基于SVM的程序设计相关网页判别系统
摘要
ACM/ICPC是一个有着重大影响力的国际大学生程序设计竞赛,互联网亦有大量的训练习题与解题报告。有些学校会建设自己的网站用于收集解题报告,供师生参考学习。该系统可服务于这样的网站,用于自动化评判网页。
该判别系统接受一个url(或本地网页)作为输入x,判别后输出结果,一个重要的参数为字符串y,用于阐明该网页的类别。记集合A={网页|它描述的是计算机数据结构编程的相关内容},那么y为“yes”或“no”,前者表示x∈A;后者表示x∉A。
该系统可分为三个模块。
收集模块用于网页收集与标注,实现网页到原始训练集的处理。
训练模块以上个模块的输出作为输入,先后进行中文分词处理、初始特征空间统计,并通过卡方检验得到抽取后的特征空间,由此可计算每篇文档的特征向量,归一化后送往SVM进行训练。这是一个机器学习过程,通过对径向基核函数较关键的参数gamma与C调优,得到训练好的模型。
判别模块通过web页面与用户交互,可接受url或本地网页文档作为输入,输出结果包括网页的标题、正文、分词结果、特征向量、判别结果、判别结果准确率估计这6个参数。除了与人交互外,判别模块同时也提供了web 服务,可以供其他代码跨平台批量调用。
关键词:程序设计,支持向量机,文本分类,机器学习,统计
A System Based on SVM to Judge the WebPage about Programming Design
ABSTRACT
ACM/ICPC is a famous international collegiate programming contest. There are many exercises and problem-solving reports in the Internet. Some schools would build their own websites to collect them for teaching, and this system is helpful to judge the webpages automatically.
This judge system accepts an url (or a local webpage) as the input x, then outputs a result which contains an important parameter y, indicating the type of this webpage. We assign set A={webpage | the content it describes is relative to computer programming or data structure}, so y is "yes" or "no". The former means x∈A;and the later means x∉A.
This system is divided into 3 parts. Collecting module collects and labels the webpages. Namely, it transforms webpages to raw training set.
Training module uses the former module's output as input, then complete following tasks:Chinese word segmentation, raw feature space statistics, Chi-square testing, feature vector caculate per document. Next sends the vector set into SVM after being normalized to train. This is a machine learning procedure, gaining a module by adjusting parameters gamma and C of the RBF.
Judging module’s output contains title, content, word segmentation, feature vector, result and probability estimate. In addition, this judge system provides web service, which makes it convenient that other codes’ cross platform and batch calling.
Key words: programming design, SVM, text classification, machine learning, statistics
目 录
摘要 I
ABSTRACT II
1绪论 3
1.1 研究背景 3
1.2 文本分类研究现状 3
1.3 主要研究内容 4
1.4 本文章节安排 5
2理论基础与相关工具 6
2.1 中文分词 6
2.2 卡方检验 7
2.3 神经网络 10
2.3.1 神经网络简介 11
2.3.2核方法与径向基函数 12
2.4 支持向量机 12
2.4.1线性可分模式的最优超平面 12
2.4.2线性不可分模式的最优超平面 14
2.5 前端工具 15
2.6 后端工具 16
3需求分析 19
3.1 功能需求 19
3.2 界面需求 19
3.3运行环境需求 20
4系统设计 21
5系统实现 24
5.1 数据收集模块 24
5.1.1 web 界面实现 24
5.1.2 根据URL或本地文件解析网页 26
5.1.3 数据库设计与实现 27
5.1.4 数据库访问对象的单例实现 29
5.1.5 爬虫模块实现 29
5.1.5 收集数据说明 30
5.2 数据处理模块 33
5.2.1中文分词的IKAnalyzer实现 33
5.2.2 java 并发与同步 35
5.2.3 文档结构化存储的数据结构实现 37
5.2.4卡方检验实现 39
5.2.5 特征向量计算与归一化 41
5.2.6 libsvm工具箱的javaAPI使用 43
5.2.7 使用libsvm进行训练 46
5.2.8 机器学习效果评价 49
5.3判别模块 49
5.3.1用户交互web界面实现 50
5.3.2 文件上传功能实现 53
5.3.3 web service调用 53
6总结与展望 55
6.1 本文工作总结 55
6.2 未来研究展望 55
参考文献 57
致谢 58
翻译原文 59
译文 75
附录 86
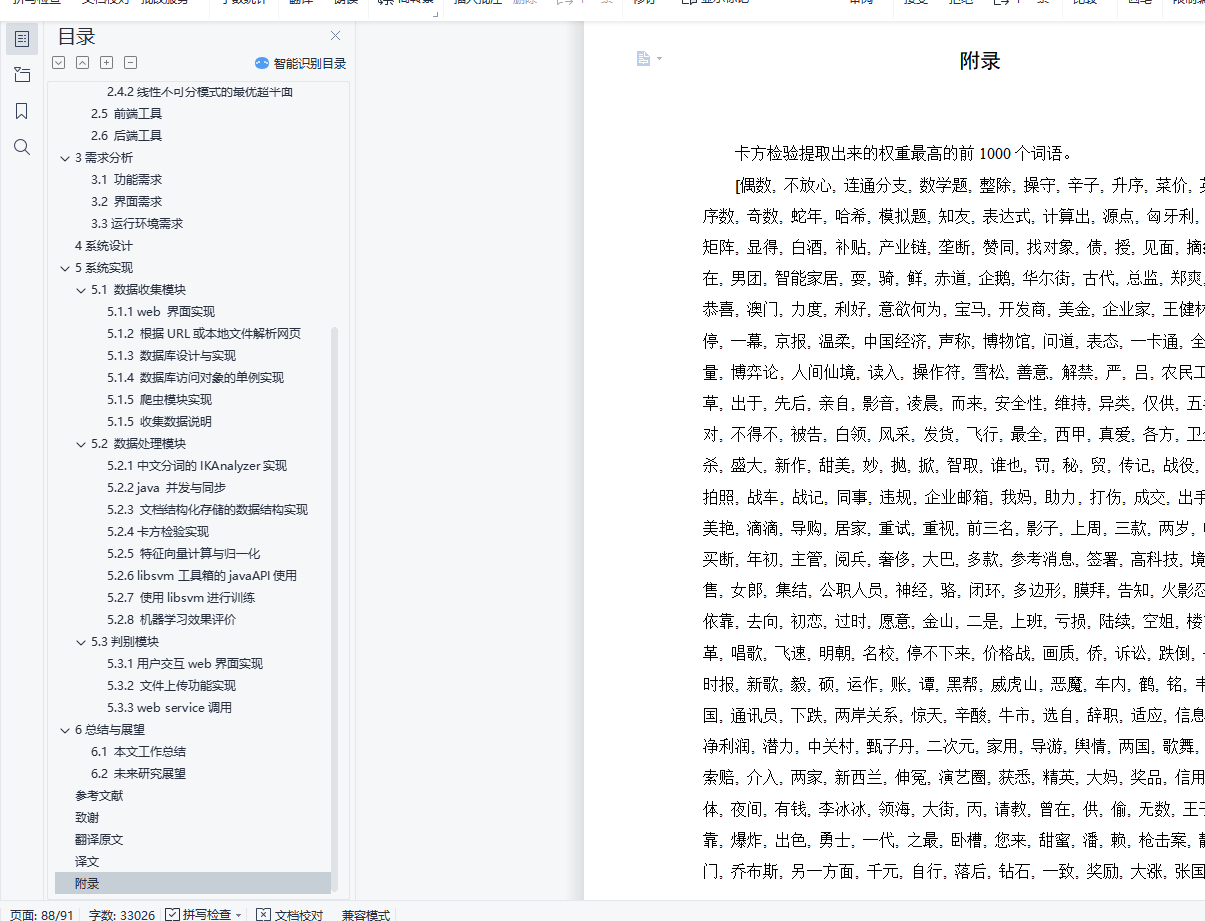
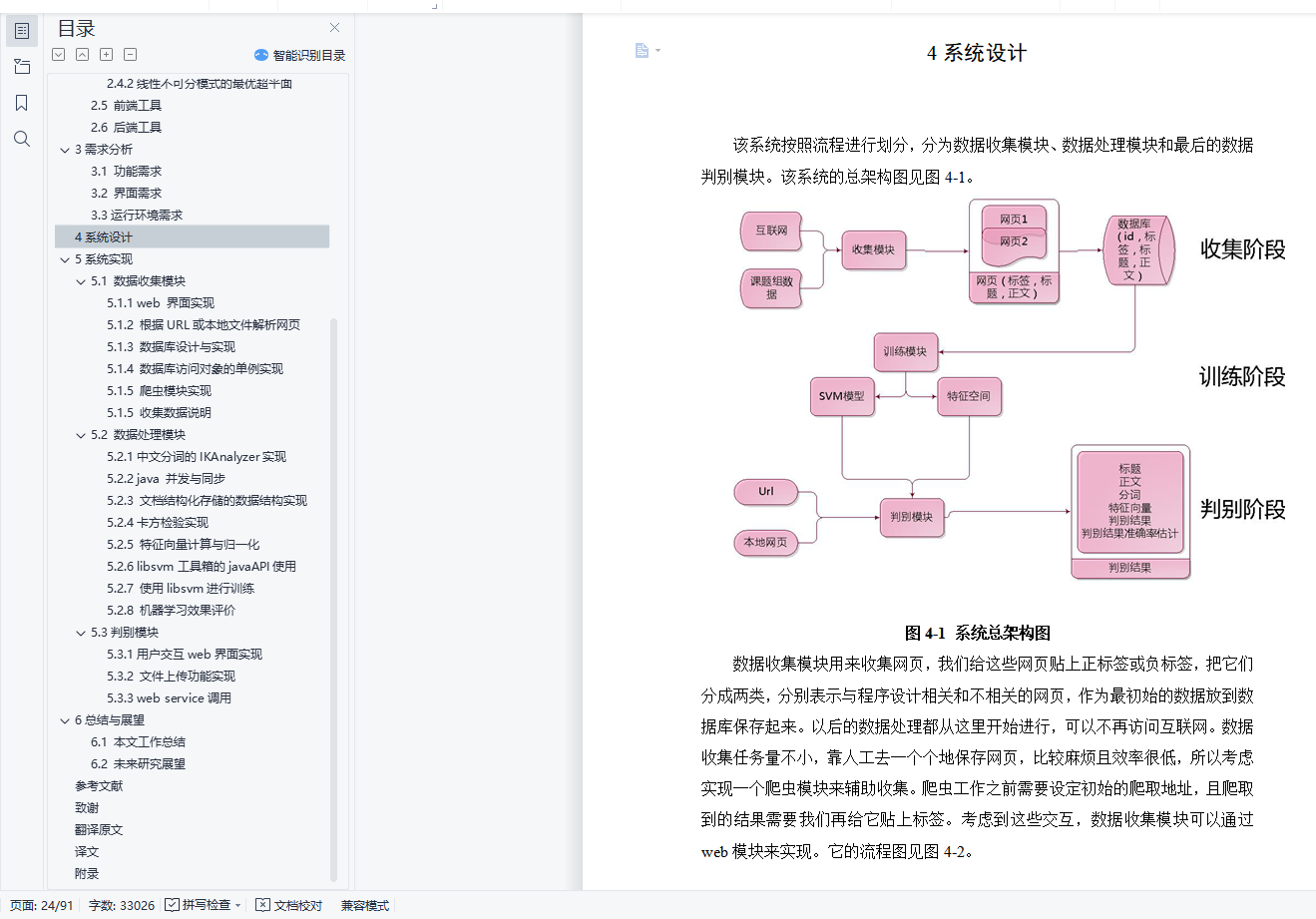
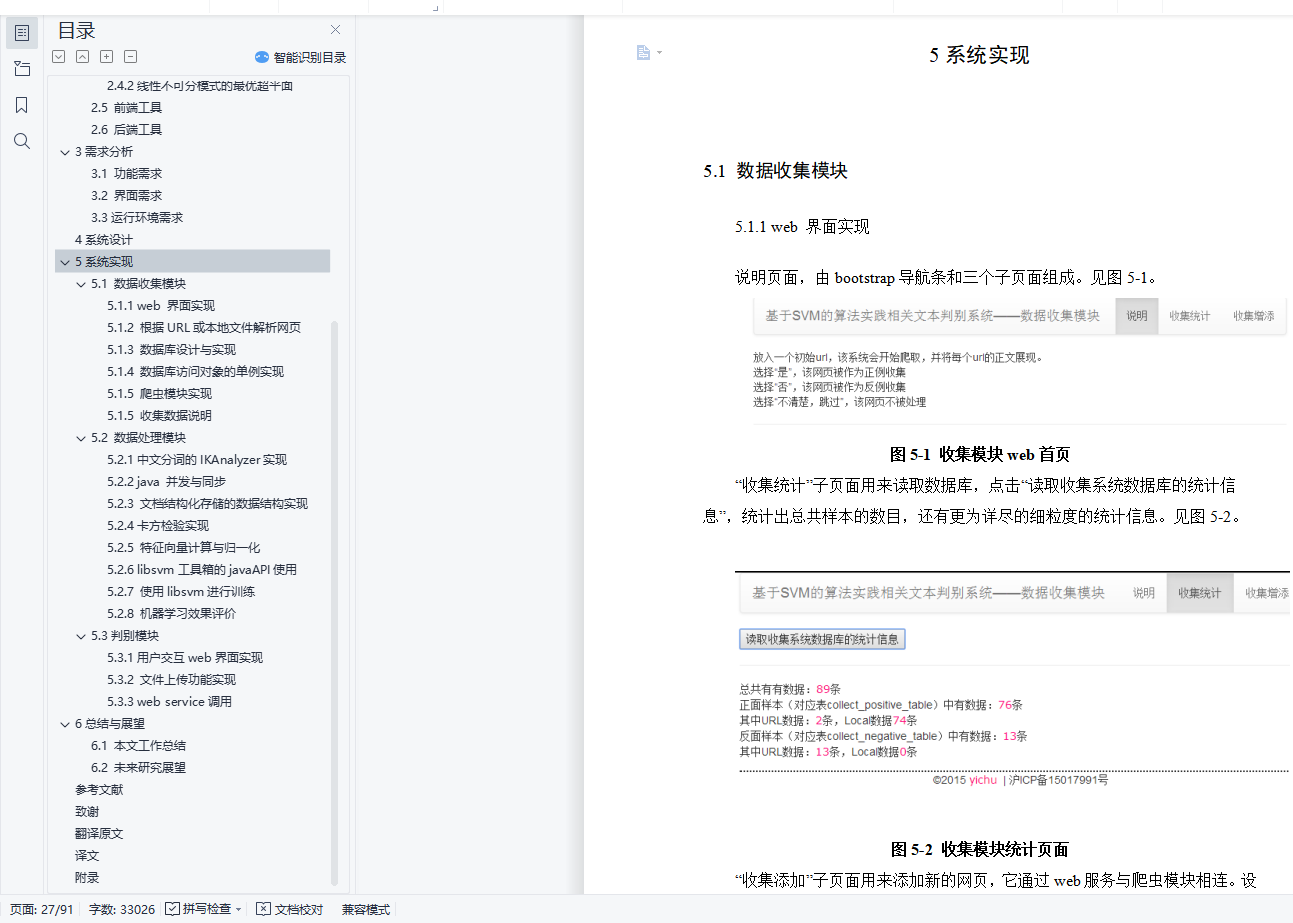
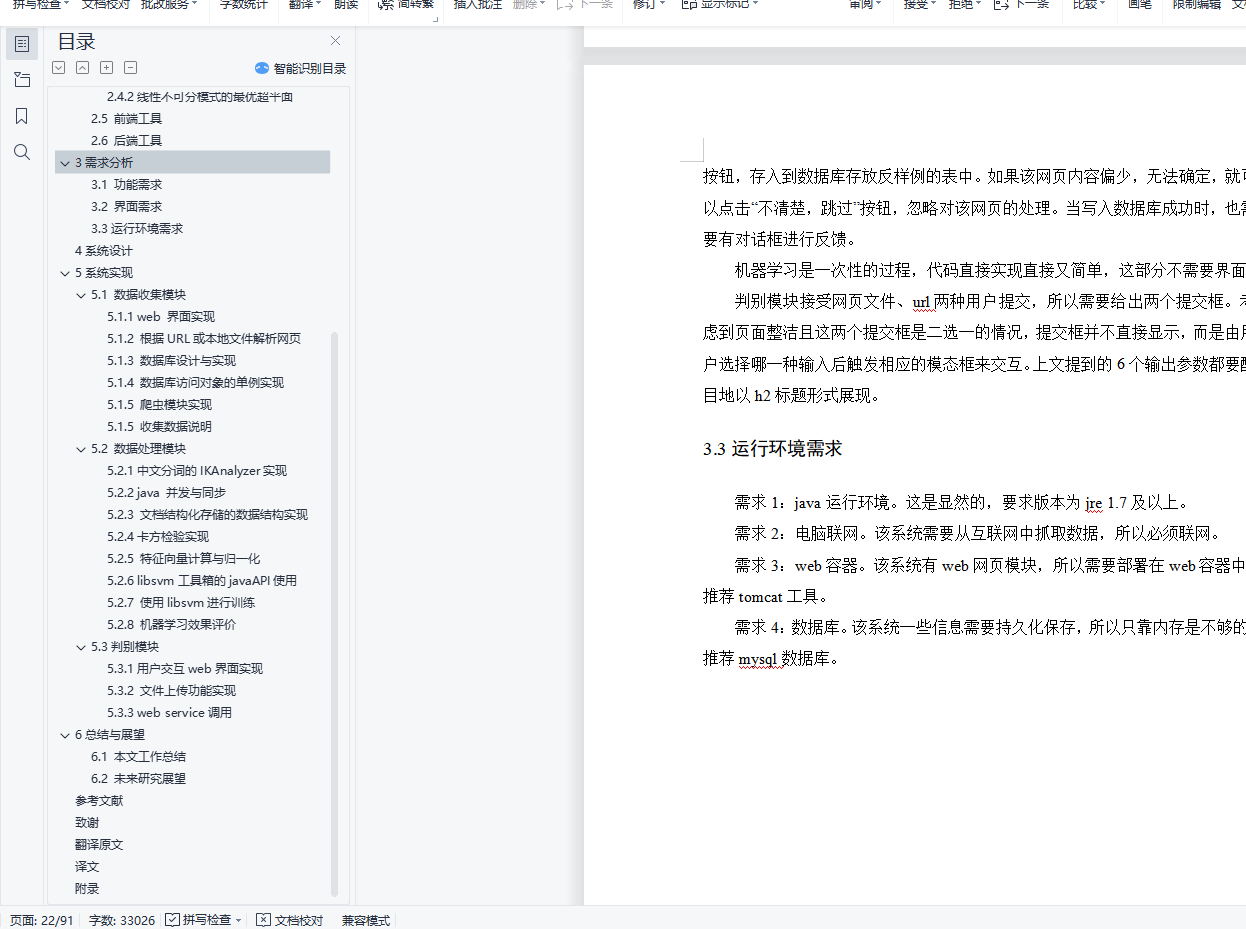
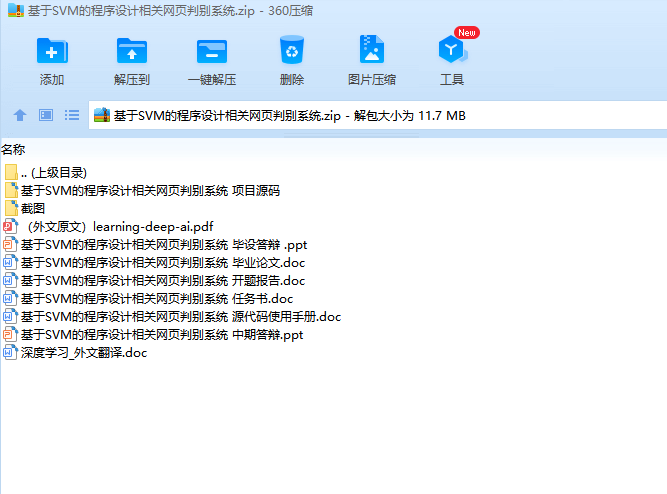
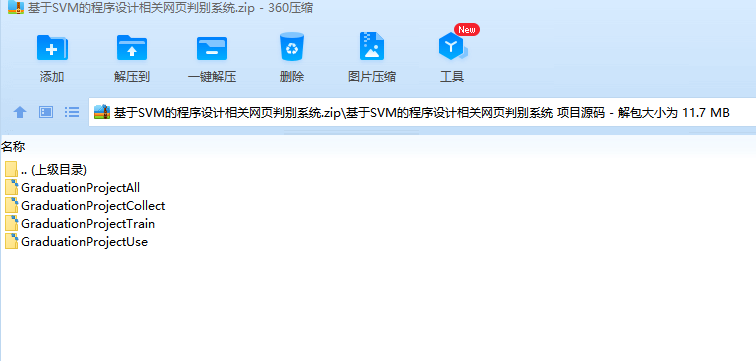
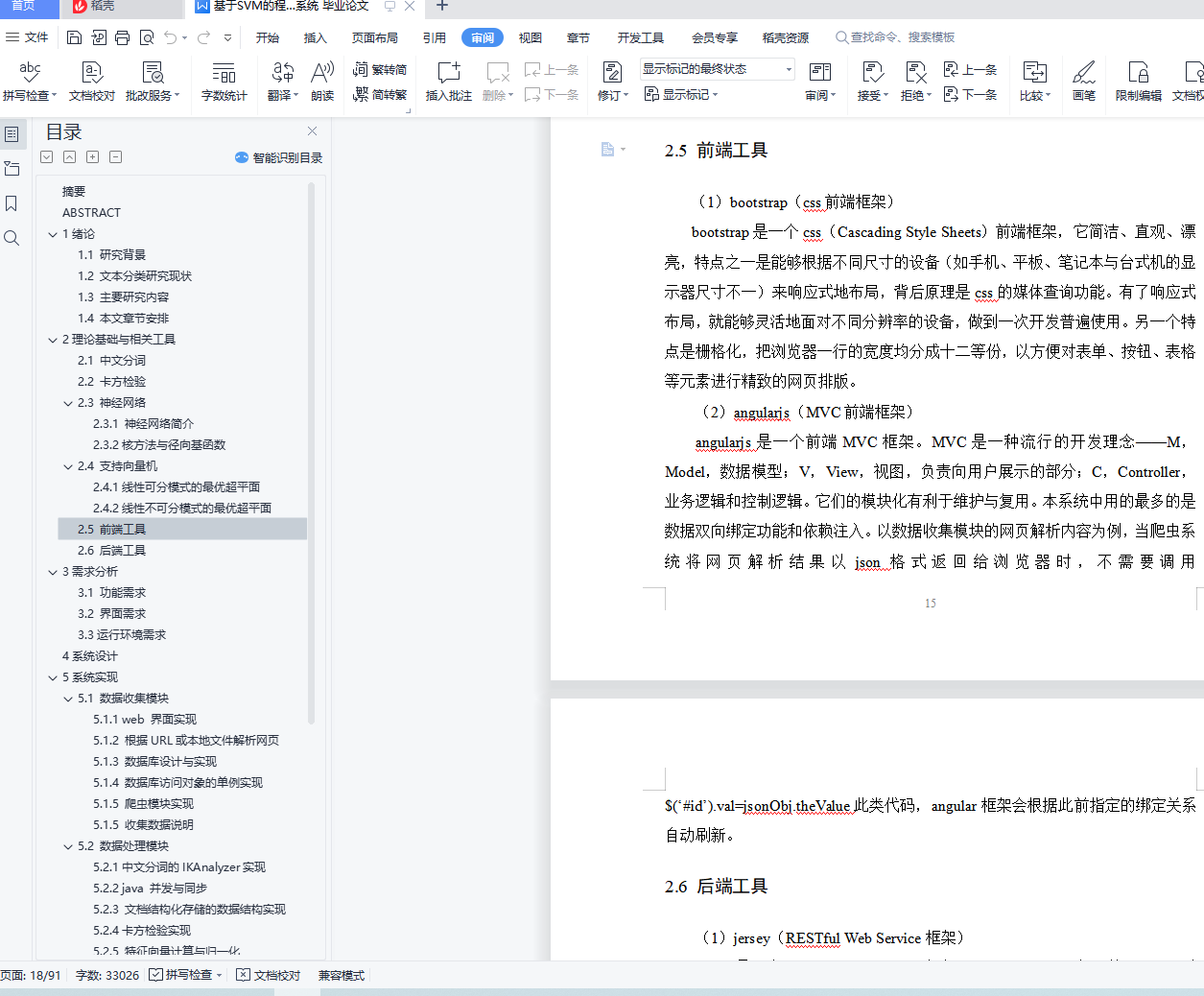
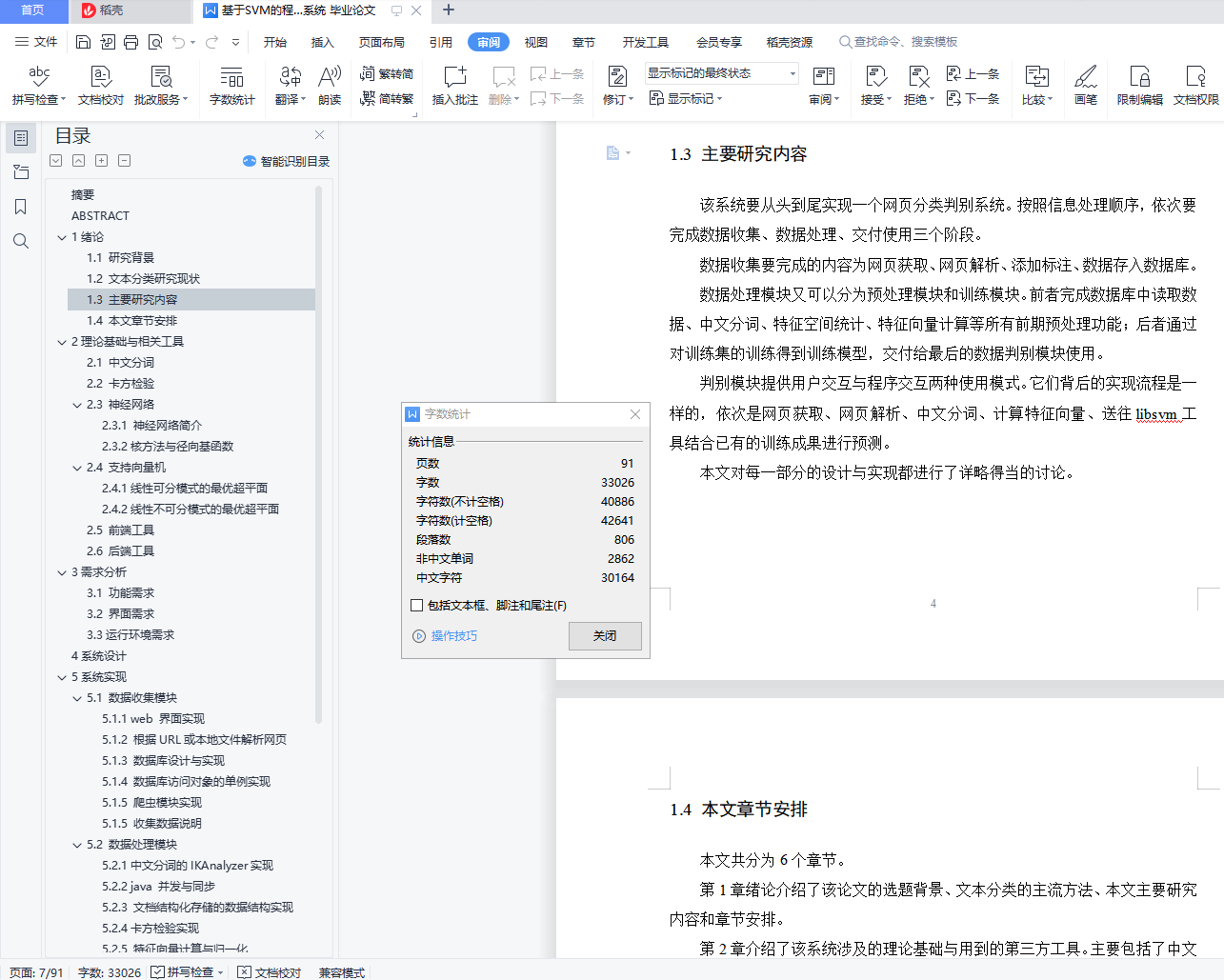
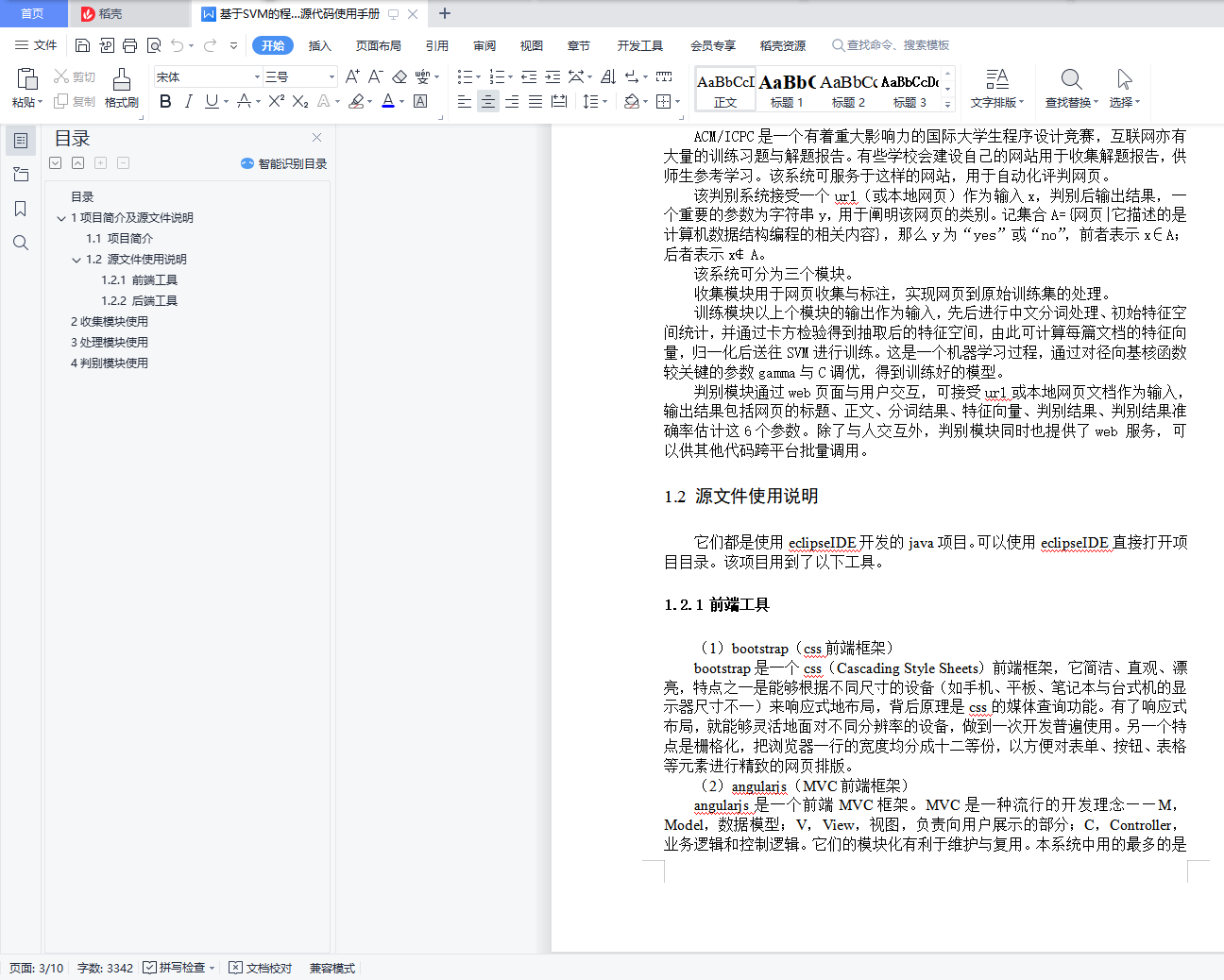
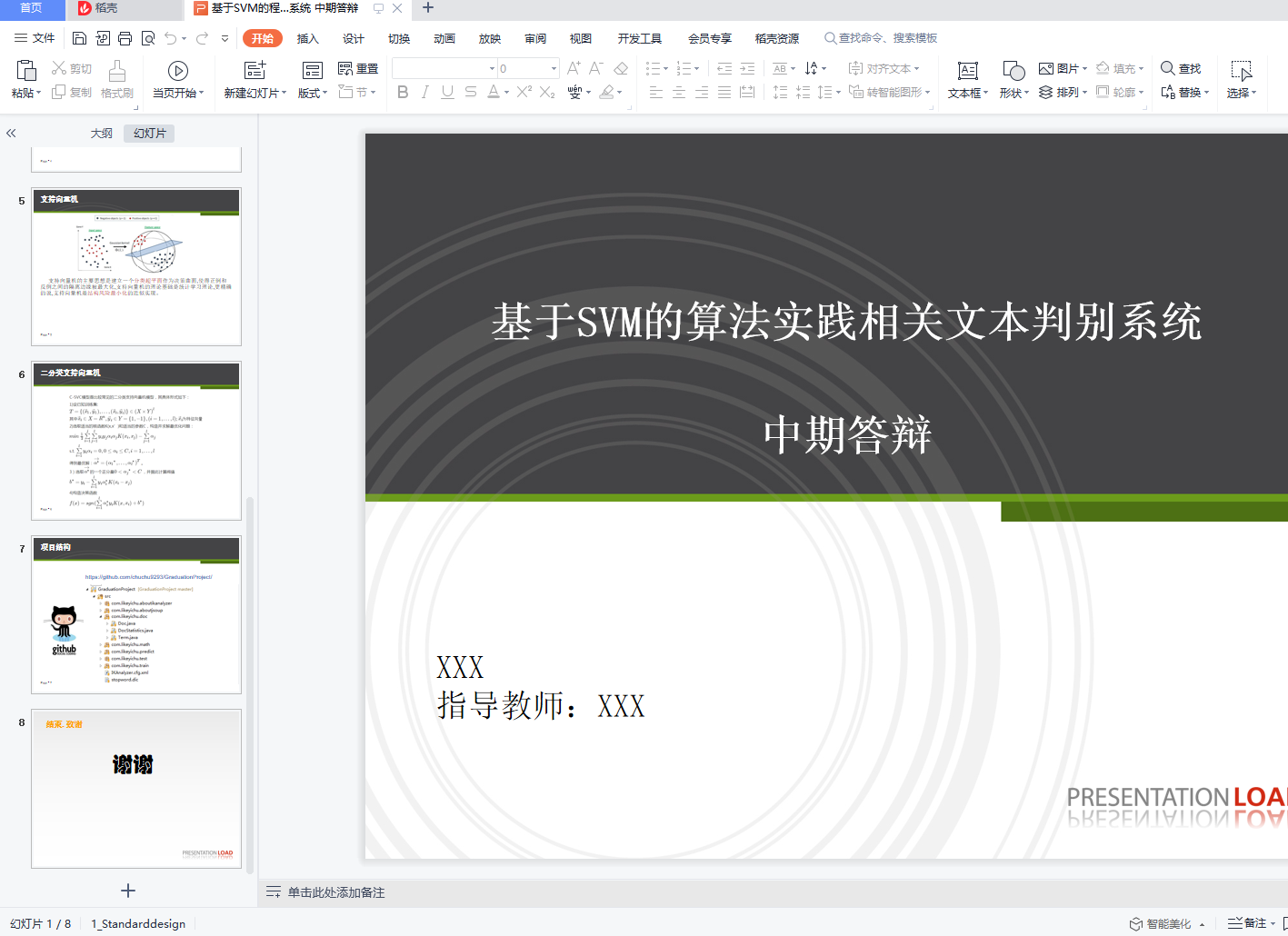
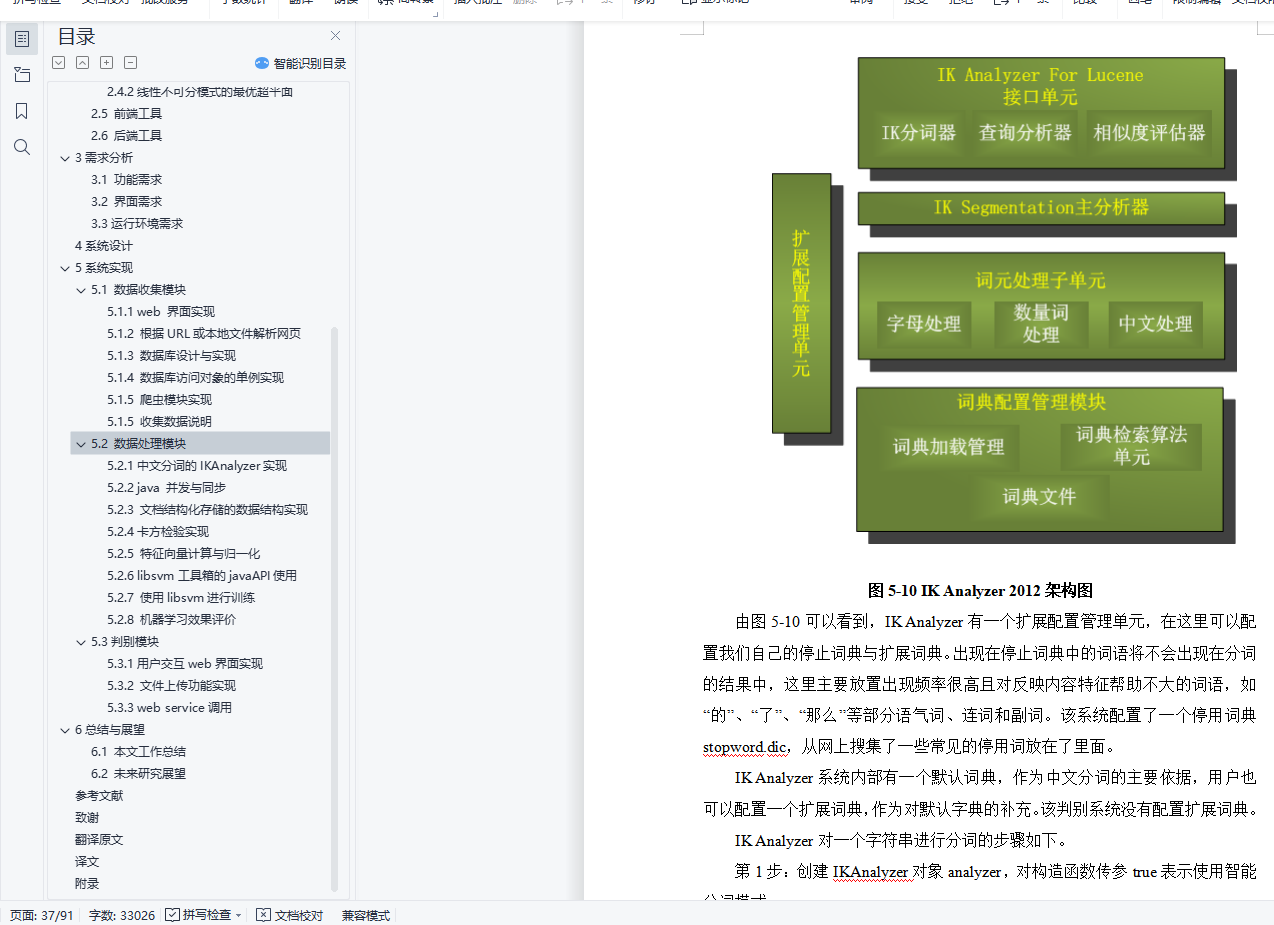
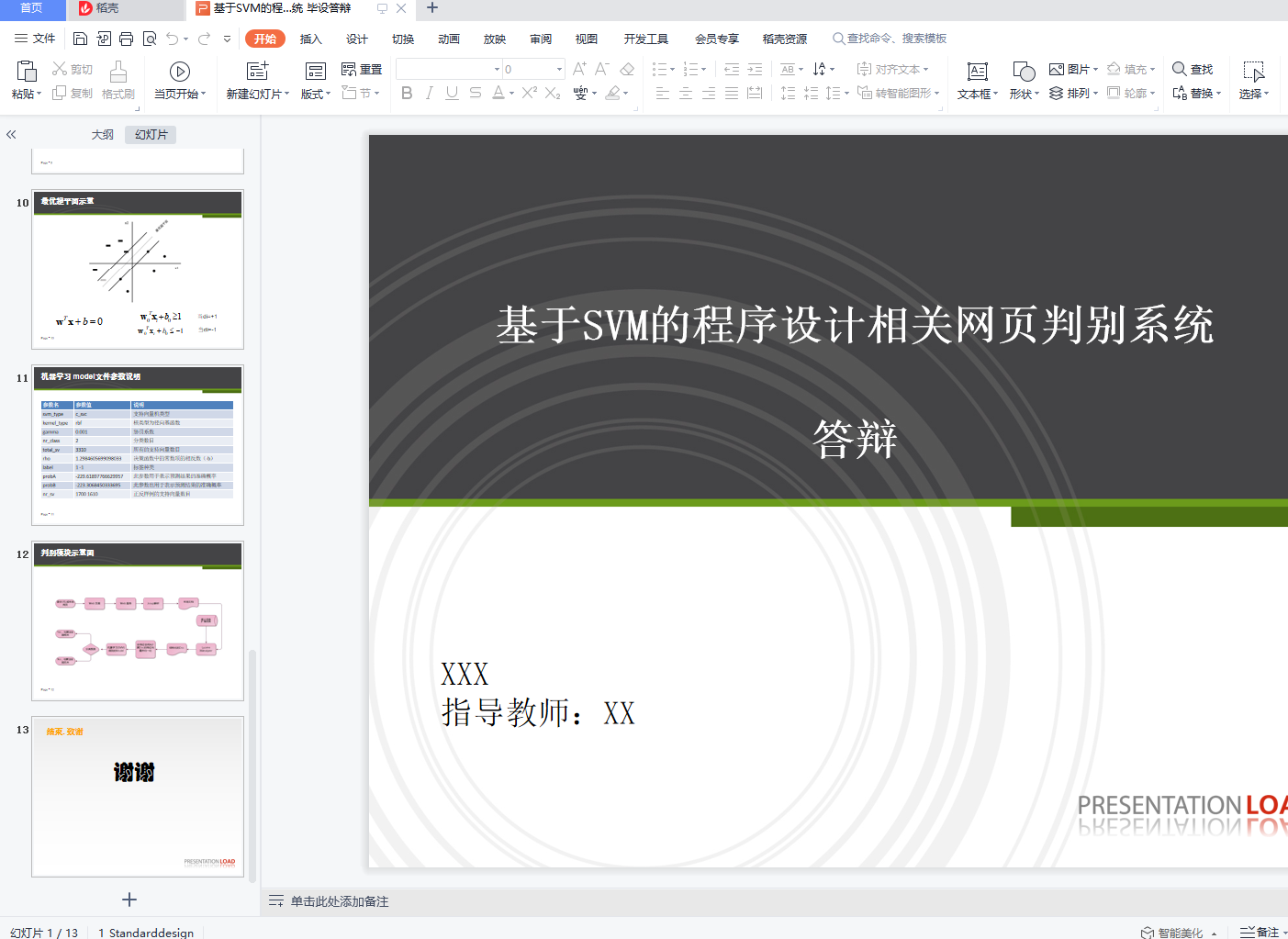
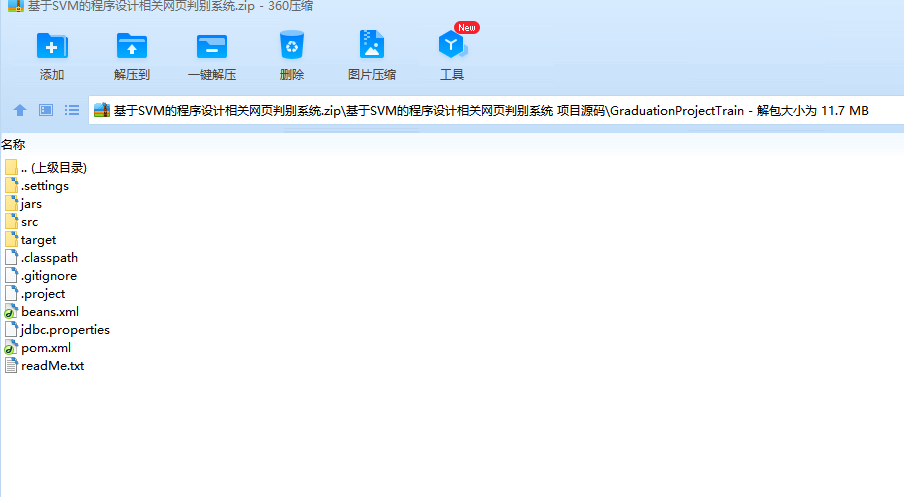
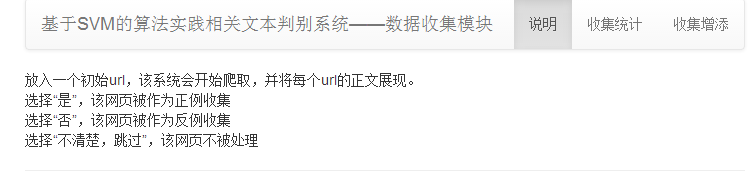
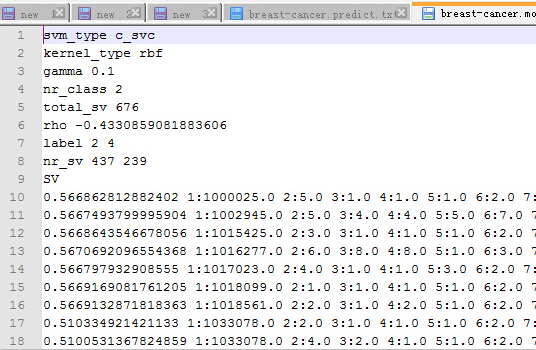
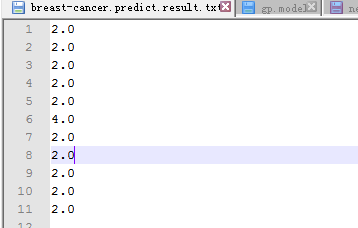
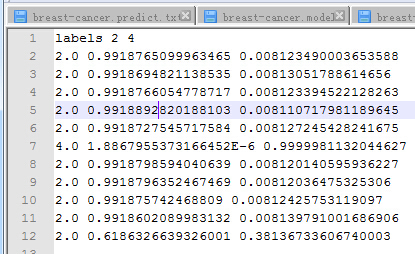
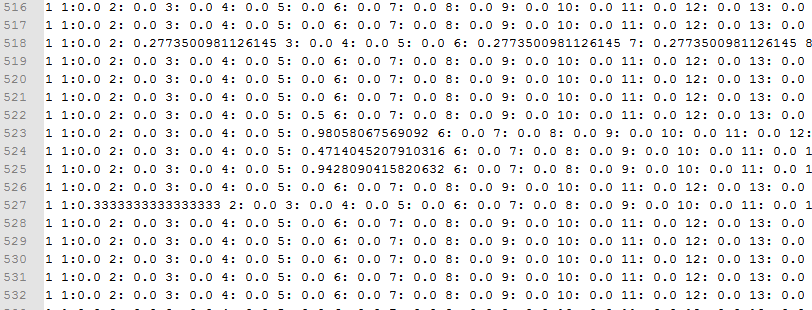
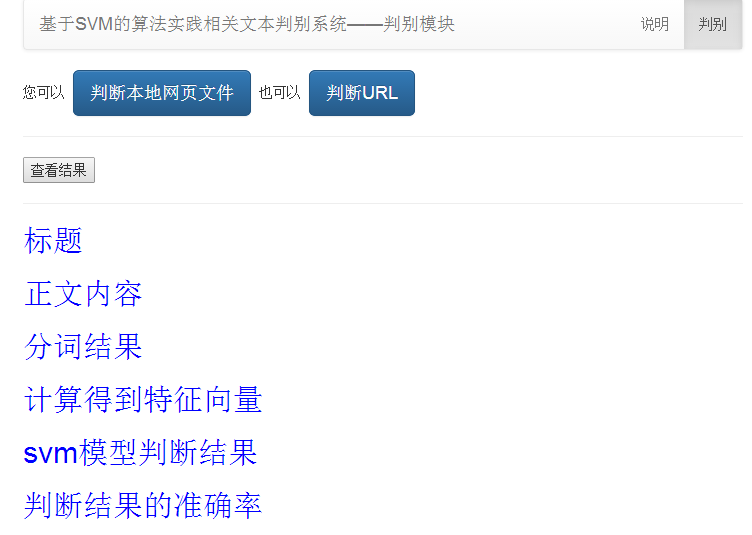
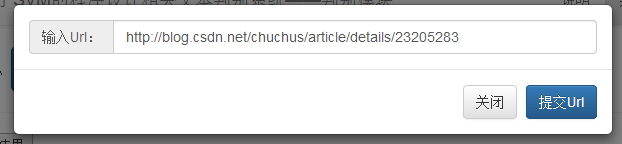
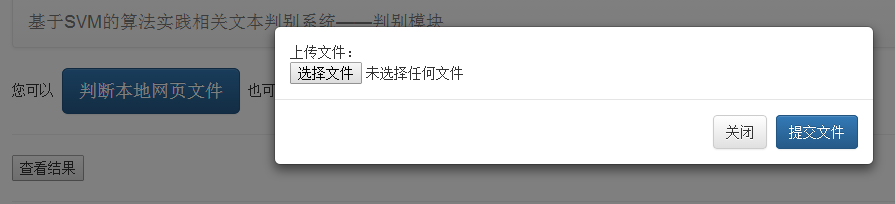
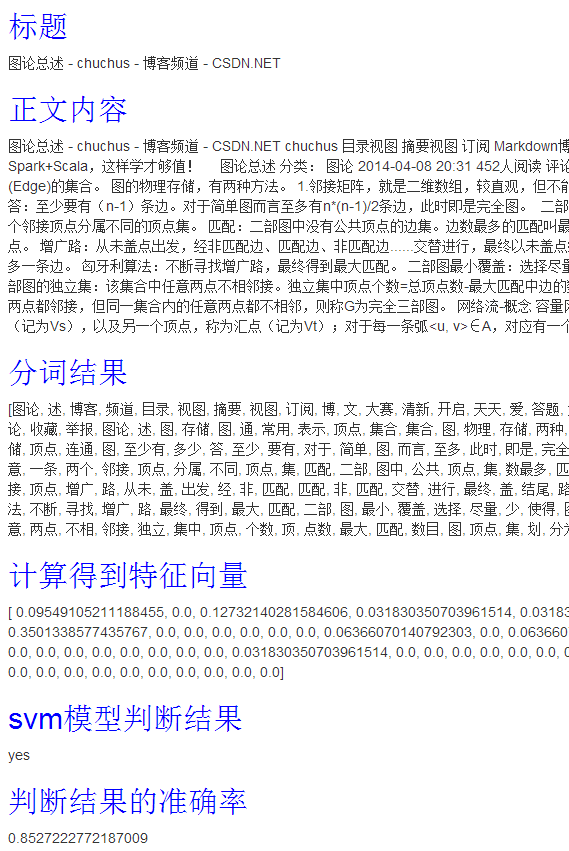
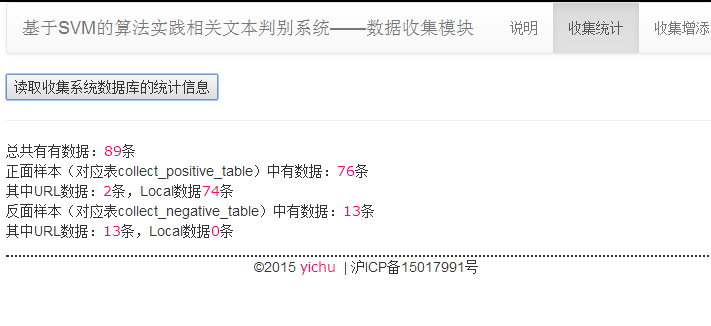
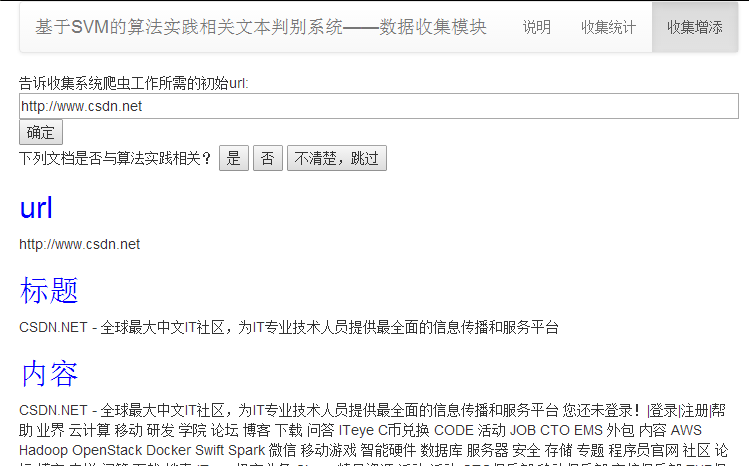
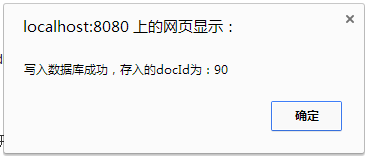
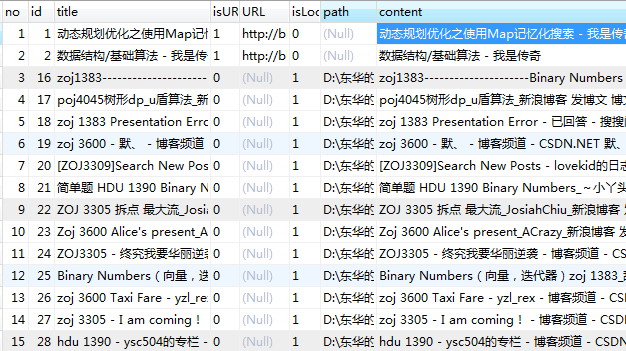
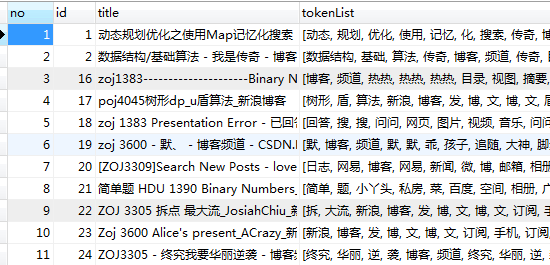

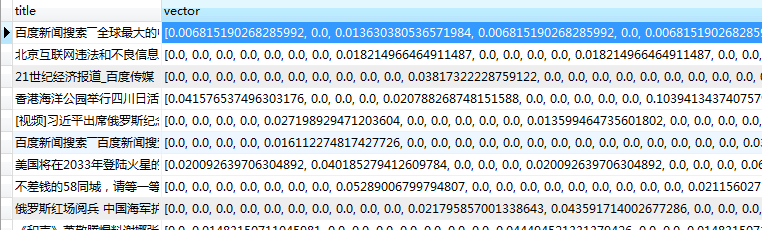
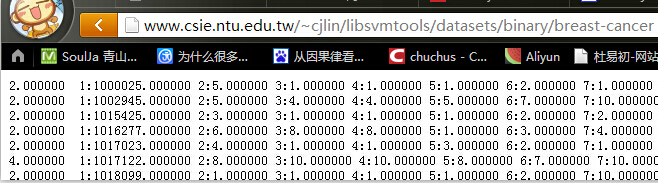