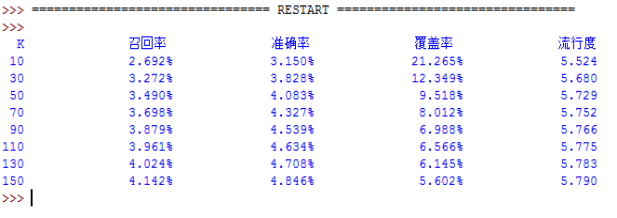
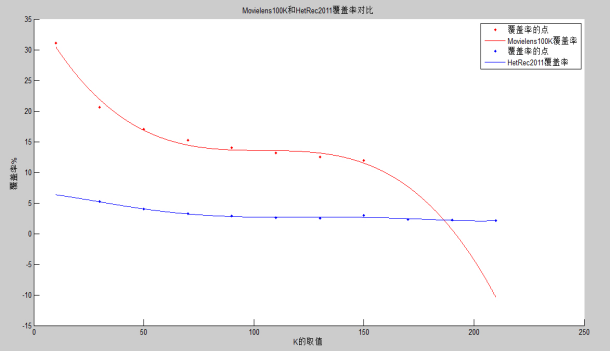
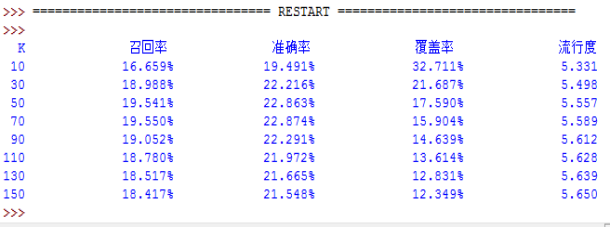
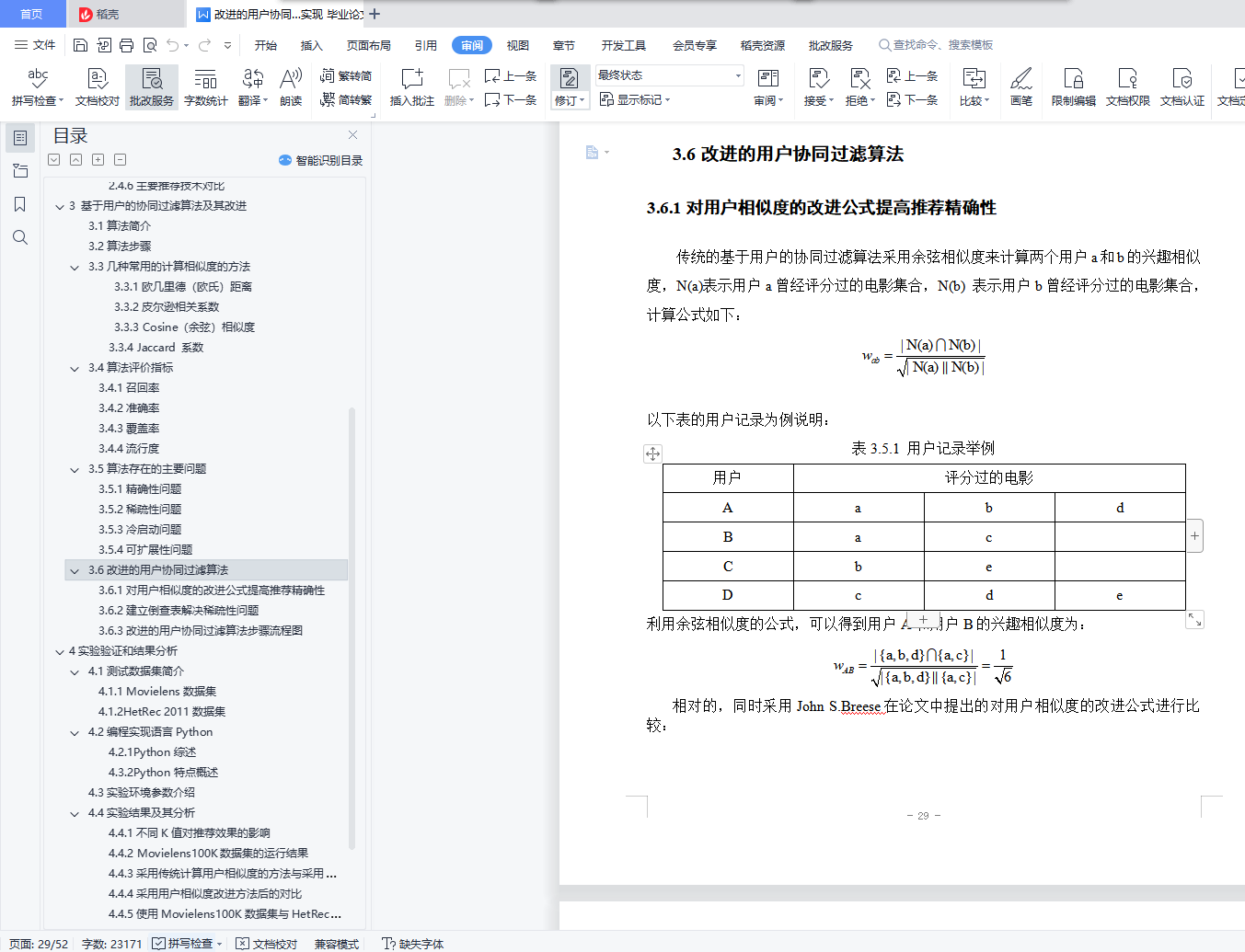
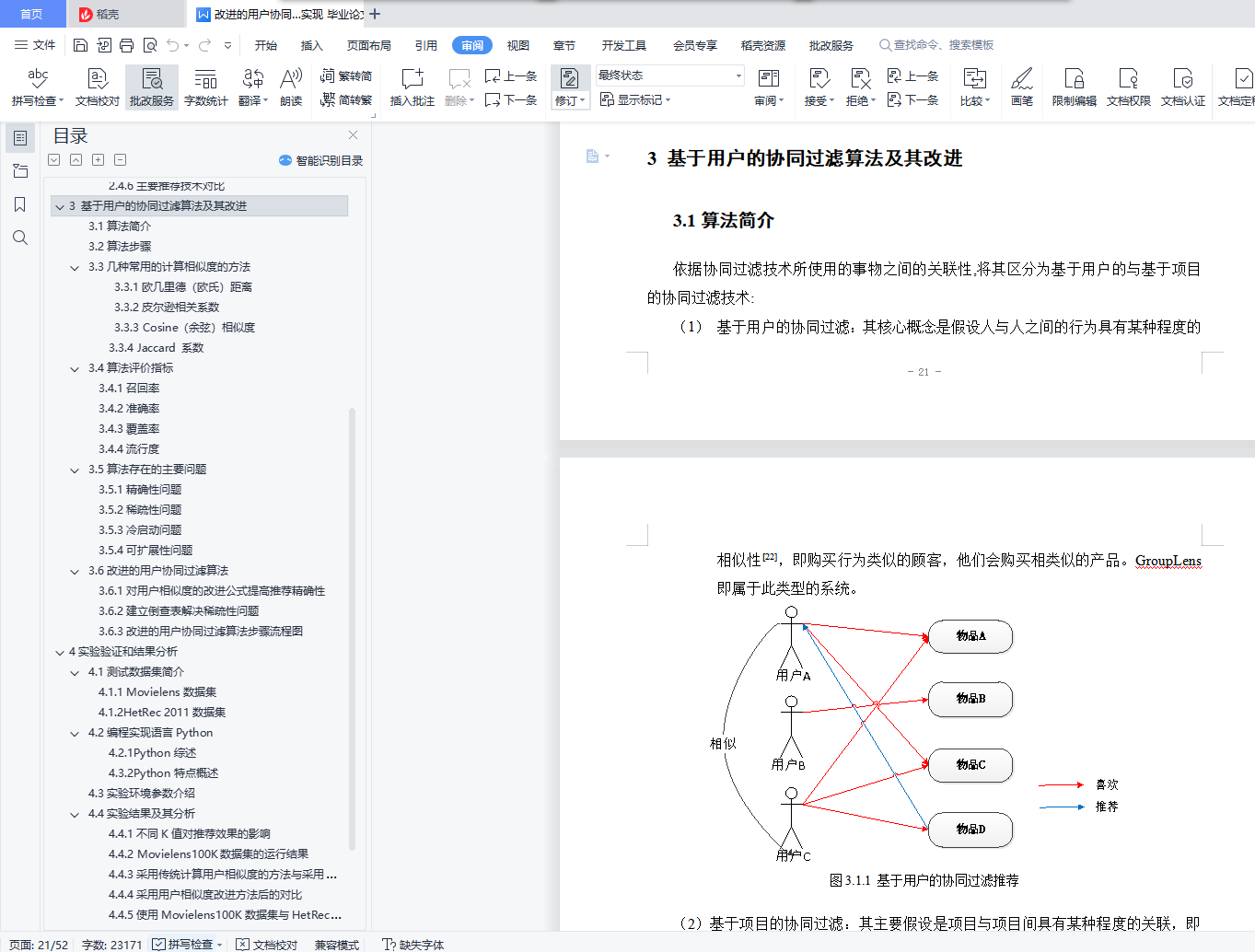
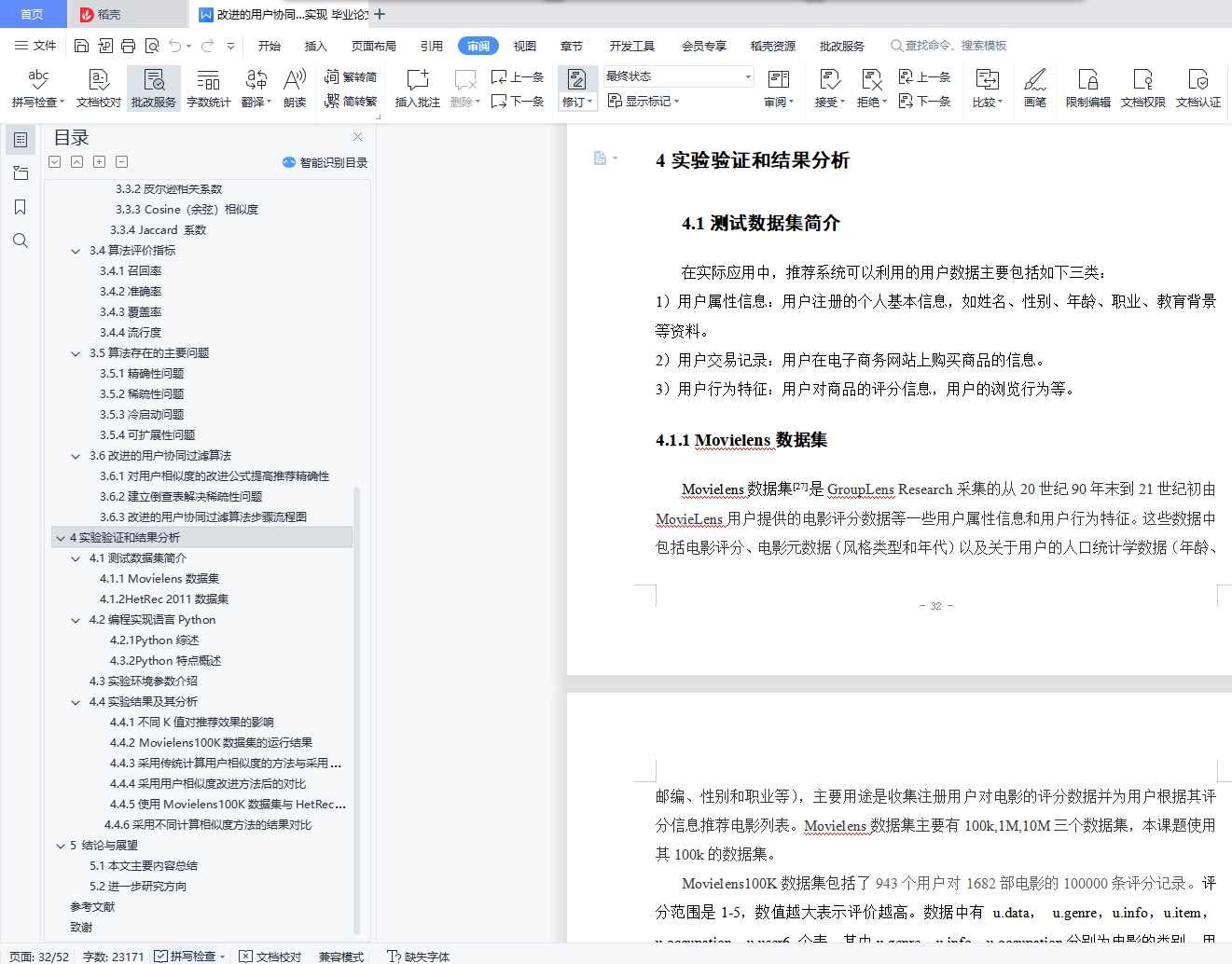
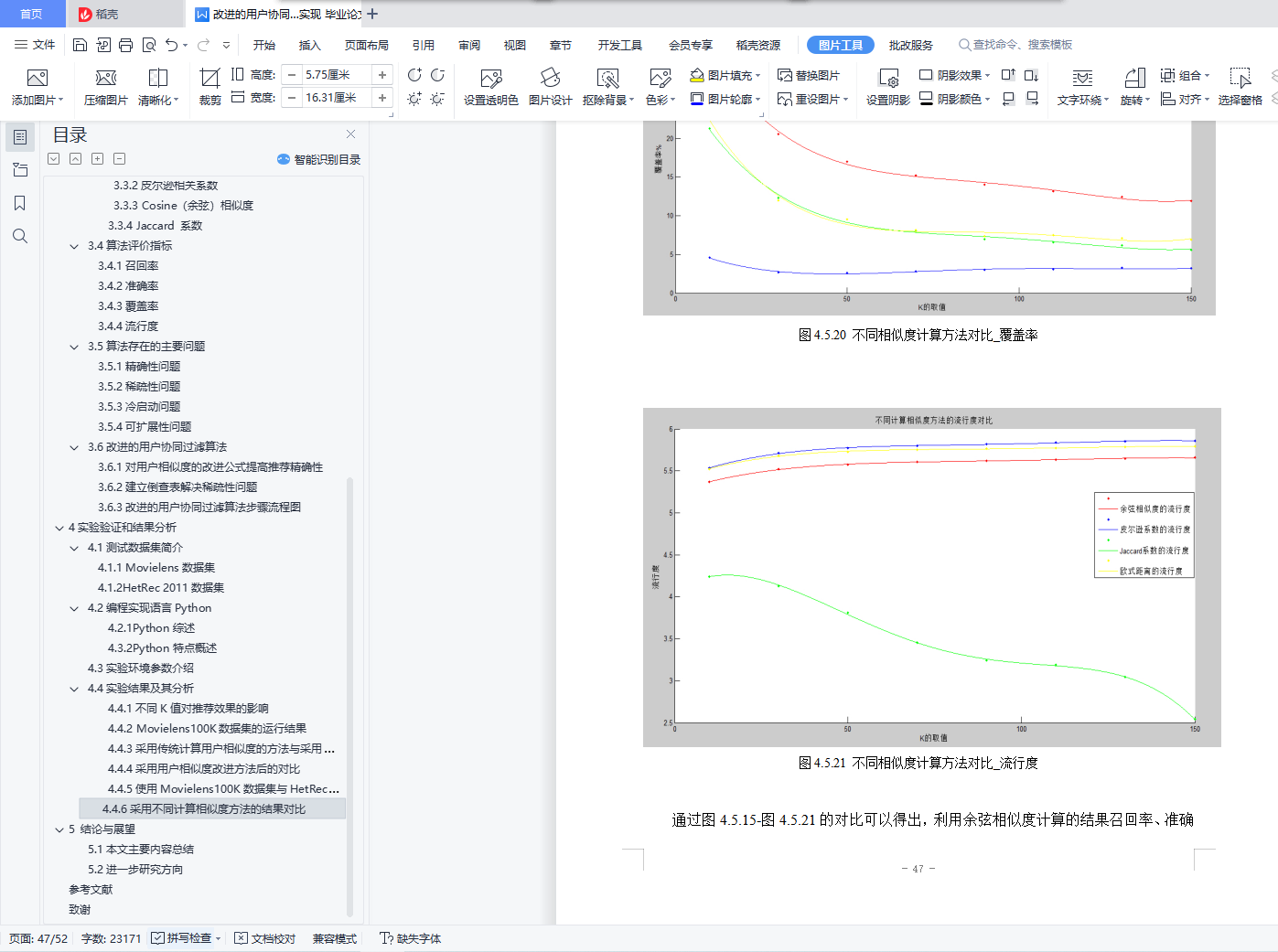
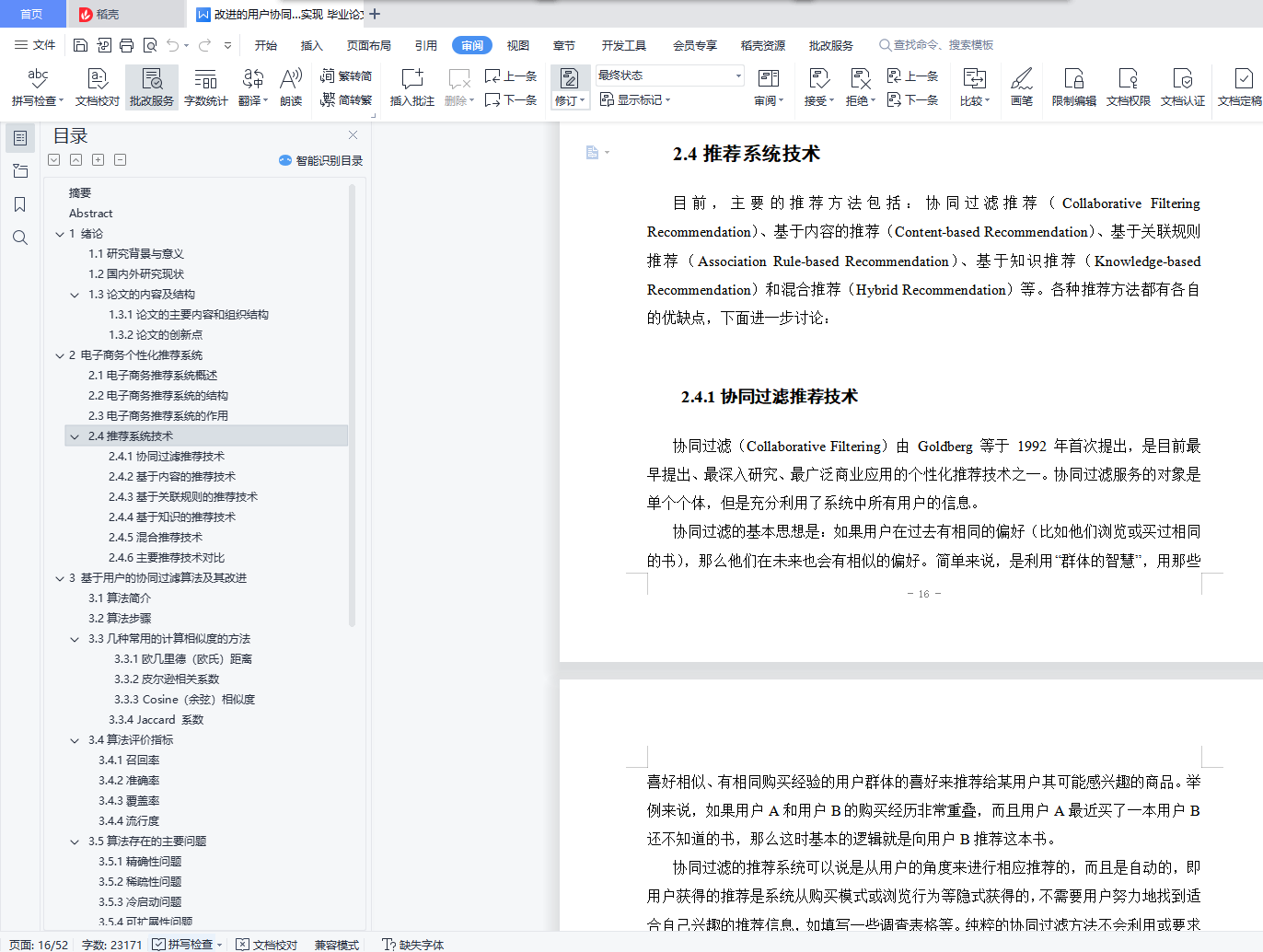
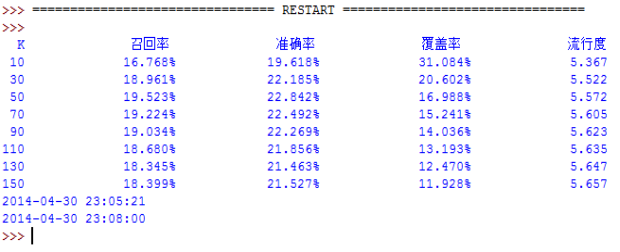
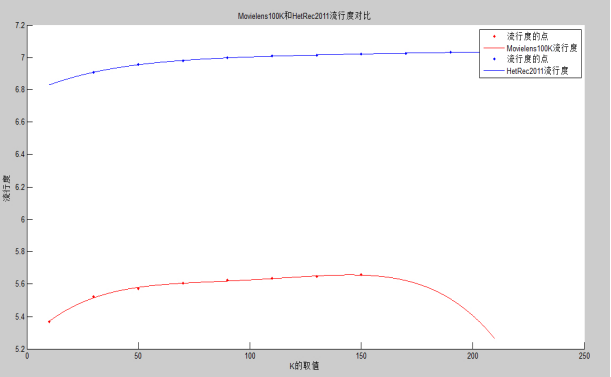
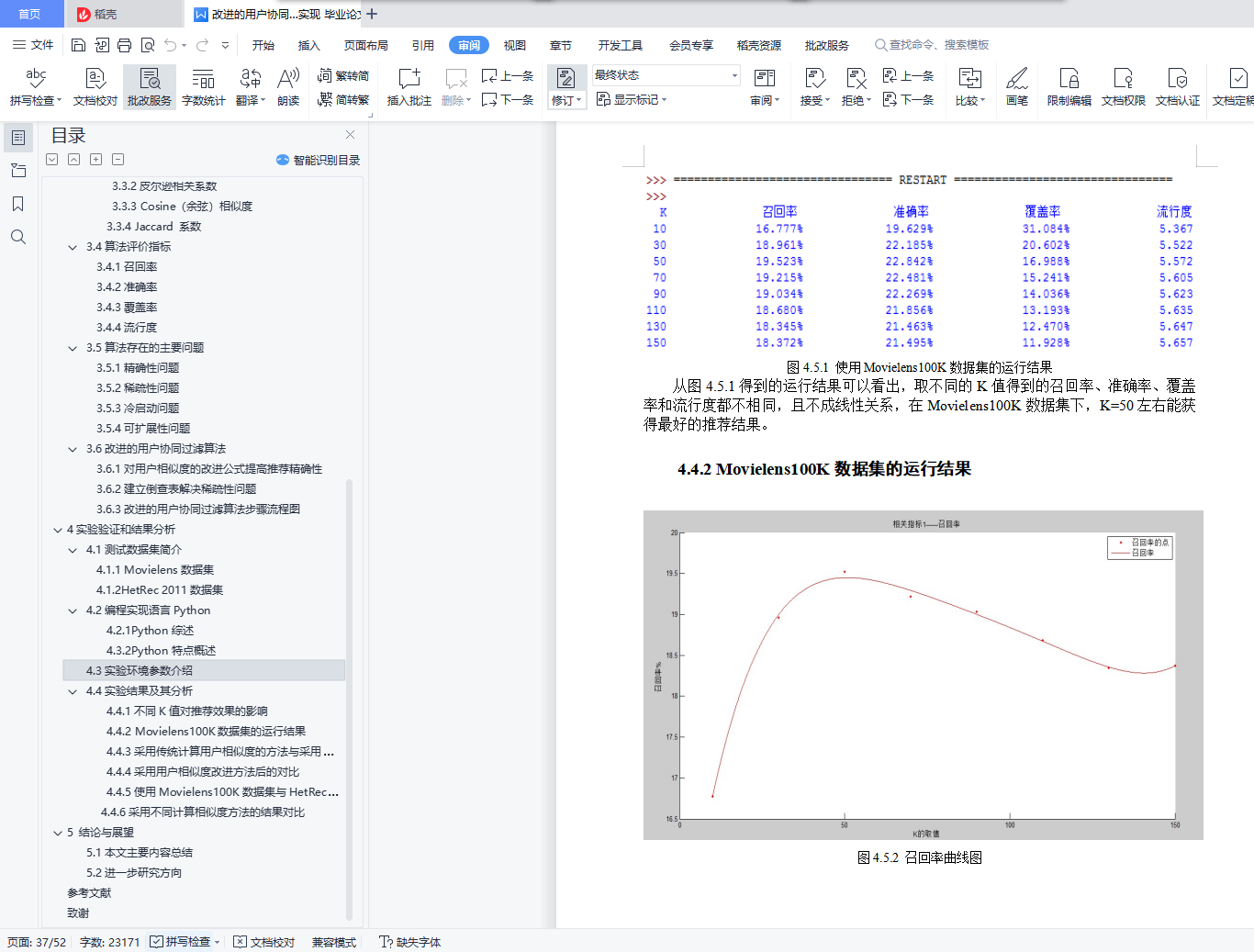
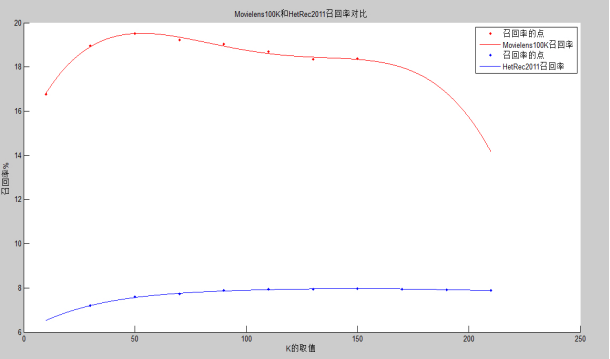
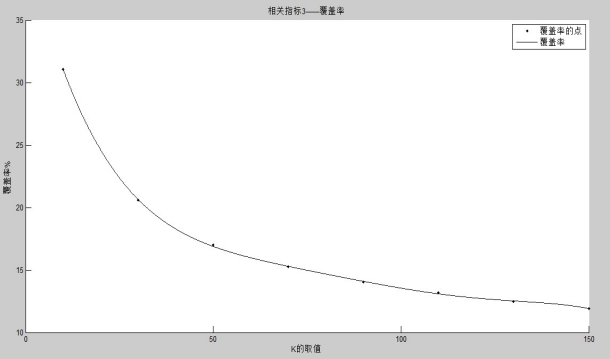
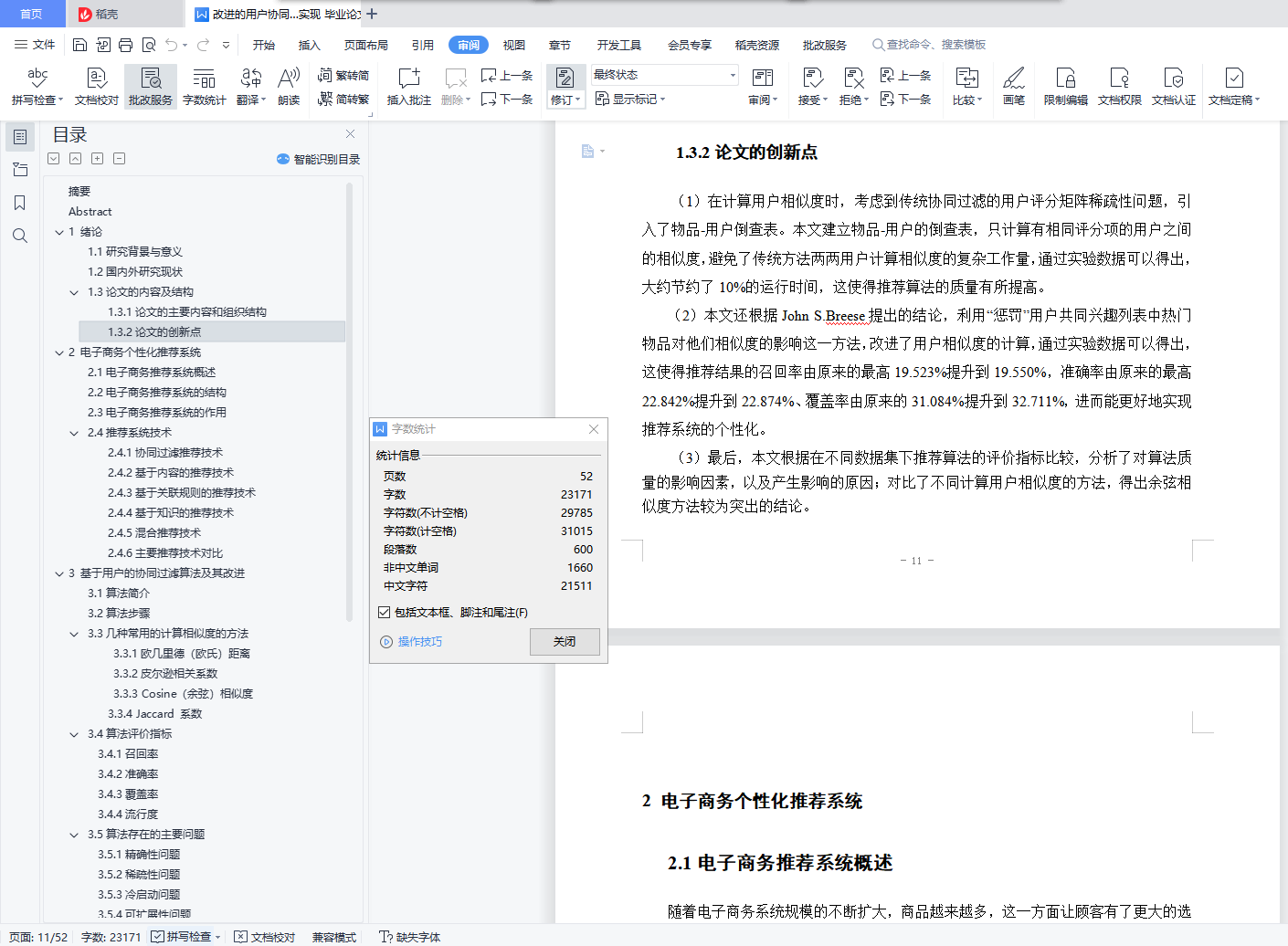