摘 要
由于计算机硬件以符合摩尔定律的速度迅猛发展,计算机数据存储、数据传输和分布式计算的成本都大幅度降低。而现代化的工厂中,往往都布置有大量的传感器,而且随着存储成本的降低,读取到的设备信息变得更加丰富,由此便会产生海量的工业数据。
对于这一变化,全世界许多国家都相继提出相应的举措。最初是德国提出了“工业4.0”的概念,之后美国也随之推出“工业互联网”,我国也相继推出“中国制造2025”的概念,其核心都指向智能制造。而工业大数据技术则是这些内容中的核心部分。
在工业设备的运行过程中,自然磨损、设备超载、操作不当等多种原因会导致设备的性能发生下降,甚至于产生故障或者是异常。而通过对设备加装传感器进行监控,获取到设备的实时信息并且加以梳理计算,就可以得到设备各个部位的实时运行状态,从而实现对设备的监控。而如果出现了设备故障现象,则可以通过对历史数据进行数据挖掘、清洗形成故障模型,导入设备的最新运行数据进行故障诊断。
设备的故障诊断方法可以按照诊断依据分为三种:基于机理模型的方法,基于数据驱动的方法,基于知识工程的方法[1]。本文将采用基于数据驱动的方法中的基于分类的方法进行故障模型的构建。同时为了对比不同分类方法的性能,本文采用了两种分类方式进行比较。
本文主要研究工作和成果如下:
(1)建立了以决策树算法为基础的故障诊断模型;
(2)实现了以支持向量机为核心的数据驱动方法。对支持向量机的核心原理进行了研究,以风力涡轮机齿轮箱的健康数据、故障数据进行了SVM的分类检测,实现了简单的故障诊断;
(3)比对了两种策略的精度以及其他的一些的性能度量。
关键词:故障诊断;工业大数据;数据驱动;决策树;支持向量机
Abstract
As the computer hardware rapidly develops at a rate consistent with Moore's Law, the cost of computer data storage, data transmission, and distributed computing has been greatly Reduced. In the modern factories, a large number of sensors are often arranged, and as the cost of storage decreases, the read device information becomes more abundant, and thus a large amount of industrial data will be generated.
For this change, many countries around the world have put forward corresponding measures in succession. Initially, Germany proposed the concept of "Industry 4.0". After the United States introduced the "Industrial Internet," China has also successively introduced the concept of "Made in China 2025." Its core points to intelligent manufacturing. Industrial big data technology is a core part of these contents.
During the operation of industrial equipment, natural wear, equipment overload, improper operation, and other reasons can cause the performance of the equipment to drop, and even result in failure or abnormality. By adding sensors to the equipment for monitoring, obtaining real-time information from the equipment and combing calculations, the real-time running status of various parts of the equipment can be obtained, thereby realizing the monitoring of the equipment. If there is a device failure, you can perform data mining and cleaning on the historical data to form a fault model and import the latest operating data of the device for fault diagnosis.
The equipment fault diagnosis methods can be divided into three types according to the diagnosis basis: based on the mechanism model method, based on the data-driven method, based on the knowledge engineering method. This article will use a classification-based approach based on a Data-Driven approach to build a fault model. At the same time, in order to compare the performance of different classification methods, this paper uses two classification methods to compare.
The main research work and achievements of this article are as follows:
(1) Establish a fault diagnosis model based on decision tree algorithm, and study the differences between ID3 and C4.5;
(2) A Data-Driven approach based on Support Vector Machines(SVM) is implemented. The core principle of SVM is studied. The classification and detection of SVM is performed based on the health data and fault data of the wind turbine gearbox, and a simple fault diagnosis is realized.
(3) Compare the running time of two strategies, the accuracy rate of fault diagnosis, and some other performance metrics.
Key Words:Fault Diagnosis; Industrial Big Data; Data-Driven Method; Decision Tree; Support Vector Machine
目 录
摘 要 I
Abstract II
1 绪论 1
1.1 选题背景和意义 1
1.2 国内外研究现况及发展趋势 2
1.2.1 国内研究现状 2
1.2.2 国外研究现状 2
1.3 主要研究内容 3
2 故障诊断的总体设计方案 4
2.1 故障模型的要求 4
2.2 决策树建立故障树模型 4
2.2.1 信息熵 4
2.2.2 信息增益 5
2.2.3 ID3算法 5
2.3 支持向量机二分类原理 8
2.3.1 SVM原理 8
2.3.2 对偶问题 11
2.3.3 SVM核函数 13
3 具体方案设计 16
3.1 数据获取 16
3.2 数据存取 17
3.2.1 本地环境 17
3.2.2 大数据平台环境 19
3.3 决策树实现 21
3.3.1 连续属性值离散化 21
3.3.2 样本初始化 23
3.3.3 生成决策树 25
3.3.4 数据测试类 29
3.4 支持向量机实现 30
3.5 人机交互界面设计 32
3.5.1 界面组件介绍 33
3.5.2 操作命令介绍 34
3.5.3 适用场景 36
4 性能分析 38
4.1 性能度量 38
4.2 决策树性能度量 39
4.3 支持向量机性能度量 40
5 结论 42
5.1 全文总结 42
5.2 展望 43
致谢 44
参考文献 45
附录 48
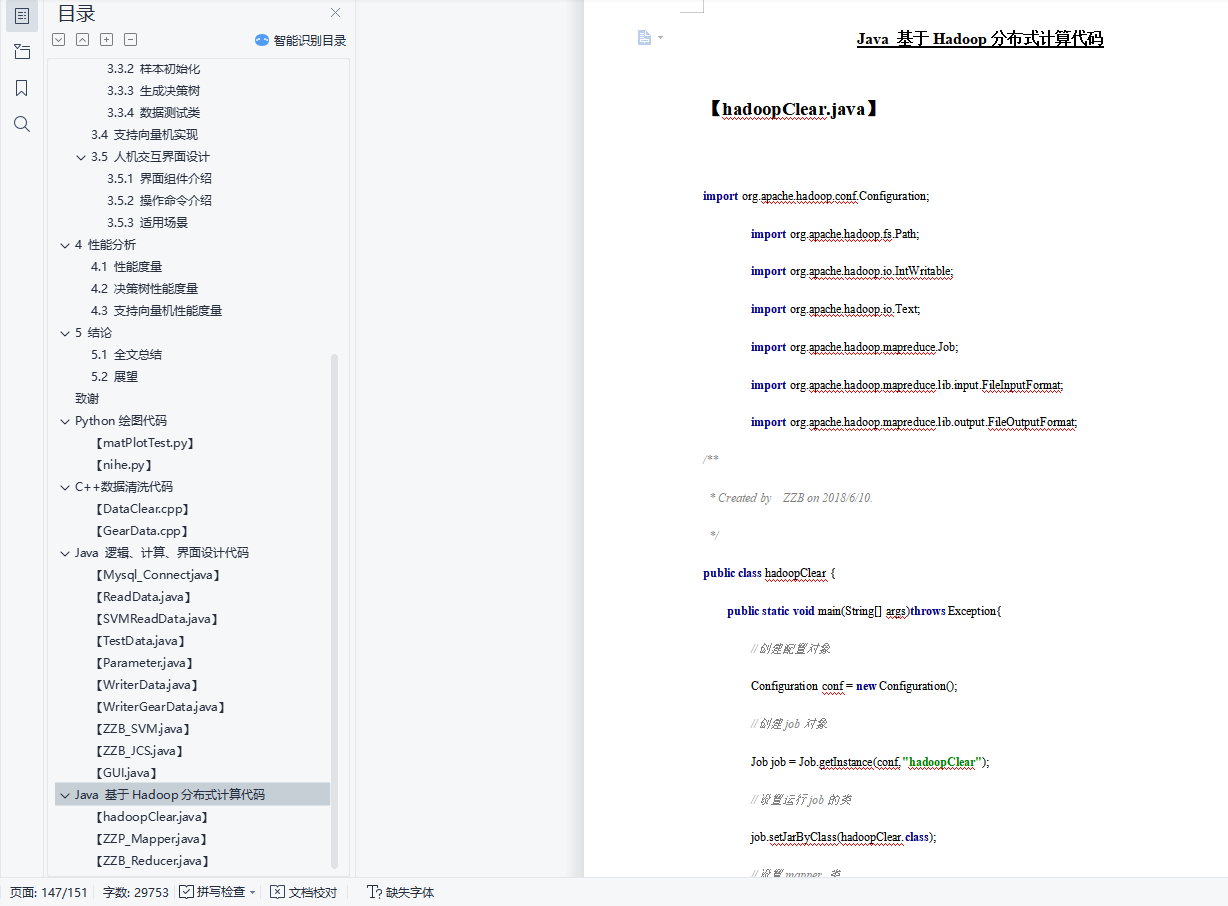
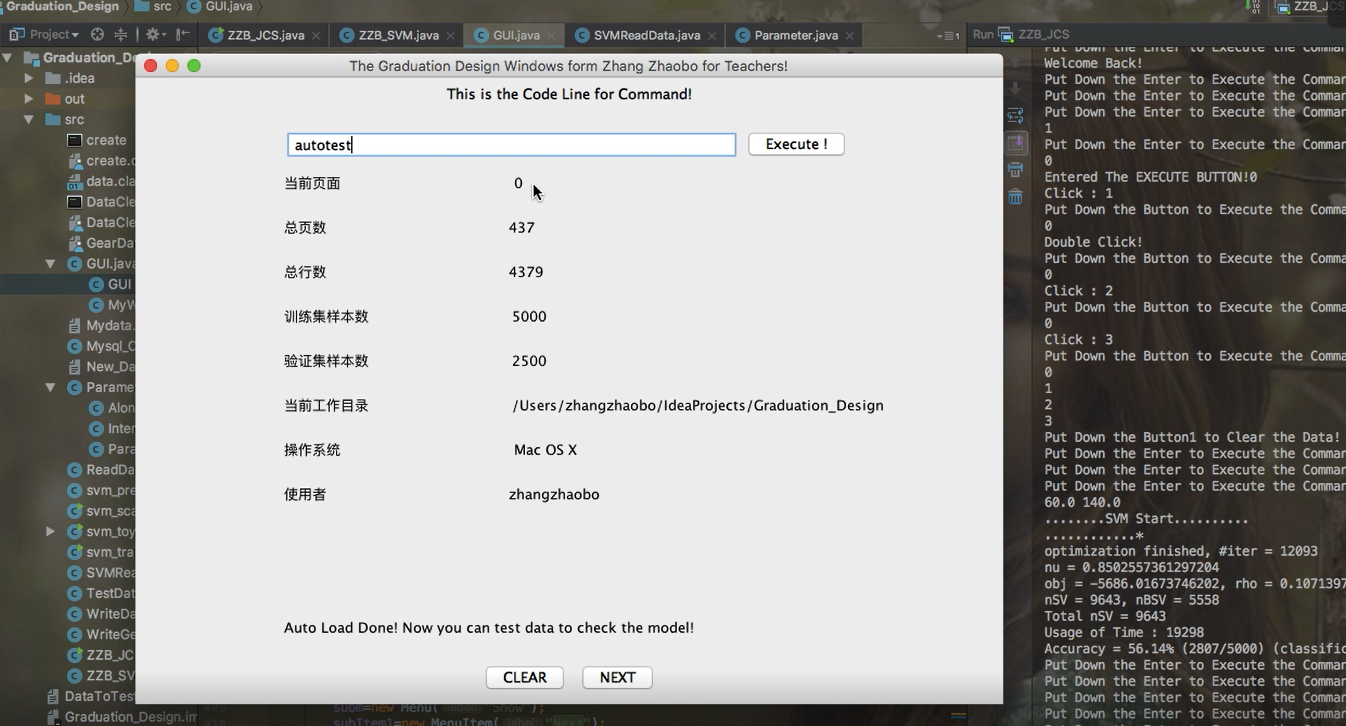
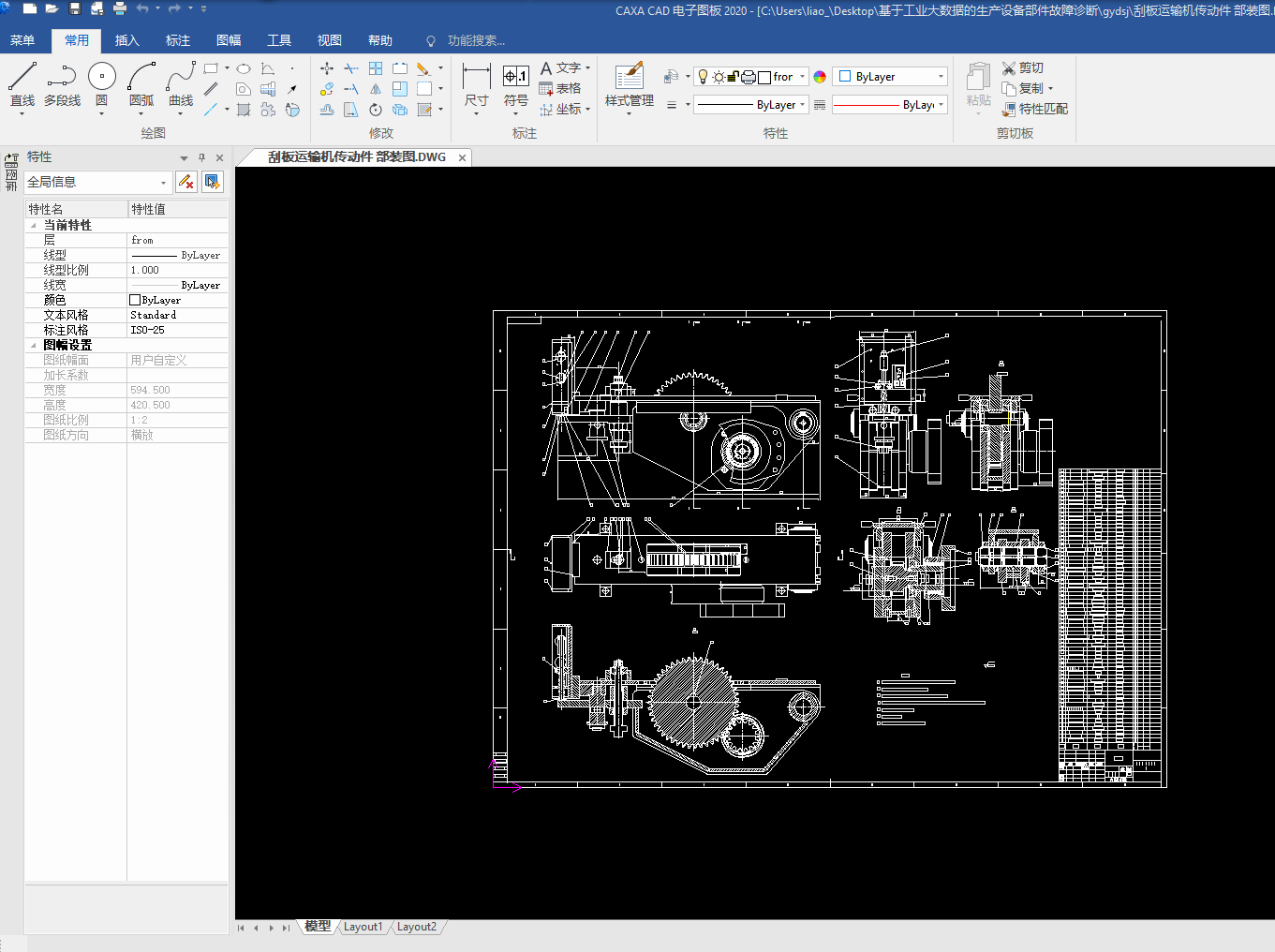
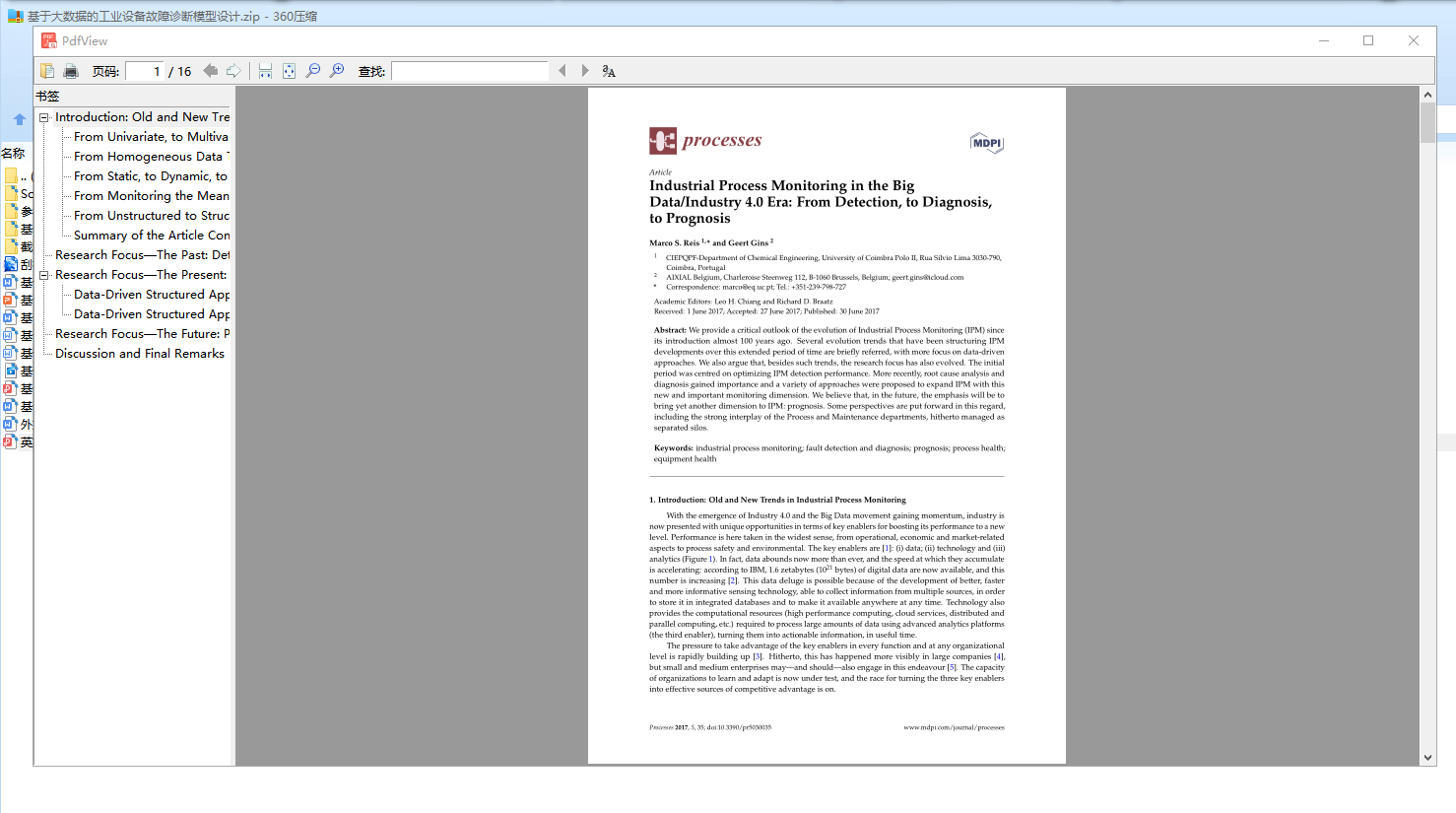
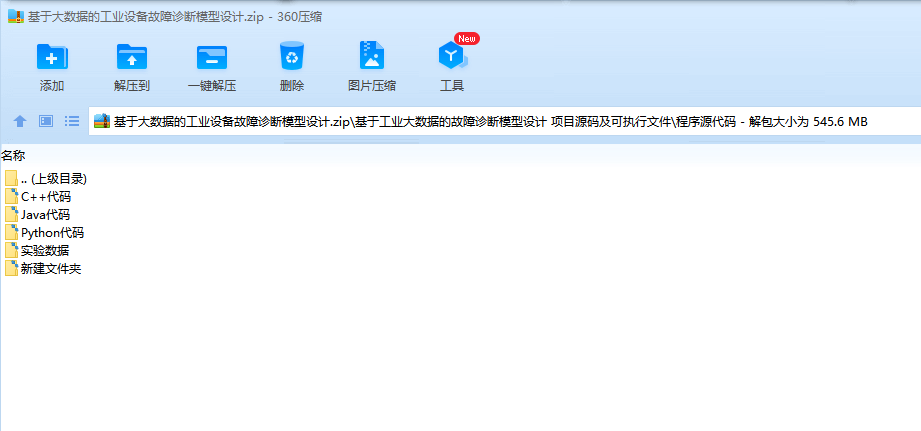
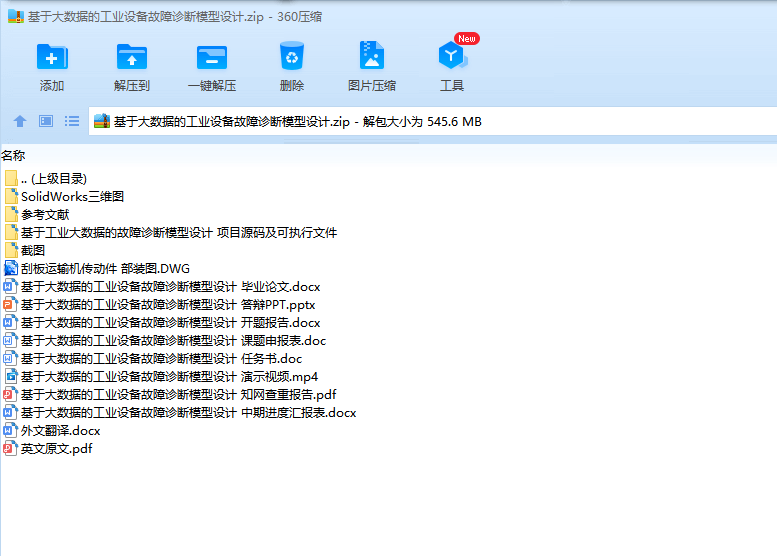
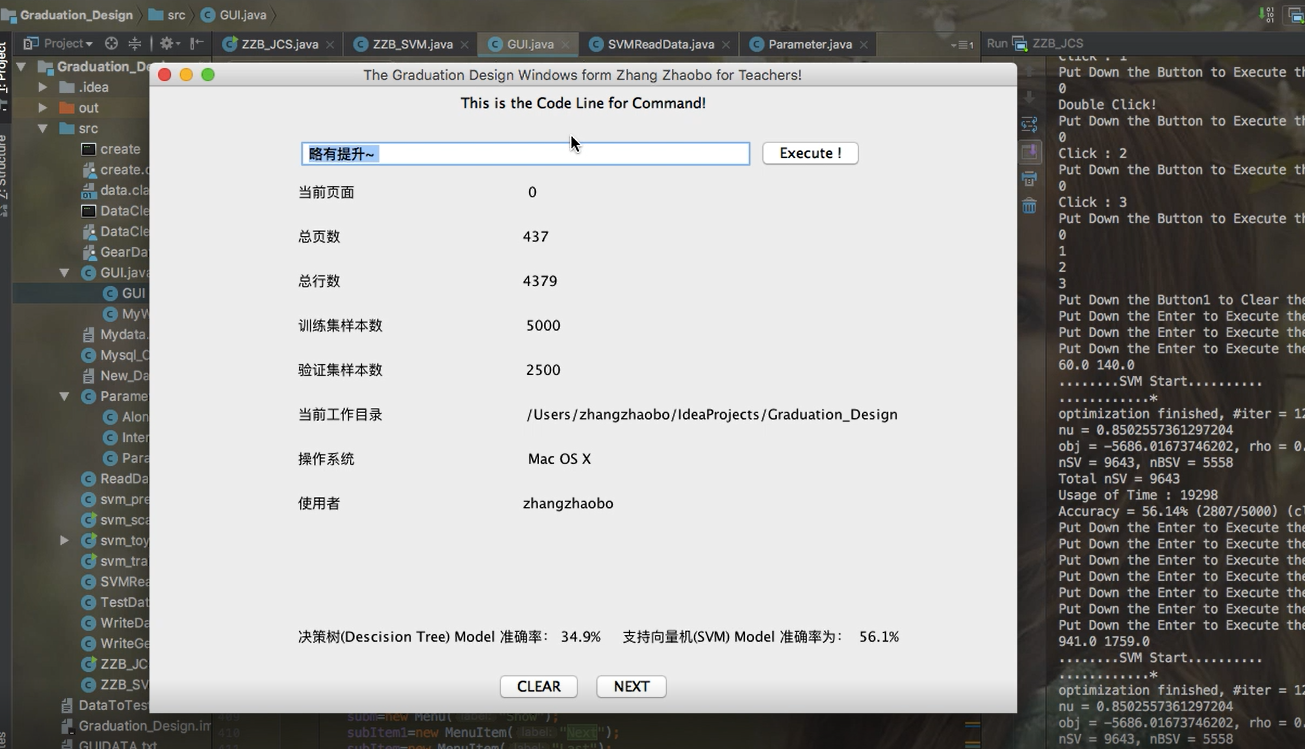
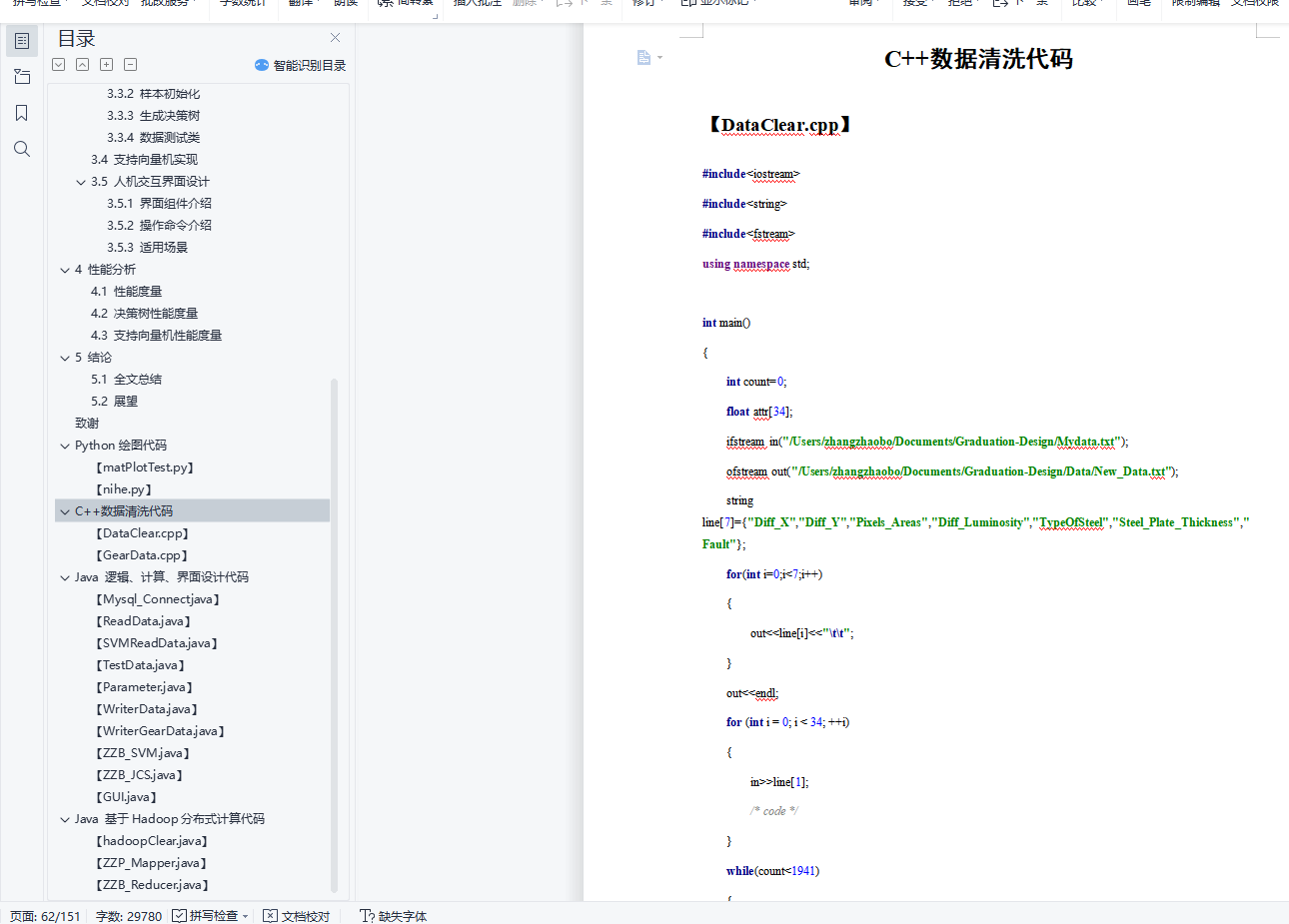
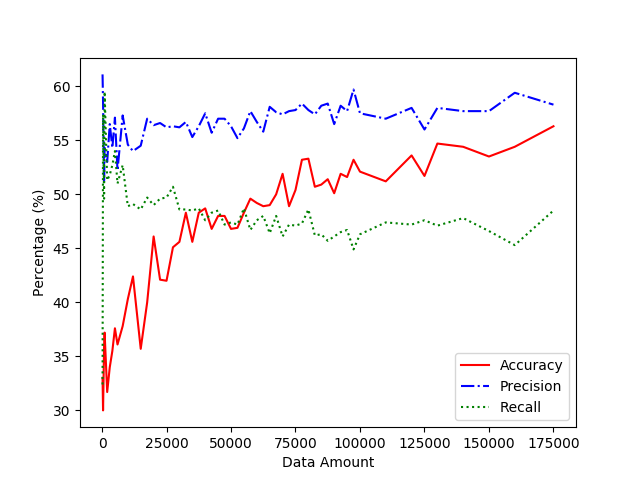
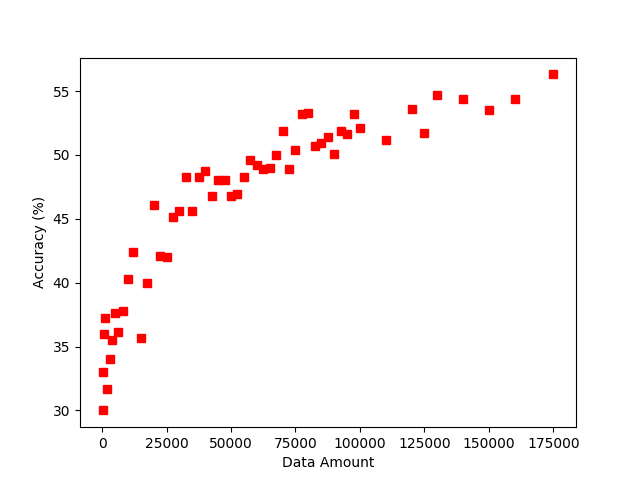
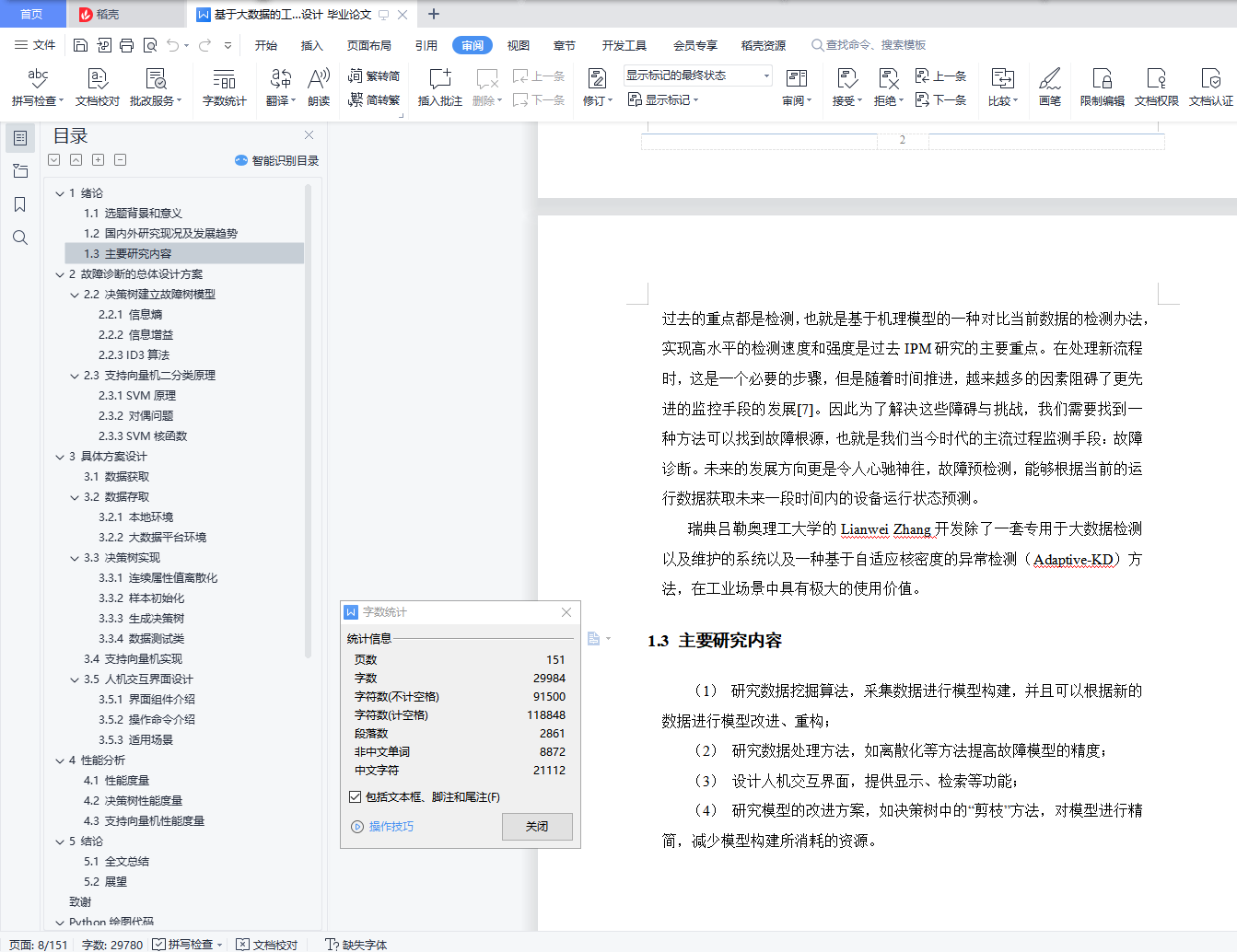
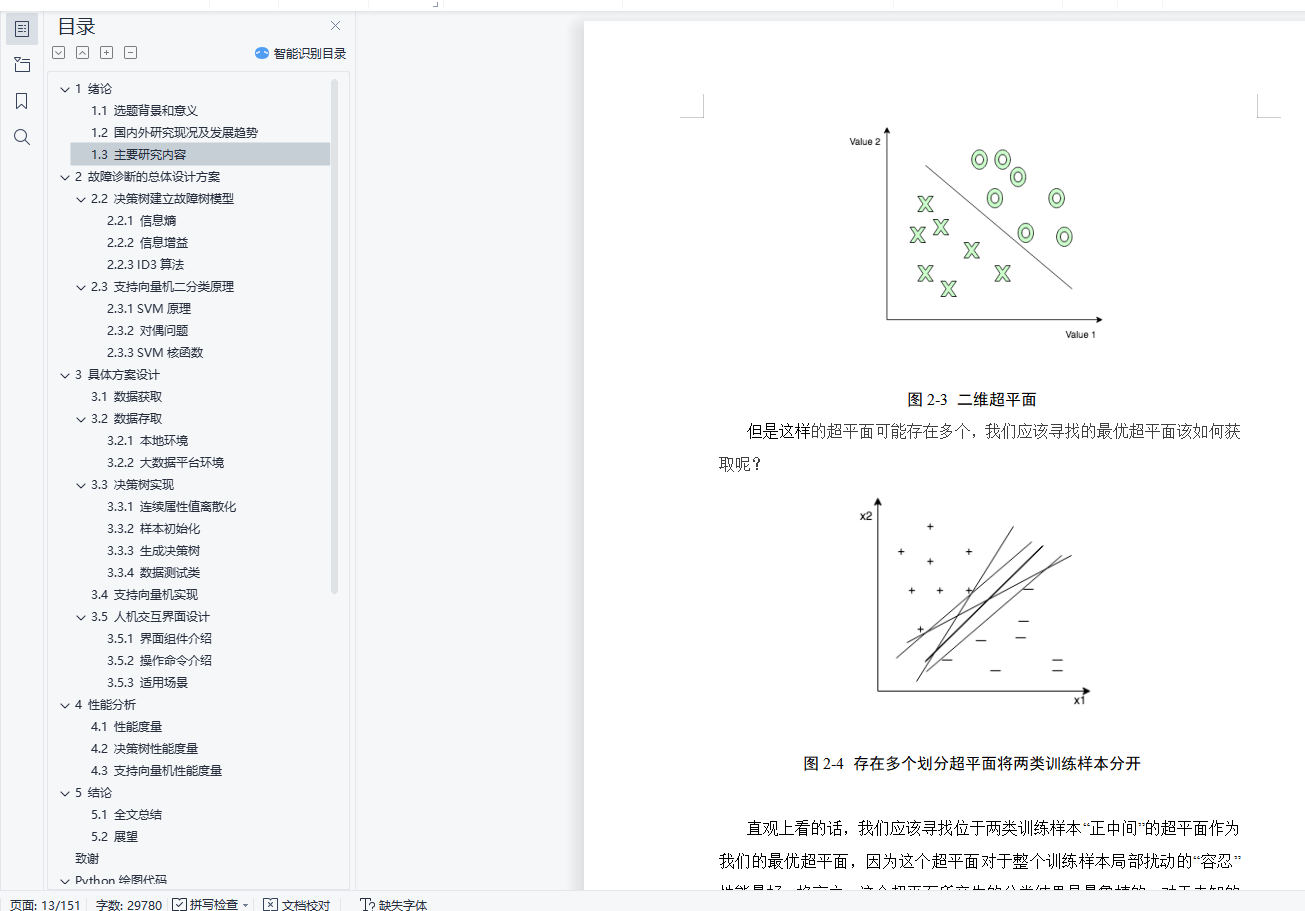
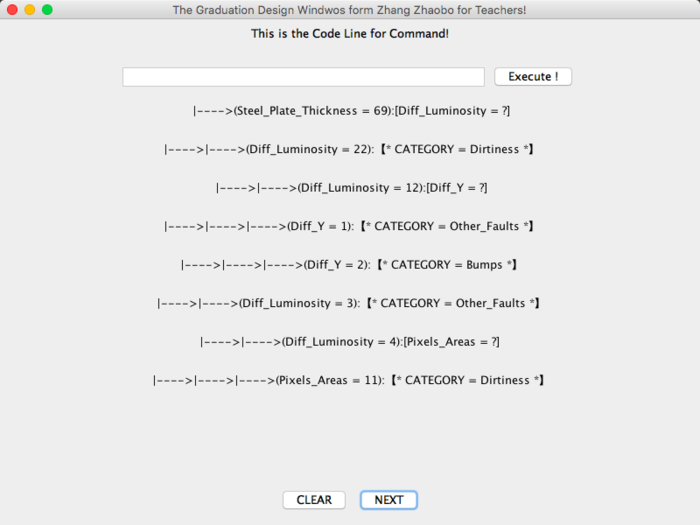
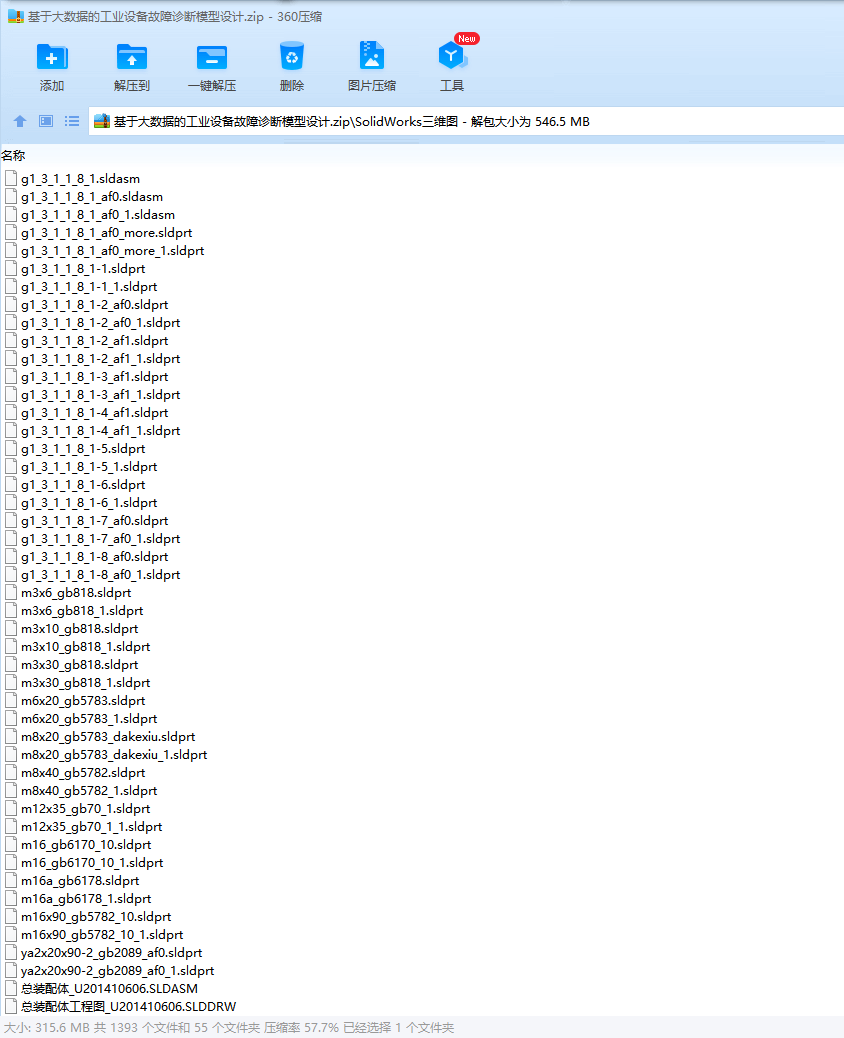
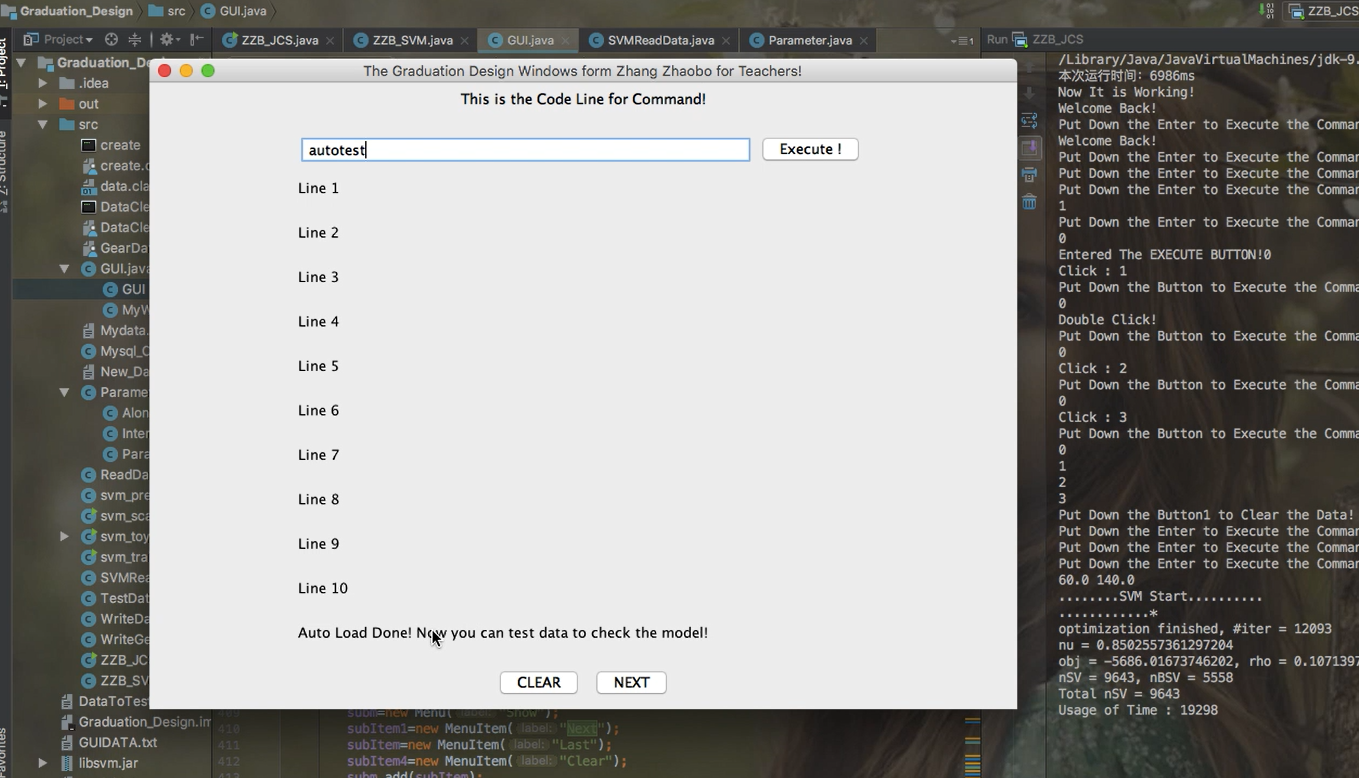
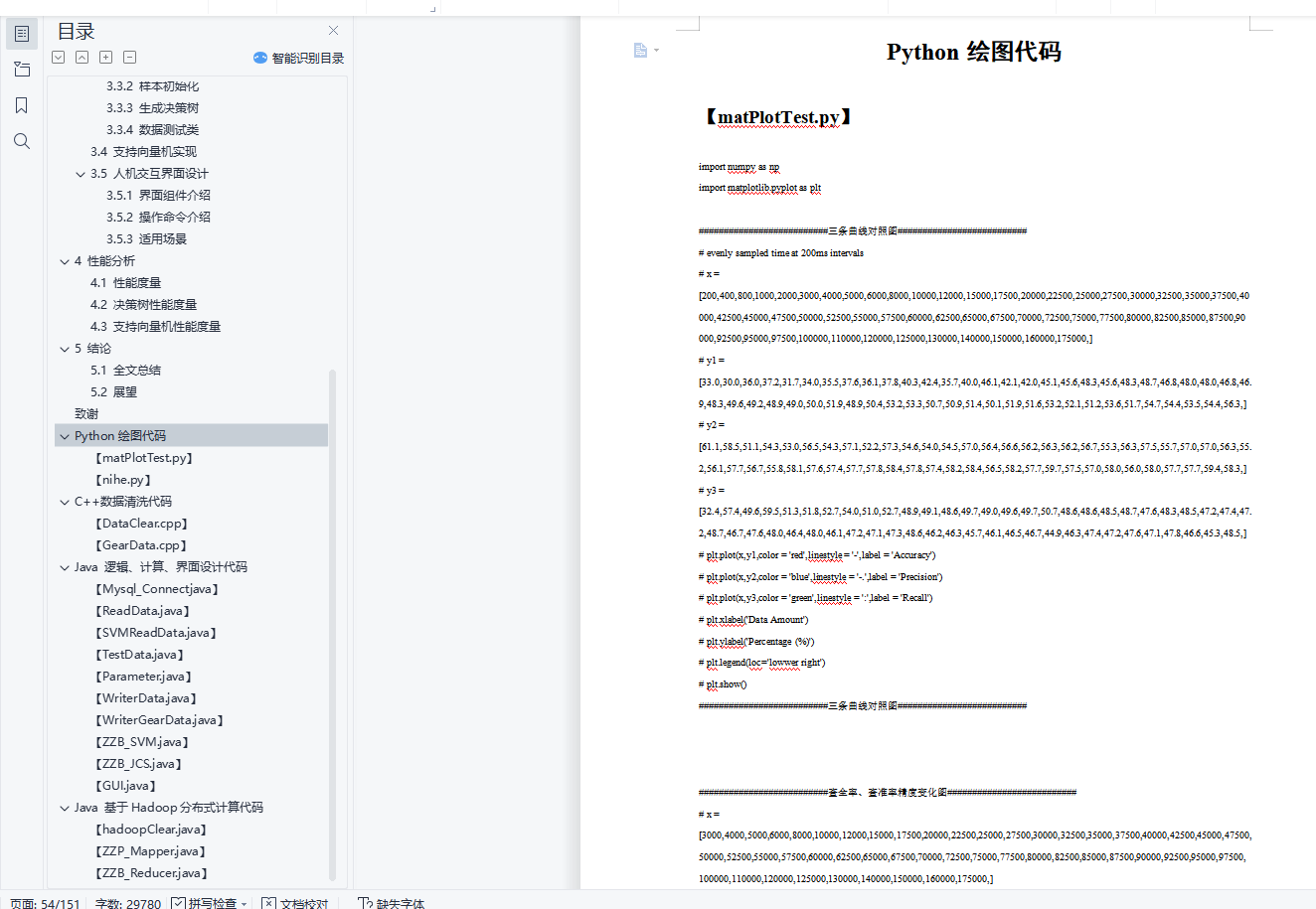
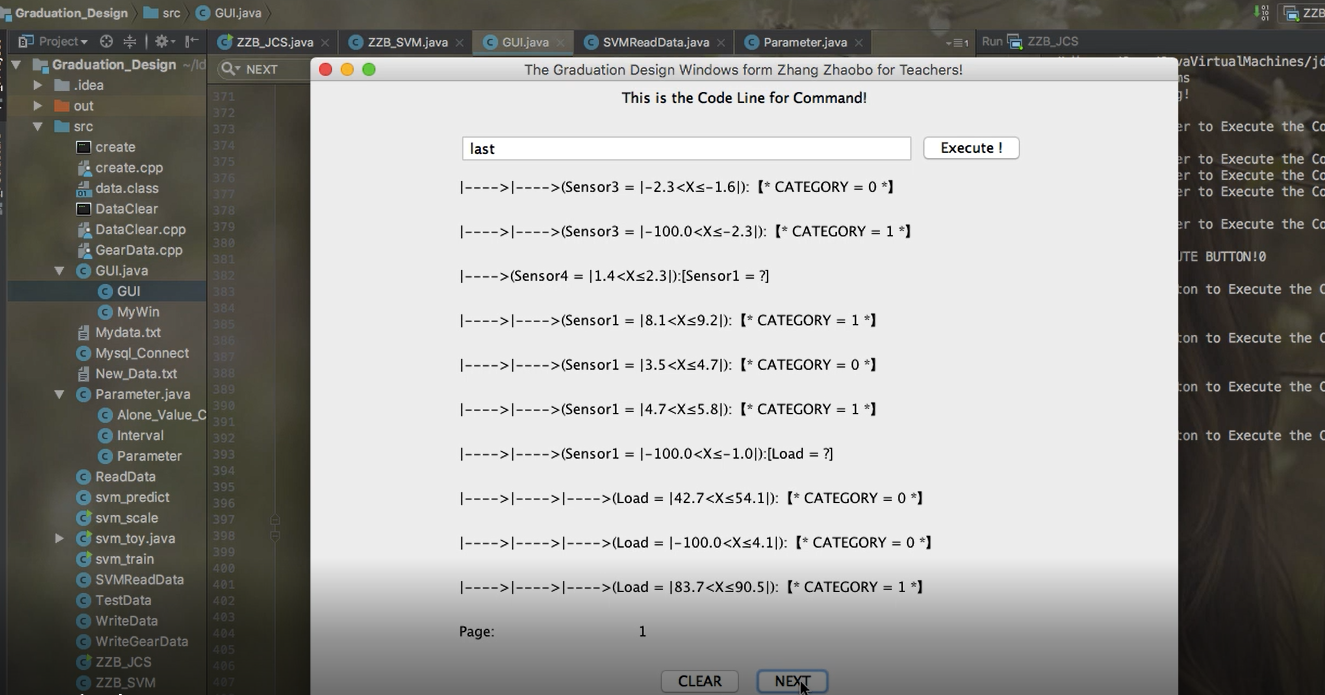
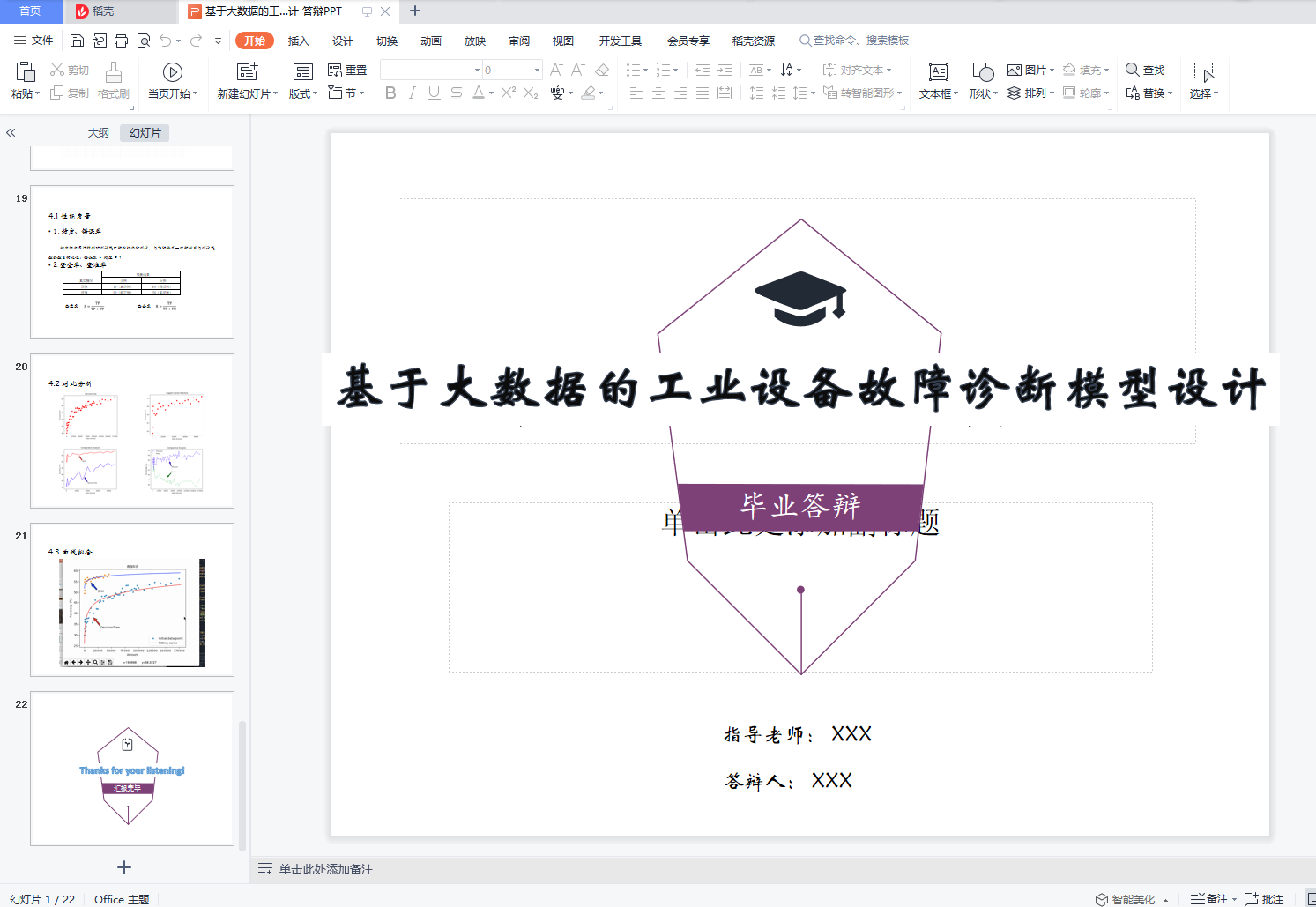
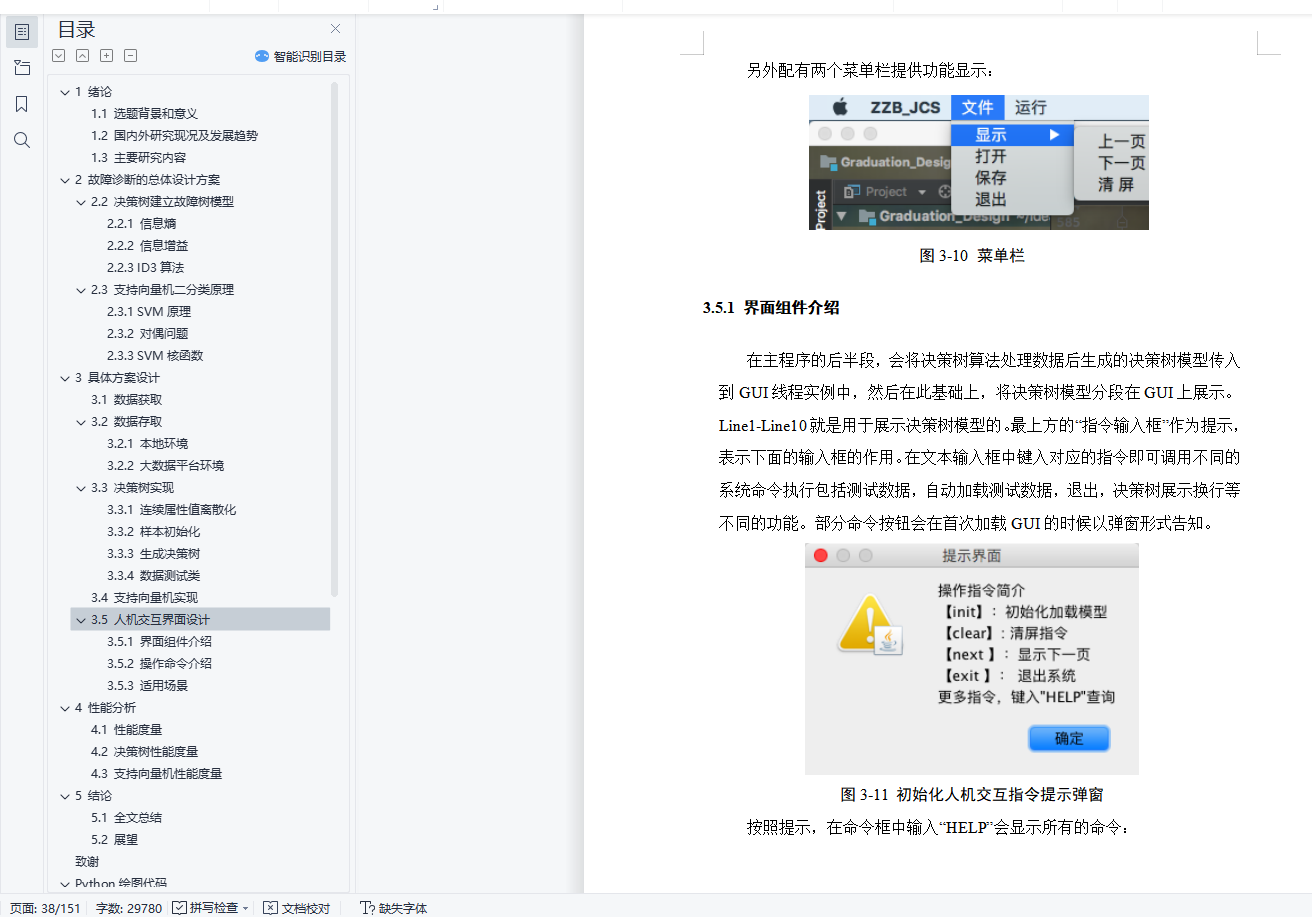
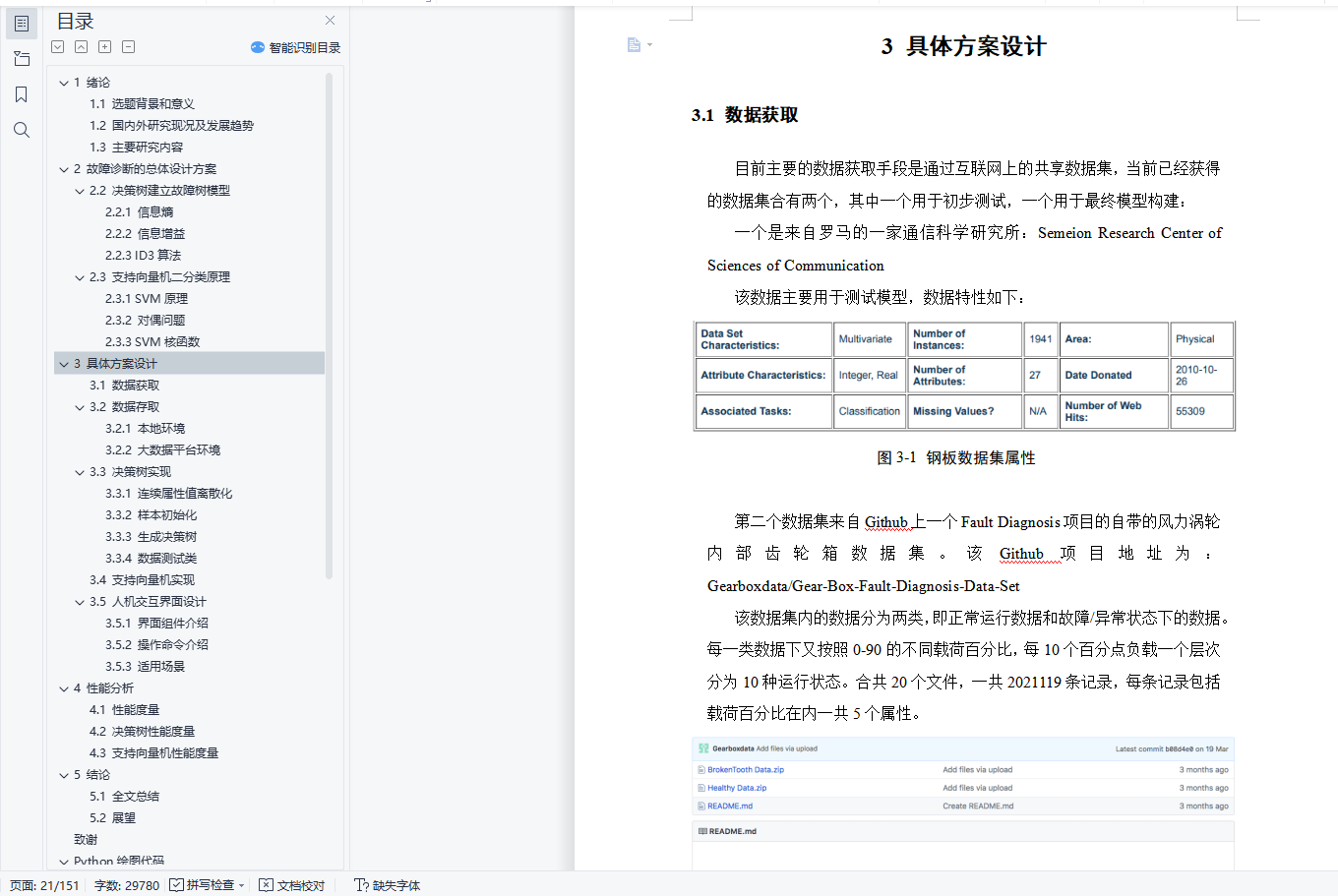
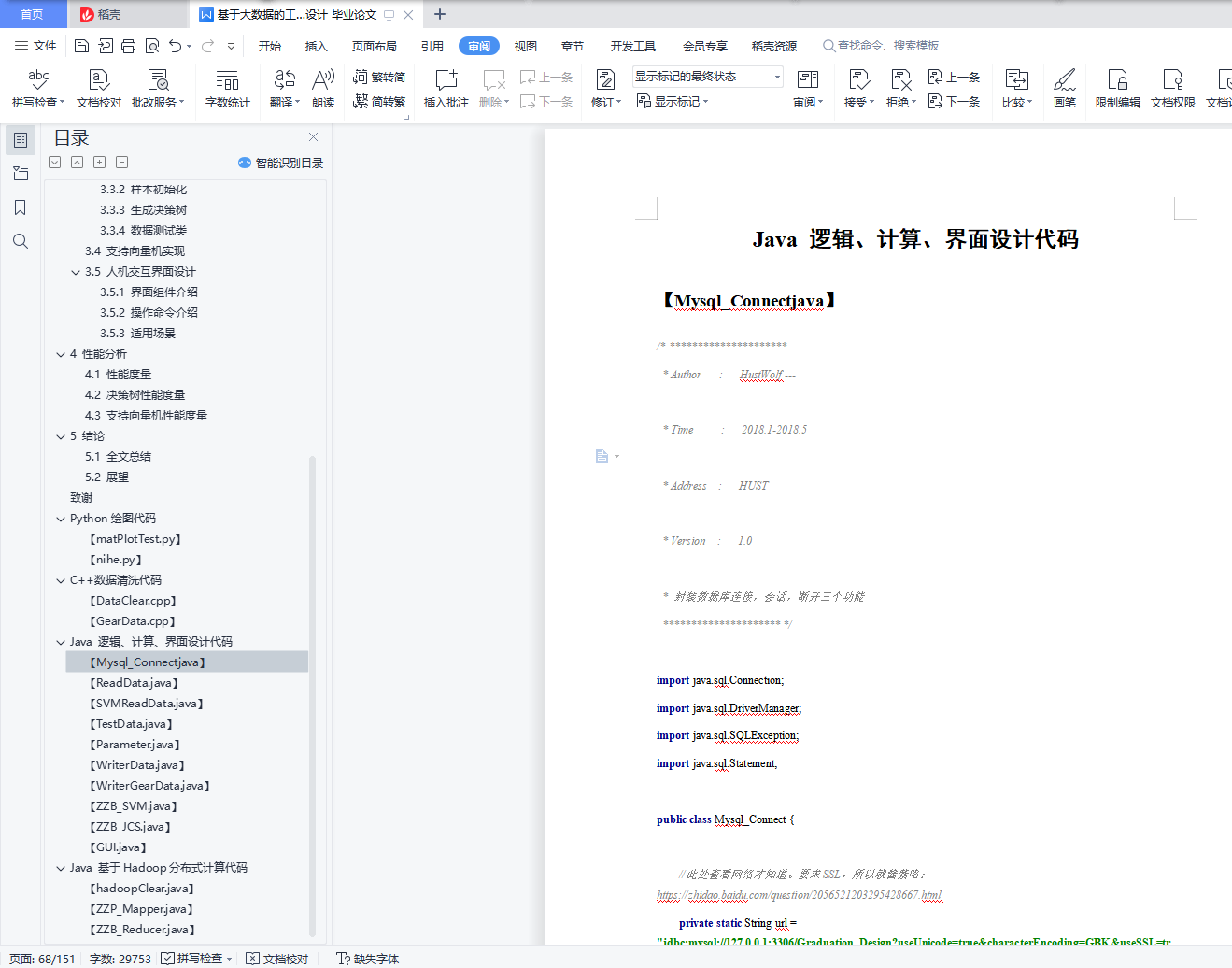
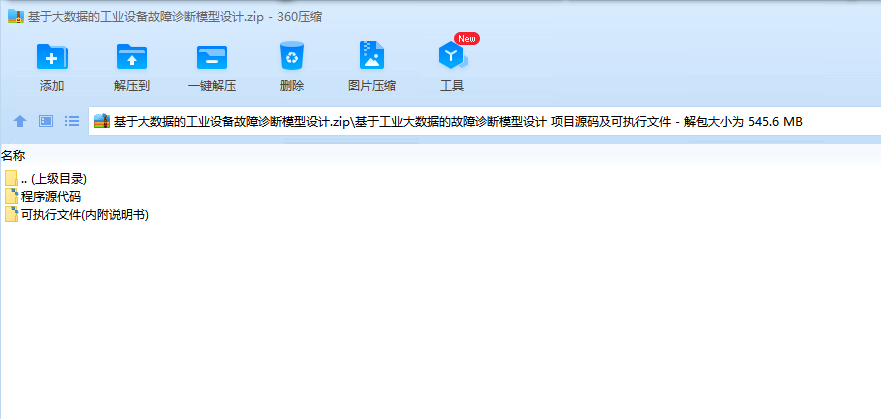
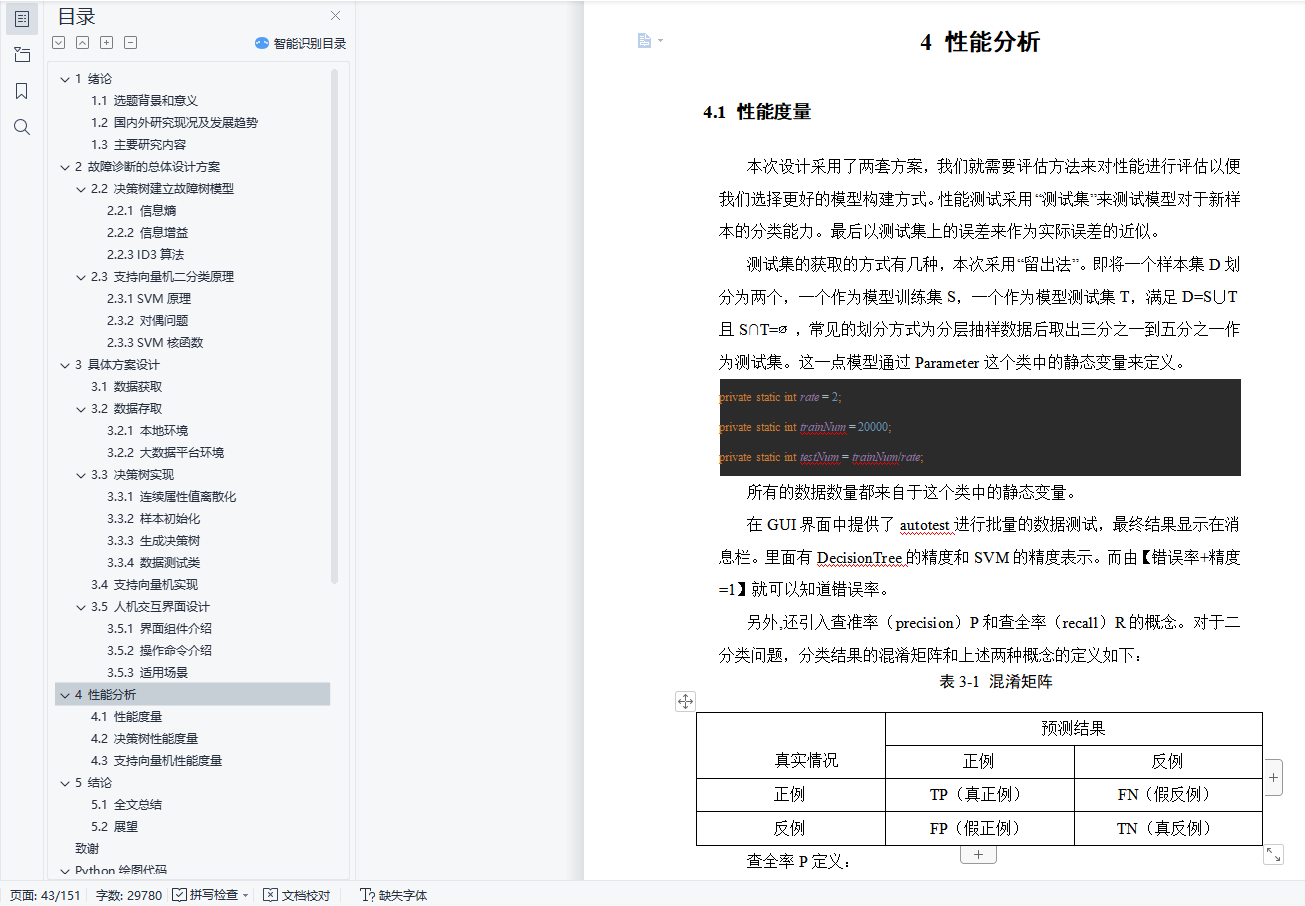
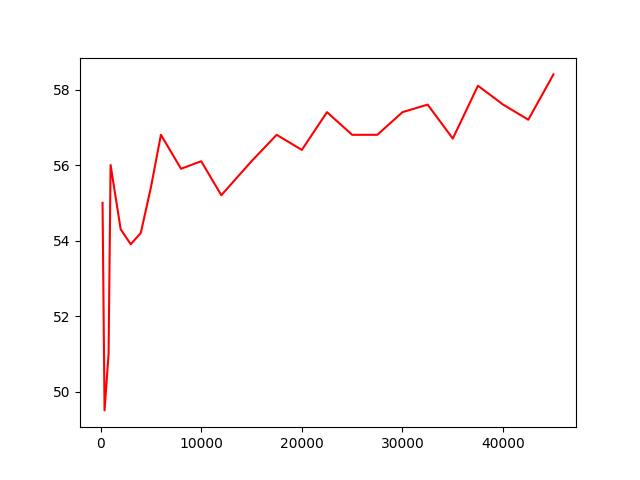
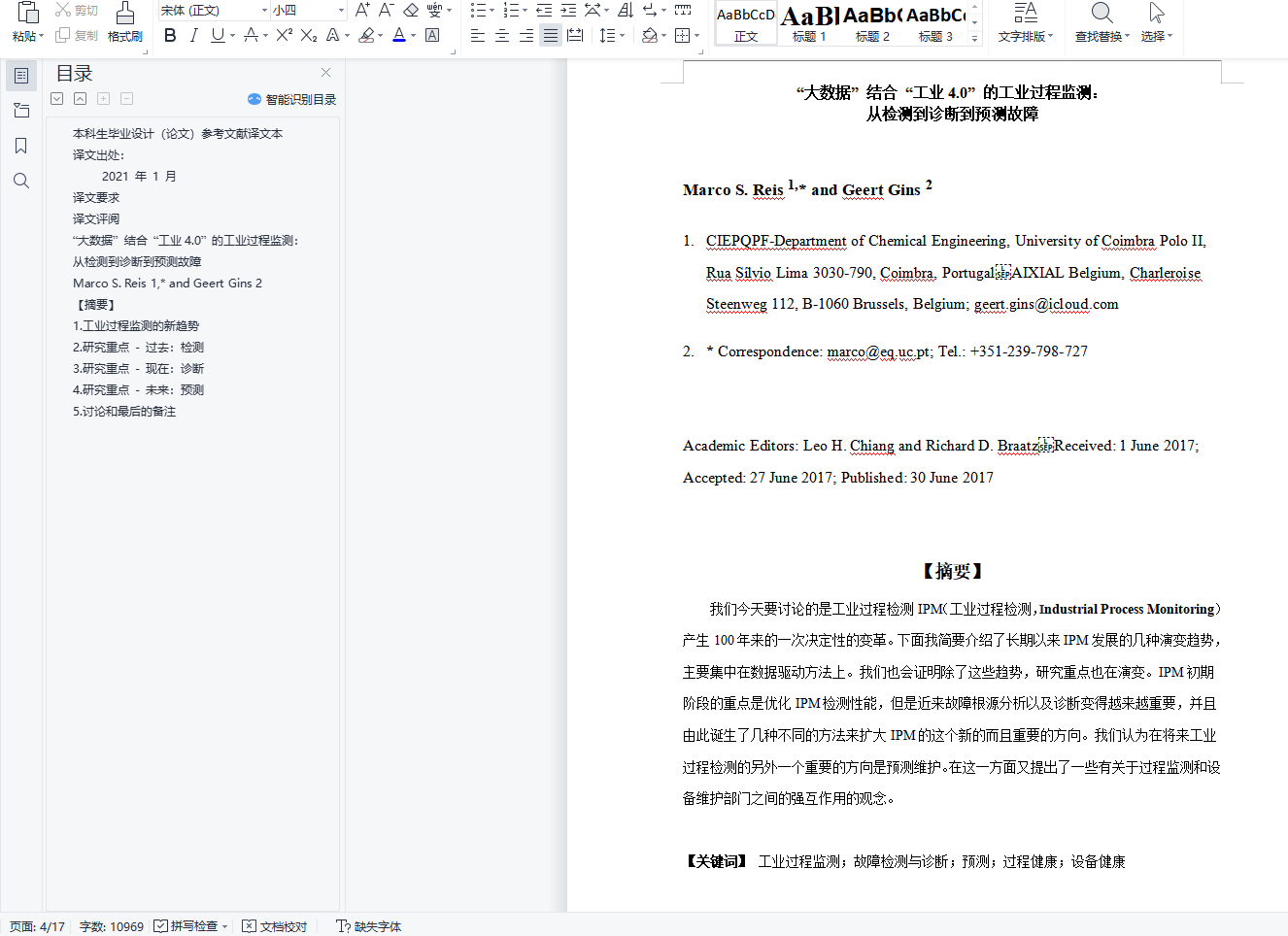
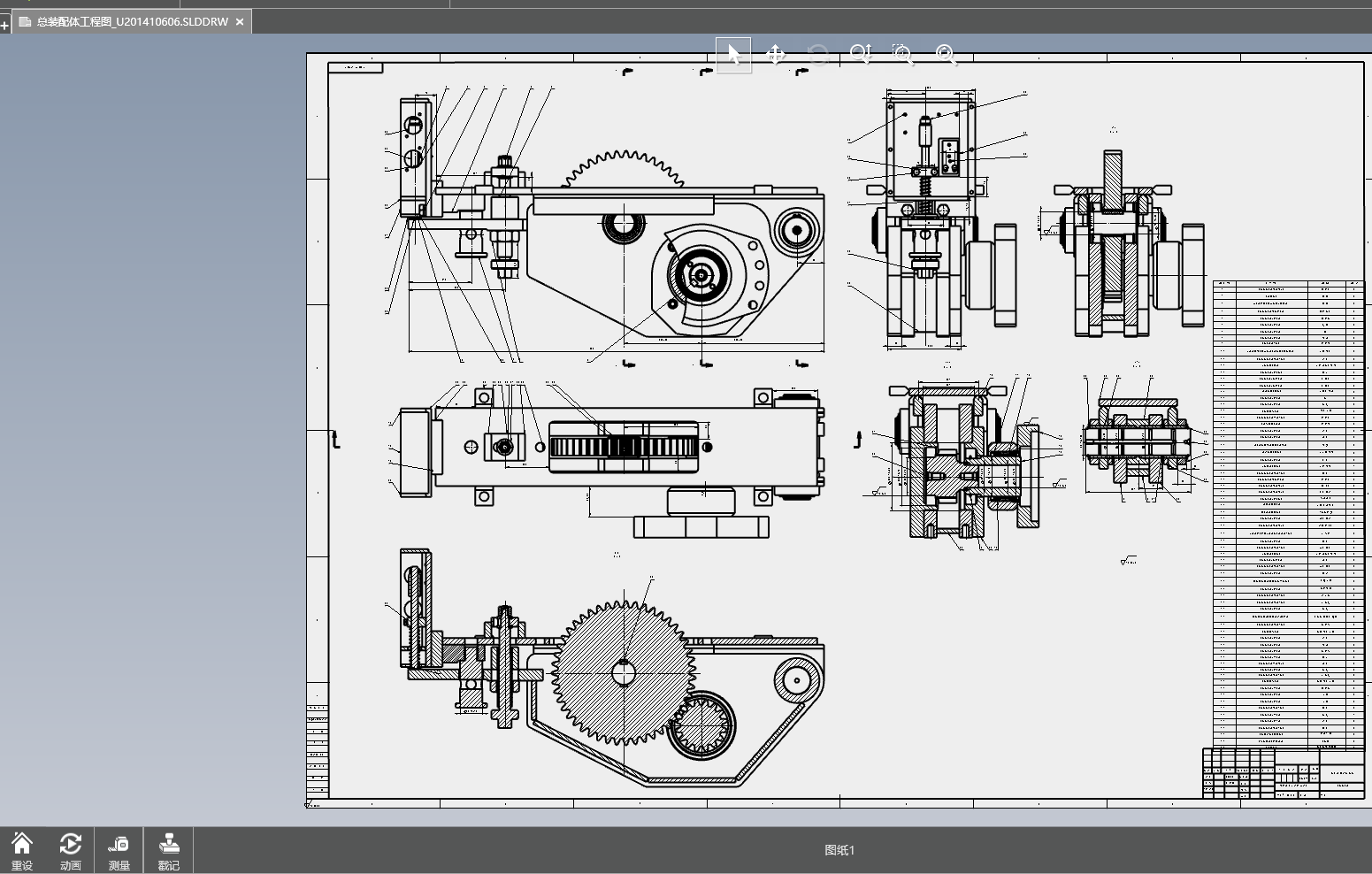
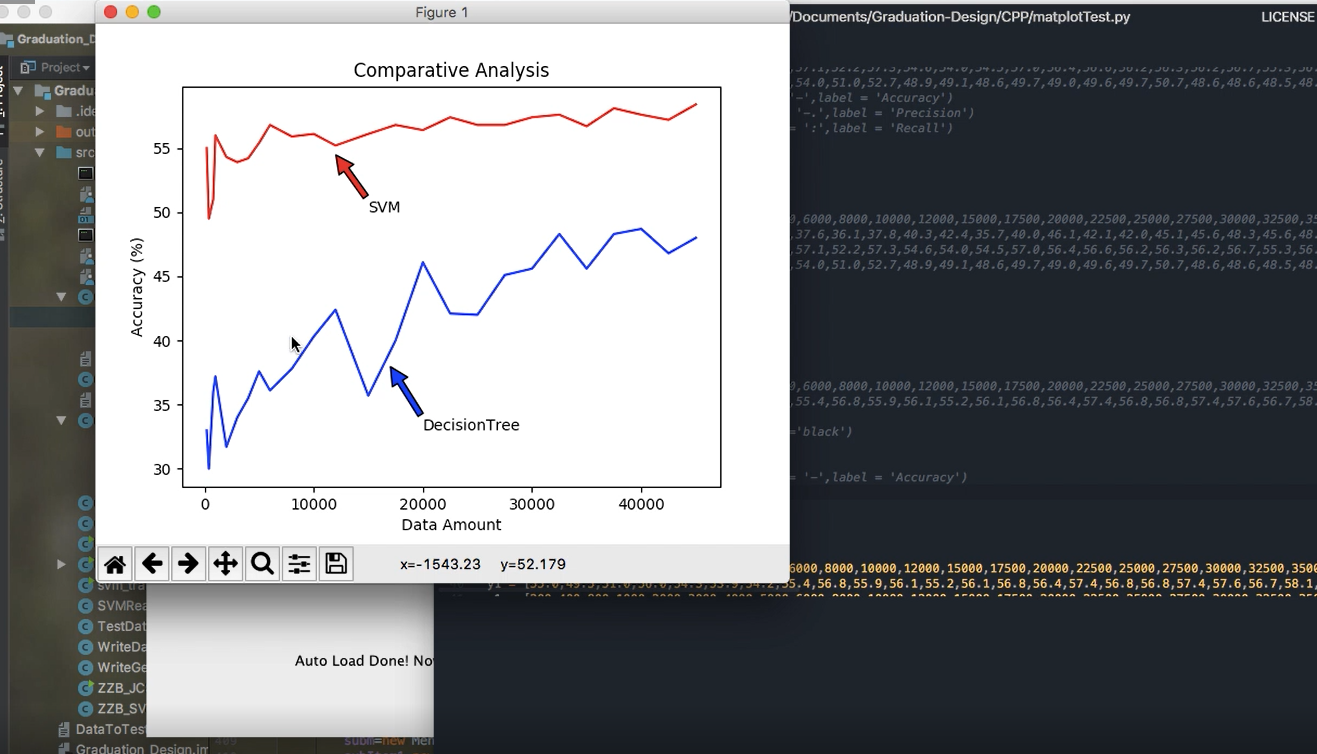
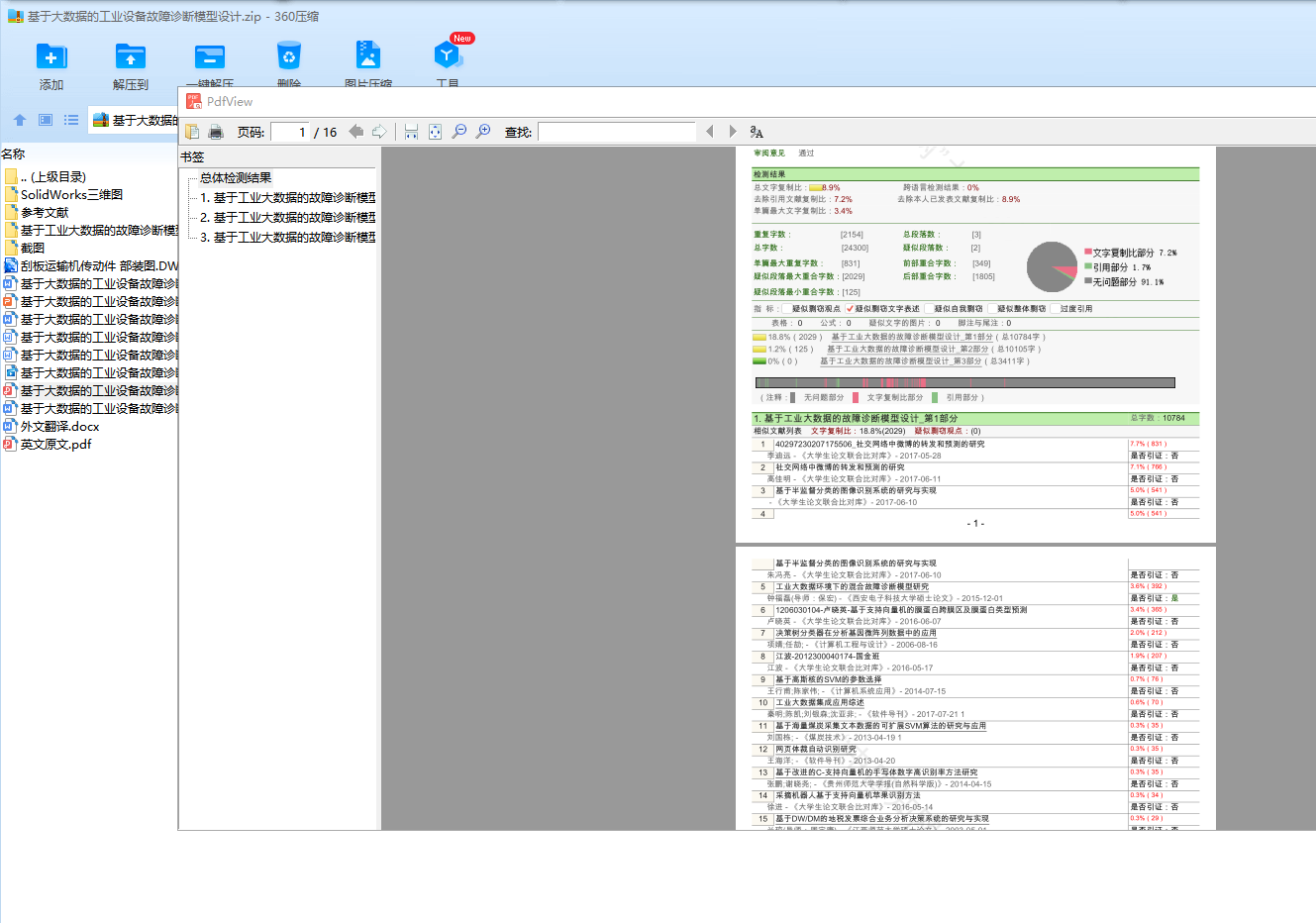
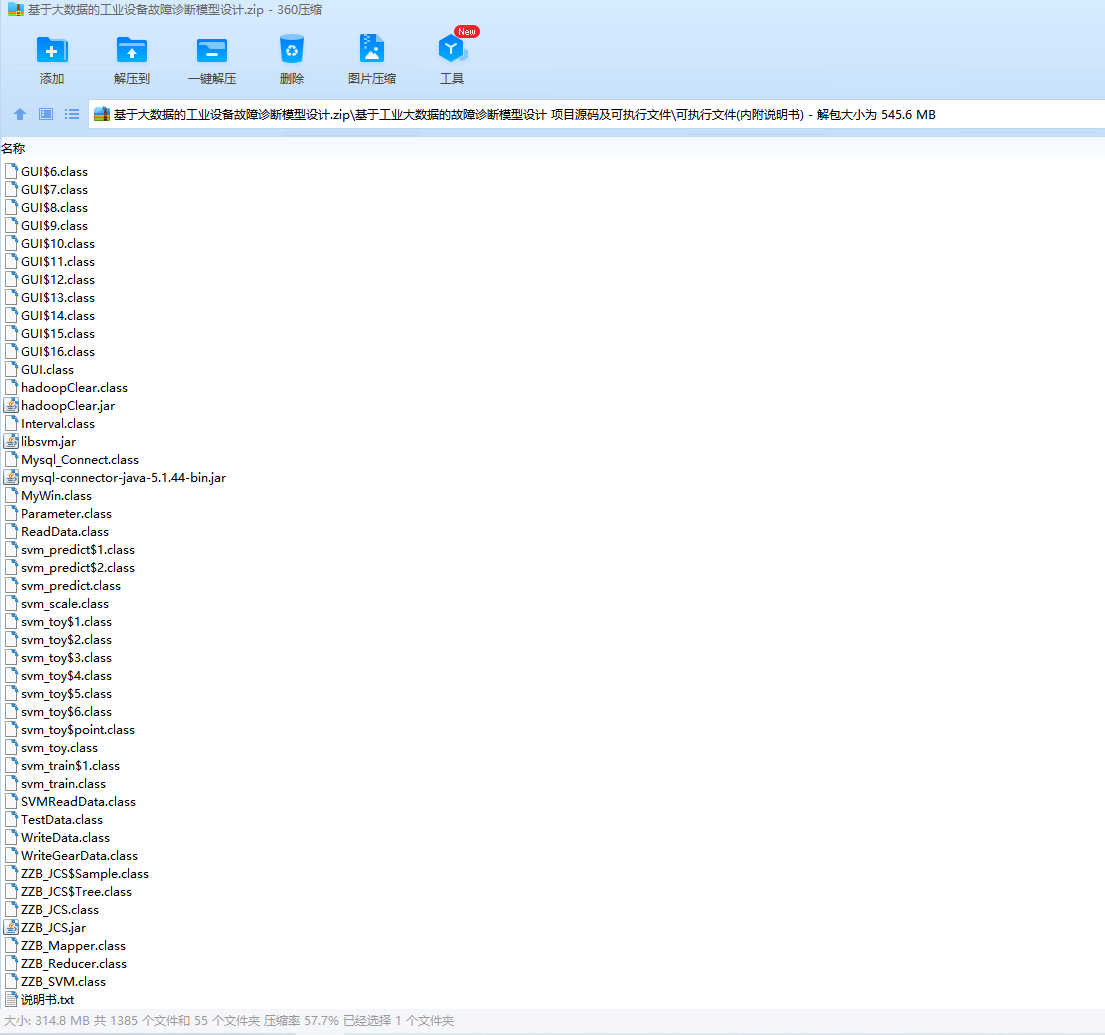
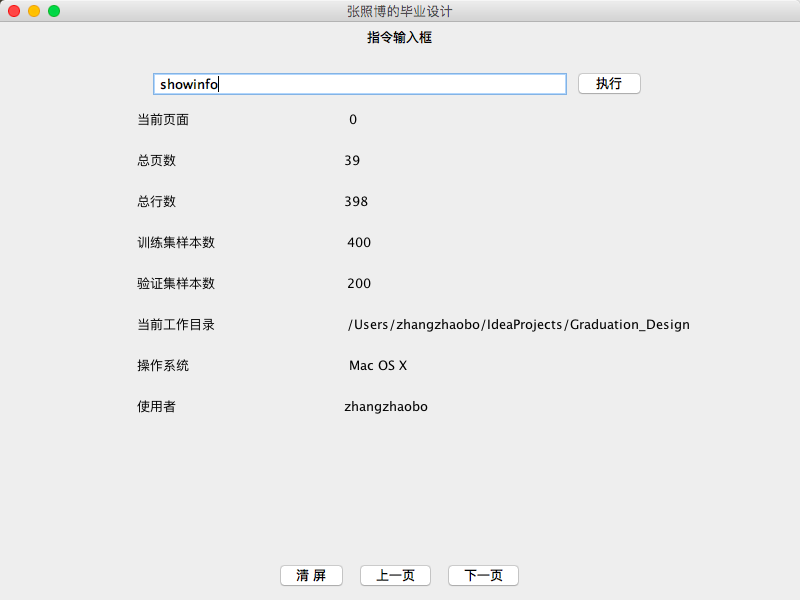
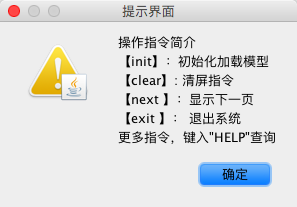
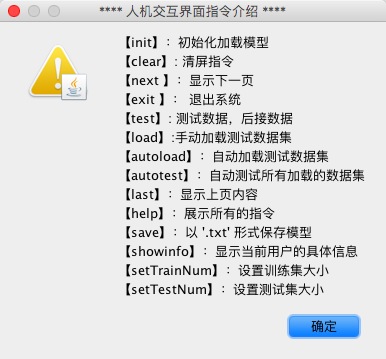
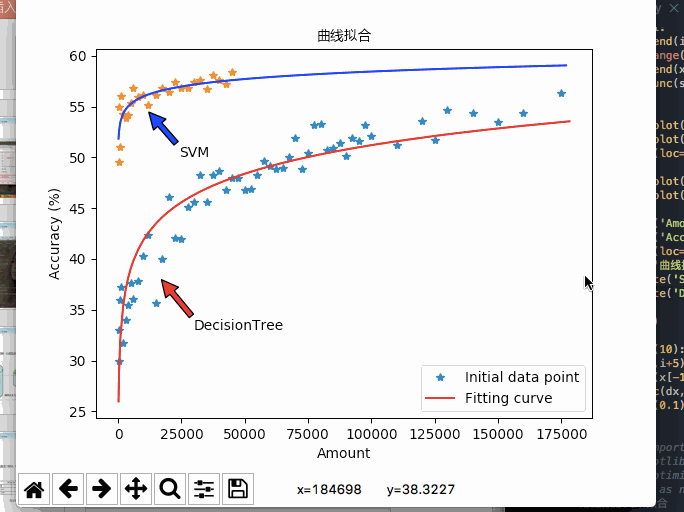
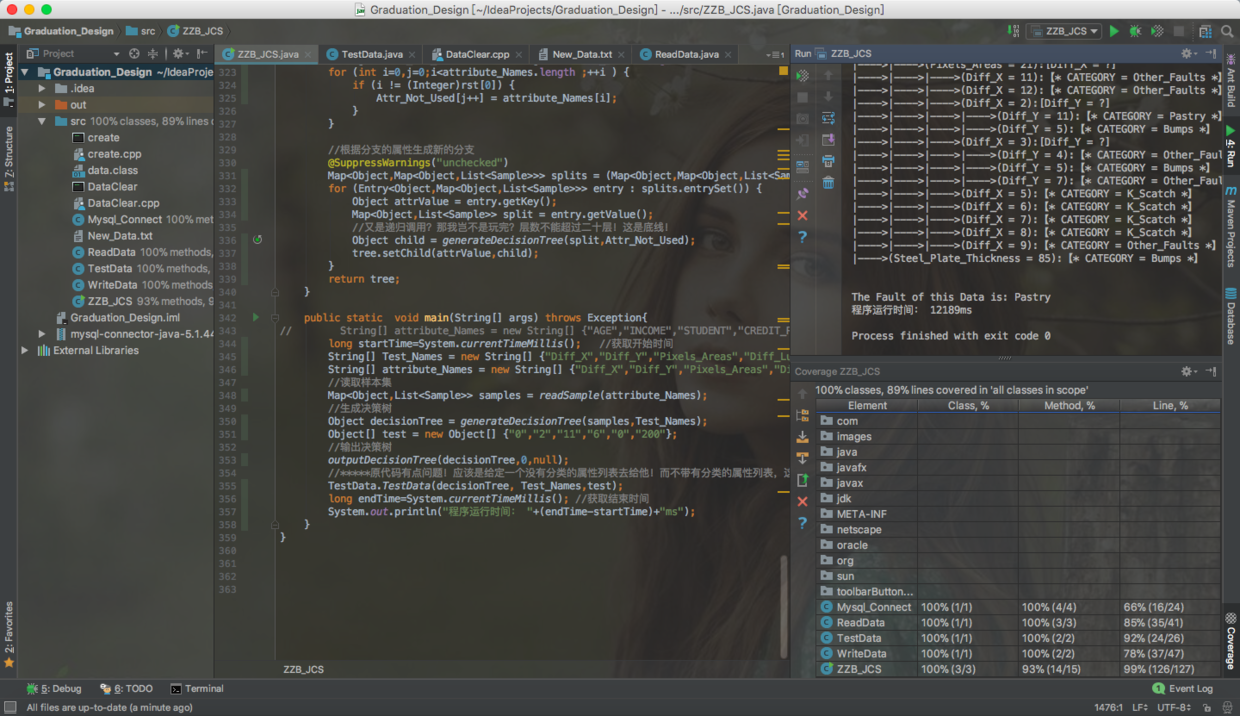