摘 要
卷积神经网络是近年来人工智能领域取得重大突破的一种重要手段,给出了图像识别、语音识别和自然语言处理领域中关键问题的优化解决方案,尤其适合处理图像方面的任务,如人脸识别和手写体识别。手写数字识别是用卷积神经网络解决的经典问题,采用一般方法训练出来的神经网络达到了97%的识别率,几乎与人类的识别精度一致,但在执行速度上没有人类识别得快。在实际商业应用中不可避免地会遇到数据量过大的问题,如在手写数字识别中有60000条规格为28*28单位像素的训练样本需要训练,这样会导致执行速度较慢,CPU在处理这样包含大量高精度浮点数的任务时,其消耗的时间是不可接受的,在训练期要花费数小时的时间。
针对上述问题,该文将构建基于CUDA架构的编程环境,采用CUDA/C++编程实现卷积神经网络算法,将卷积神经网络算法应用于手写数字识别问题中,在选择合适的网络模型和相关参数的情况下,利用GPU的高度并发性能,提高卷积神经网络训练数据的速度。通过对GPU实现和CPU实现进行对比实验,验证对卷积神经网络算法进行CUDA并行化训练和识别是可行有效的,实验表明在普通PC机上采用GPU实现的卷积神经网络算法比CPU实现的卷积神经网络算法虽然在准确率上仅提升了0.29%,但在速度上加快了15%。
关键词:CUDA,卷积神经网络,深度学习,并行计算
ABSTRACT
Convolutional neural network is a major breakthrough in the field of artificial intelligence in recent years, an important means of image recognition given to optimize speech recognition and natural language processing solutions, especially for process images tasks, such as human face recognition and handwriting recognition. Handwritten numeral recognition is a classic problem with the convolutional neural network to solve, using the general method of trained neural network reaches 97% recognition rate, with almost the same human recognition accuracy, but the speed of execution without human recognition quickly. In practical commercial applications will inevitably encounter the problem of data overload, as there are 60,000 training sample size is 28 * 28 pixel units need to be trained in digital handwriting recognition, this will result in slower performance, CPU in when processing tasks such contains a lot of high-precision floating-point number, the elapsed time is unacceptable, in the training period to spend a few hours.
To solve these problems, this paper will build a CUDA architecture-based programming environment using CUDA / C ++ programming to implement the convolution neural network algorithm. The convolution neural network algorithm is applied to handwritten numeral recognition problem. After selecting an appropriate network model and related parameters, the use of highly concurrent GPU performance, convolution neural network training to improve the speed of data. Then use the model to achieve the GPU and CPU to complete some comparative tests to verify the fact that using the CUDA to parallel implementing convolution neural network algorithm for training and recognition is feasible and effective, experiments show that on the common PC the neural network algorithm convolution convolution neural network algorithm to achieve GPU than CPU implementations on the speed by 15%, on the accuracy improved 0.29%.
Key words:CUDA, CNN, Deep Learning, Parallel Computing
目 录
摘 要
ABSTRACT
目 录
1 绪论
1.1 深度学习的发展和现状
1.2 研究意义
2 人工神经网络和卷积神经网络的结构及算法
2.1 人工神经网络
2.1.1 神经元
2.1.2 多层前馈网络
2.1.3 BP算法
2.2 卷积神经网络
2.2.1 局部连接与权值共享
2.2.2 网络结构
2.2.3 卷积层
2.2.4 采样层
3 CUDA平台介绍
3.1 CUDA简介
3.2 CUDA编程
3.2.1 CUDA的执行模型
3.2.2 常用函数及用法
3.3 CUDA开发环境搭建
4 数据方阵化处理及网络模型设计
4.1 数据方阵化
4.1.1 手写数字数据库MNIST介绍
4.1.1 手写数字数据库MNIST的数据方阵化
4.2 网络模型设计
4.2.1 网络结构
4.2.2 权重初始化方式
4.2.3 激活函数的选择
4.2.4 实验总结
5 卷积神经网络并行化设计及实现
5.1 基于CUDA的卷积神经网络的训练过程
5.1.1 网络初始化
5.1.2 网络前向传播过程
5.1.3 网络的反向传播过程
5.1.4 网络的权值和偏置更新过程
5.1.5 保存训练结果
5.2 并行化分析及实现
5.2.1 并行化分析
5.2.2梯度下降算法的实现
5.2.3 全连接层的前馈和反馈实现
5.2.4 Softmax回归算法的实现
5.3 对比实验
6 结论
致 谢
参 考 文 献
X9LNO5}1O]1T74PGT@X5FF.png)
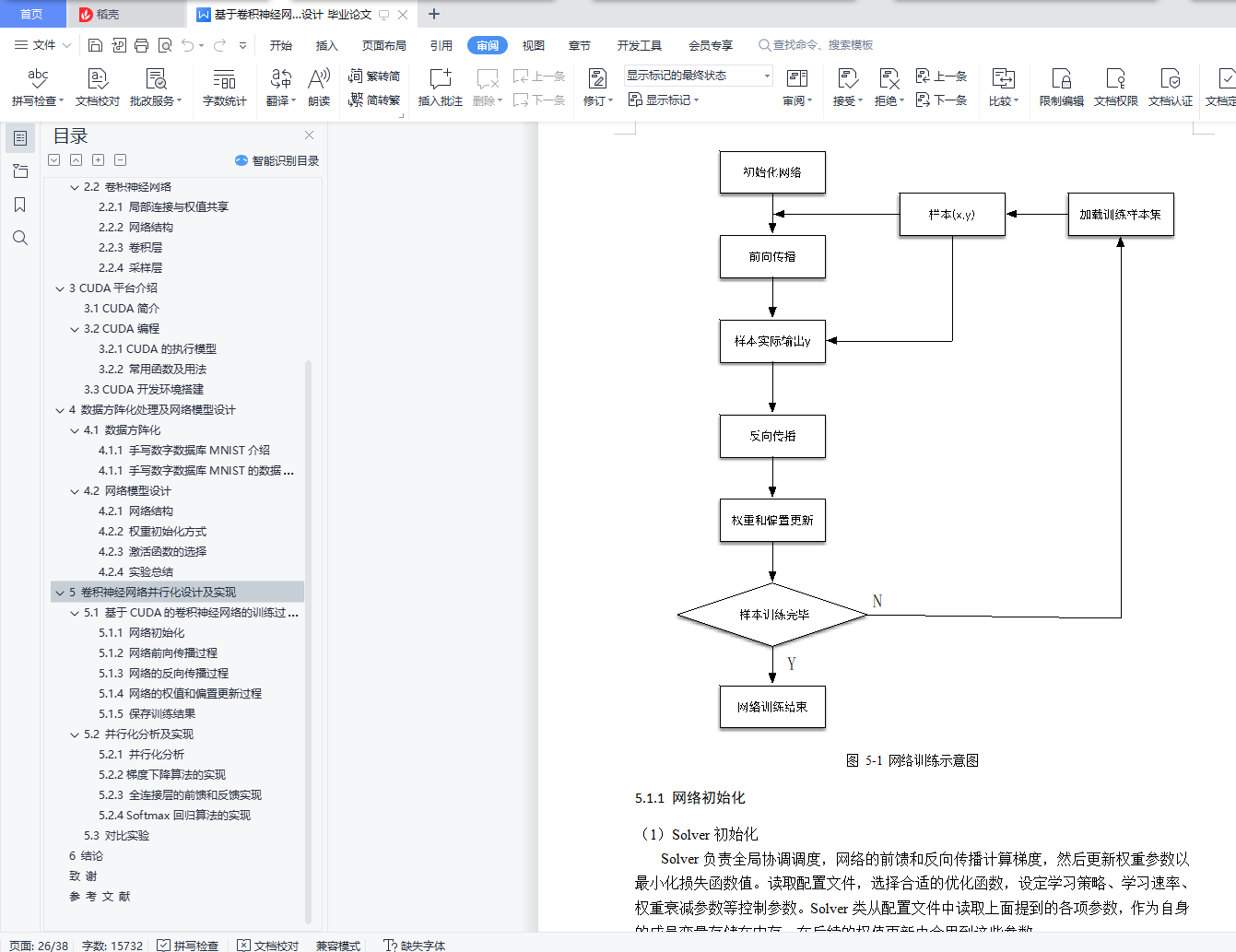
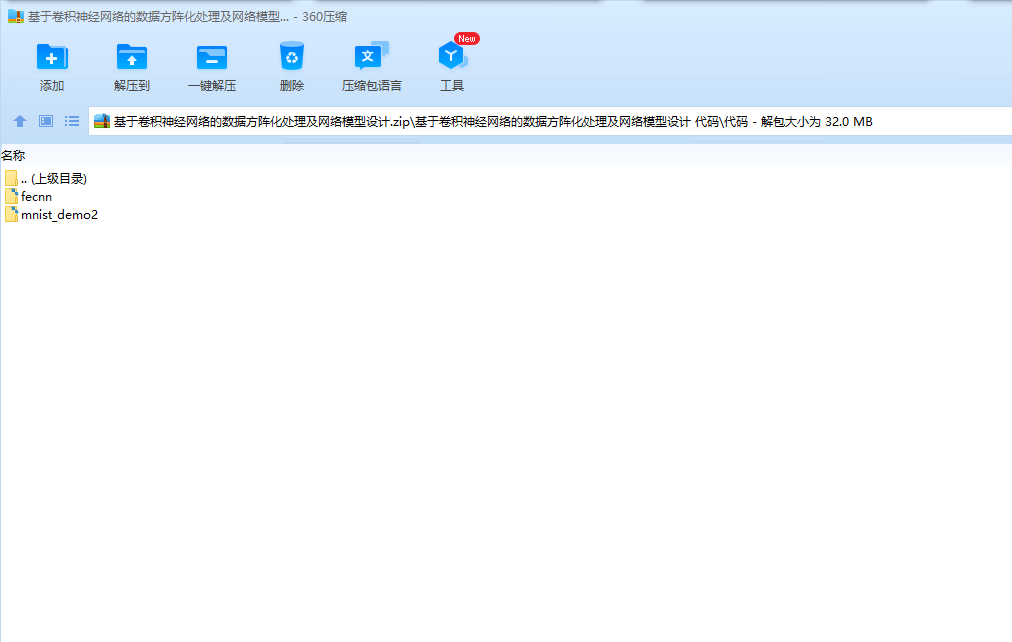
]MNZQU@M7R8.png)
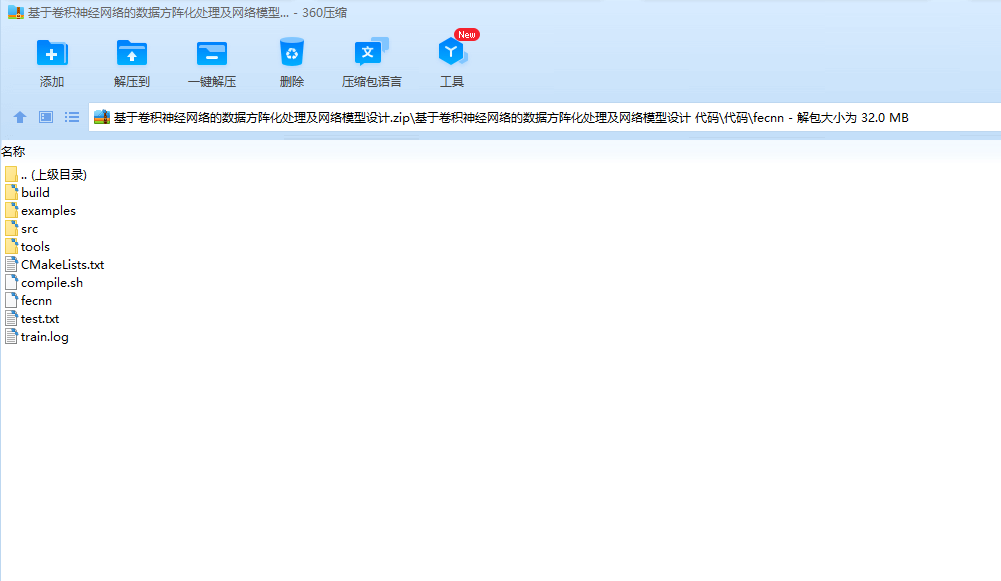
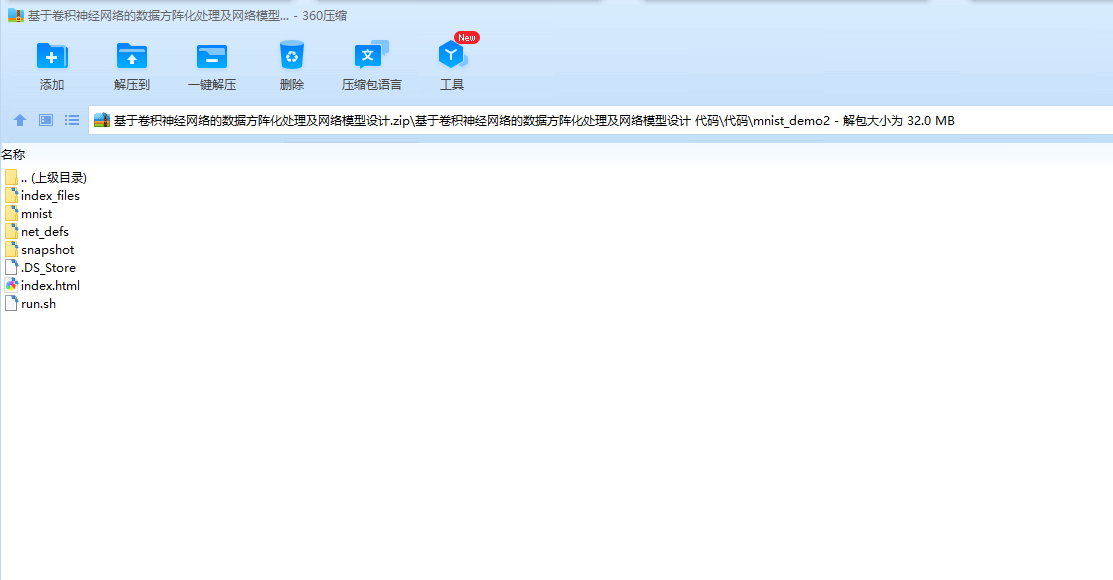
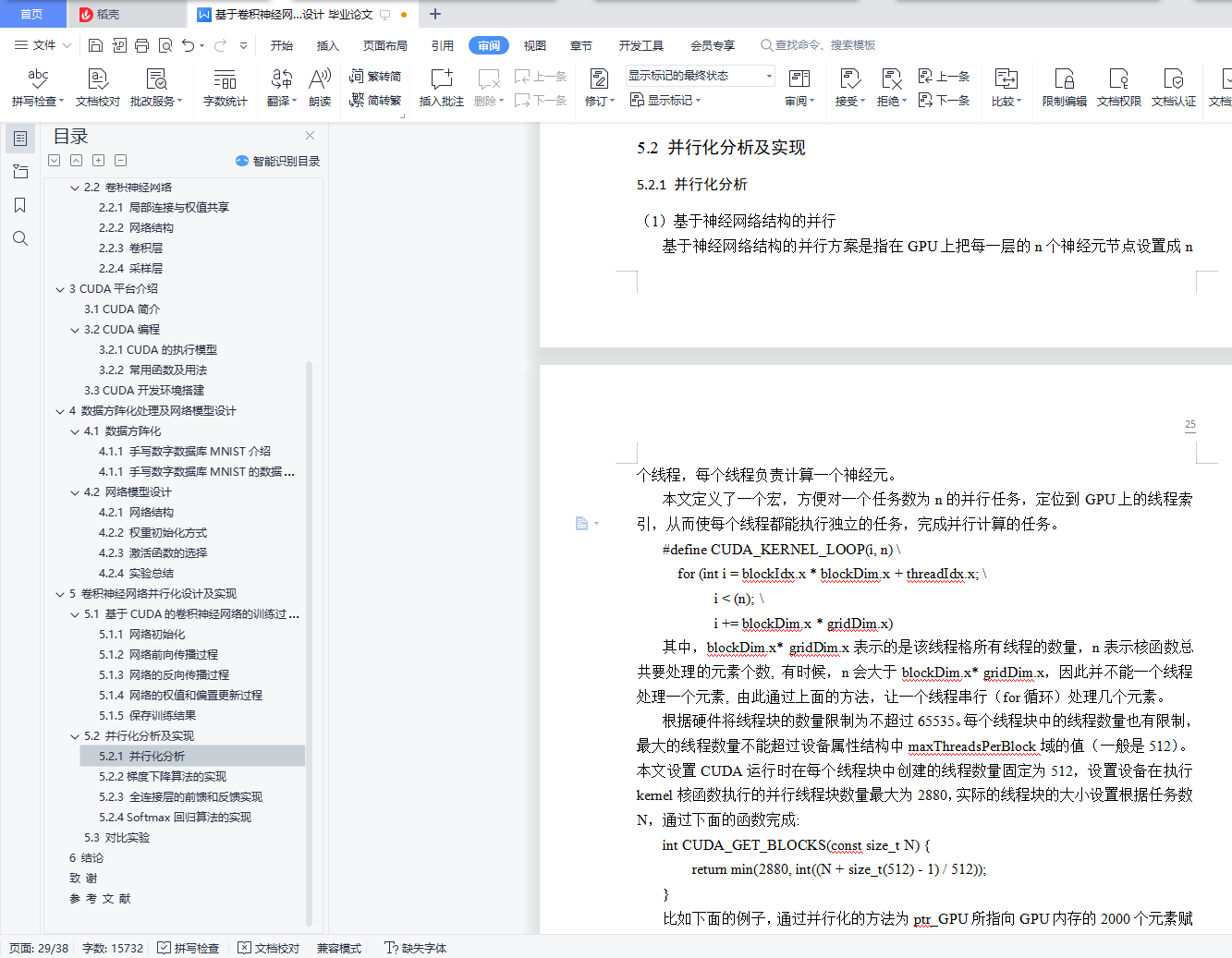
$}]K.png)
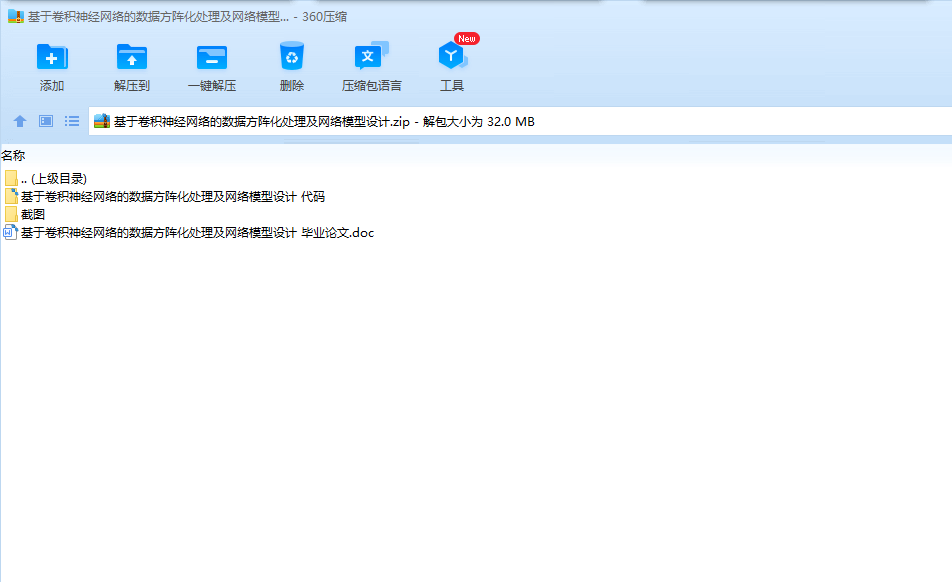



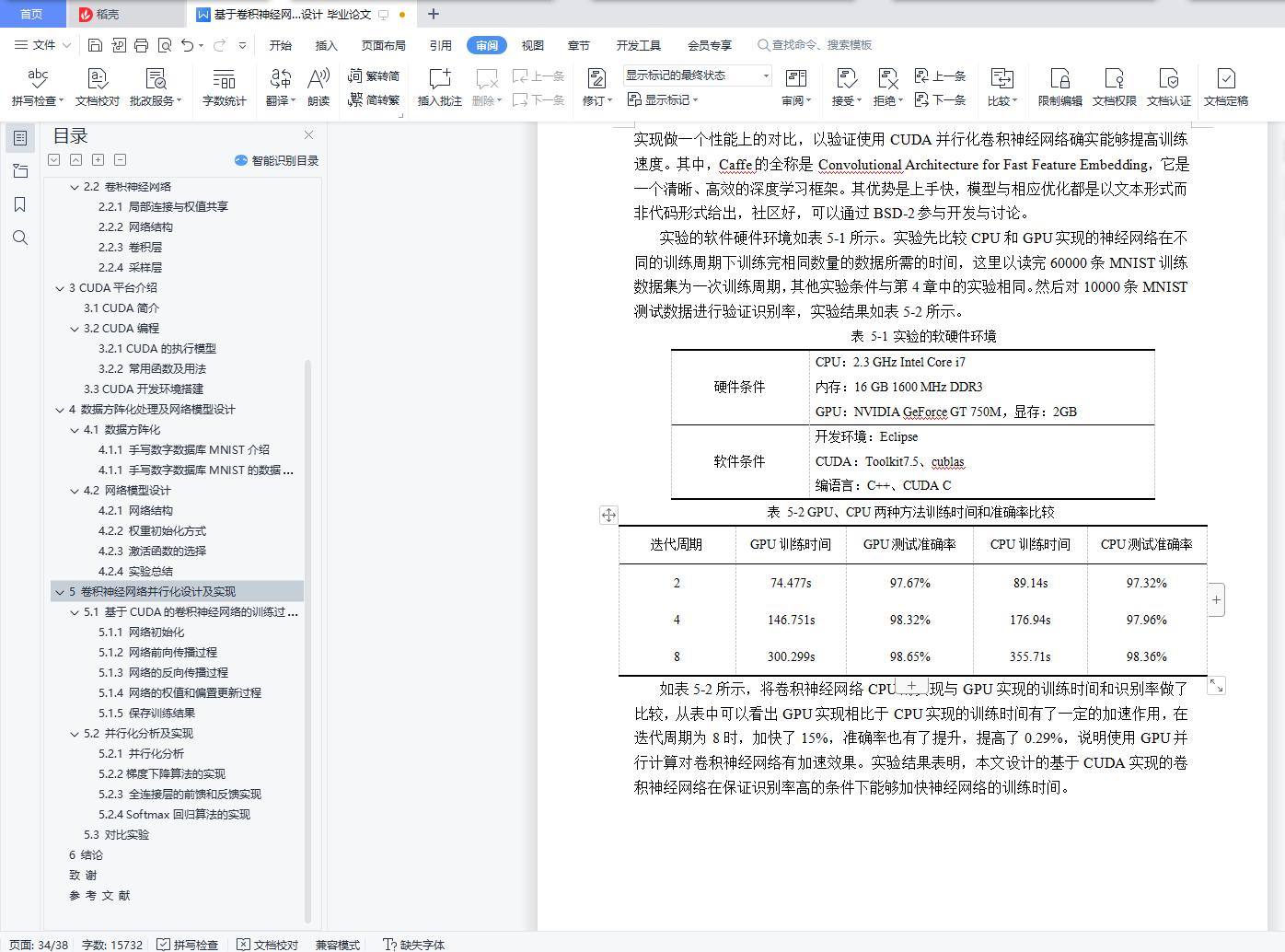
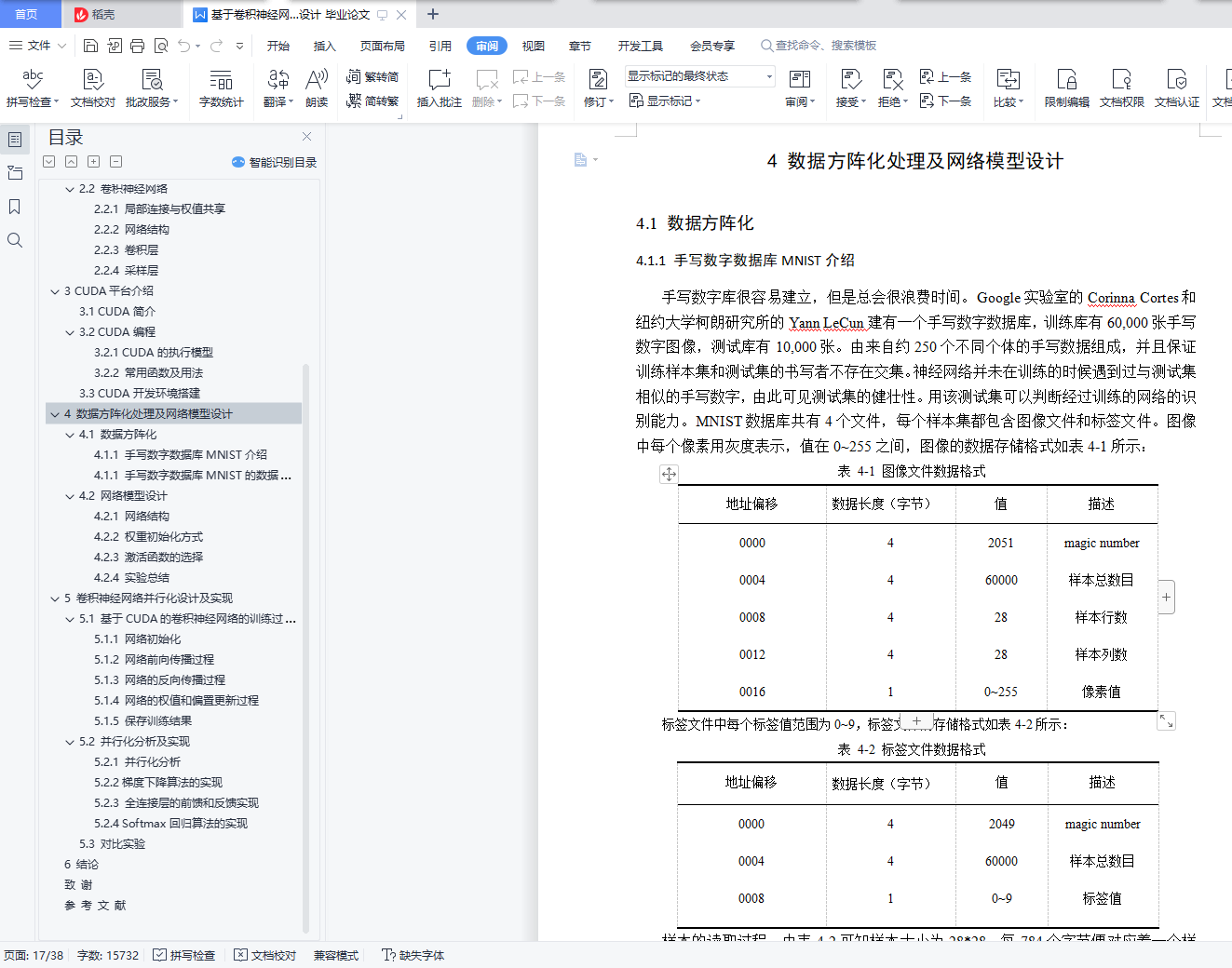
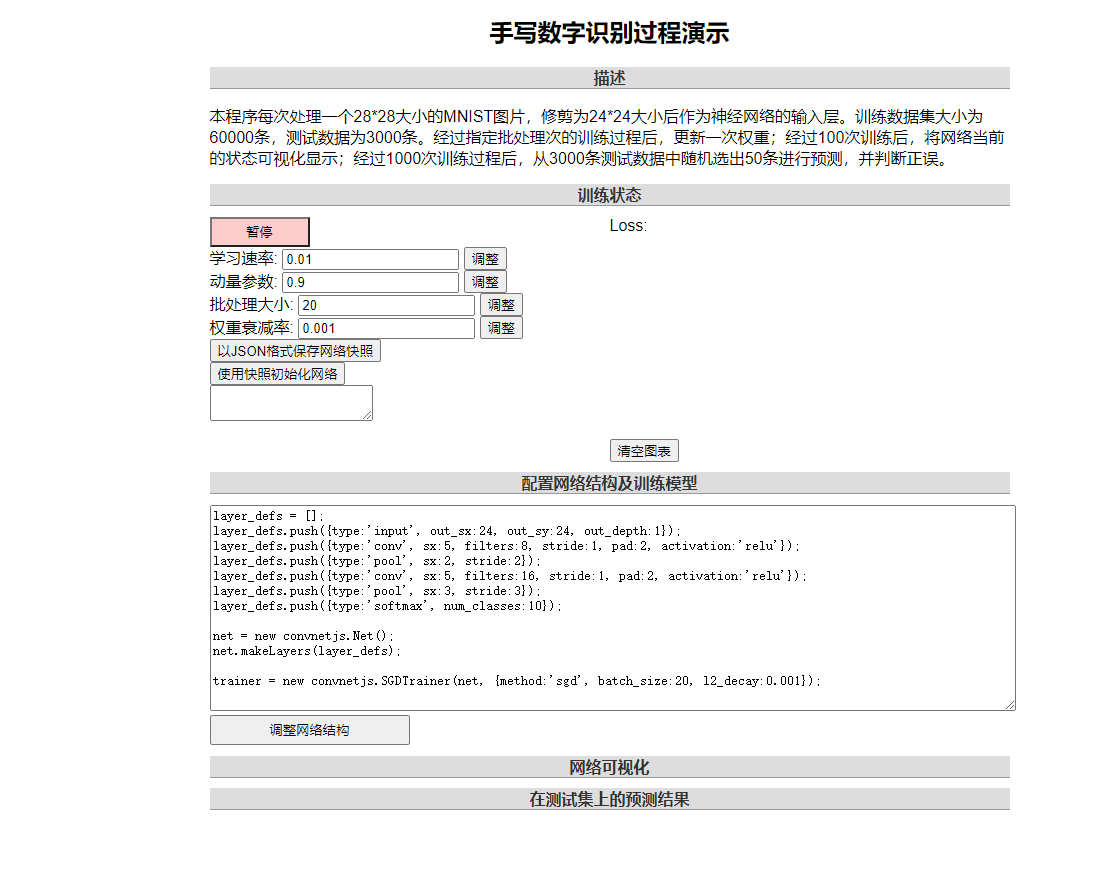