目 录
摘 要 I
ABSTRACT II
第一章 绪论 1
1.1 课题简介及目的 1
1.2 课题研究意义 2
1.3 研究现状和发展趋势 2
1.4 本文的结构安排 3
第二章 算法的开发环境简介 4
2.1 Python 4
2.2 Ubuntu 4
2.3 Visual Studio Code 4
2.4 ROCm GPU加速器 5
2.5 TensorFlow-ROCm 5
2.6 Keras 5
2.7 Scipy 5
第三章 算法所需技术介绍 6
3.1 卷积神经网络(CNN) 6
3.2 生成对抗网络(GAN) 7
3.3 Pix2pix模型 11
3.4 CycleGAN网络 14
第四章 算法设计分析 17
4.1 算法的数学实现 18
4.2 神经网络架构 19
4.2.1 生成网络G 19
4.2.2 判别网络 D 20
4.2.3 优化和推理 21
第五章 算法的实现与测试 22
5.1 开发环境配置 22
5.1.1 基本模型 22
5.1.2 Python 22
5.1.3 安装Visual Studio Code 22
5.1.4 配置ROCm GPU加速器 23
5.1.5 配置Tensorflow-GPU,Keras,Scipy 24
5.2 算法实现与验证 26
5.2.1 输入管线 26
5.2.2 导入并重用 Pix2pix 模型 29
5.2.3 损失函数 31
5.2.4 模型训练 33
第六章 总结与未来展望 38
参考文献 39
致谢 40
摘 要
特效图片被广泛应用于电影电视剧的制作以及个性化照片的生成。现有的图像特效制作软件,如PhotoShop、美图秀秀、LightRoom等,都是基于人工修改,而且这些软件通常比较复杂,学习使用常常需要较长周期,很难满足紧急的特效图片制作需求。而图像合成算法虽然可以实现自动的图像到图像的翻译,形成特效图片,但往往需要成对的训练数据(如Pix2pix模型),而这样的数据通常难以获得。
本文针对上述问题,基于几种图像合成算法,提出一种可以不使用成对的训练数据即可自动生成特效图片的算法。该算法基于CycleGAN算法改进而来,通过使用对抗神经网络GAN,比较了风格迁移、物体变形、季节转换、照片增强等工作状况下的神经网络的结果。实现了特效图片的生成,经过比较,该神经网络模型在无成对训练数据的情况下比Pix2pix模型表现的更好。
关键词:CycleGAN 深度学习 特效制作 Pix2pix 对抗神经网络
ABSTRACT
Special effect pictures are widely used in the production of movies and TV series as well as the generation of personalized photos. Existing software for producing special-effect images, such as Photoshop, Meitu XiuXiu and Lightroom, are all based on manual modification. These software are usually complex to learn, and takes long time to master. It is difficult to meet some urgent needs of special-effect image production. Although image synthesis algorithms can realize special-effect images production through image-to-image translation, it often needs paired training data, which is usually difficult to obtain.
Aiming at the above problems, based on several image synthesis algorithms, this paper proposes an improved CycleGAN algorithm that can automatically generate special-effect pictures without using paired training data. By using Generative Adversarial Network GAN, the results of neural network under the working conditions of style transfer, object deformation, seasonal transformation, photo enhancement are compared. After comparison, the neural network model performs better than pix2pix model in the absence of paired training data.
Key words: CycleGAN Deep learning Image generation Pix2pix Adversarial Nets
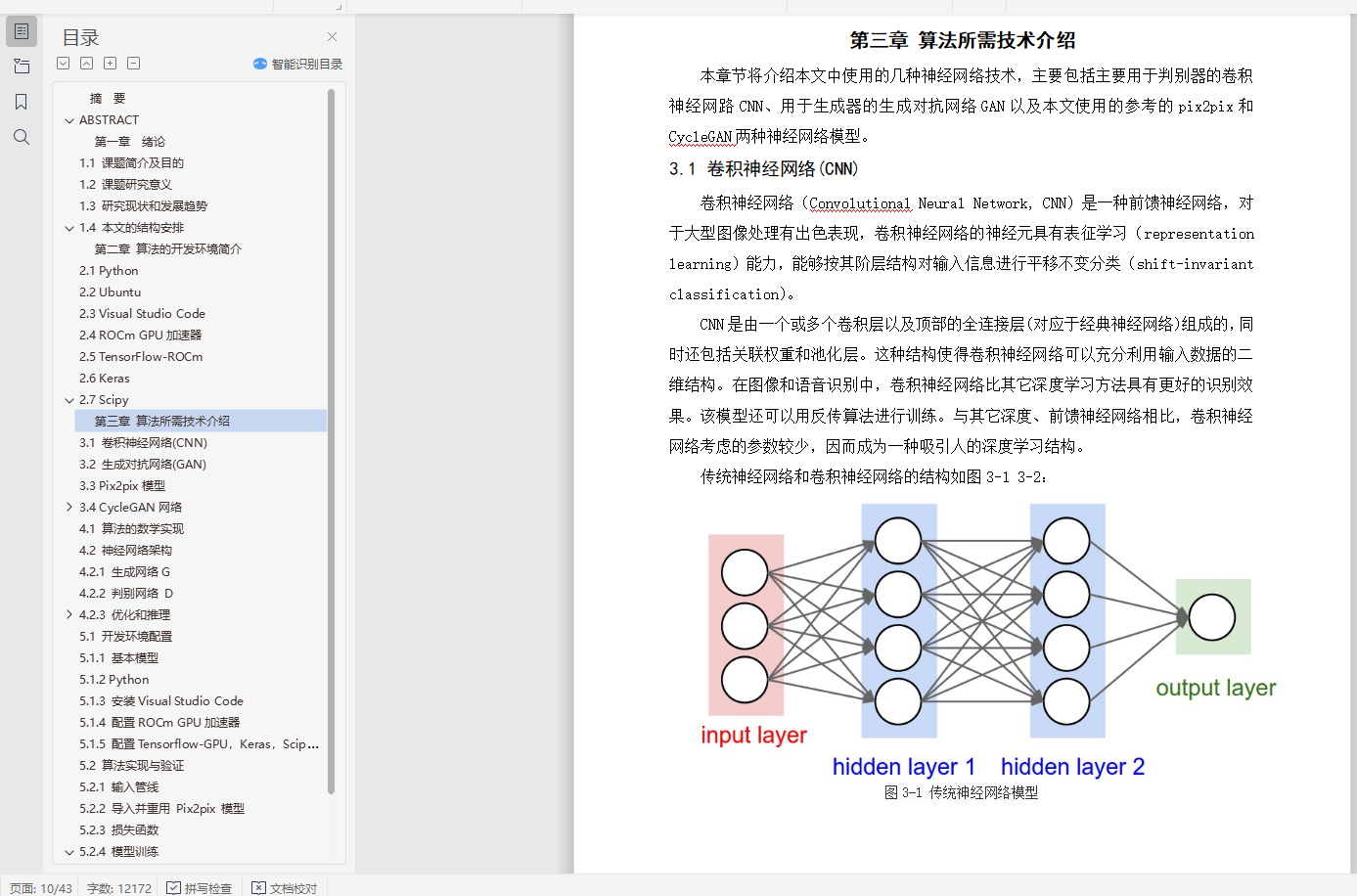
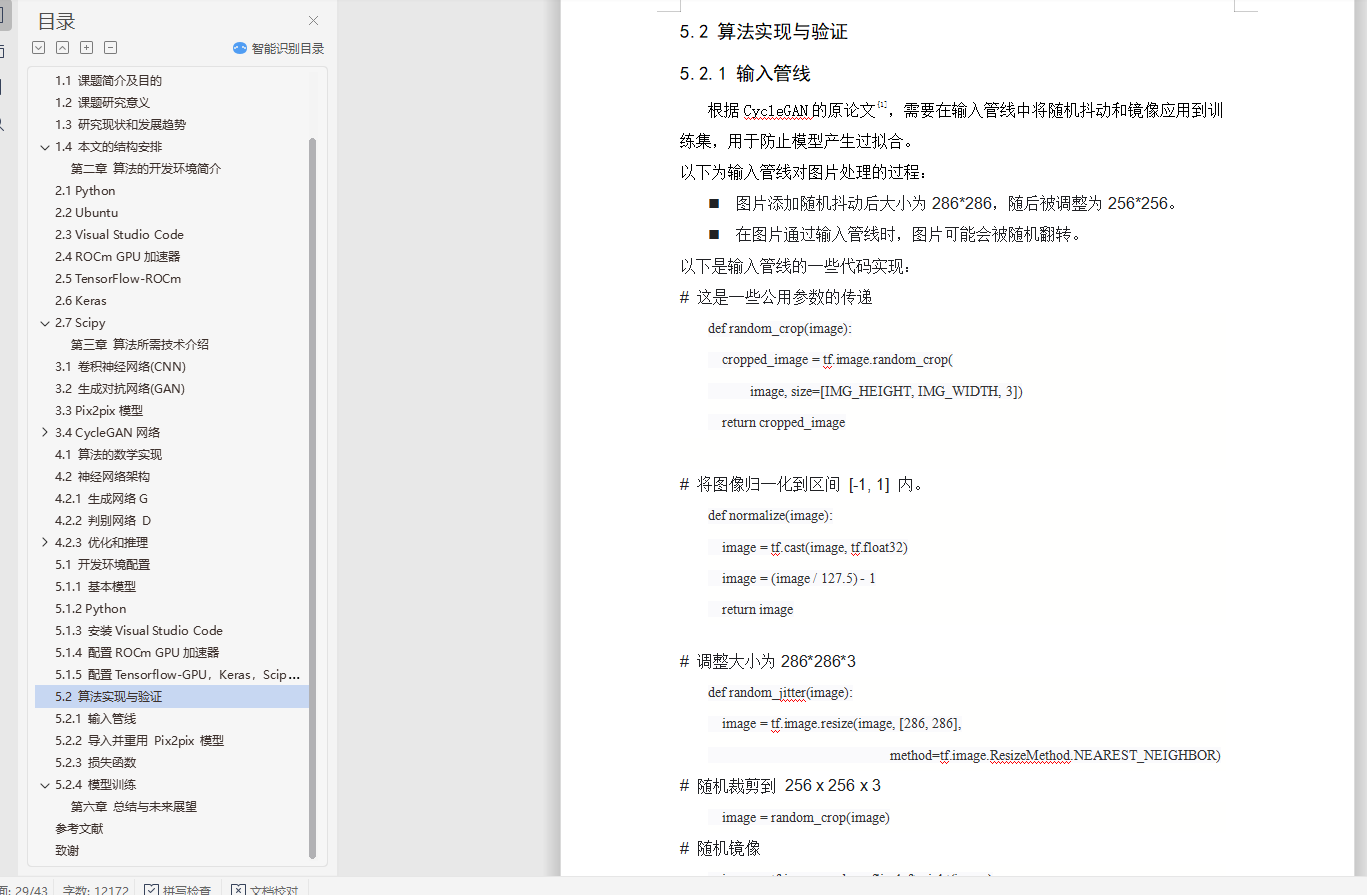
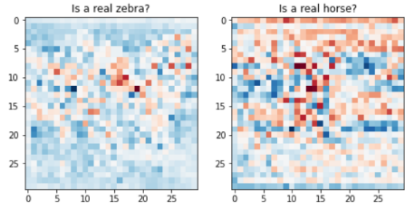
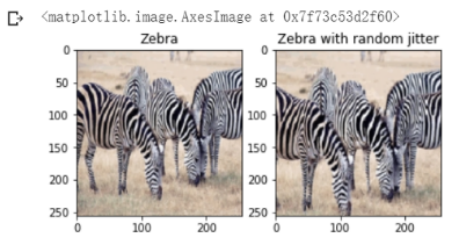
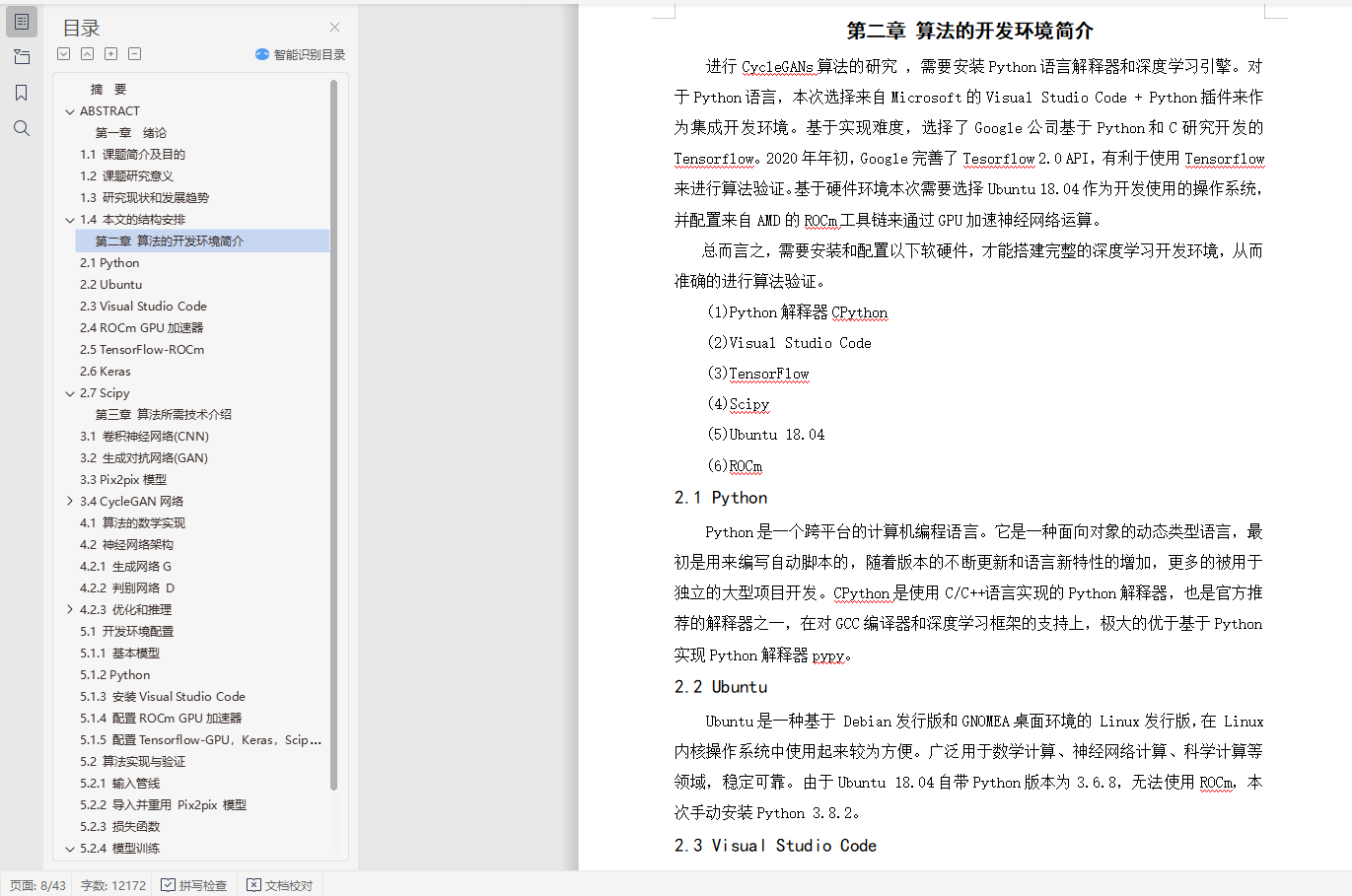
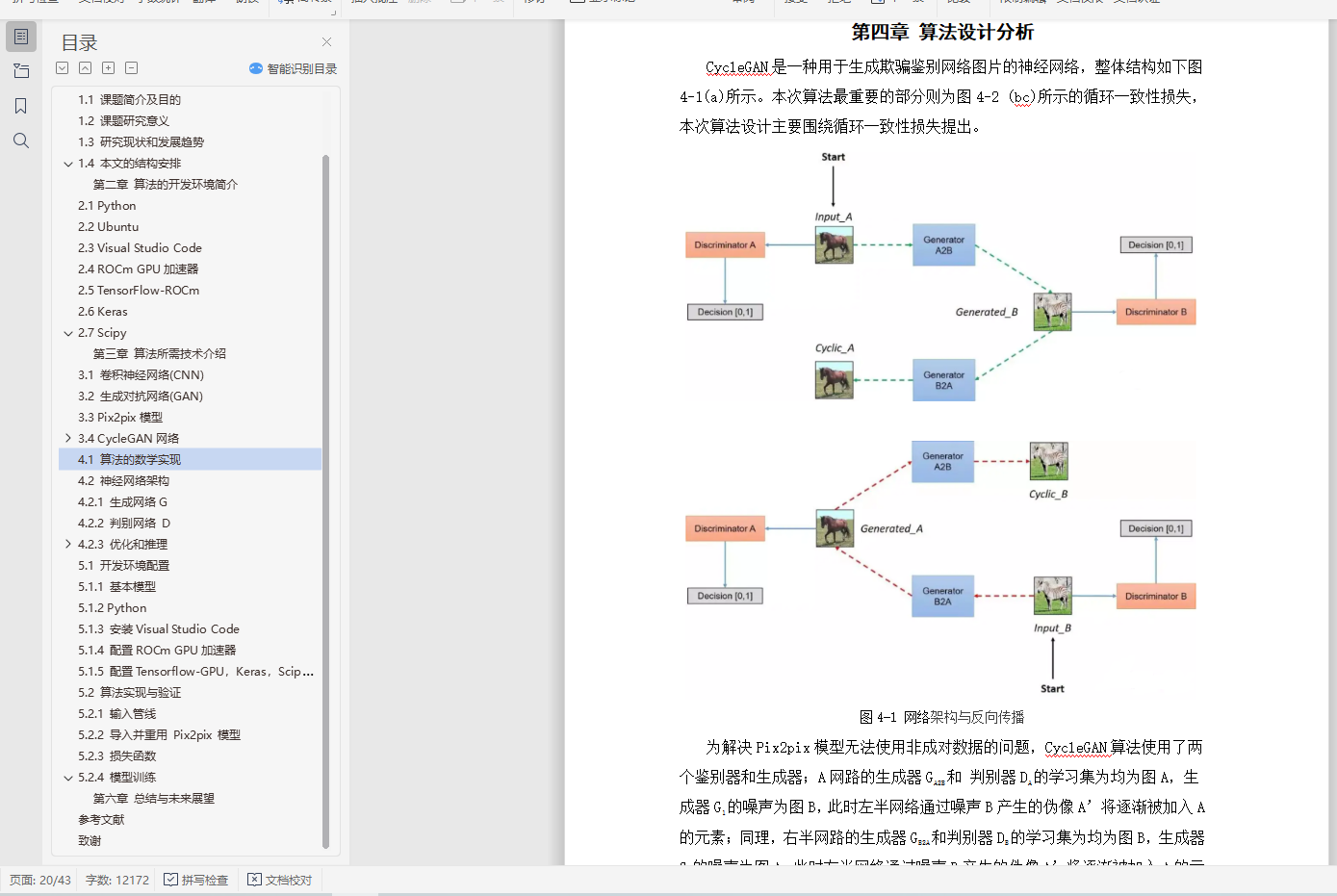
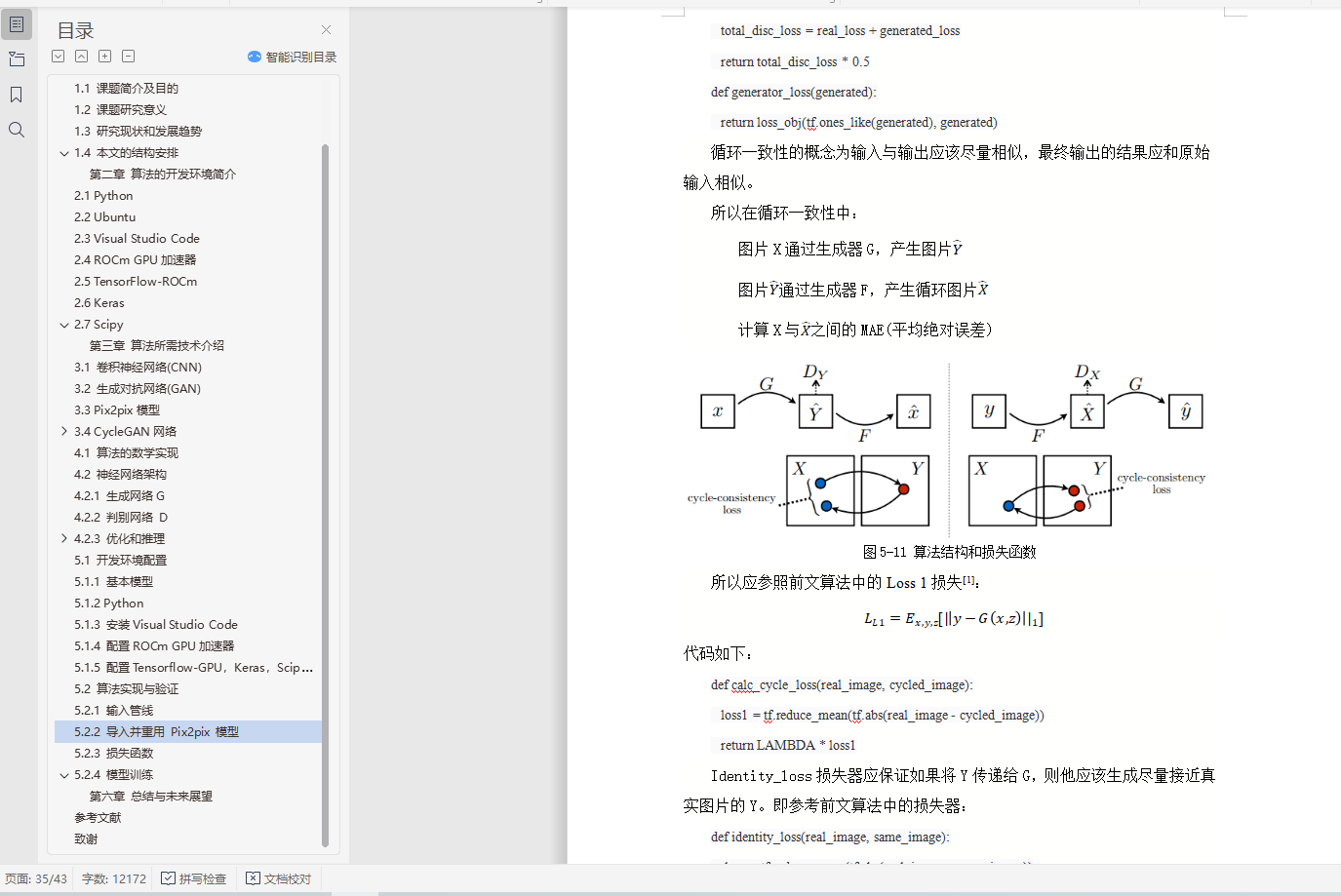
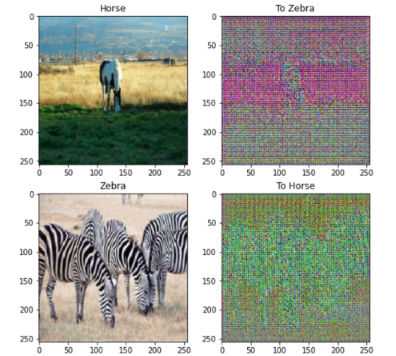
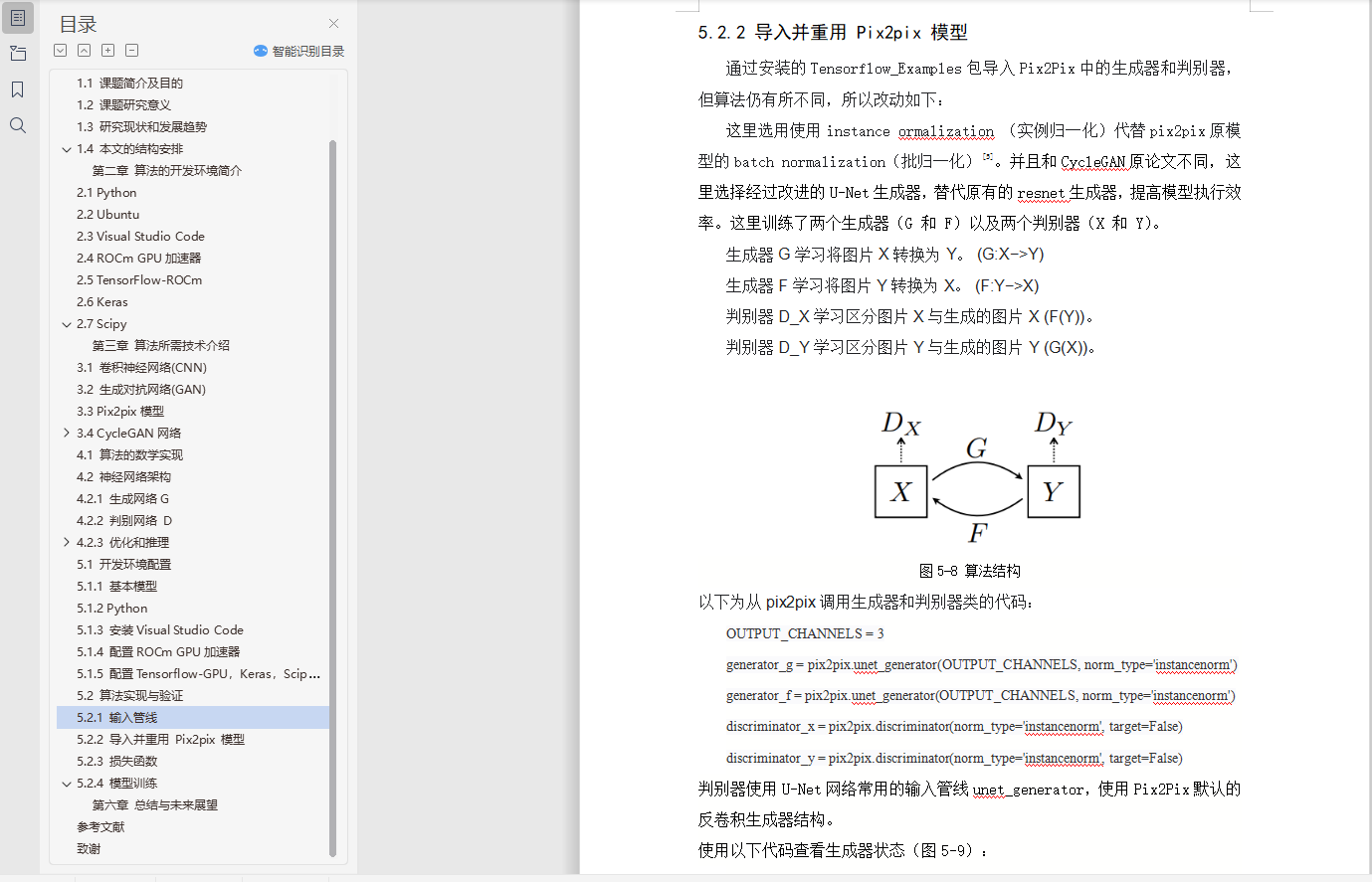
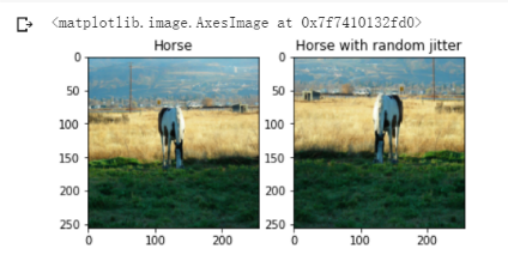
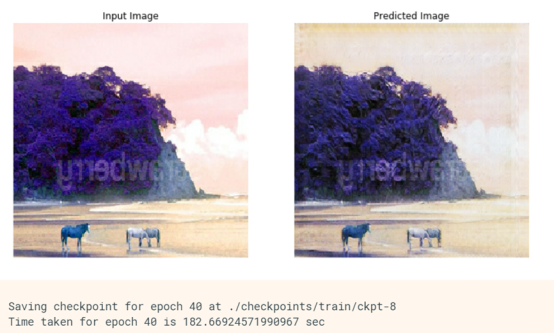
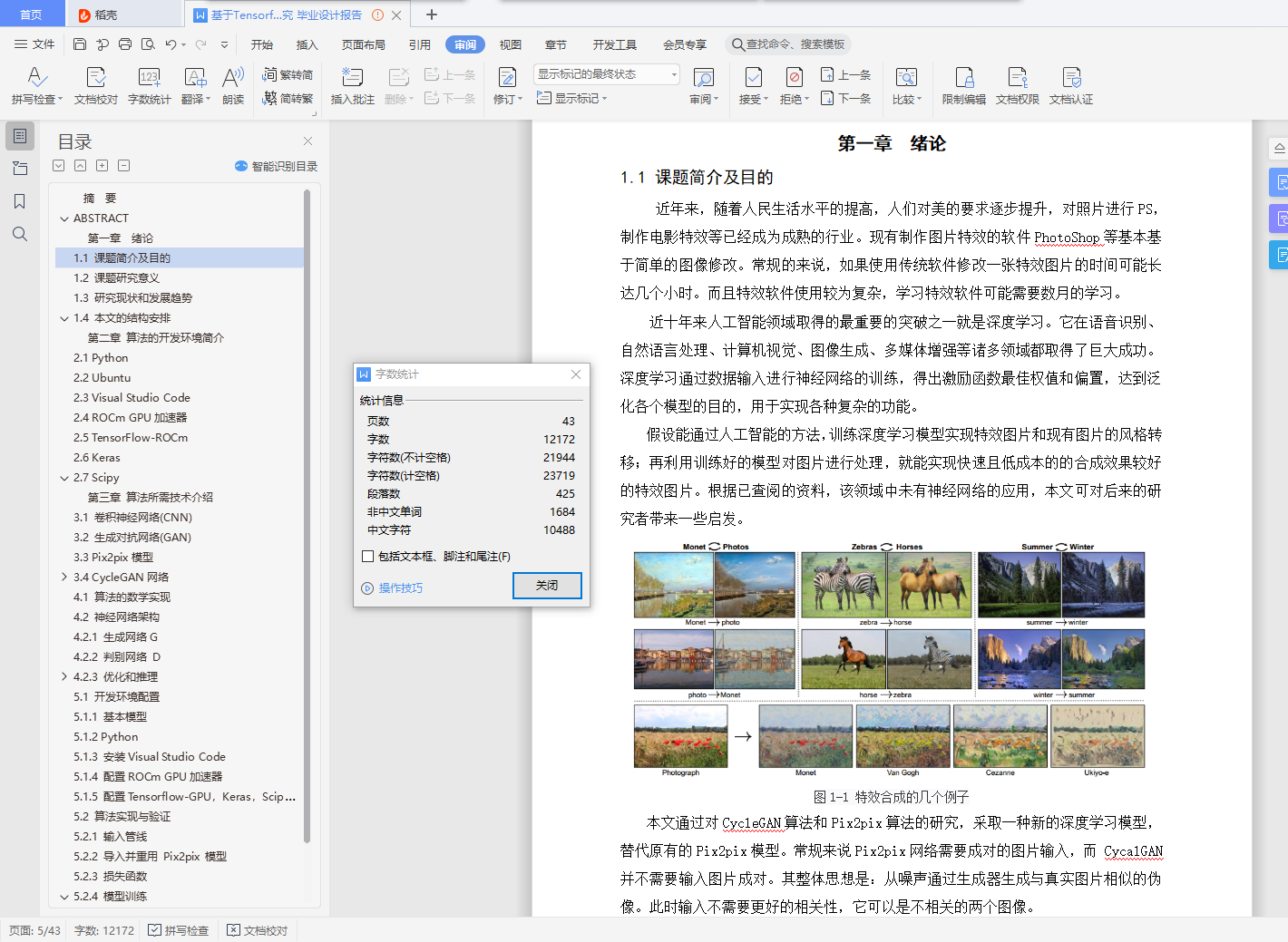
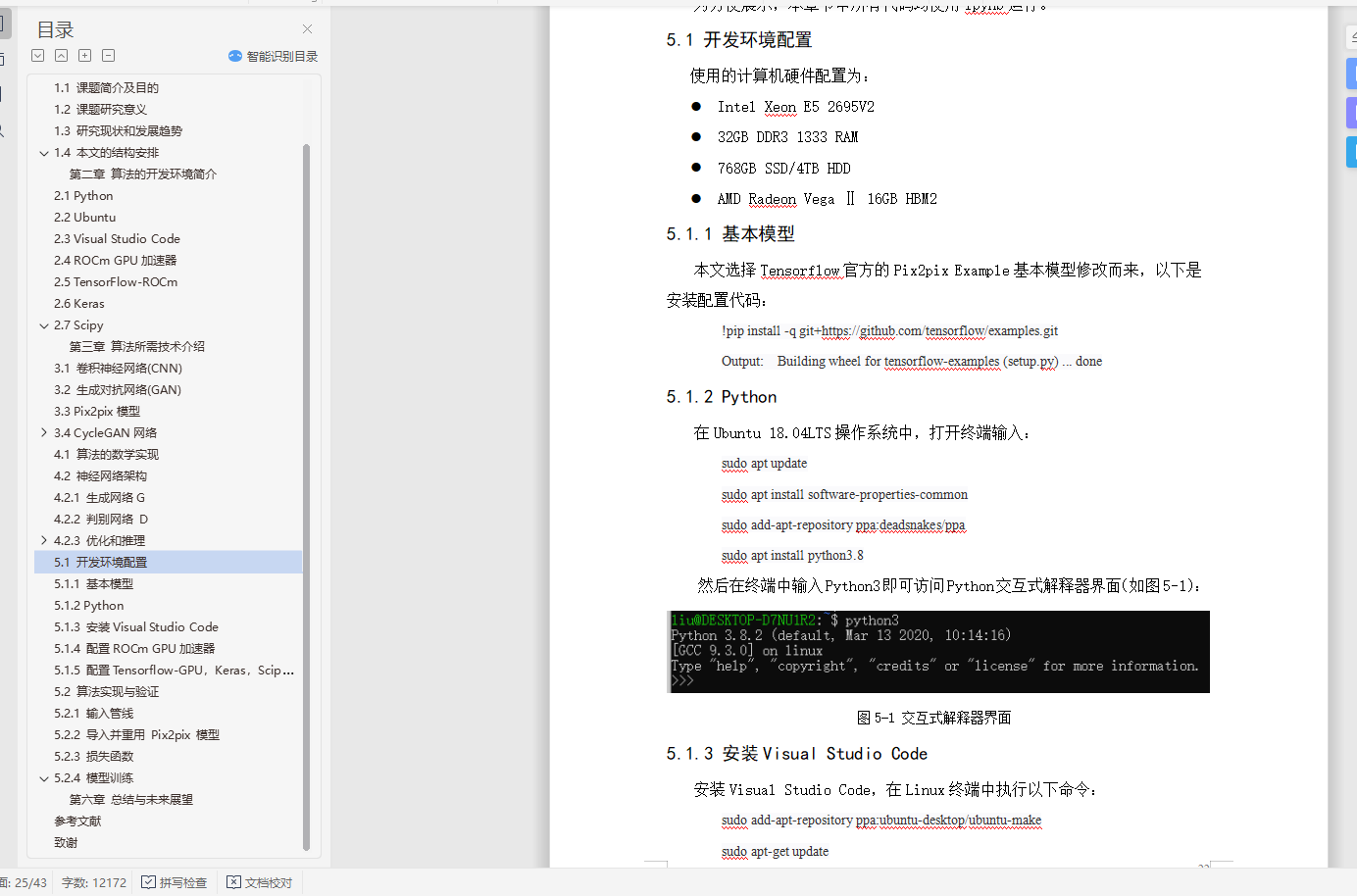
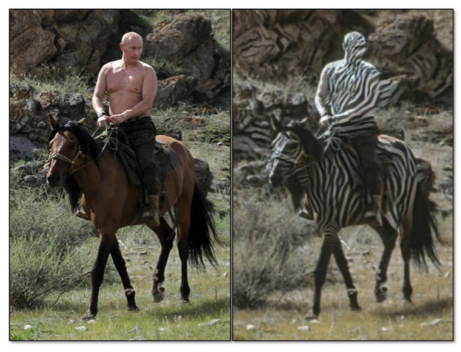