摘 要
随着科技的快速发展和存储技术的飞速提升,使得我们生活在了数据的海洋,各行各业都积累着海量数据。我们如何利用这些数据,发掘出隐藏在其中的有价值的信息是数据挖掘诞生的重要因素。上世纪90年代,沃尔玛在其海量交易数据中发现了经典的“啤酒与尿布的故事”,揭示了美国人的某种购物习惯,并根据该特点调整布局,利润大幅提升,该过程被称作“购物篮分析”。这是数据挖掘早期在实际应用中的成功案例,也是频繁项集挖掘的起源。
现如今,人们越来越重视隐藏在海量数据下的潜在价值,数据挖掘技术不断被运用到互联网、电信、金融、商业等领域。其中,频繁项集挖掘技术在商业领域的运用成为重要的研究课题。
以下是本篇论文的主要内容:
(1)对频繁项集挖掘的有关概念以及理论做了具体的阐述;
(2)详细介绍两类最经典的频繁项集挖掘算法:apriori算法和fp-growth算法;
(3)分别实现这两个算法,详细介绍apriori如何迭代生成所有的频繁项集,fp-growth的fp-tree的构造过程以及如何通过fp-tree发掘频繁项集,根据频繁项集计算关联规则,对算法运行结果加以分析。
(4)对比分析apriori算法和fp-growth算法各自的优缺点及适用场景。
关键词:数据挖掘;Apriori算法;FP-Growth算法;购物篮分析
Abstract
With the rapid development of science and technology and the rapid improvement of storage technology, we have been living in the ocean of data, and all trades and professions are accumulating huge amounts of data. How to use these data to discover valuable information hidden in it is an important factor in the birth of data mining. In the 90s of last century, WAL-MART found the classic "story of beer and diaper" in its massive transaction data, revealing some of the Americans' shopping habits, and adjusting their distribution according to the characteristics. The profit was greatly improved. The process was called "shopping basket analysis". This is a successful case in the early application of data mining, and also the origin of frequent itemsets mining.
Nowadays, people pay more and more attention to the potential value hidden under mass data. Data mining technology has been used in the fields of Internet, telecommunications, finance, commerce and so on. Among them, the application of frequent itemsets mining technology in the commercial field has become an important research topic.
The following is the main content of this paper:
(1) the concepts and theories of frequent itemsets mining are elaborated.
(2) introduce two kinds of classic frequent itemsets mining algorithm: Apriori algorithm and FP-growth algorithm.
(3) the two algorithms are implemented in detail, in detail, how Apriori iteratively generates all the frequent itemsets, the construction process of FP-growth FP-tree and how to discover frequent itemsets through FP-tree, and calculate the association rules according to the frequent itemsets, and analyze the results of the algorithm.
(4) comparative analysis of the advantages and disadvantages of Apriori algorithm and FP-growth algorithm and its application scenarios.
Keywords: Data mining; Apriori algorithm; FP-Growth algorithm; Shopping basket analysis
目 录
第一章 绪论 1
1.1 选题背景与研究意义 1
1.2 应用领域 1
1.3 主要研究内容 2
1.4 论文组织结构 2
第二章 理论基础 4
2.1 数据挖掘 4
2.2 频繁项集 5
2.3 关联规则 5
2.4 开发工具及语言 6
2.5 数据准备及预处理 6
第三章 算法实现 8
3.1 Apriori算法 8
3.1.1 Apriori算法简介 8
3.1.2 Apriori算法原理 8
3.1.3 Apriori算法优缺点 12
3.2 Fp-growth算法 12
3.2.1 Fp-growth算法简介 12
3.2.2 Fp-growth算法原理 13
3.2.3 Fp-growth算法优缺点 18
第四章 挖掘关联规则 20
4.1 计算关联规则 20
4.2 结果分析 22
4.3 算法对比 25
第五章 总结与展望 26
5.1 总结 26
5.2 展望 26
致谢 27
参考文献 28
C{ZX$P2FAWBT~_{_@BC(`3.png)

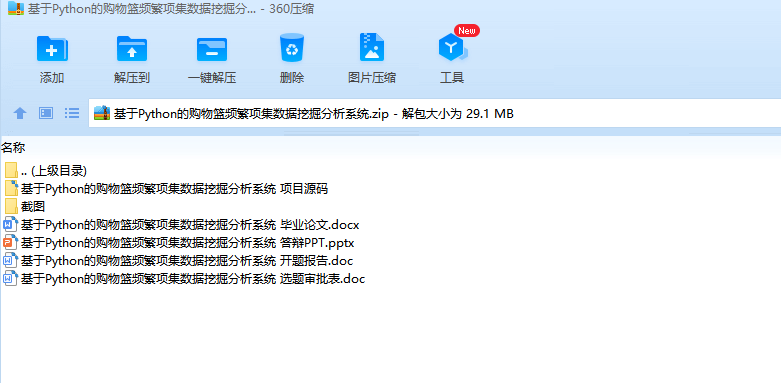
QLE$D96B4}GJZ(4D.png)
(G[~2H.png)
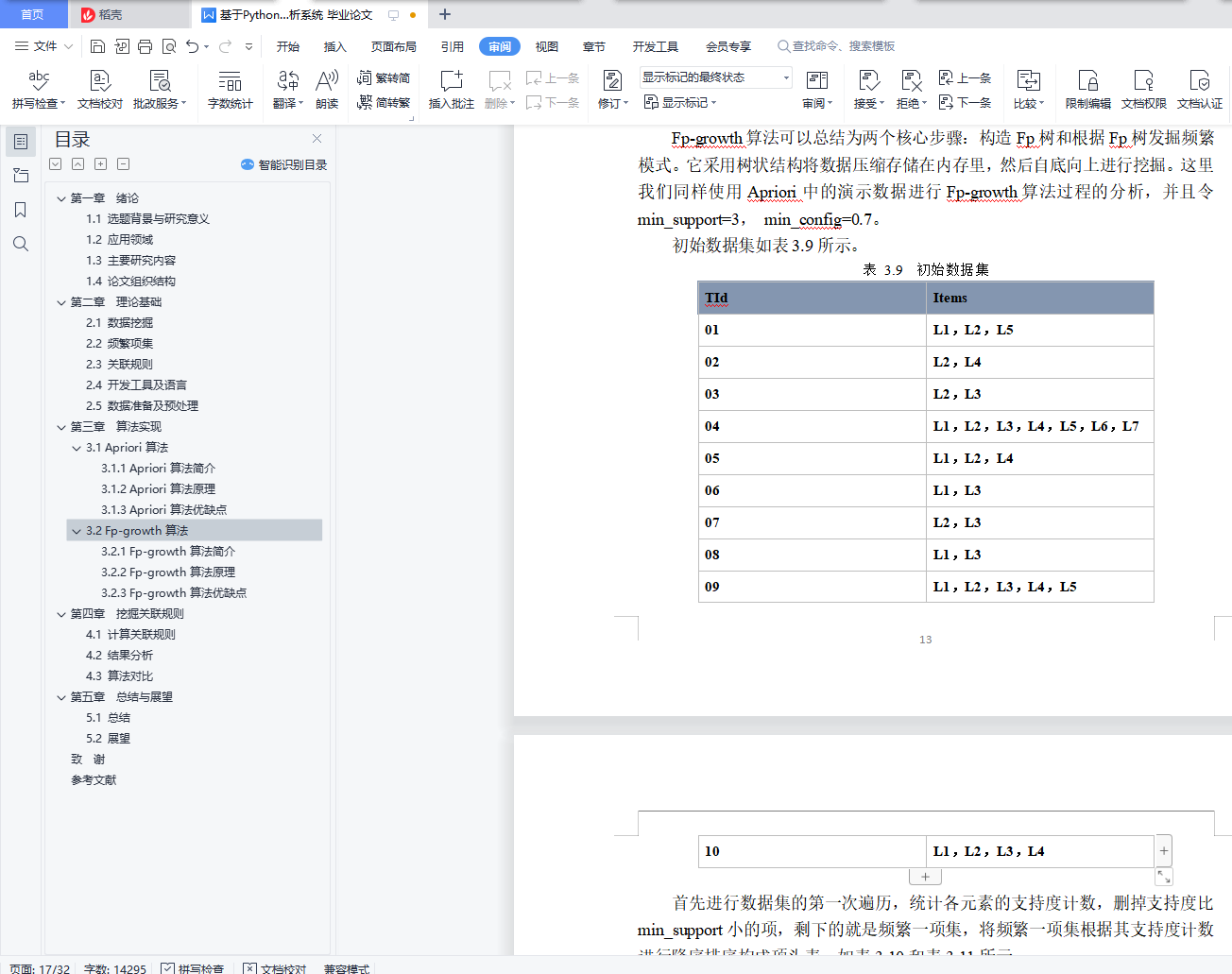
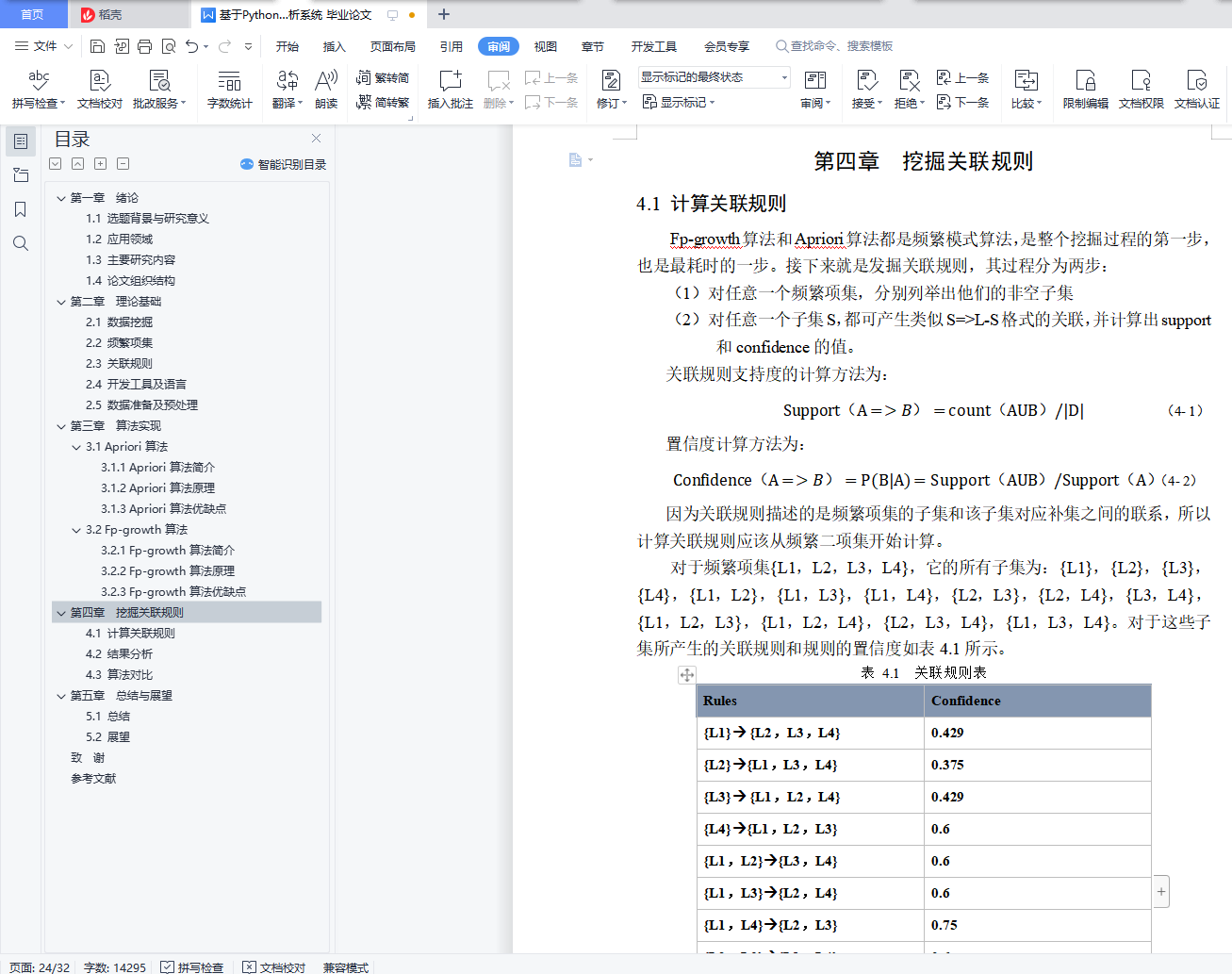
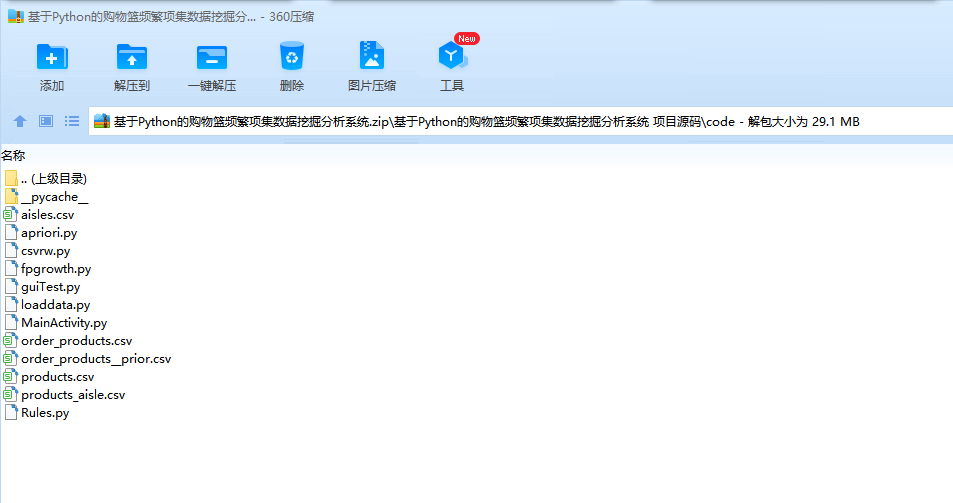
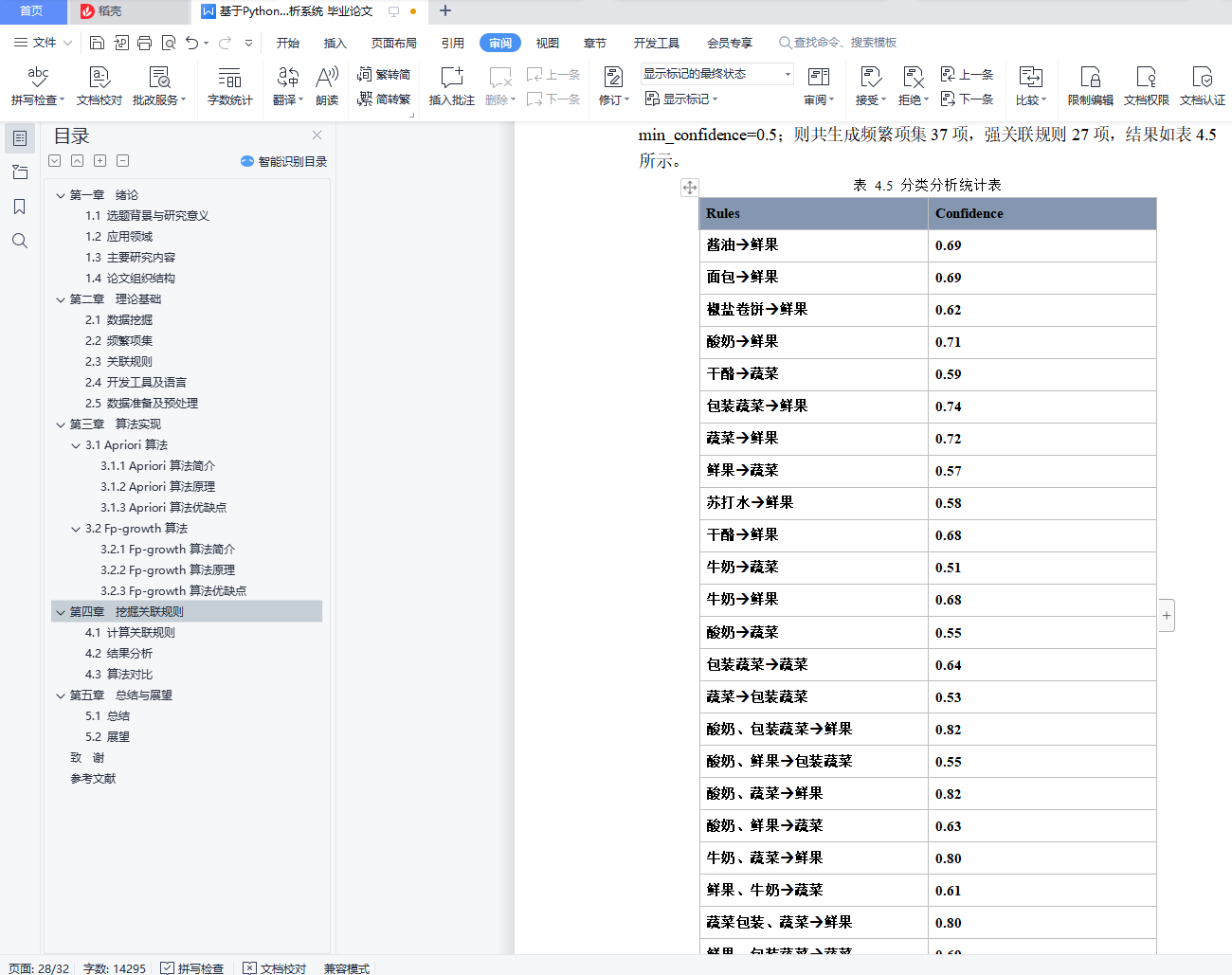
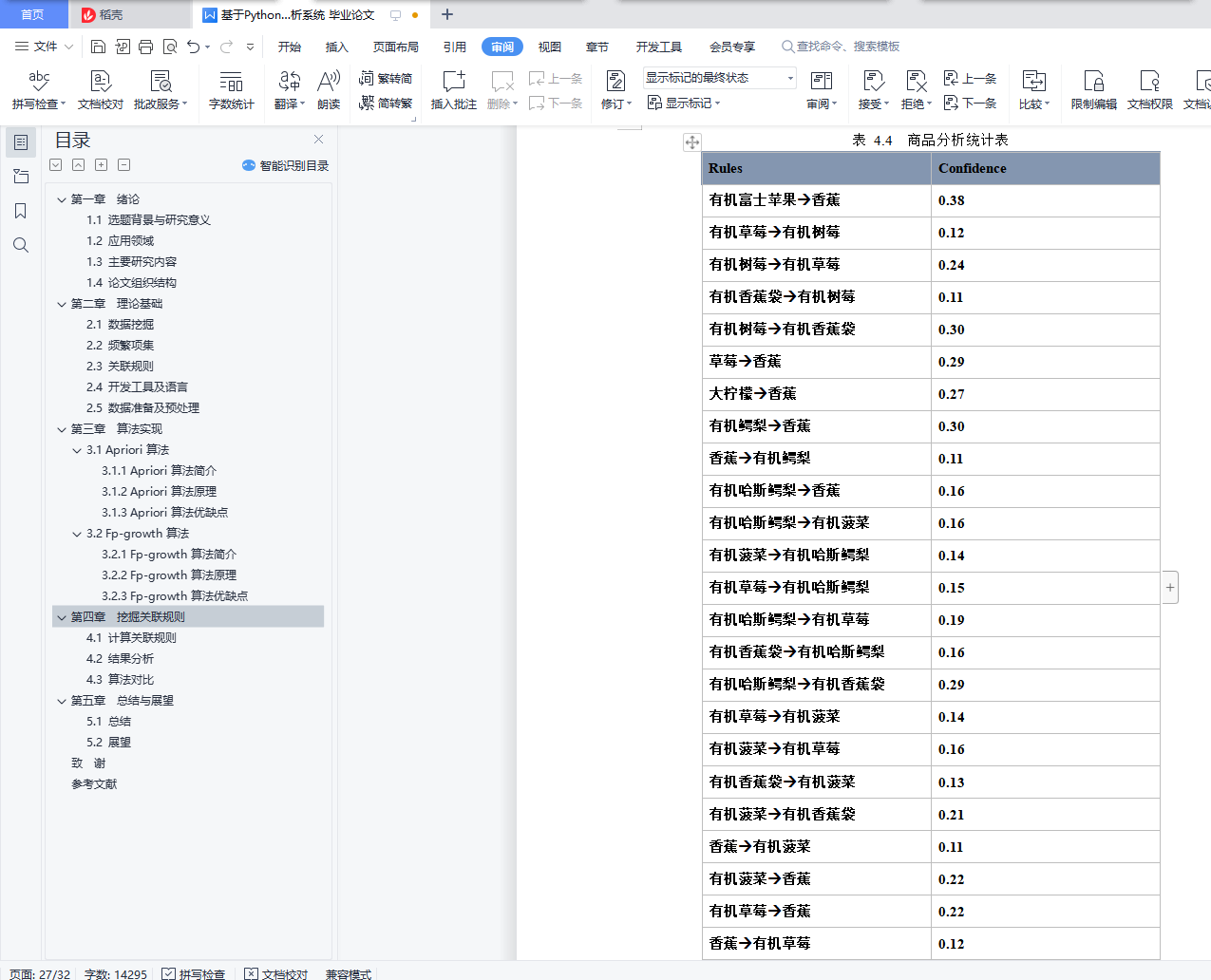
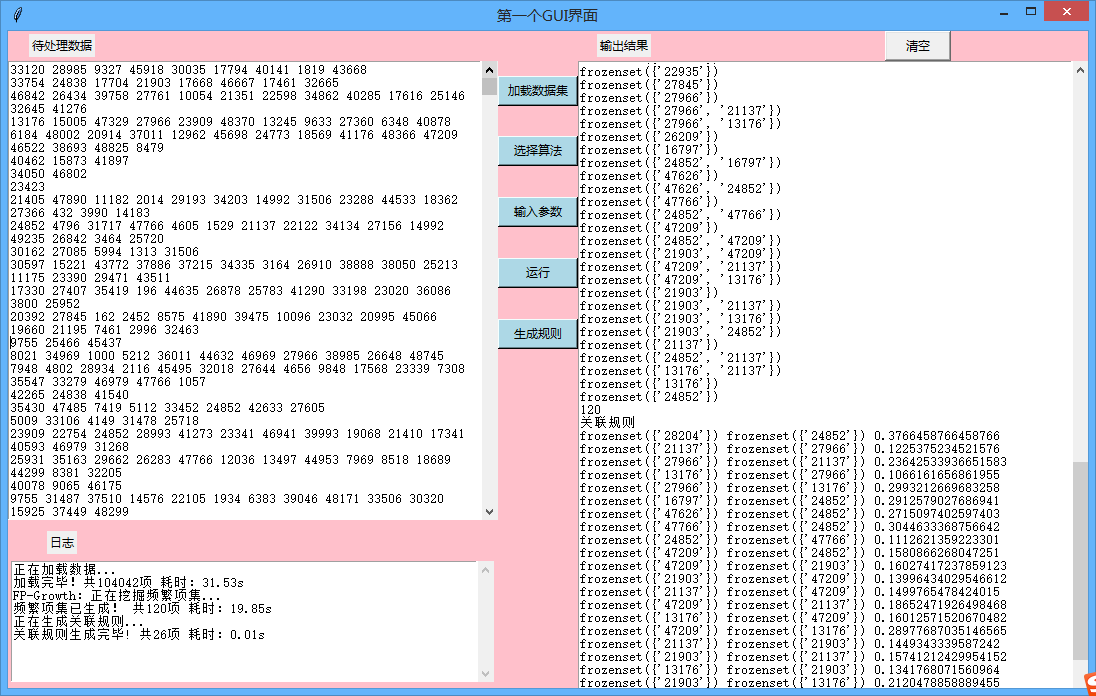
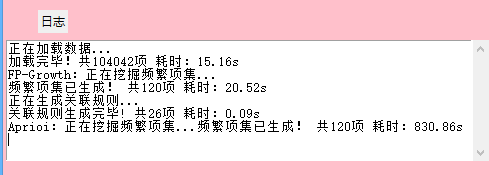
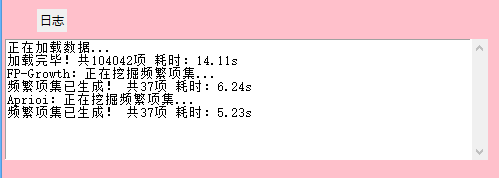